Chapter Twelve Fundamentals of Data Analysis Preparing the
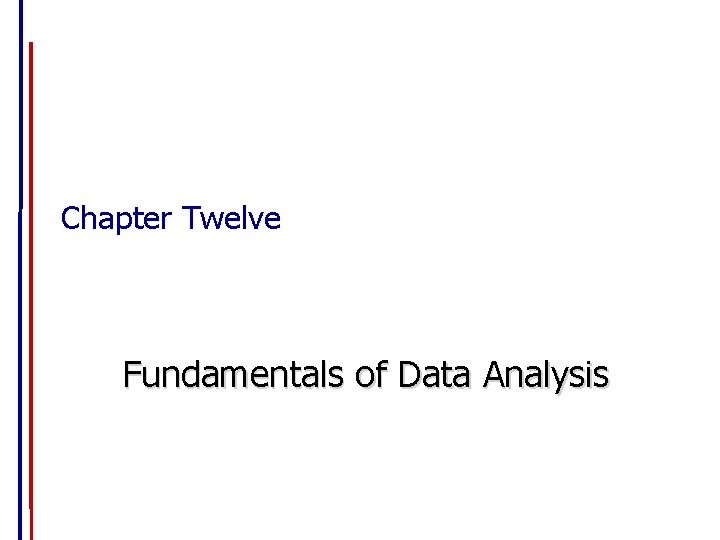
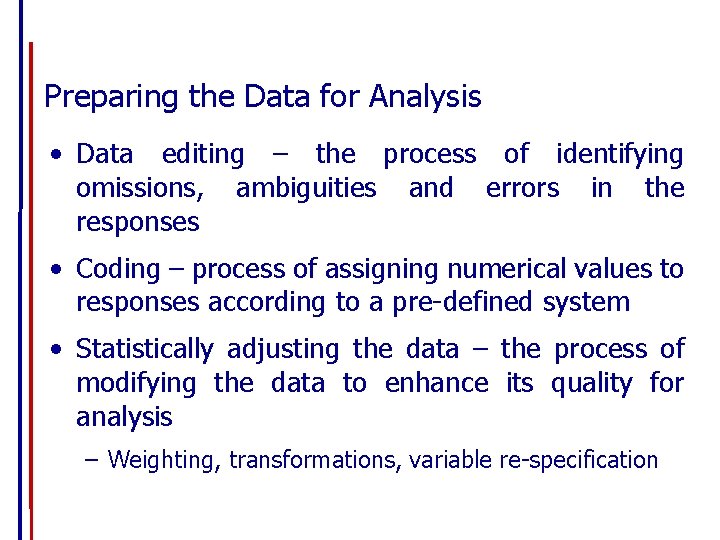
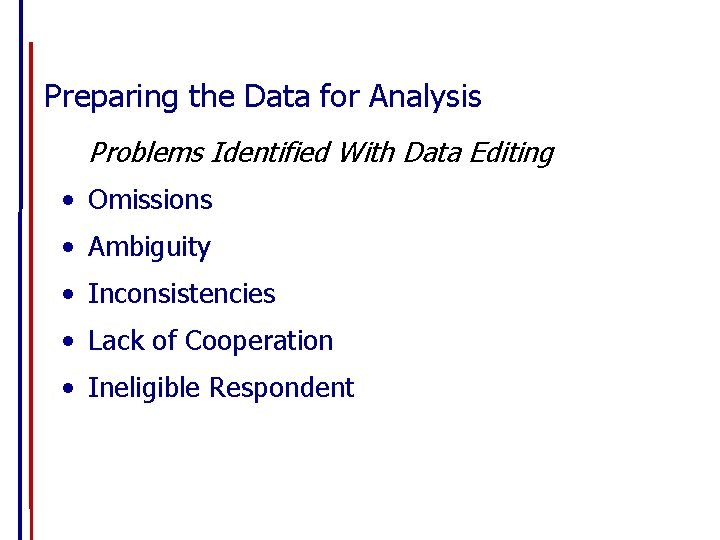
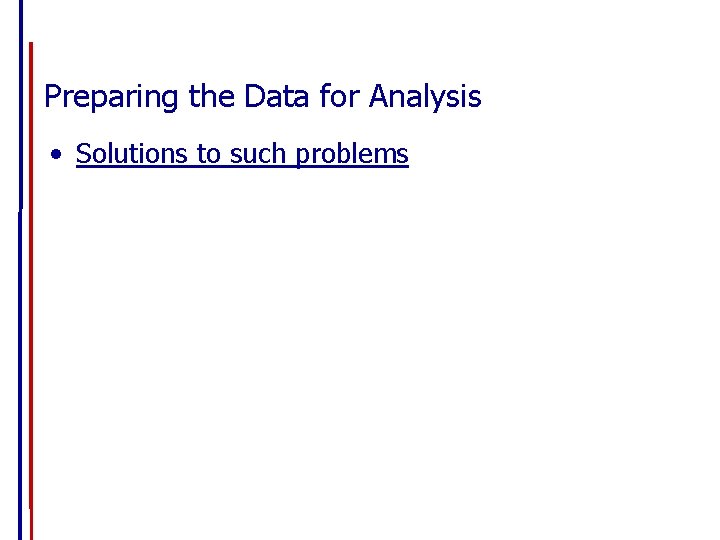
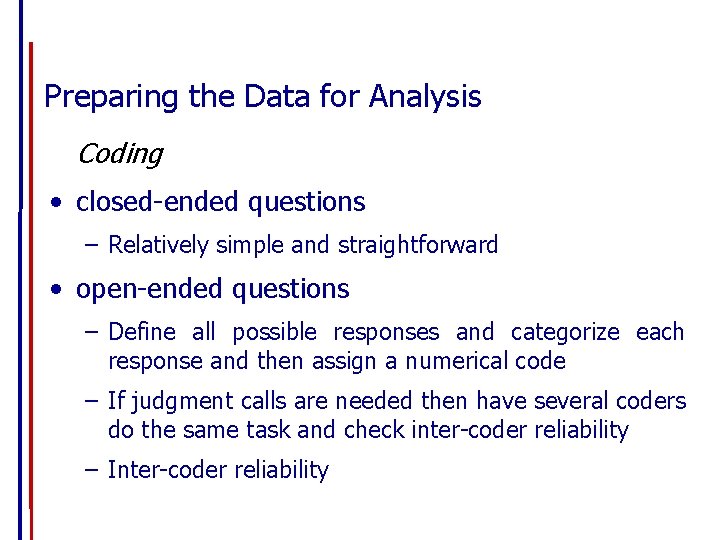
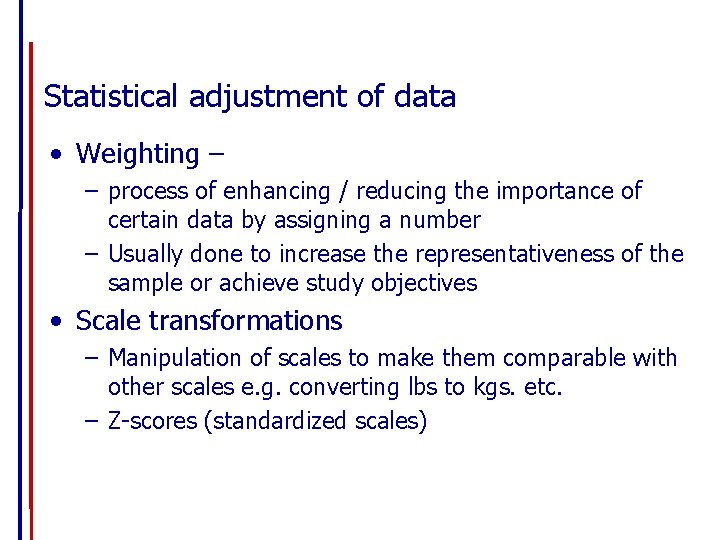
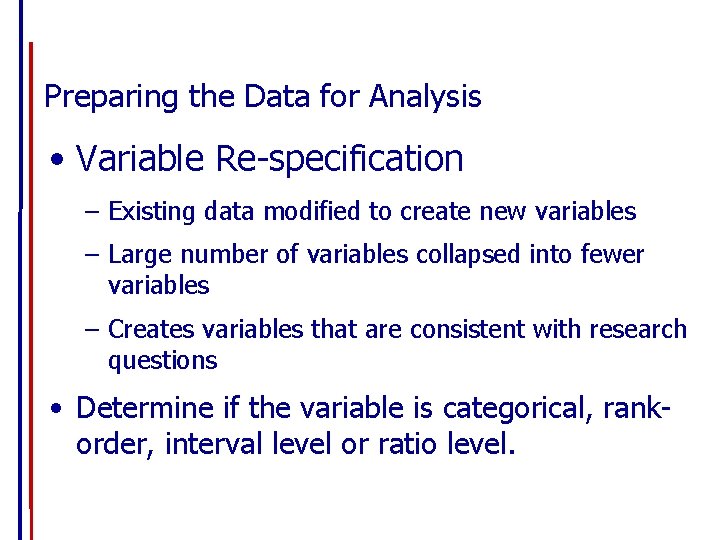
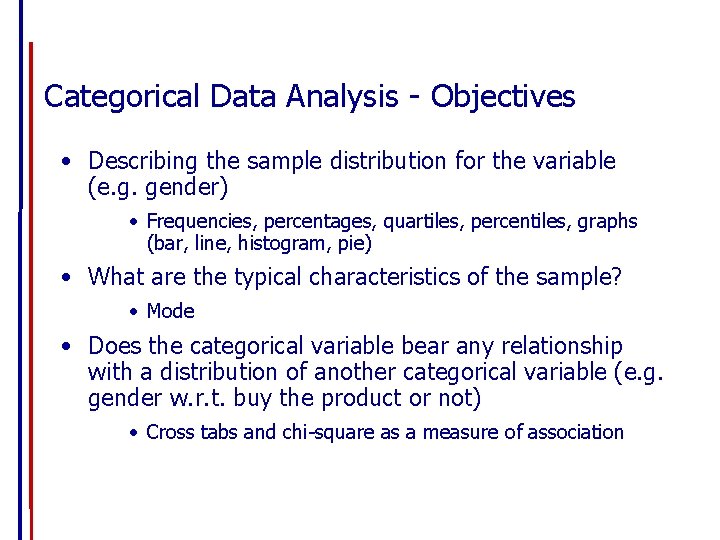
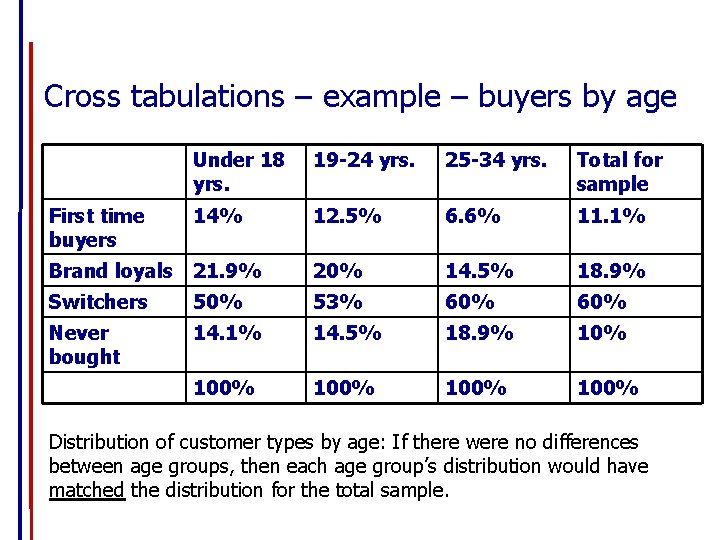
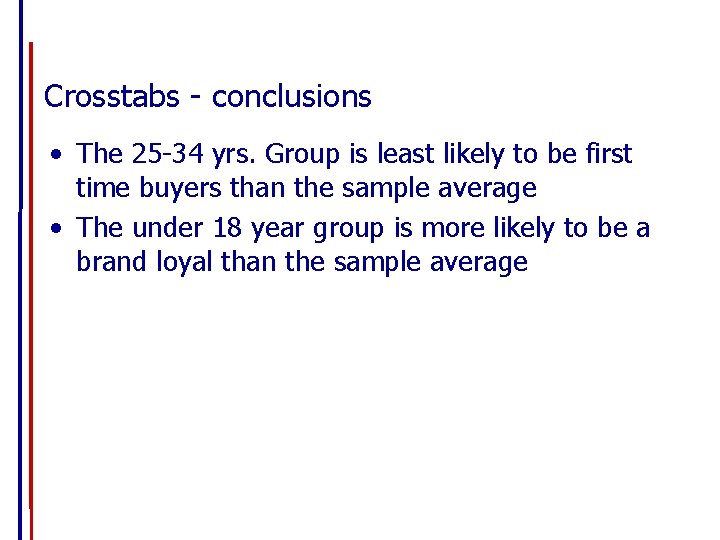
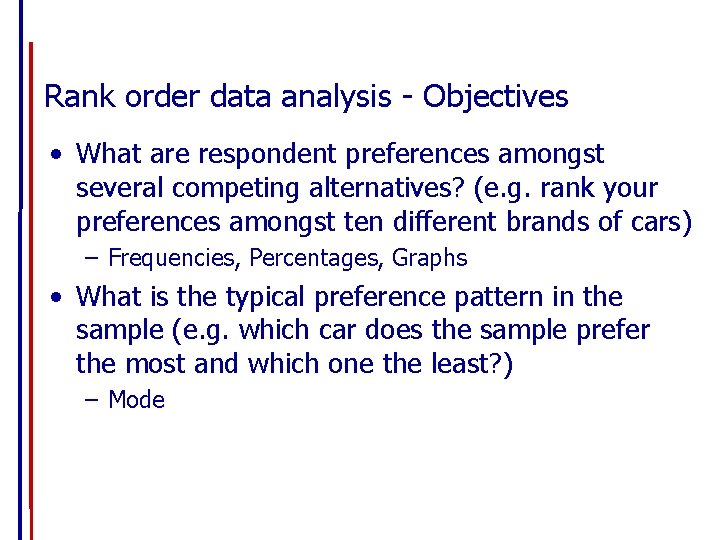
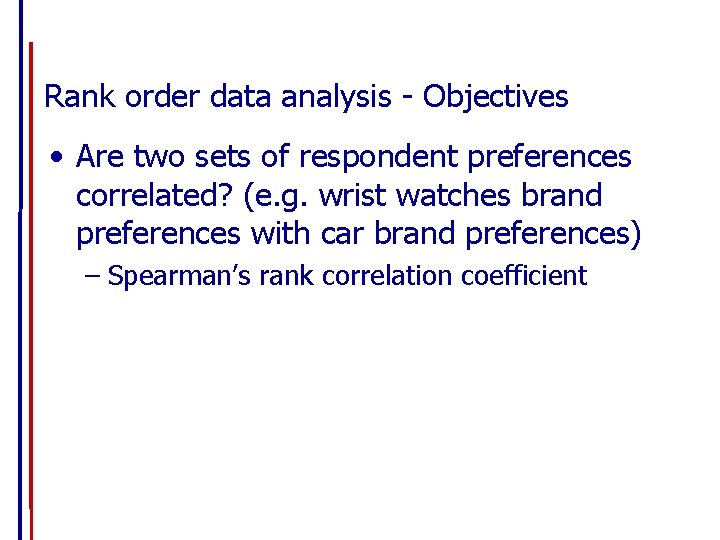
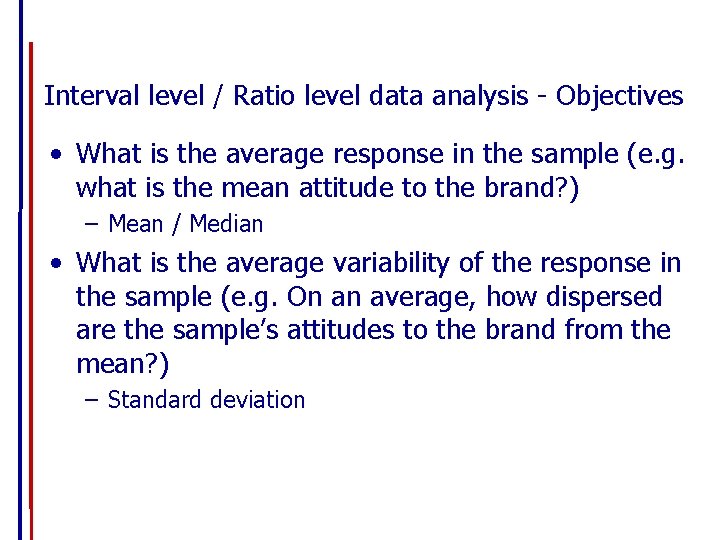
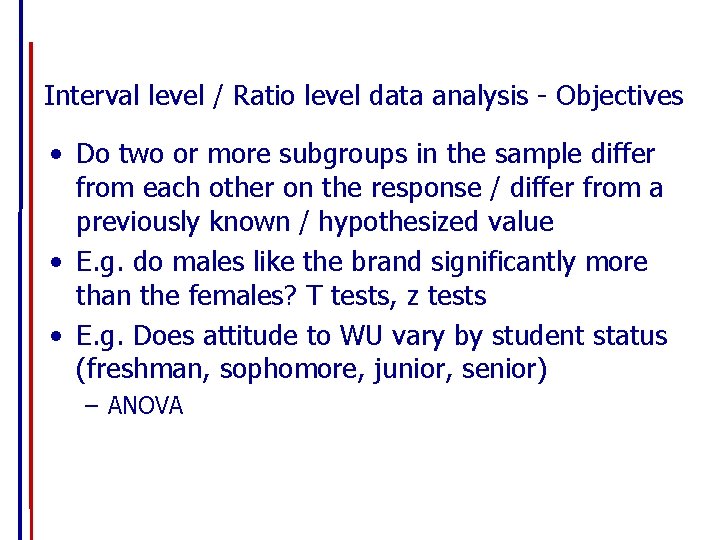
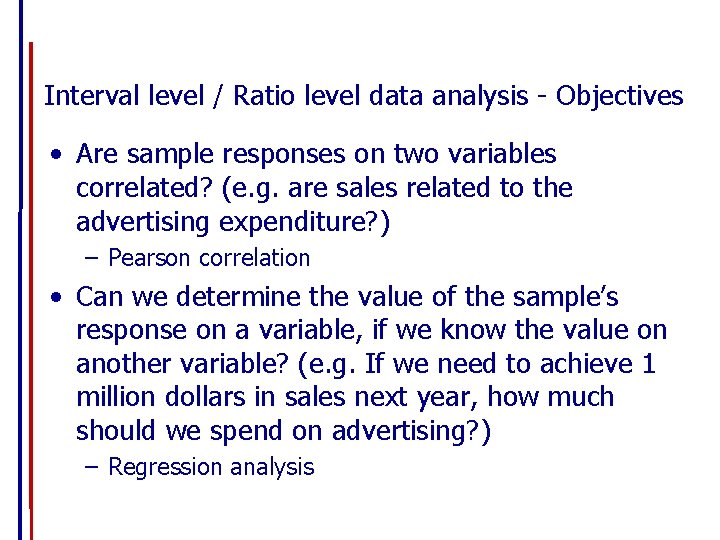
- Slides: 15
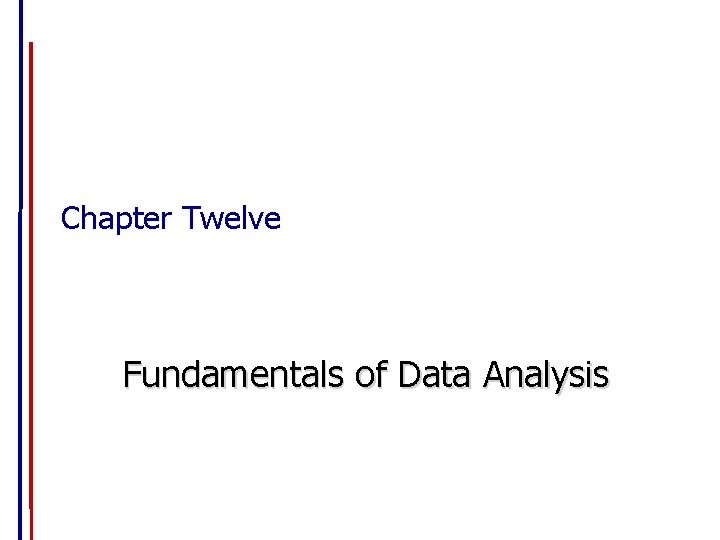
Chapter Twelve Fundamentals of Data Analysis
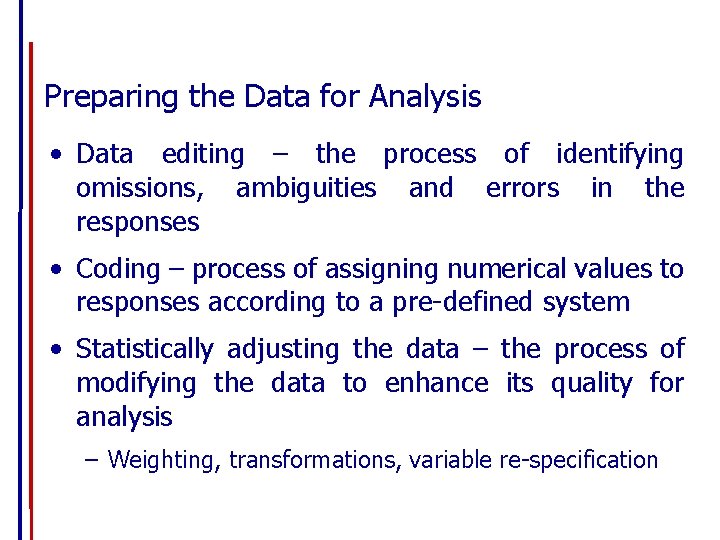
Preparing the Data for Analysis • Data editing – the process of identifying omissions, ambiguities and errors in the responses • Coding – process of assigning numerical values to responses according to a pre-defined system • Statistically adjusting the data – the process of modifying the data to enhance its quality for analysis – Weighting, transformations, variable re-specification
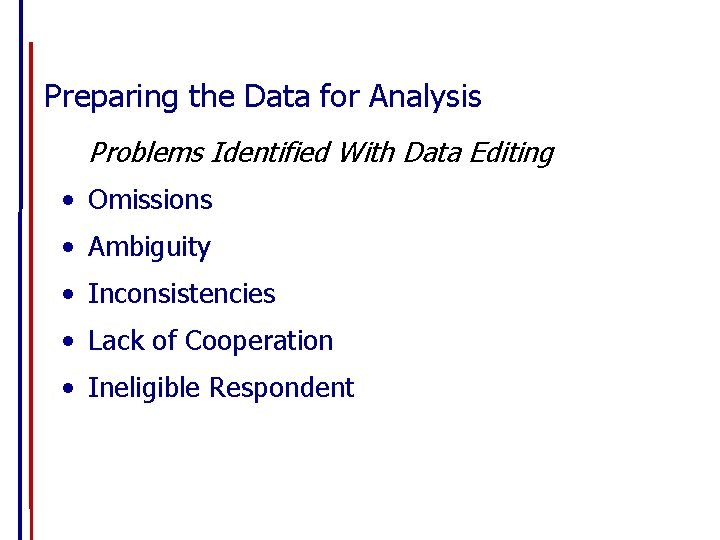
Preparing the Data for Analysis Problems Identified With Data Editing • Omissions • Ambiguity • Inconsistencies • Lack of Cooperation • Ineligible Respondent
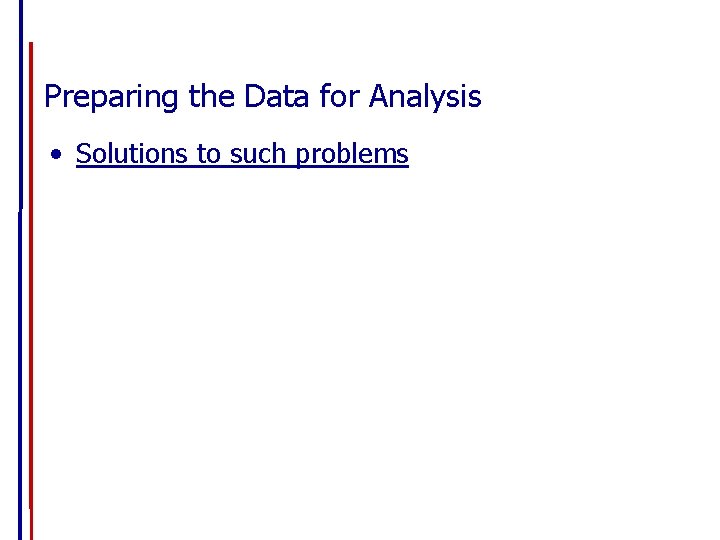
Preparing the Data for Analysis • Solutions to such problems
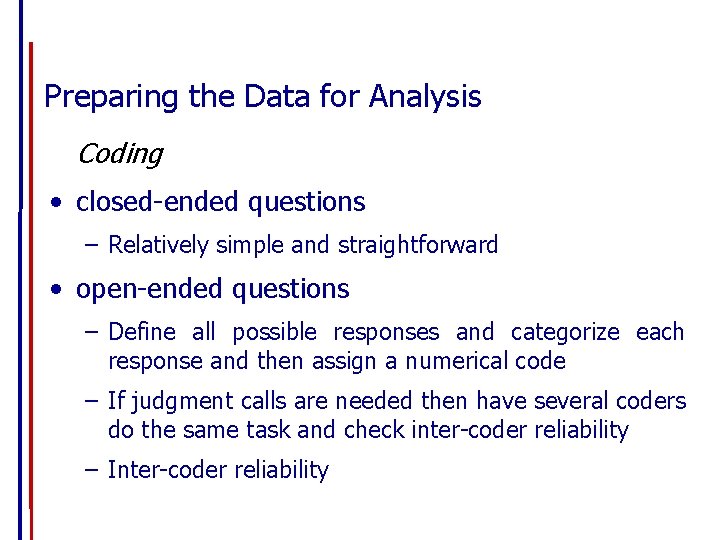
Preparing the Data for Analysis Coding • closed-ended questions – Relatively simple and straightforward • open-ended questions – Define all possible responses and categorize each response and then assign a numerical code – If judgment calls are needed then have several coders do the same task and check inter-coder reliability – Inter-coder reliability
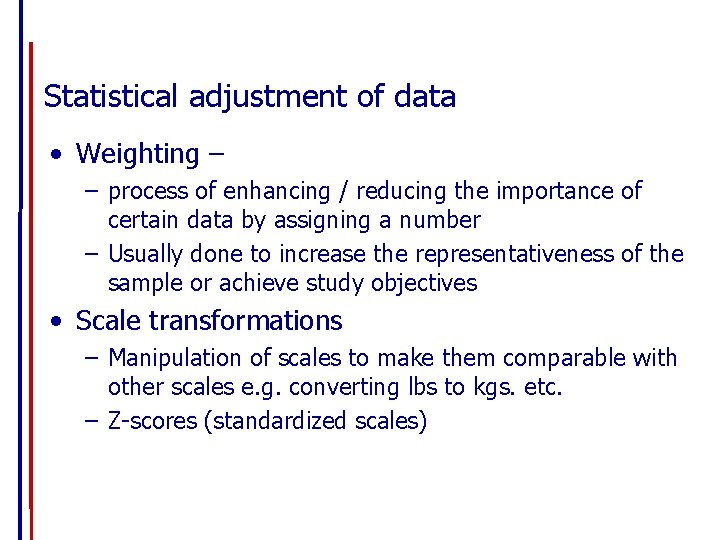
Statistical adjustment of data • Weighting – – process of enhancing / reducing the importance of certain data by assigning a number – Usually done to increase the representativeness of the sample or achieve study objectives • Scale transformations – Manipulation of scales to make them comparable with other scales e. g. converting lbs to kgs. etc. – Z-scores (standardized scales)
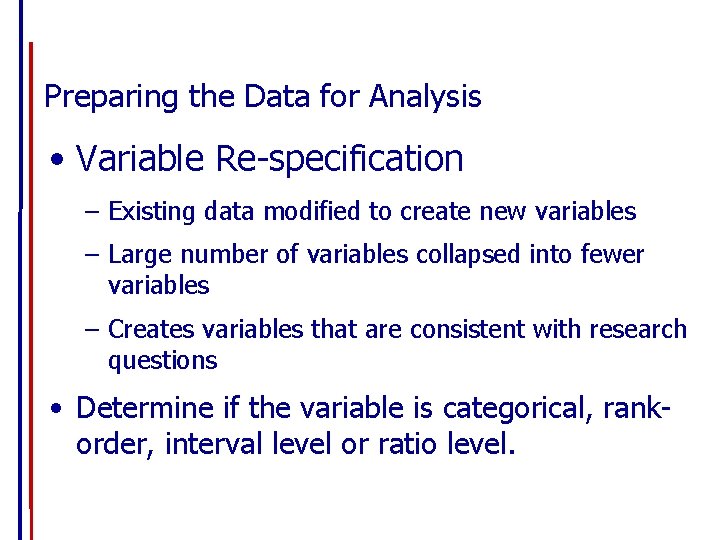
Preparing the Data for Analysis • Variable Re-specification – Existing data modified to create new variables – Large number of variables collapsed into fewer variables – Creates variables that are consistent with research questions • Determine if the variable is categorical, rankorder, interval level or ratio level.
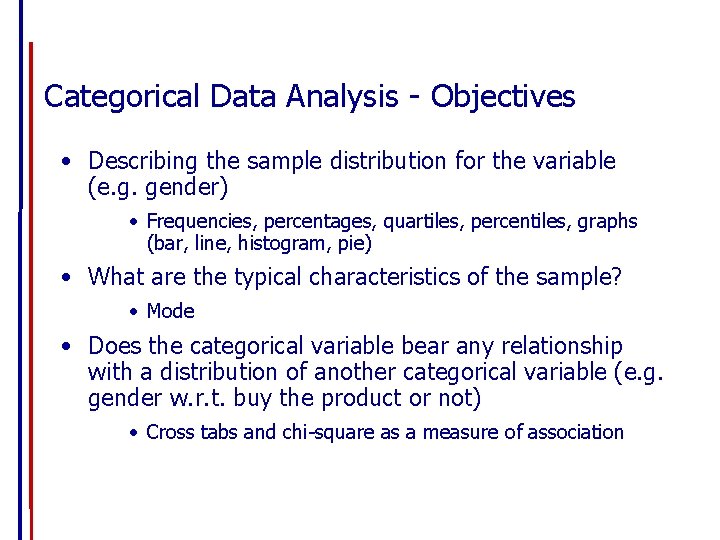
Categorical Data Analysis - Objectives • Describing the sample distribution for the variable (e. g. gender) • Frequencies, percentages, quartiles, percentiles, graphs (bar, line, histogram, pie) • What are the typical characteristics of the sample? • Mode • Does the categorical variable bear any relationship with a distribution of another categorical variable (e. g. gender w. r. t. buy the product or not) • Cross tabs and chi-square as a measure of association
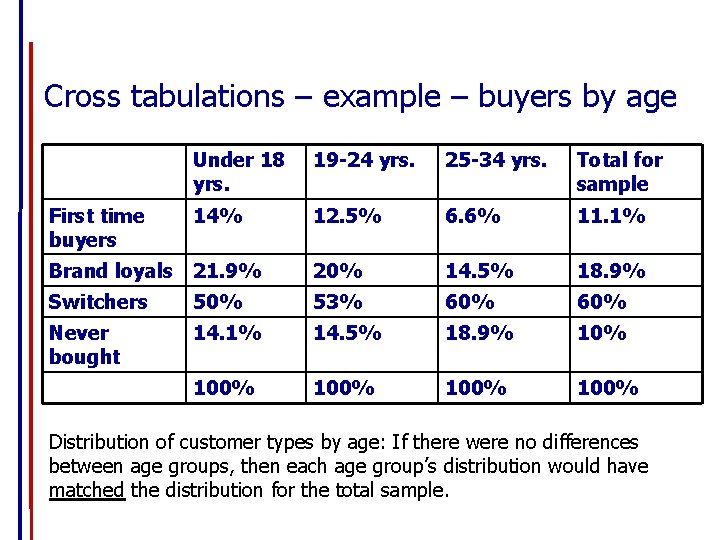
Cross tabulations – example – buyers by age Under 18 yrs. 19 -24 yrs. 25 -34 yrs. Total for sample First time buyers 14% 12. 5% 6. 6% 11. 1% Brand loyals 21. 9% 20% 14. 5% 18. 9% Switchers 50% 53% 60% Never bought 14. 1% 14. 5% 18. 9% 100% 100% Distribution of customer types by age: If there were no differences between age groups, then each age group’s distribution would have matched the distribution for the total sample.
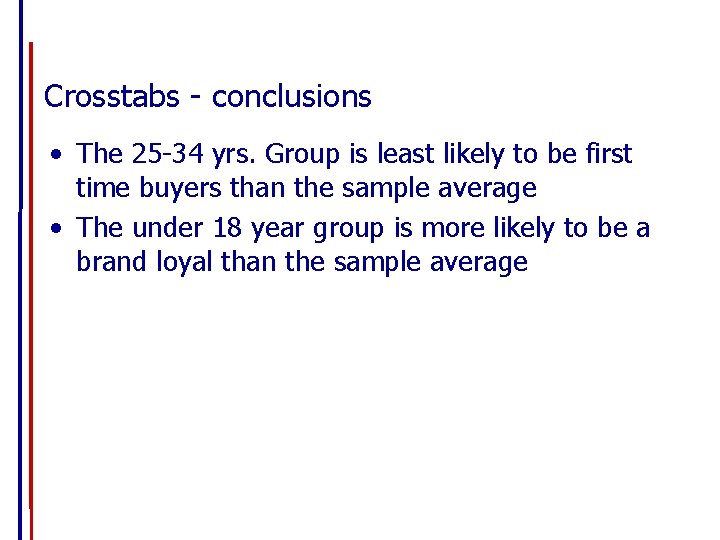
Crosstabs - conclusions • The 25 -34 yrs. Group is least likely to be first time buyers than the sample average • The under 18 year group is more likely to be a brand loyal than the sample average
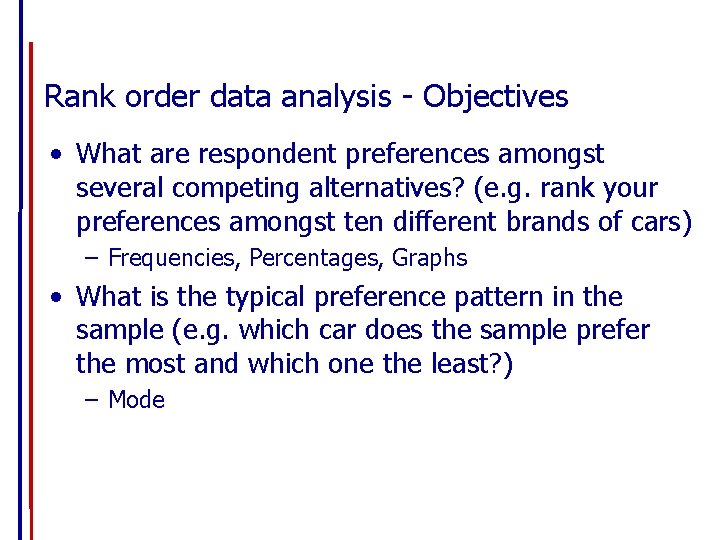
Rank order data analysis - Objectives • What are respondent preferences amongst several competing alternatives? (e. g. rank your preferences amongst ten different brands of cars) – Frequencies, Percentages, Graphs • What is the typical preference pattern in the sample (e. g. which car does the sample prefer the most and which one the least? ) – Mode
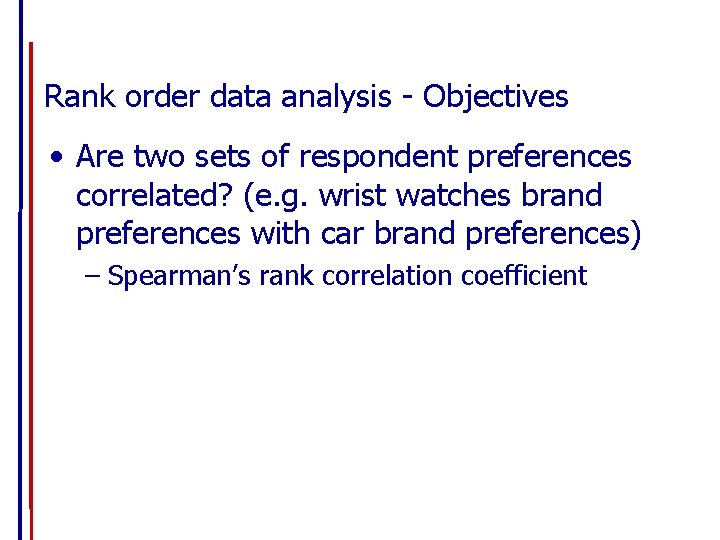
Rank order data analysis - Objectives • Are two sets of respondent preferences correlated? (e. g. wrist watches brand preferences with car brand preferences) – Spearman’s rank correlation coefficient
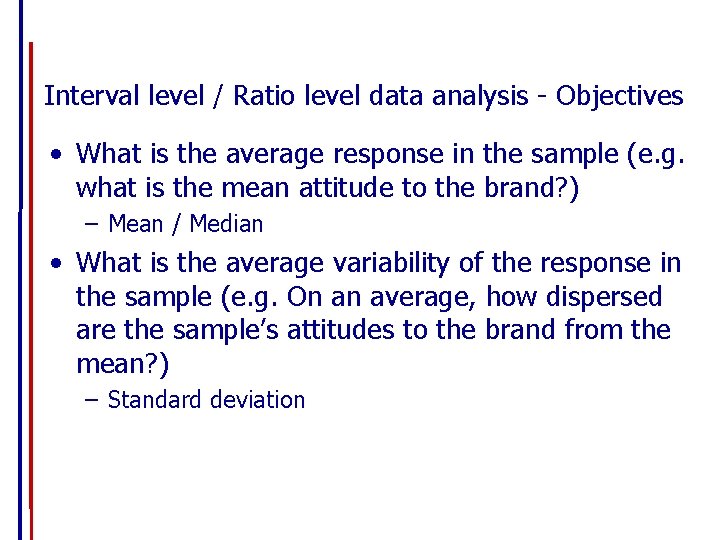
Interval level / Ratio level data analysis - Objectives • What is the average response in the sample (e. g. what is the mean attitude to the brand? ) – Mean / Median • What is the average variability of the response in the sample (e. g. On an average, how dispersed are the sample’s attitudes to the brand from the mean? ) – Standard deviation
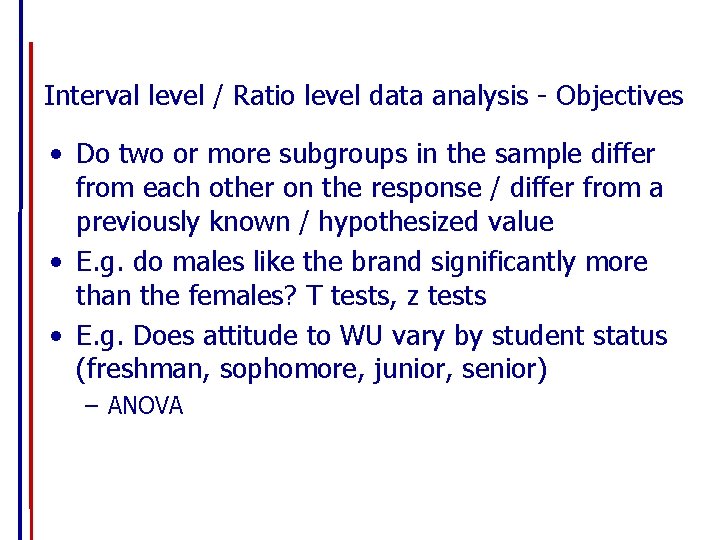
Interval level / Ratio level data analysis - Objectives • Do two or more subgroups in the sample differ from each other on the response / differ from a previously known / hypothesized value • E. g. do males like the brand significantly more than the females? T tests, z tests • E. g. Does attitude to WU vary by student status (freshman, sophomore, junior, senior) – ANOVA
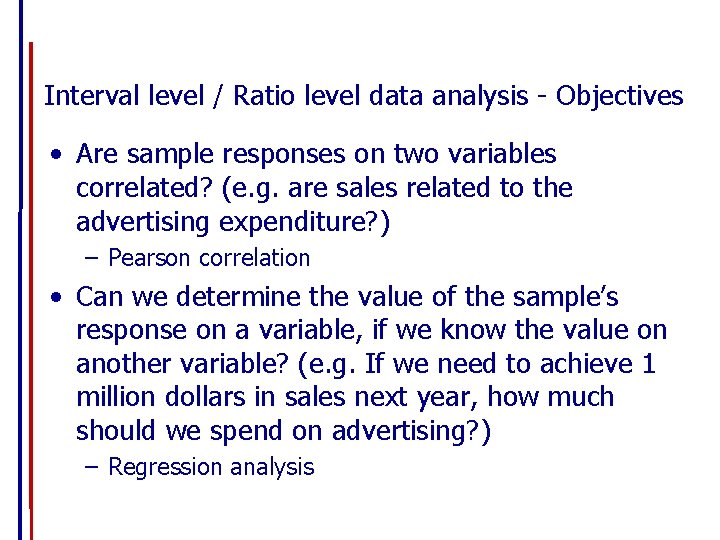
Interval level / Ratio level data analysis - Objectives • Are sample responses on two variables correlated? (e. g. are sales related to the advertising expenditure? ) – Pearson correlation • Can we determine the value of the sample’s response on a variable, if we know the value on another variable? (e. g. If we need to achieve 1 million dollars in sales next year, how much should we spend on advertising? ) – Regression analysis
Whether the weather tongue twister
Preparing data for analysis in research
Fundamental data analysis
Data preparing exploring examining and displaying
Chapter 18.4 participating in a job interview
Chapter 17:1 developing job-keeping skills
Chapter 18 preparing for the world of work
Chapter 15 preparing for careers
Part two—preparing a chart of accounts
Chapter 1 preparing your path to success
Milady chapter 30
Marketing essentials meaning
Hilbert space
Mathematical analysis of non-recursive algorithms
The 7
What are the terrible twelve water pollution