Isolating Red Flags to Enhance Diagnosis i RED
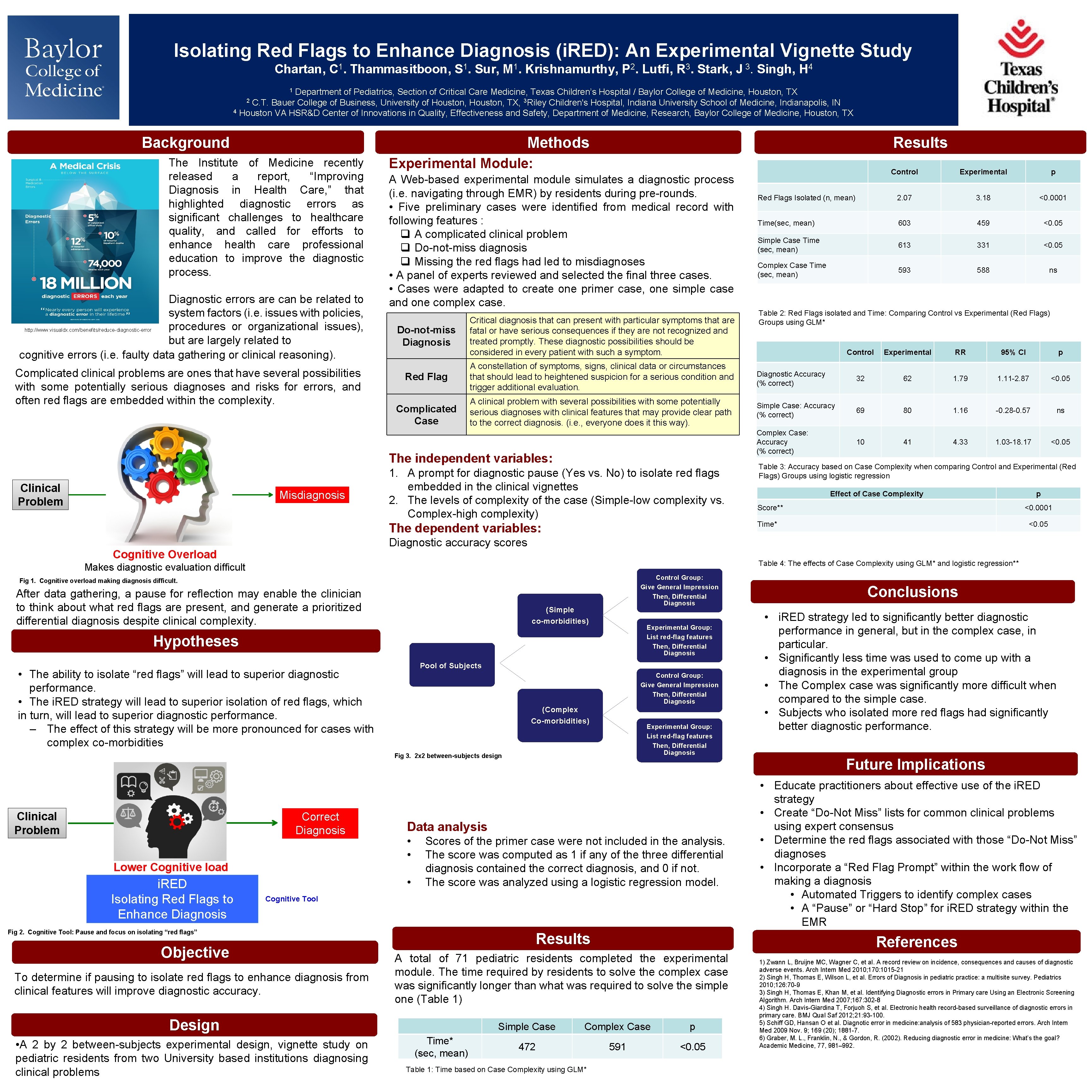
- Slides: 1
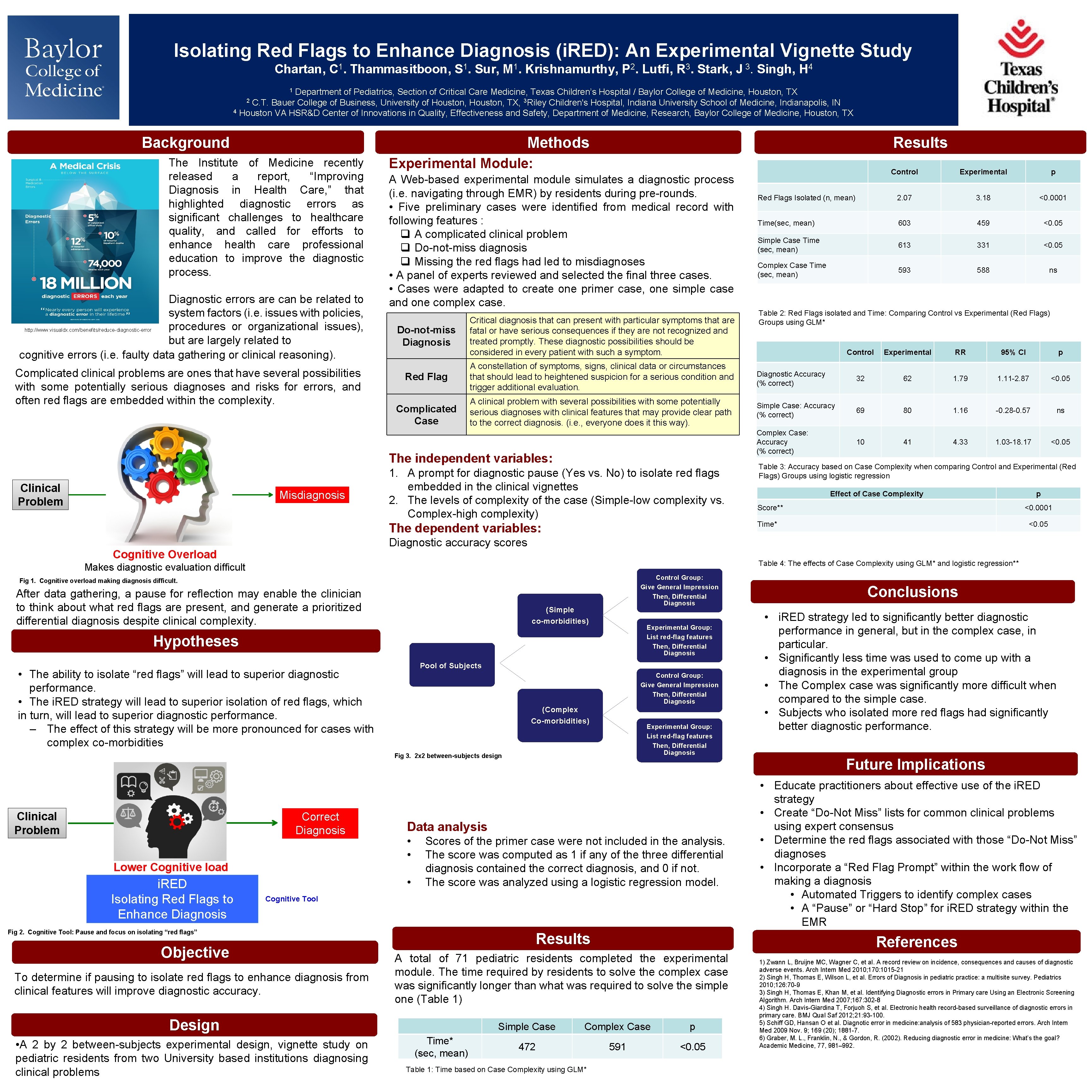
Isolating Red Flags to Enhance Diagnosis (i. RED): An Experimental Vignette Study Poster Title Chartan, C 1. Thammasitboon, S 1. Sur, M 1. Krishnamurthy, P 2. Lutfi, R 3. Stark, J 3. Singh, H 4 1 Department of Pediatrics, Section of Critical Care Medicine, Texas Children’s Hospital / Baylor College of Medicine, Houston, TX Researchers’/Presenters’ Names Houston VA HSR&D Center of Innovations in Quality, Effectiveness and Safety, Department of Medicine, Research, Baylor College of Medicine, Houston, TX 2 C. T. Bauer College of Business, University of Houston, TX, 3 Riley Children's Hospital, Indiana University School of Medicine, Indianapolis, IN 4 Institution/Organization/Company Methods Background Abstract The Institute of Medicine recently (Click on thereleased text to edit)a report, “Improving Diagnosis in Health Care, ” that highlighted diagnostic errors as significant challenges to healthcare quality, and called for efforts to enhance health care professional education to improve the diagnostic process. Diagnostic errors are can be related to system factors (i. e. issues with policies, procedures or organizational issues), http: //www. visualdx. com/benefits/reduce-diagnostic-error but are largely related to cognitive errors (i. e. faulty data gathering or clinical reasoning). Complicated clinical problems are ones that have several possibilities with some potentially serious diagnoses and risks for errors, and often red flags are embedded within the complexity. Results Experimental Module: A Web-based experimental module simulates a diagnostic process (i. e. navigating through EMR) by residents during pre-rounds. • Five preliminary cases were identified from medical record with following features : q A complicated clinical problem q Do-not-miss diagnosis q Missing the red flags had led to misdiagnoses • A panel of experts reviewed and selected the final three cases. • Cases were adapted to create one primer case, one simple case and one complex case. Do-not-miss Diagnosis Critical diagnosis that can present with particular symptoms that are fatal or have serious consequences if they are not recognized and treated promptly. These diagnostic possibilities should be considered in every patient with such a symptom. Misdiagnosis Red Flags Isolated (n, mean) 2. 07 3. 18 <0. 0001 Time(sec, mean) 603 459 <0. 05 Simple Case Time (sec, mean) 613 331 <0. 05 Complex Case Time (sec, mean) 593 588 ns Table 2: Red Flags isolated and Time: Comparing Control vs Experimental (Red Flags) Groups using GLM* Control Experimental RR 95% CI p Diagnostic Accuracy (% correct) 32 62 1. 79 1. 11 -2. 87 <0. 05 Complicated Case A clinical problem with several possibilities with some potentially serious diagnoses with clinical features that may provide clear path to the correct diagnosis. (i. e. , everyone does it this way). Simple Case: Accuracy (% correct) 69 80 1. 16 -0. 28 -0. 57 ns Complex Case: Accuracy (% correct) 10 41 4. 33 1. 03 -18. 17 <0. 05 1. A prompt for diagnostic pause (Yes vs. No) to isolate red flags embedded in the clinical vignettes 2. The levels of complexity of the case (Simple-low complexity vs. Complex-high complexity) Table 3: Accuracy based on Case Complexity when comparing Control and Experimental (Red Flags) Groups using logistic regression Effect of Case Complexity Score** p <0. 0001 Time* <0. 05 Diagnostic accuracy scores Cognitive Overload Table 4: The effects of Case Complexity using GLM* and logistic regression** Makes diagnostic evaluation difficult Background Control Group: Give General Impression Fig 1. Cognitive overload making diagnosis difficult. After data gathering, a pause for reflection may enable the clinician to think about what red flags are present, and generate a prioritized differential diagnosis despite clinical complexity. Conclusions Then, Differential Diagnosis (Simple co-morbidities) • i. RED strategy led to significantly better diagnostic performance in general, but in the complex case, in particular. • Significantly less time was used to come up with a diagnosis in the experimental group • The Complex case was significantly more difficult when compared to the simple case. • Subjects who isolated more red flags had significantly better diagnostic performance. Experimental Group: List red-flag features Hypotheses Then, Differential Diagnosis Pool of Subjects • The ability to isolate “red flags” will lead to superior diagnostic performance. • The i. RED strategy will lead to superior isolation of red flags, which in turn, will lead to superior diagnostic performance. – The effect of this strategy will be more pronounced for cases with complex co-morbidities Control Group: Give General Impression Then, Differential Diagnosis (Complex Co-morbidities) Experimental Group: List red-flag features Then, Differential Diagnosis Fig 3. 2 x 2 between-subjects design Correct Diagnosis Lower Cognitive load i. RED Isolating Red Flags to Enhance Diagnosis p Red Flag The dependent variables: Clinical Problem Experimental A constellation of symptoms, signs, clinical data or circumstances that should lead to heightened suspicion for a serious condition and trigger additional evaluation. The independent variables: Clinical Problem Control Data analysis • • • Scores of the primer case were not included in the analysis. The score was computed as 1 if any of the three differential diagnosis contained the correct diagnosis, and 0 if not. The score was analyzed using a logistic regression model. Cognitive Tool Fig 2. Cognitive Tool: Pause and focus on isolating “red flags” Objective To determine if pausing to isolate red flags to enhance diagnosis from clinical features will improve diagnostic accuracy. • Educate practitioners about effective use of the i. RED strategy • Create “Do-Not Miss” lists for common clinical problems using expert consensus • Determine the red flags associated with those “Do-Not Miss” diagnoses • Incorporate a “Red Flag Prompt” within the work flow of making a diagnosis • Automated Triggers to identify complex cases • A “Pause” or “Hard Stop” for i. RED strategy within the EMR Results References A total of 71 pediatric residents completed the experimental module. The time required by residents to solve the complex case was significantly longer than what was required to solve the simple one (Table 1) Design • A 2 by 2 between-subjects experimental design, vignette study on pediatric residents from two University based institutions diagnosing clinical problems Future Implications Time* (sec, mean) Simple Case Complex Case p 472 591 <0. 05 Table 1: Time based on Case Complexity using GLM* 1) Zwann L, Bruijne MC, Wagner C, et al. A record review on incidence, consequences and causes of diagnostic adverse events. Arch Intern Med 2010; 170: 1015 -21 2) Singh H, Thomas E, Wilson L, et al. Errors of Diagnosis in pediatric practice: a multisite survey. Pediatrics 2010; 126: 70 -9 3) Singh H, Thomas E, Khan M, et al. Identifying Diagnostic errors in Primary care Using an Electronic Screening Algorithm. Arch Intern Med 2007; 167: 302 -8 4) Singh H. Davis-Giardina T, Forjuoh S, et al. Electronic health record-based surveillance of diagnostic errors in primary care. BMJ Qual Saf 2012; 21: 93 -100. 5) Schiff GD, Hansan O et al. Diagnotic error in medicine: analysis of 583 physician-reported errors. Arch Intern Med 2009 Nov. 9; 169 (20); 1881 -7. 6) Graber, M. L. , Franklin, N. , & Gordon, R. (2002). Reducing diagnostic error in medicine: What’s the goal? Academic Medicine, 77, 981– 992.