Does Humanitarian Aid Crowd Out Development Aid A
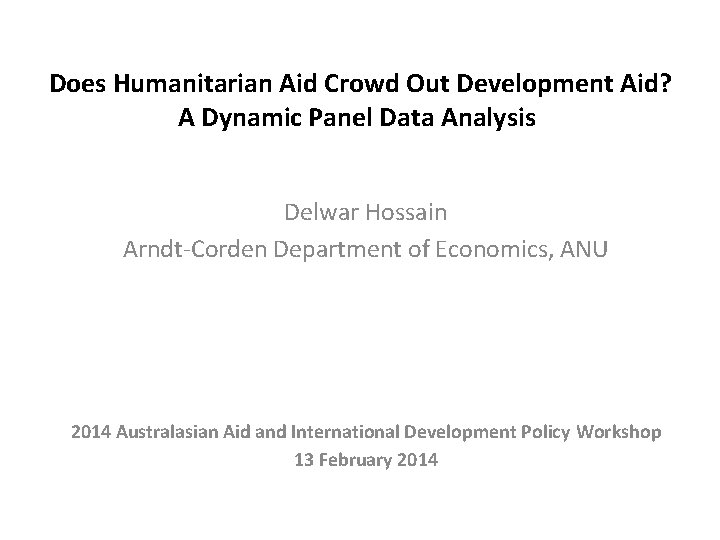
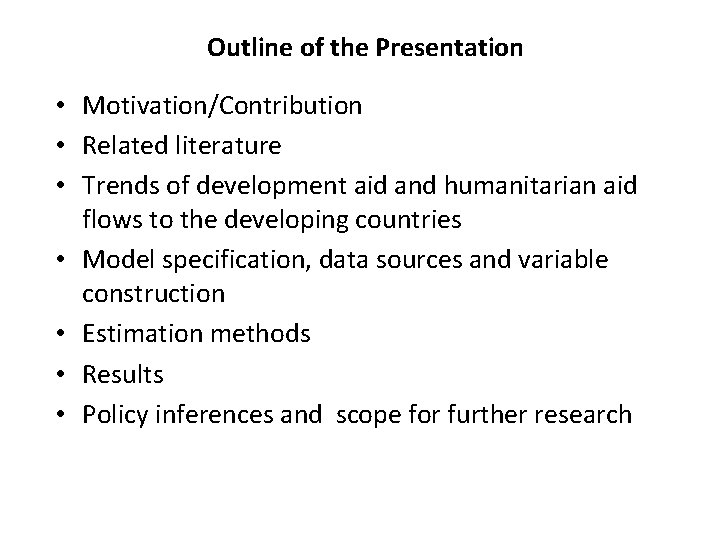
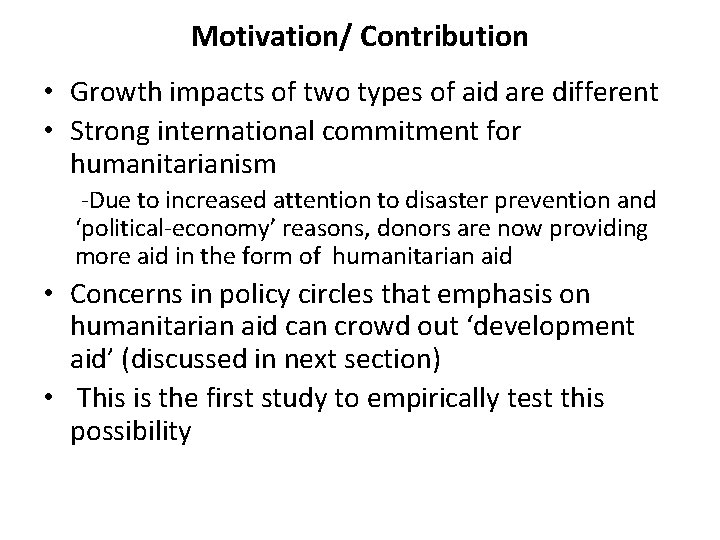
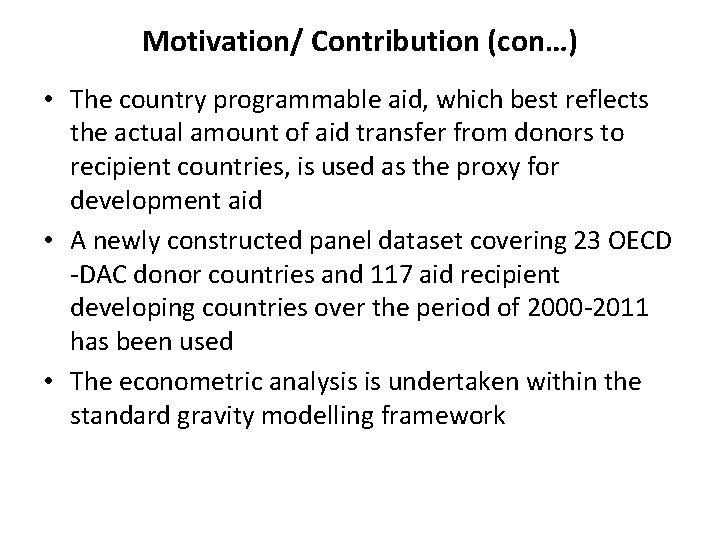
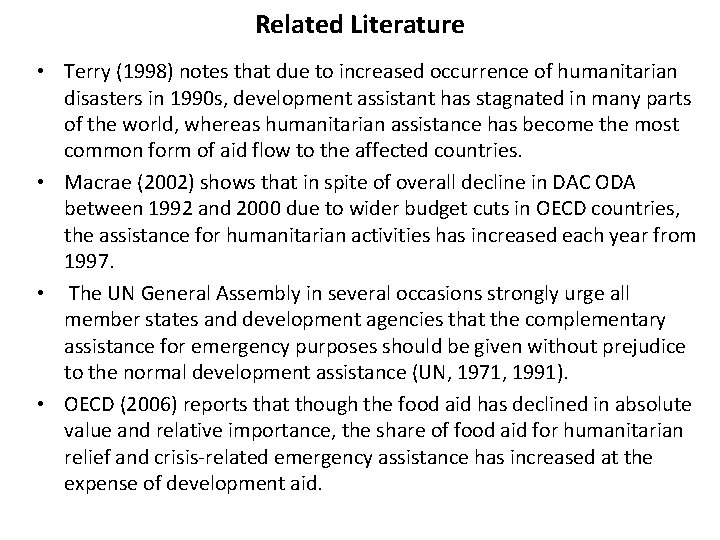
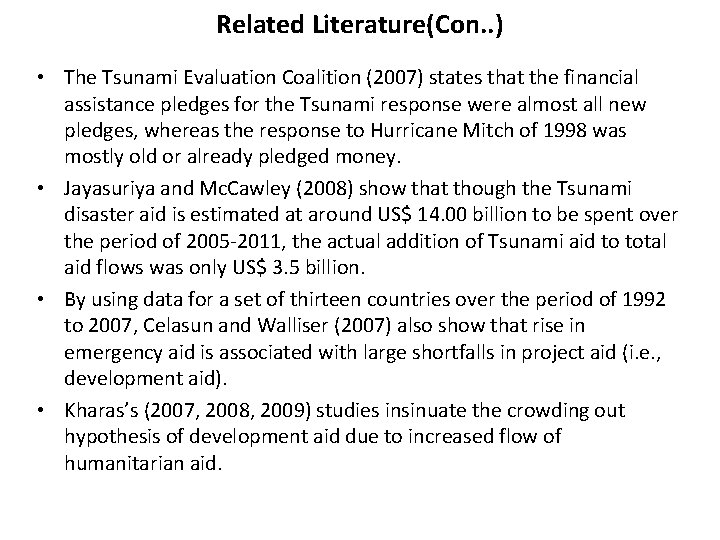
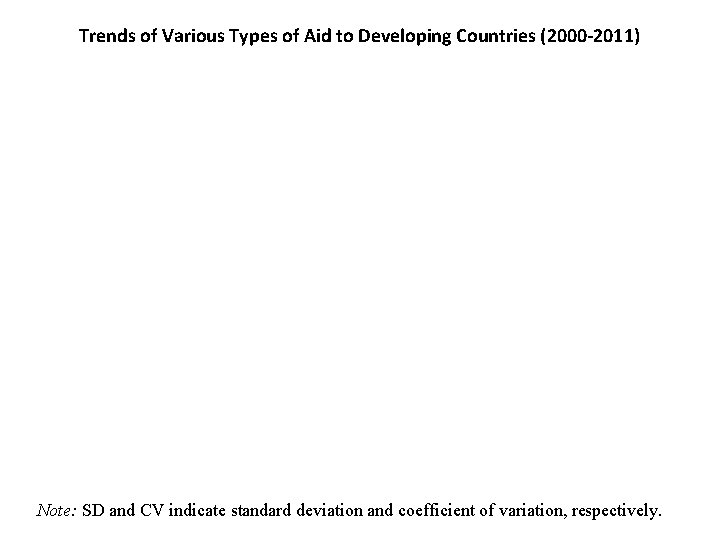
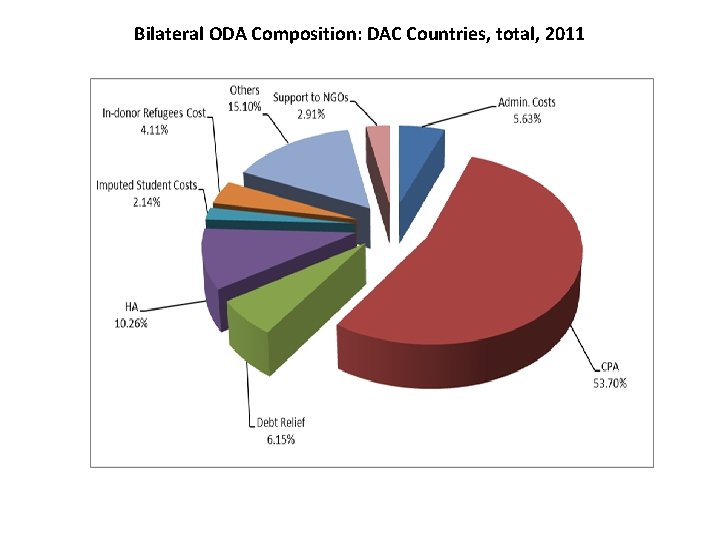
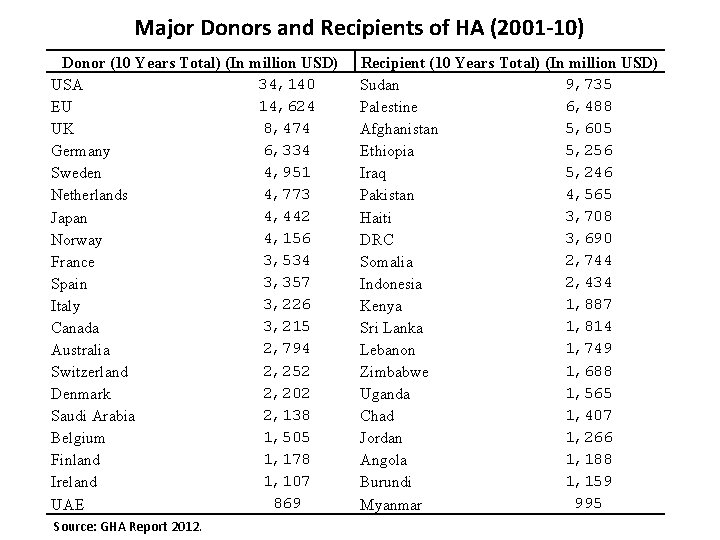
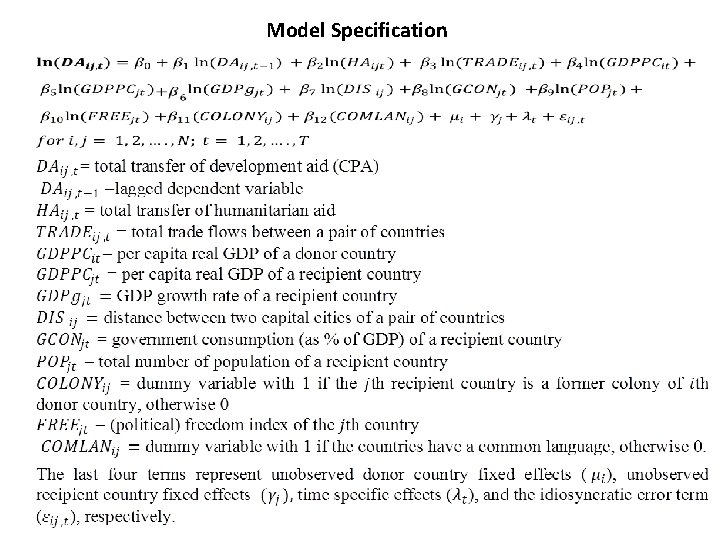
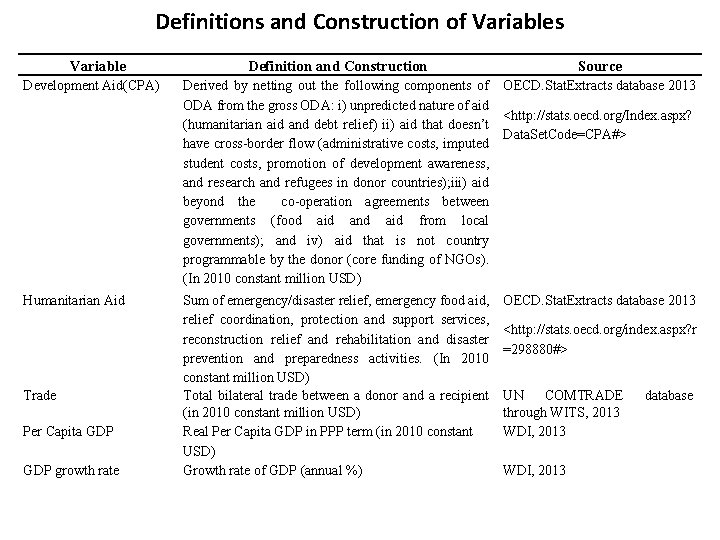
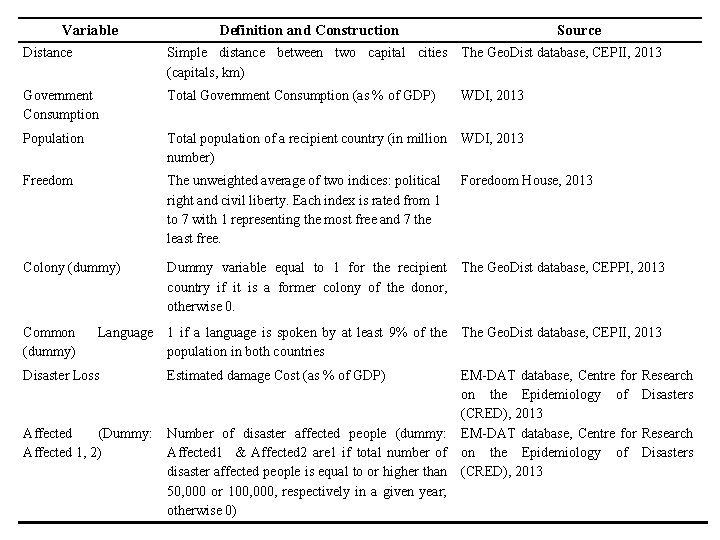
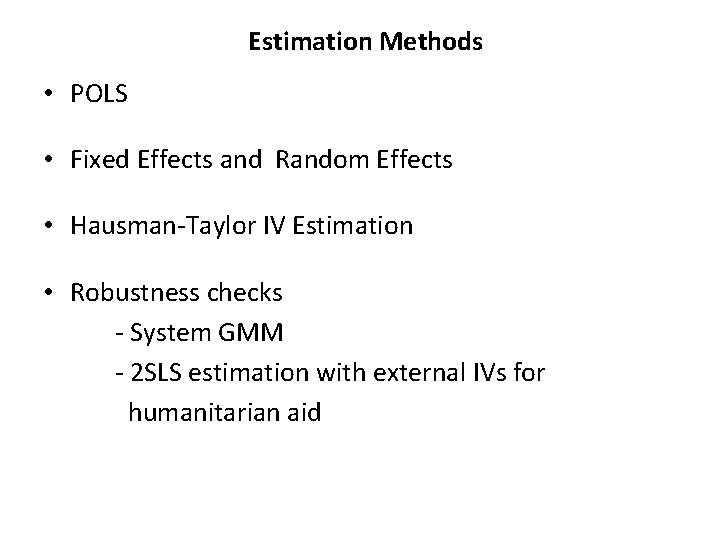
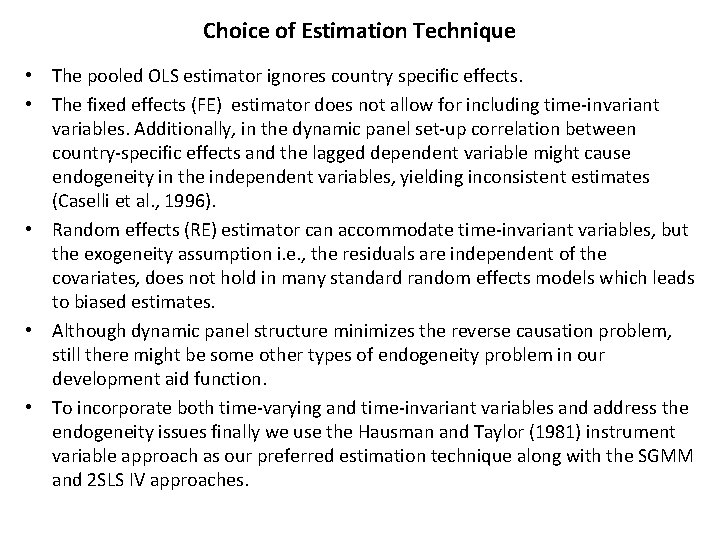
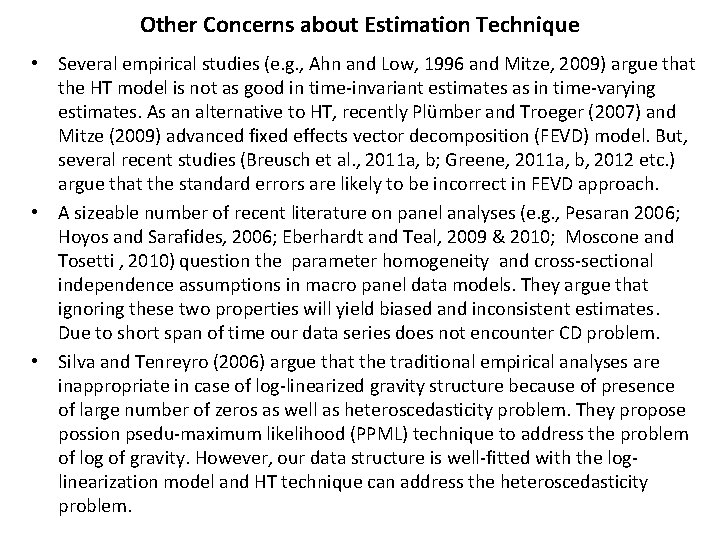
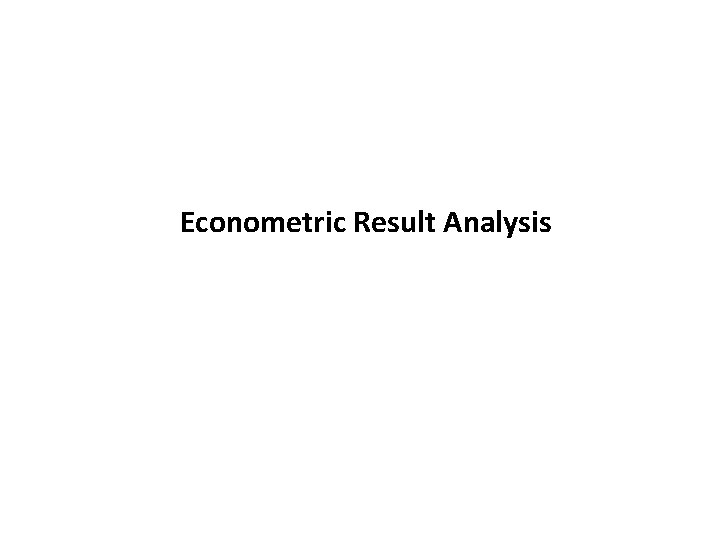
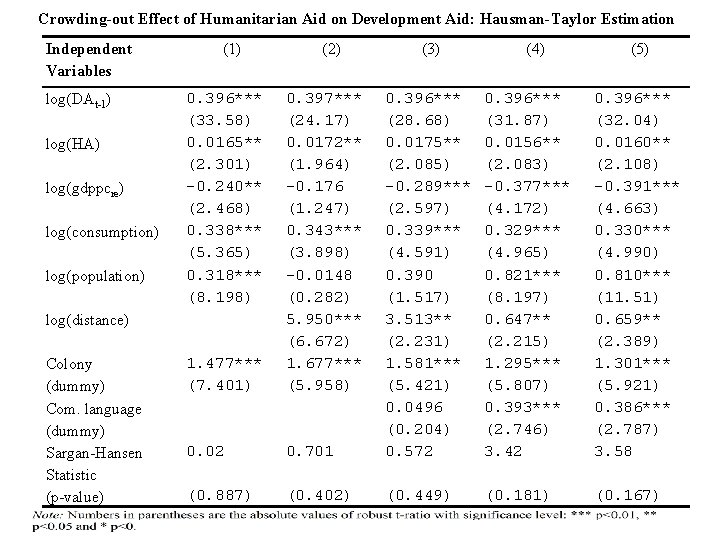
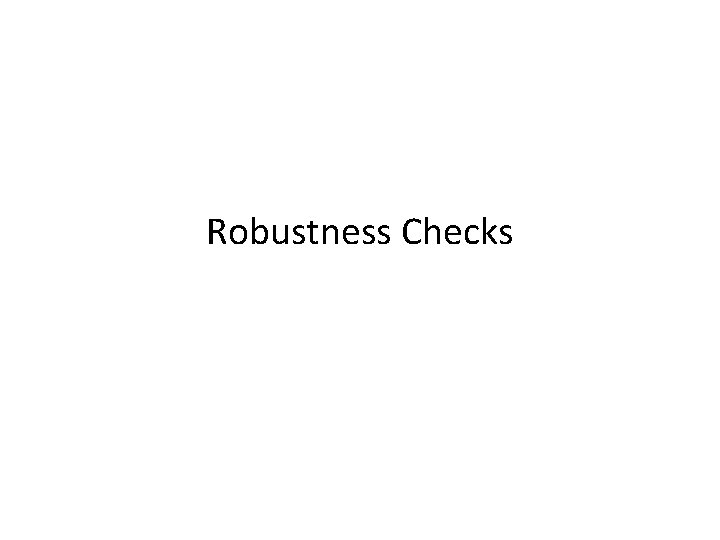
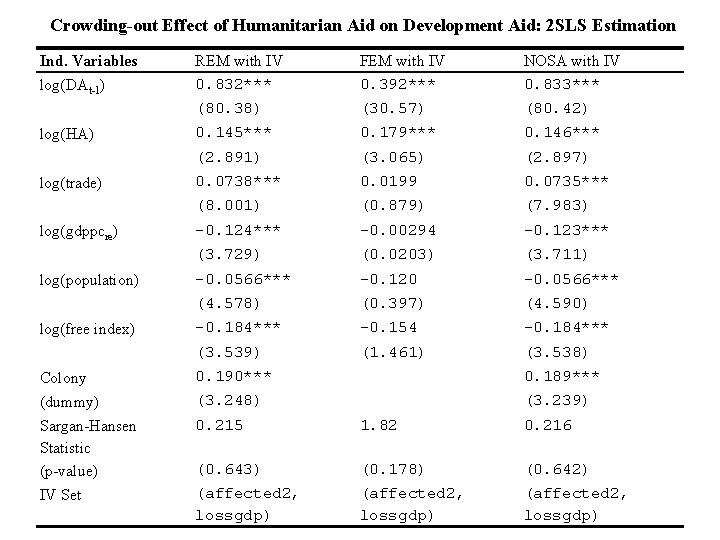
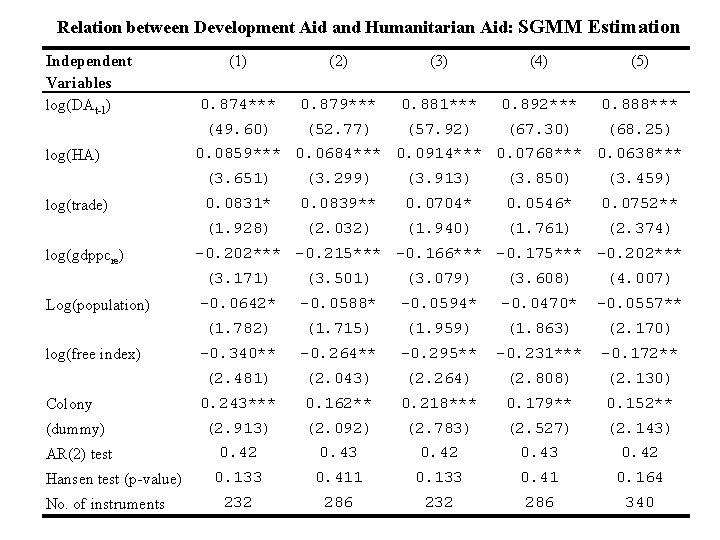
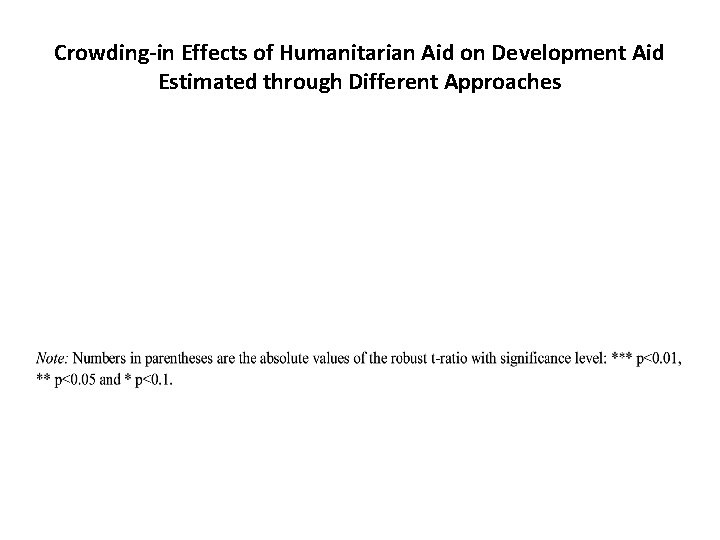
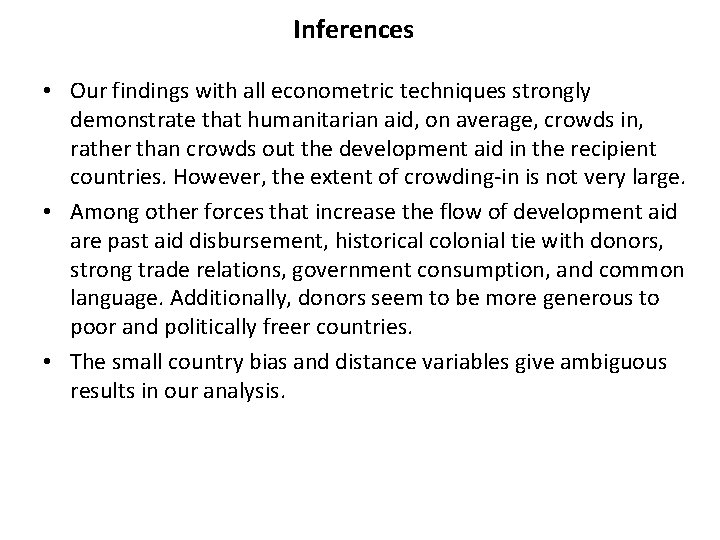
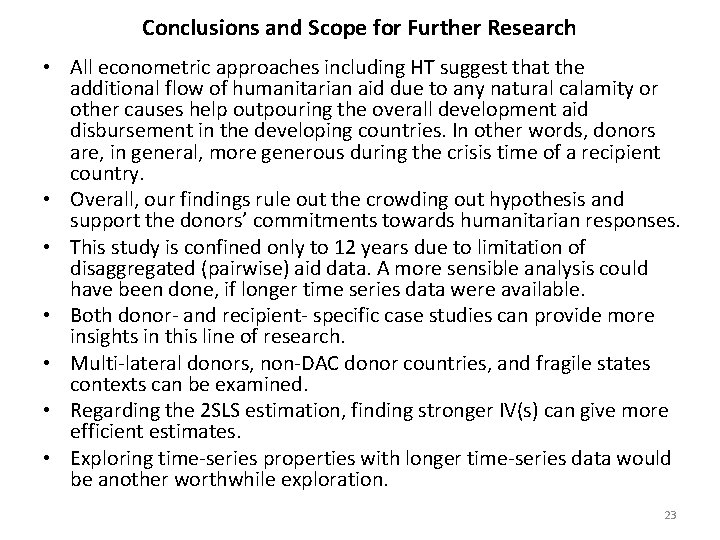
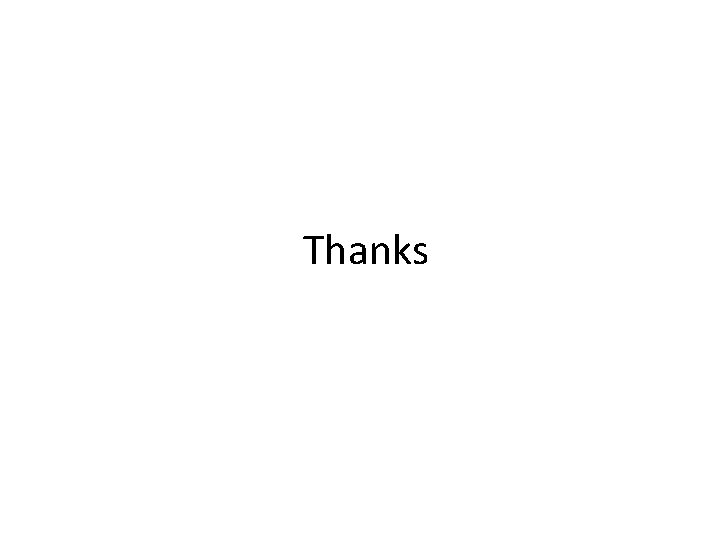
- Slides: 24
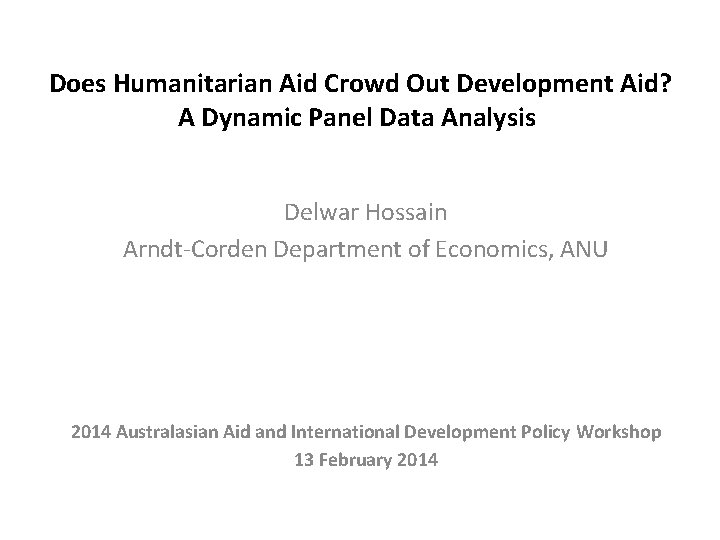
Does Humanitarian Aid Crowd Out Development Aid? A Dynamic Panel Data Analysis Delwar Hossain Arndt-Corden Department of Economics, ANU 2014 Australasian Aid and International Development Policy Workshop 13 February 2014
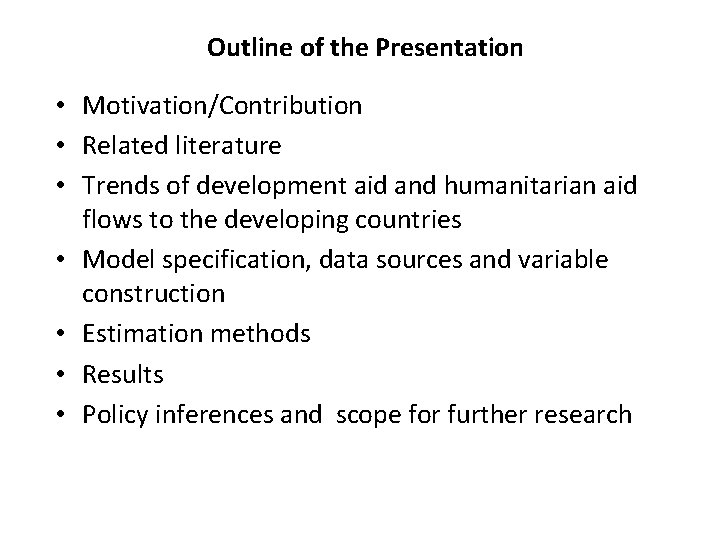
Outline of the Presentation • Motivation/Contribution • Related literature • Trends of development aid and humanitarian aid flows to the developing countries • Model specification, data sources and variable construction • Estimation methods • Results • Policy inferences and scope for further research
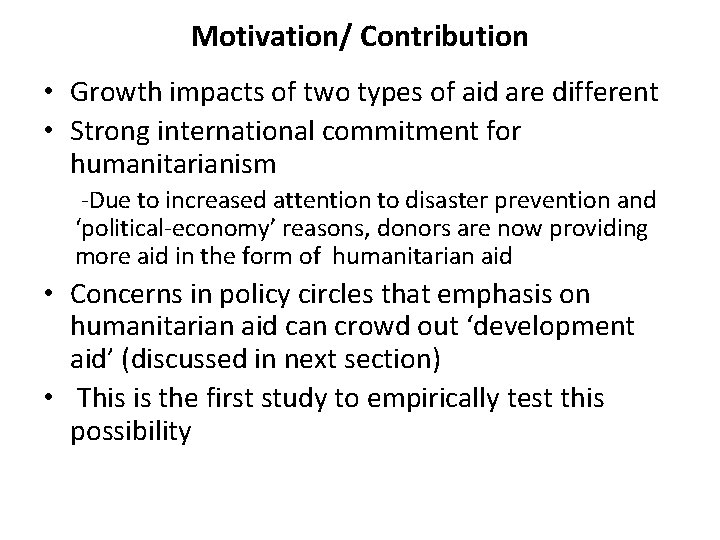
Motivation/ Contribution • Growth impacts of two types of aid are different • Strong international commitment for humanitarianism -Due to increased attention to disaster prevention and ‘political-economy’ reasons, donors are now providing more aid in the form of humanitarian aid • Concerns in policy circles that emphasis on humanitarian aid can crowd out ‘development aid’ (discussed in next section) • This is the first study to empirically test this possibility
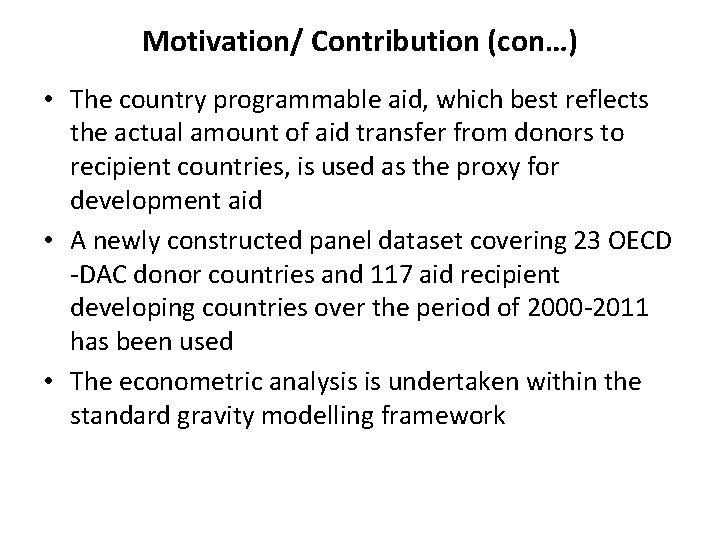
Motivation/ Contribution (con…) • The country programmable aid, which best reflects the actual amount of aid transfer from donors to recipient countries, is used as the proxy for development aid • A newly constructed panel dataset covering 23 OECD -DAC donor countries and 117 aid recipient developing countries over the period of 2000 -2011 has been used • The econometric analysis is undertaken within the standard gravity modelling framework
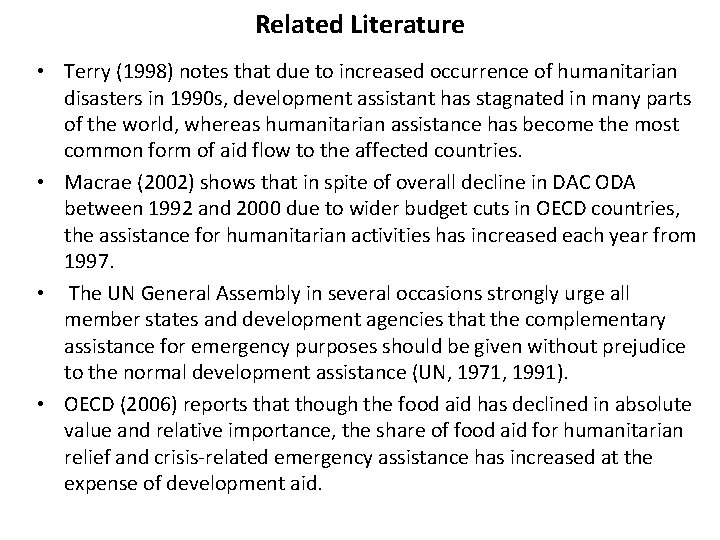
Related Literature • Terry (1998) notes that due to increased occurrence of humanitarian disasters in 1990 s, development assistant has stagnated in many parts of the world, whereas humanitarian assistance has become the most common form of aid flow to the affected countries. • Macrae (2002) shows that in spite of overall decline in DAC ODA between 1992 and 2000 due to wider budget cuts in OECD countries, the assistance for humanitarian activities has increased each year from 1997. • The UN General Assembly in several occasions strongly urge all member states and development agencies that the complementary assistance for emergency purposes should be given without prejudice to the normal development assistance (UN, 1971, 1991). • OECD (2006) reports that though the food aid has declined in absolute value and relative importance, the share of food aid for humanitarian relief and crisis-related emergency assistance has increased at the expense of development aid.
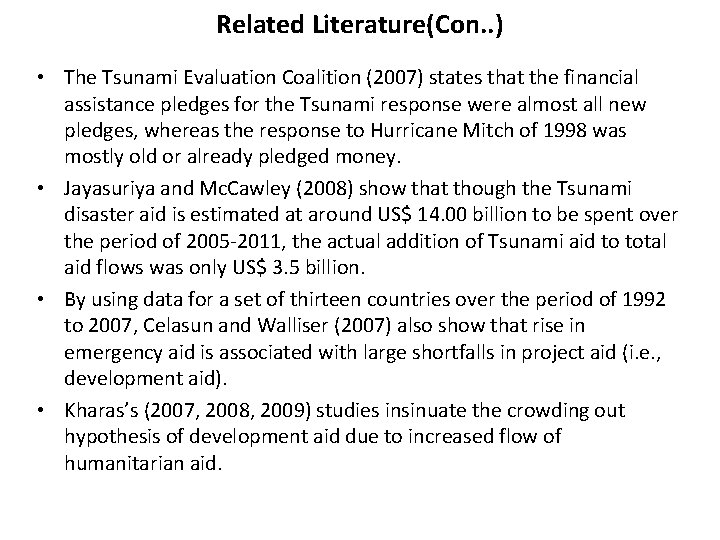
Related Literature(Con. . ) • The Tsunami Evaluation Coalition (2007) states that the financial assistance pledges for the Tsunami response were almost all new pledges, whereas the response to Hurricane Mitch of 1998 was mostly old or already pledged money. • Jayasuriya and Mc. Cawley (2008) show that though the Tsunami disaster aid is estimated at around US$ 14. 00 billion to be spent over the period of 2005 -2011, the actual addition of Tsunami aid to total aid flows was only US$ 3. 5 billion. • By using data for a set of thirteen countries over the period of 1992 to 2007, Celasun and Walliser (2007) also show that rise in emergency aid is associated with large shortfalls in project aid (i. e. , development aid). • Kharas’s (2007, 2008, 2009) studies insinuate the crowding out hypothesis of development aid due to increased flow of humanitarian aid.
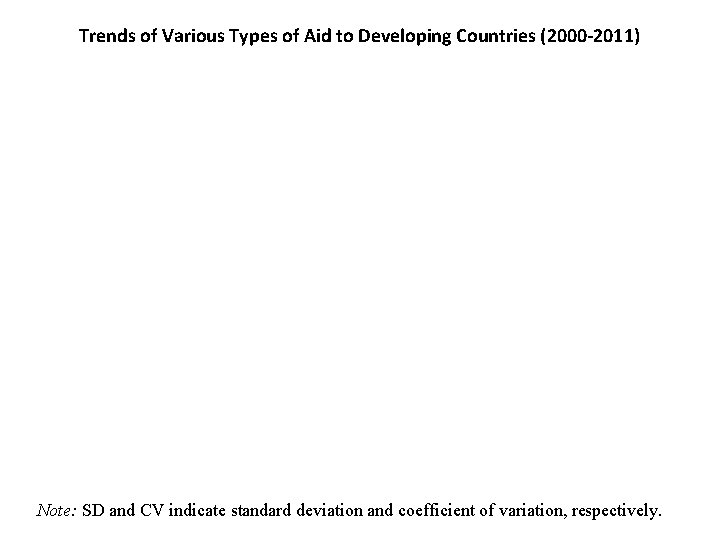
Trends of Various Types of Aid to Developing Countries (2000 -2011) Note: SD and CV indicate standard deviation and coefficient of variation, respectively.
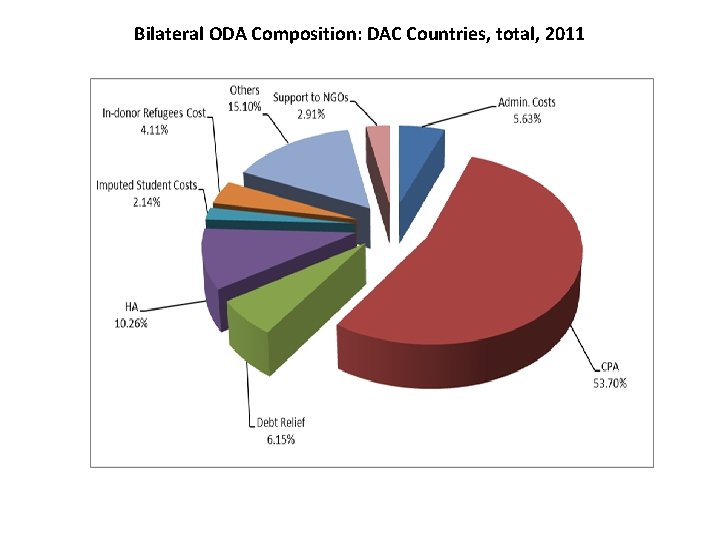
Bilateral ODA Composition: DAC Countries, total, 2011
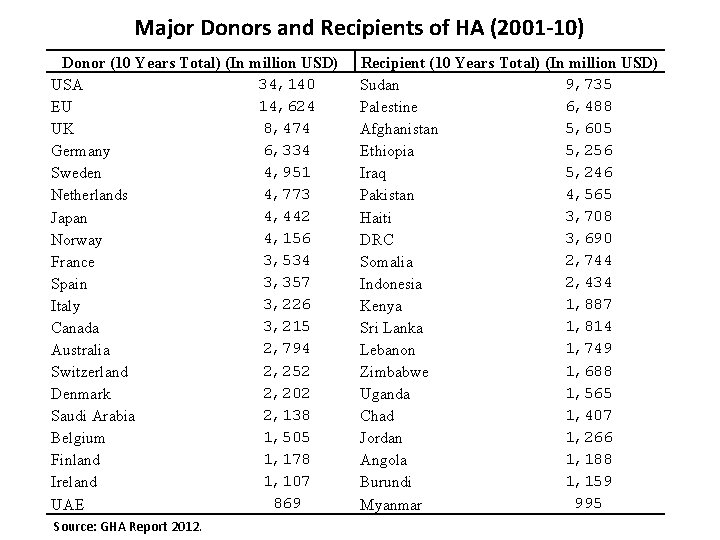
Major Donors and Recipients of HA (2001 -10) Donor (10 Years Total) (In million USD) 34, 140 USA 14, 624 EU 8, 474 UK 6, 334 Germany 4, 951 Sweden 4, 773 Netherlands 4, 442 Japan 4, 156 Norway 3, 534 France 3, 357 Spain 3, 226 Italy 3, 215 Canada 2, 794 Australia 2, 252 Switzerland 2, 202 Denmark 2, 138 Saudi Arabia 1, 505 Belgium 1, 178 Finland 1, 107 Ireland 869 UAE Source: GHA Report 2012. Recipient (10 Years Total) (In million USD) 9, 735 Sudan 6, 488 Palestine 5, 605 Afghanistan 5, 256 Ethiopia 5, 246 Iraq 4, 565 Pakistan 3, 708 Haiti 3, 690 DRC 2, 744 Somalia 2, 434 Indonesia 1, 887 Kenya 1, 814 Sri Lanka 1, 749 Lebanon 1, 688 Zimbabwe 1, 565 Uganda 1, 407 Chad 1, 266 Jordan 1, 188 Angola 1, 159 Burundi 995 Myanmar
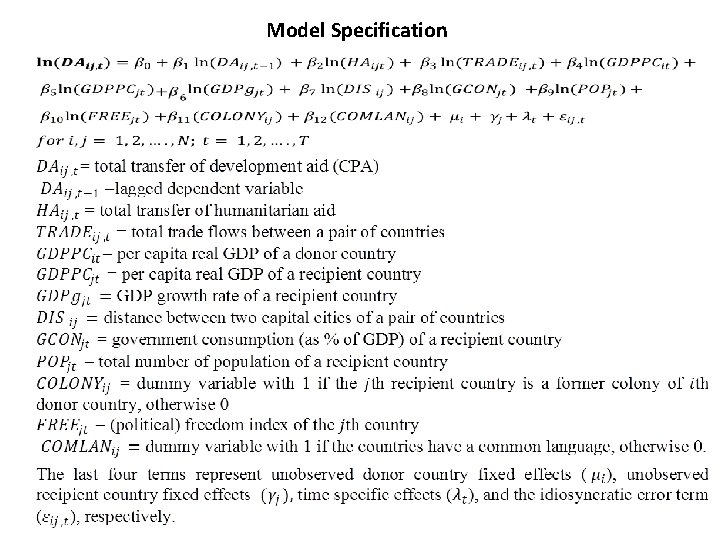
Model Specification
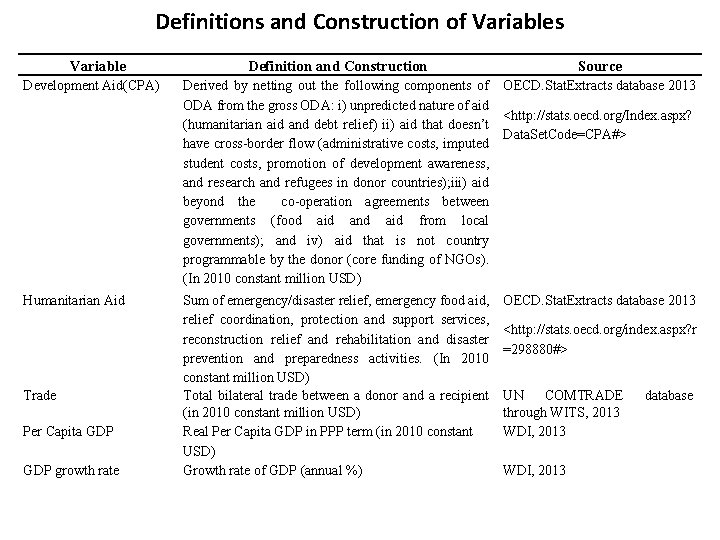
Definitions and Construction of Variables Variable Definition and Construction Source Development Aid(CPA) Derived by netting out the following components of OECD. Stat. Extracts database 2013 ODA from the gross ODA: i) unpredicted nature of aid <http: //stats. oecd. org/Index. aspx? (humanitarian aid and debt relief) ii) aid that doesn’t Data. Set. Code=CPA#> have cross-border flow (administrative costs, imputed student costs, promotion of development awareness, and research and refugees in donor countries); iii) aid beyond the co-operation agreements between governments (food aid and aid from local governments); and iv) aid that is not country programmable by the donor (core funding of NGOs). (In 2010 constant million USD) Humanitarian Aid Sum of emergency/disaster relief, emergency food aid, relief coordination, protection and support services, reconstruction relief and rehabilitation and disaster prevention and preparedness activities. (In 2010 constant million USD) Total bilateral trade between a donor and a recipient (in 2010 constant million USD) Real Per Capita GDP in PPP term (in 2010 constant USD) Growth rate of GDP (annual %) Trade Per Capita GDP growth rate OECD. Stat. Extracts database 2013 <http: //stats. oecd. org/index. aspx? r =298880#> UN COMTRADE through WITS, 2013 WDI, 2013 database
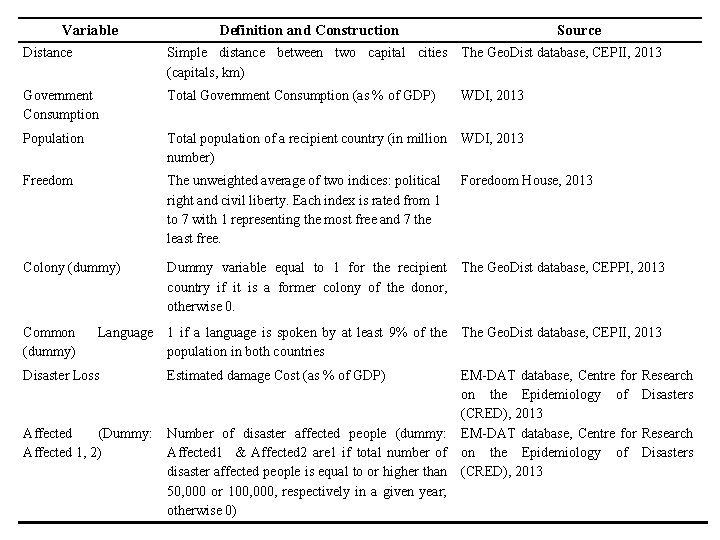
Variable Definition and Construction Source Distance Simple distance between two capital cities The Geo. Dist database, CEPII, 2013 (capitals, km) Government Consumption Total Government Consumption (as % of GDP) Population Total population of a recipient country (in million WDI, 2013 number) Freedom The unweighted average of two indices: political right and civil liberty. Each index is rated from 1 to 7 with 1 representing the most free and 7 the least free. Colony (dummy) Dummy variable equal to 1 for the recipient The Geo. Dist database, CEPPI, 2013 country if it is a former colony of the donor, otherwise 0. Common (dummy) WDI, 2013 Foredoom House, 2013 Language 1 if a language is spoken by at least 9% of the The Geo. Dist database, CEPII, 2013 population in both countries Disaster Loss Estimated damage Cost (as % of GDP) EM-DAT database, Centre for Research on the Epidemiology of Disasters (CRED), 2013 Affected (Dummy: Number of disaster affected people (dummy: EM-DAT database, Centre for Research Affected 1, 2) Affected 1 & Affected 2 are 1 if total number of on the Epidemiology of Disasters disaster affected people is equal to or higher than (CRED), 2013 50, 000 or 100, 000, respectively in a given year; otherwise 0)
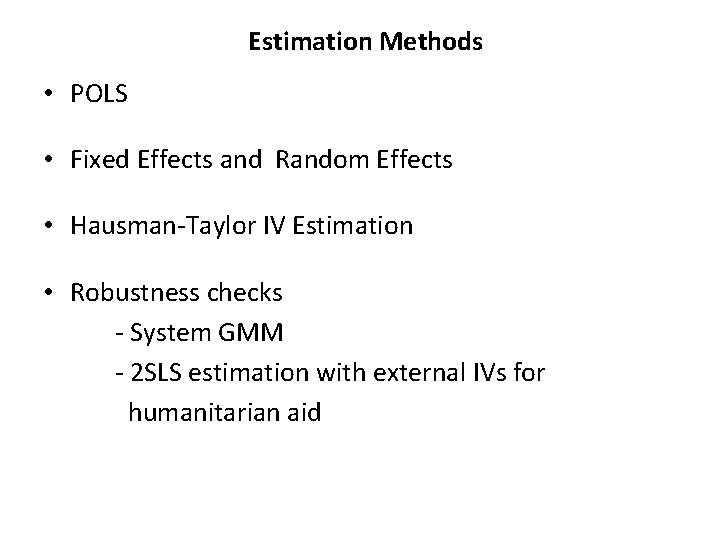
Estimation Methods • POLS • Fixed Effects and Random Effects • Hausman-Taylor IV Estimation • Robustness checks - System GMM - 2 SLS estimation with external IVs for humanitarian aid
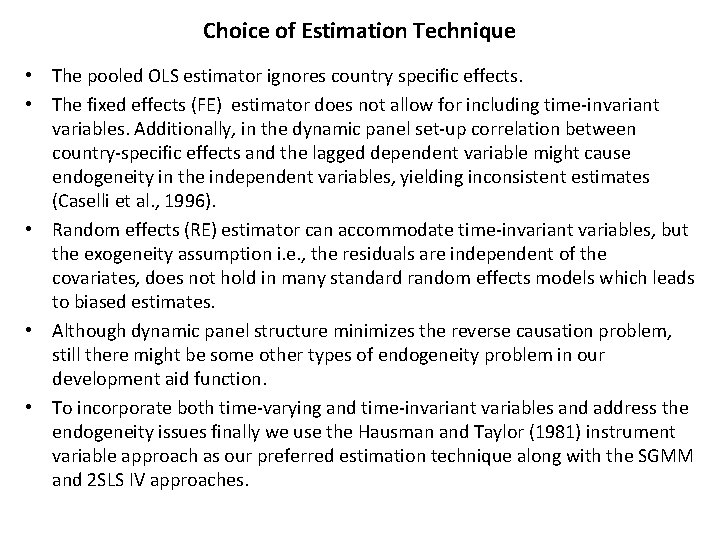
Choice of Estimation Technique • The pooled OLS estimator ignores country specific effects. • The fixed effects (FE) estimator does not allow for including time-invariant variables. Additionally, in the dynamic panel set-up correlation between country-specific effects and the lagged dependent variable might cause endogeneity in the independent variables, yielding inconsistent estimates (Caselli et al. , 1996). • Random effects (RE) estimator can accommodate time-invariant variables, but the exogeneity assumption i. e. , the residuals are independent of the covariates, does not hold in many standard random effects models which leads to biased estimates. • Although dynamic panel structure minimizes the reverse causation problem, still there might be some other types of endogeneity problem in our development aid function. • To incorporate both time-varying and time-invariant variables and address the endogeneity issues finally we use the Hausman and Taylor (1981) instrument variable approach as our preferred estimation technique along with the SGMM and 2 SLS IV approaches.
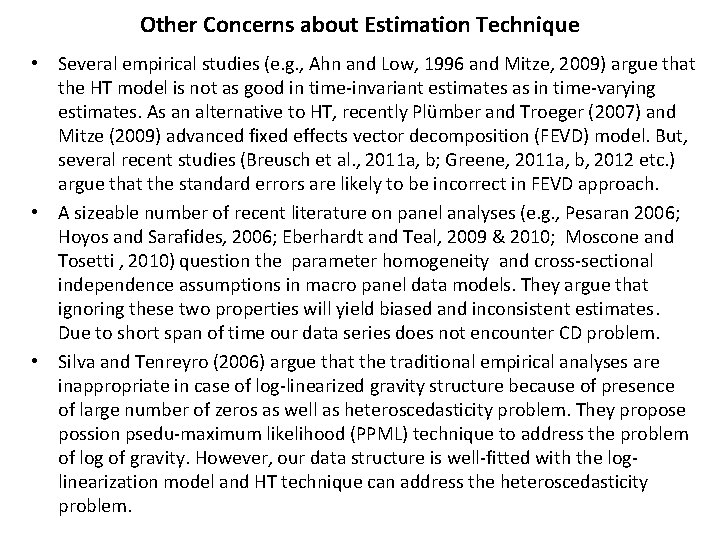
Other Concerns about Estimation Technique • Several empirical studies (e. g. , Ahn and Low, 1996 and Mitze, 2009) argue that the HT model is not as good in time-invariant estimates as in time-varying estimates. As an alternative to HT, recently Plümber and Troeger (2007) and Mitze (2009) advanced fixed effects vector decomposition (FEVD) model. But, several recent studies (Breusch et al. , 2011 a, b; Greene, 2011 a, b, 2012 etc. ) argue that the standard errors are likely to be incorrect in FEVD approach. • A sizeable number of recent literature on panel analyses (e. g. , Pesaran 2006; Hoyos and Sarafides, 2006; Eberhardt and Teal, 2009 & 2010; Moscone and Tosetti , 2010) question the parameter homogeneity and cross-sectional independence assumptions in macro panel data models. They argue that ignoring these two properties will yield biased and inconsistent estimates. Due to short span of time our data series does not encounter CD problem. • Silva and Tenreyro (2006) argue that the traditional empirical analyses are inappropriate in case of log-linearized gravity structure because of presence of large number of zeros as well as heteroscedasticity problem. They propose possion psedu-maximum likelihood (PPML) technique to address the problem of log of gravity. However, our data structure is well-fitted with the loglinearization model and HT technique can address the heteroscedasticity problem.
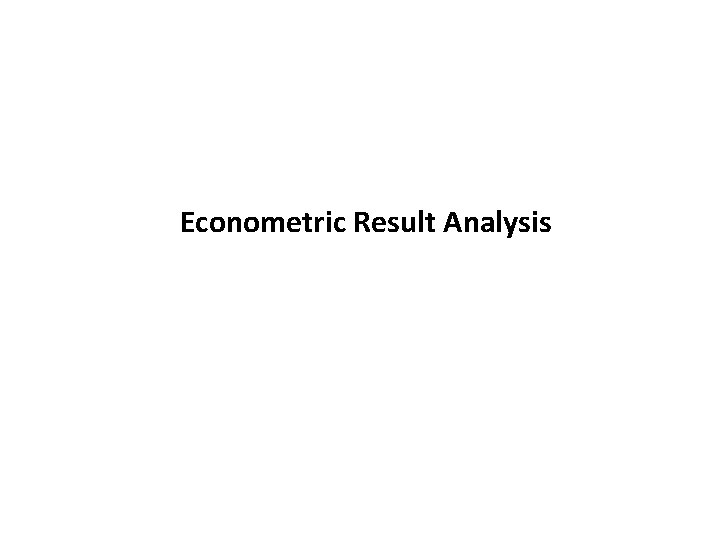
Econometric Result Analysis
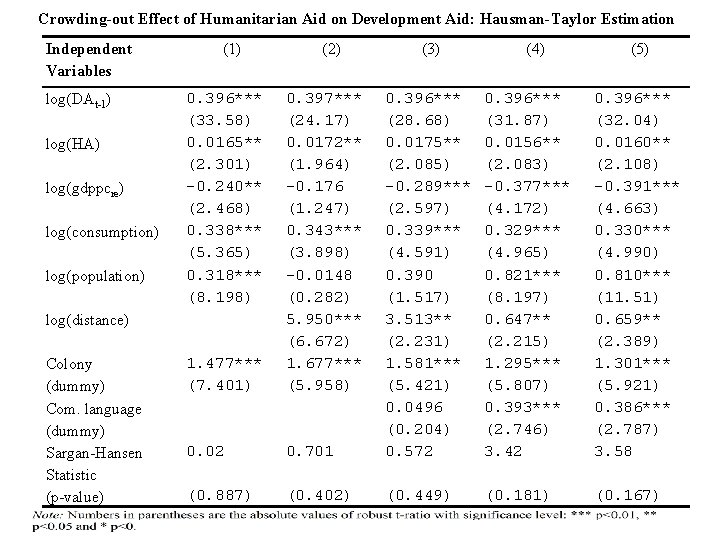
Crowding-out Effect of Humanitarian Aid on Development Aid: Hausman-Taylor Estimation Independent Variables log(DAt-1) log(HA) log(gdppcre) log(consumption) log(population) log(distance) Colony (dummy) Com. language (dummy) Sargan-Hansen Statistic (p-value) (1) (2) (3) (4) (5) 0. 396*** (33. 58) 0. 0165** (2. 301) -0. 240** (2. 468) 0. 338*** (5. 365) 0. 318*** (8. 198) 1. 477*** (7. 401) 0. 02 0. 397*** (24. 17) 0. 0172** (1. 964) -0. 176 (1. 247) 0. 343*** (3. 898) -0. 0148 (0. 282) 5. 950*** (6. 672) 1. 677*** (5. 958) 0. 701 0. 396*** (28. 68) 0. 0175** (2. 085) -0. 289*** (2. 597) 0. 339*** (4. 591) 0. 390 (1. 517) 3. 513** (2. 231) 1. 581*** (5. 421) 0. 0496 (0. 204) 0. 572 0. 396*** (31. 87) 0. 0156** (2. 083) -0. 377*** (4. 172) 0. 329*** (4. 965) 0. 821*** (8. 197) 0. 647** (2. 215) 1. 295*** (5. 807) 0. 393*** (2. 746) 3. 42 0. 396*** (32. 04) 0. 0160** (2. 108) -0. 391*** (4. 663) 0. 330*** (4. 990) 0. 810*** (11. 51) 0. 659** (2. 389) 1. 301*** (5. 921) 0. 386*** (2. 787) 3. 58 (0. 887) (0. 402) (0. 449) (0. 181) (0. 167)
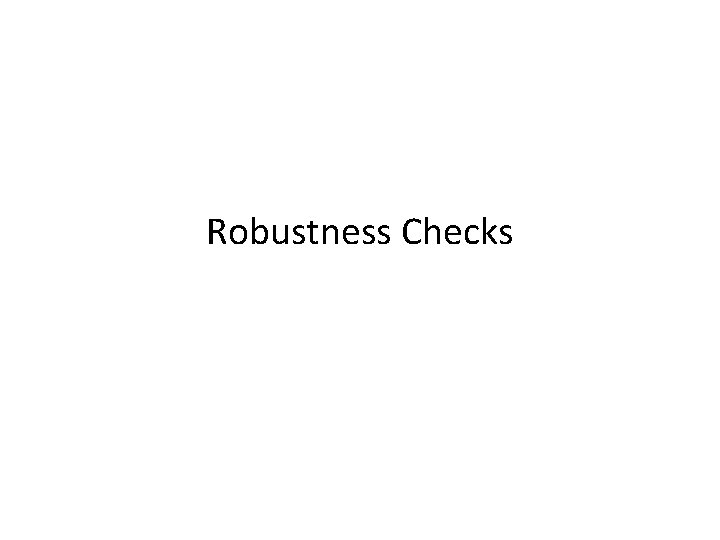
Robustness Checks
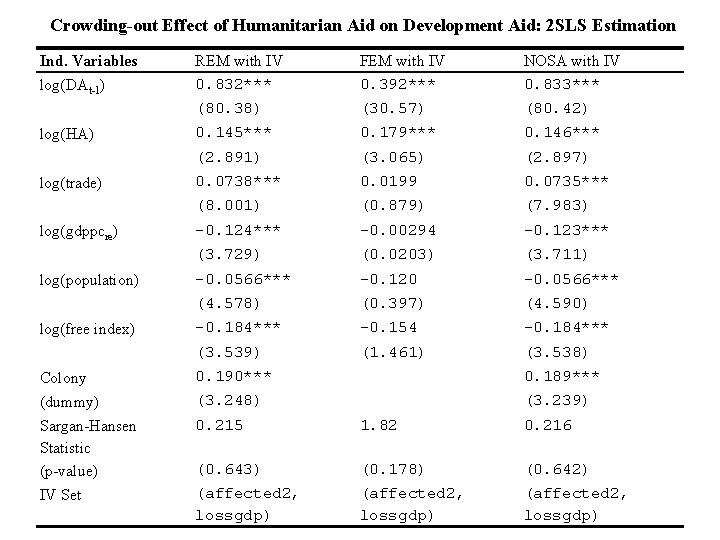
Crowding-out Effect of Humanitarian Aid on Development Aid: 2 SLS Estimation Ind. Variables log(DAt-1) REM with IV 0. 832*** FEM with IV 0. 392*** NOSA with IV 0. 833*** (80. 38) (30. 57) (80. 42) log(HA) 0. 145*** 0. 179*** 0. 146*** (2. 891) (3. 065) (2. 897) log(trade) 0. 0738*** 0. 0199 0. 0735*** (8. 001) (0. 879) (7. 983) log(gdppcre) -0. 124*** -0. 00294 -0. 123*** (3. 729) (0. 0203) (3. 711) log(population) -0. 0566*** -0. 120 -0. 0566*** (4. 578) (0. 397) (4. 590) log(free index) -0. 184*** -0. 154 -0. 184*** (3. 539) (1. 461) (3. 538) Colony 0. 190*** 0. 189*** (dummy) (3. 248) (3. 239) Sargan-Hansen Statistic (p-value) 0. 215 1. 82 0. 216 (0. 643) (0. 178) (0. 642) IV Set (affected 2, lossgdp)
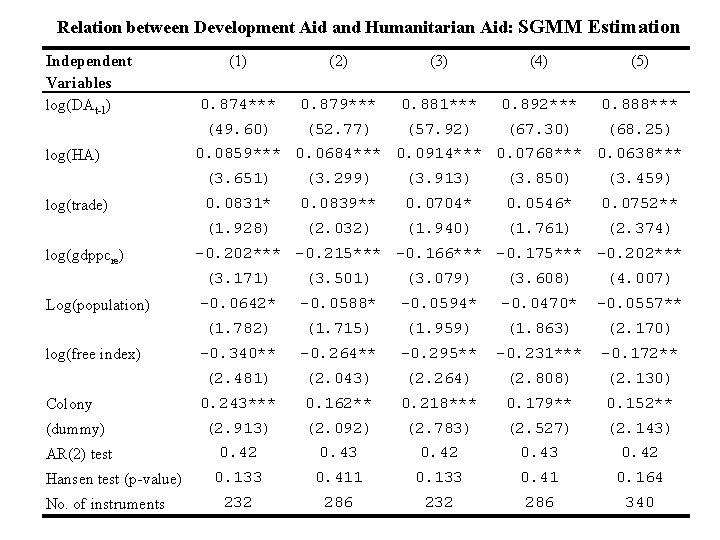
Relation between Development Aid and Humanitarian Aid: SGMM Estimation Independent Variables log(DAt-1) log(HA) (1) (2) (3) (4) (5) 0. 874*** 0. 879*** 0. 881*** 0. 892*** 0. 888*** (49. 60) (52. 77) (57. 92) (67. 30) (68. 25) 0. 0859*** 0. 0684*** 0. 0914*** 0. 0768*** 0. 0638*** (3. 651) (3. 299) (3. 913) (3. 850) (3. 459) log(trade) 0. 0831* 0. 0839** 0. 0704* 0. 0546* 0. 0752** (1. 928) (2. 032) (1. 940) (1. 761) (2. 374) log(gdppcre) -0. 202*** -0. 215*** -0. 166*** -0. 175*** -0. 202*** (3. 171) (3. 501) (3. 079) (3. 608) (4. 007) -0. 0642* -0. 0588* -0. 0594* -0. 0470* -0. 0557** (1. 782) (1. 715) (1. 959) (1. 863) (2. 170) -0. 340** -0. 264** -0. 295** -0. 231*** -0. 172** (2. 481) (2. 043) (2. 264) (2. 808) (2. 130) 0. 243*** 0. 162** 0. 218*** 0. 179** 0. 152** (dummy) (2. 913) (2. 092) (2. 783) (2. 527) (2. 143) AR(2) test 0. 42 0. 43 0. 42 0. 133 0. 411 0. 133 0. 41 0. 164 232 286 340 Log(population) log(free index) Colony Hansen test (p-value) No. of instruments
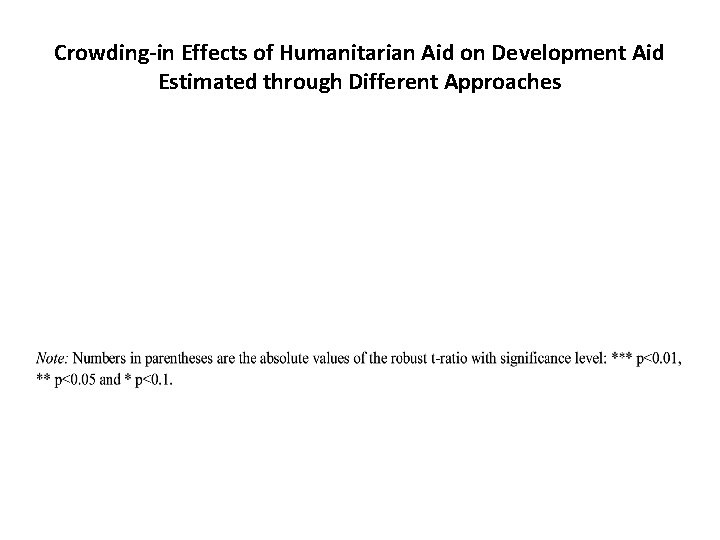
Crowding-in Effects of Humanitarian Aid on Development Aid Estimated through Different Approaches
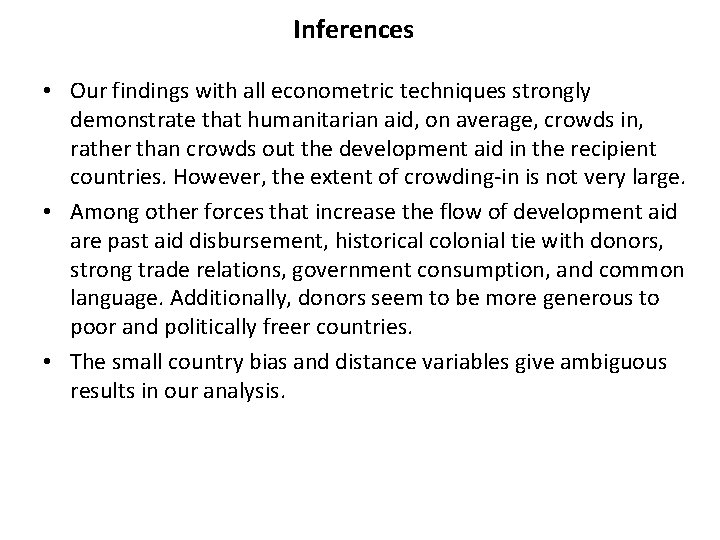
Inferences • Our findings with all econometric techniques strongly demonstrate that humanitarian aid, on average, crowds in, rather than crowds out the development aid in the recipient countries. However, the extent of crowding-in is not very large. • Among other forces that increase the flow of development aid are past aid disbursement, historical colonial tie with donors, strong trade relations, government consumption, and common language. Additionally, donors seem to be more generous to poor and politically freer countries. • The small country bias and distance variables give ambiguous results in our analysis.
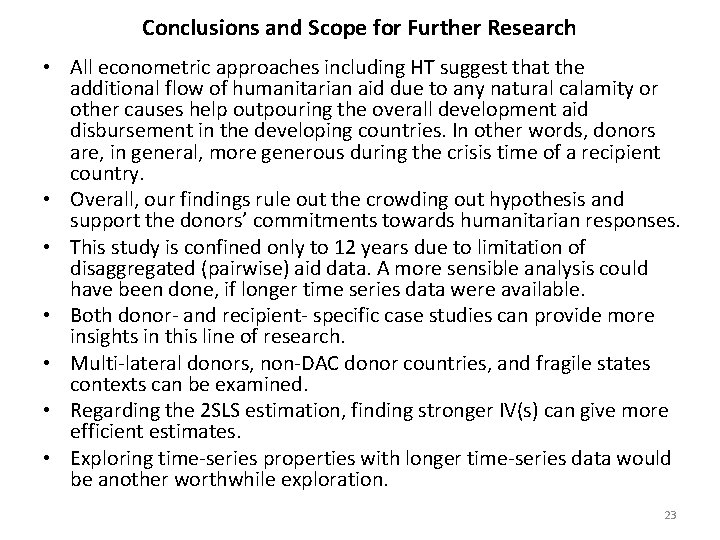
Conclusions and Scope for Further Research • All econometric approaches including HT suggest that the additional flow of humanitarian aid due to any natural calamity or other causes help outpouring the overall development aid disbursement in the developing countries. In other words, donors are, in general, more generous during the crisis time of a recipient country. • Overall, our findings rule out the crowding out hypothesis and support the donors’ commitments towards humanitarian responses. • This study is confined only to 12 years due to limitation of disaggregated (pairwise) aid data. A more sensible analysis could have been done, if longer time series data were available. • Both donor- and recipient- specific case studies can provide more insights in this line of research. • Multi-lateral donors, non-DAC donor countries, and fragile states contexts can be examined. • Regarding the 2 SLS estimation, finding stronger IV(s) can give more efficient estimates. • Exploring time-series properties with longer time-series data would be another worthwhile exploration. 23
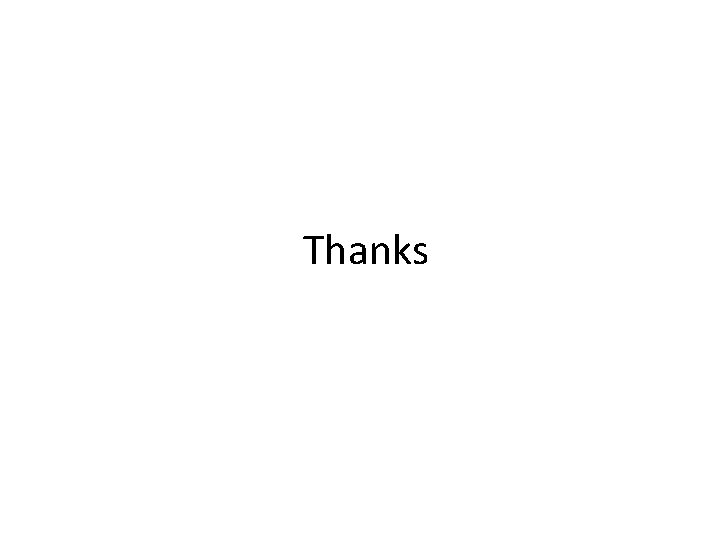
Thanks
One thing one direction
Ee.humanitarian response.info/x/vpdlixjf
Humanitarian work psychology
Humanitarian projects in prague for youth
Wcag
Humanitarian programme cycle 2021
Afjrotc ribbons
Longevity ribbon jrotc
Humanitarian engineers in cape york
International humanitarian law icrc
International humanitarian law icrc
International humanitarian law notes
Khalifa humanitarian foundation
Domistan
Humanitarian work psychology
Snob appeal propaganda
The chief determinant of popularity in high school is
Bias by photos captions and camera angles examples
Bias by photos captions and camera angles examples
Conventional crowd
Dispersed collective behavior
Many crowd the savior's kingdom
Pueblo colorado gangs
Bias through selection and omission
Machine platform crowd summary