Veryhighresolution machine learning classifications and snow covered area
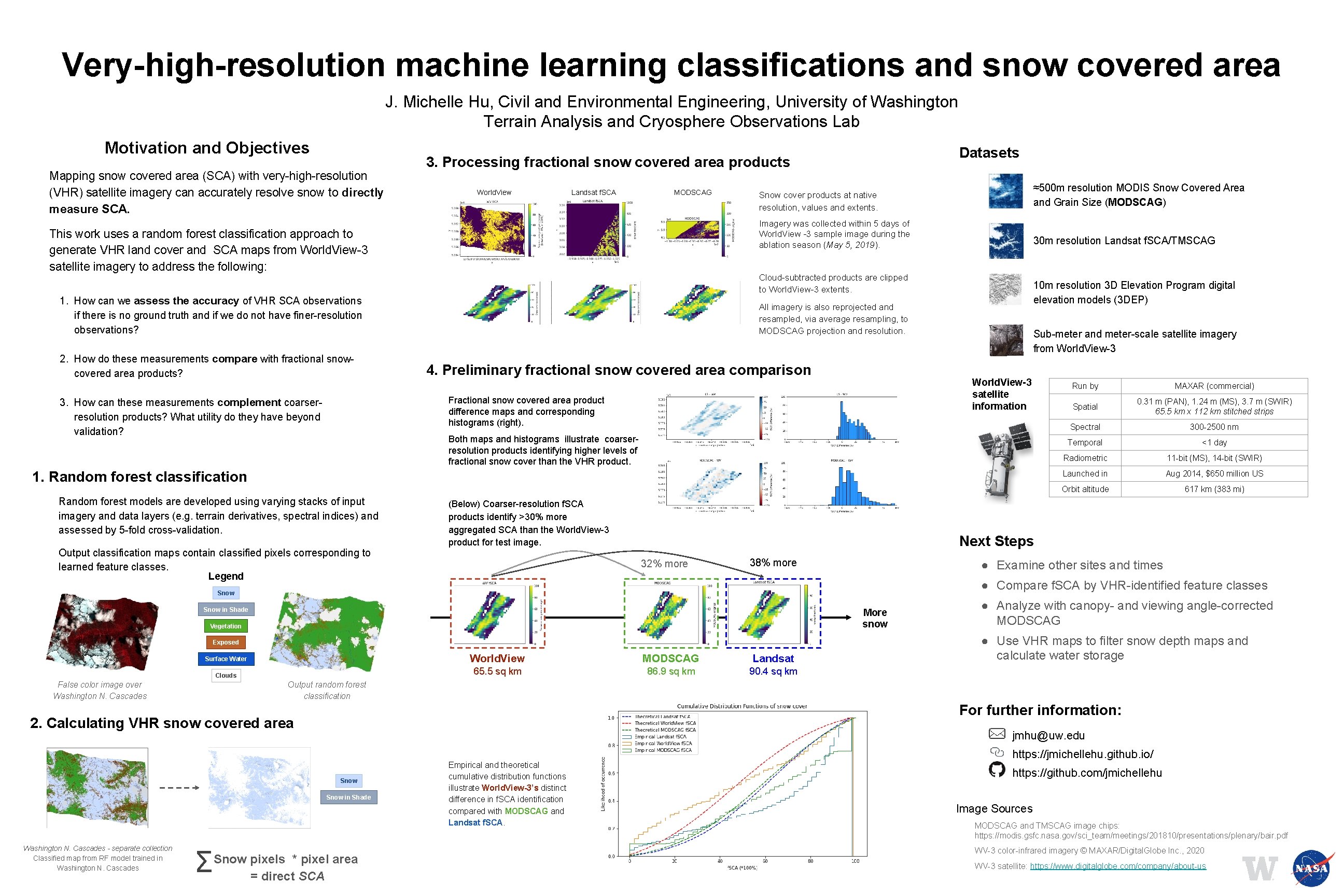
- Slides: 1
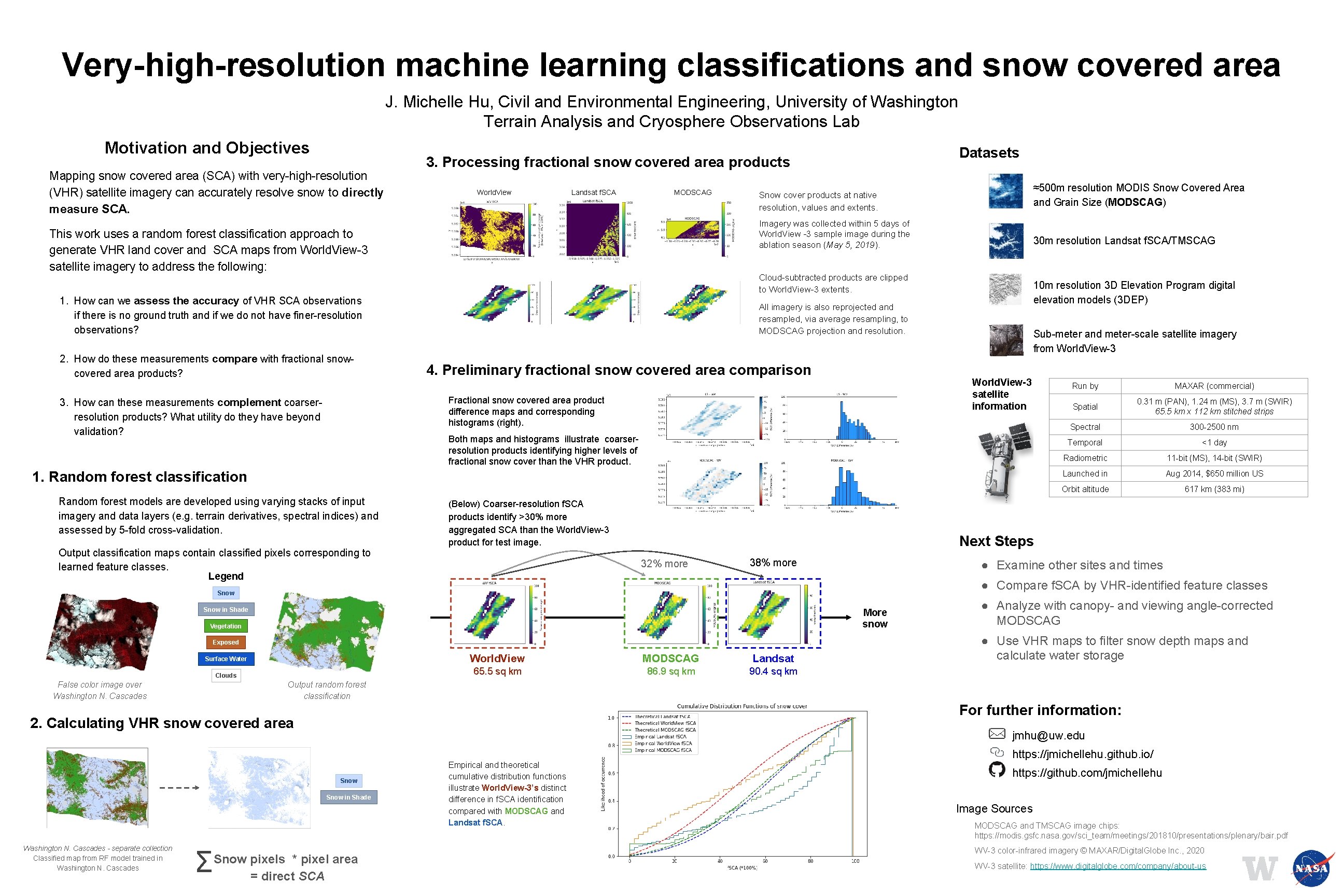
Very-high-resolution machine learning classifications and snow covered area J. Michelle Hu, Civil and Environmental Engineering, University of Washington Terrain Analysis and Cryosphere Observations Lab Motivation and Objectives Mapping snow covered area (SCA) with very-high-resolution (VHR) satellite imagery can accurately resolve snow to directly measure SCA. 3. Processing fractional snow covered area products World. View Landsat f. SCA MODSCAG This work uses a random forest classification approach to generate VHR land cover and SCA maps from World. View-3 satellite imagery to address the following: Snow cover products at native resolution, values and extents. ≈500 m resolution MODIS Snow Covered Area and Grain Size (MODSCAG) Imagery was collected within 5 days of World. View -3 sample image during the ablation season (May 5, 2019). 30 m resolution Landsat f. SCA/TMSCAG Cloud-subtracted products are clipped to World. View-3 extents. 1. How can we assess the accuracy of VHR SCA observations if there is no ground truth and if we do not have finer-resolution observations? 2. How do these measurements compare with fractional snowcovered area products? Datasets All imagery is also reprojected and resampled, via average resampling, to MODSCAG projection and resolution. 4. Preliminary fractional snow covered area comparison Sub-meter and meter-scale satellite imagery from World. View-3 satellite information Fractional snow covered area product difference maps and corresponding histograms (right). 3. How can these measurements complement coarserresolution products? What utility do they have beyond validation? 10 m resolution 3 D Elevation Program digital elevation models (3 DEP) Both maps and histograms illustrate coarserresolution products identifying higher levels of fractional snow cover than the VHR product. 1. Random forest classification Random forest models are developed using varying stacks of input imagery and data layers (e. g. terrain derivatives, spectral indices) and assessed by 5 -fold cross-validation. Output classification maps contain classified pixels corresponding to learned feature classes. (Below) Coarser-resolution f. SCA products identify >30% more aggregated SCA than the World. View-3 product for test image. MAXAR (commercial) Spatial 0. 31 m (PAN), 1. 24 m (MS), 3. 7 m (SWIR) 65. 5 km x 112 km stitched strips Spectral 300 -2500 nm Temporal <1 day Radiometric 11 -bit (MS), 14 -bit (SWIR) Launched in Aug 2014, $650 million US Orbit altitude 617 km (383 mi) Next Steps 32% more 38% more ● Examine other sites and times Legend ● Compare f. SCA by VHR-identified feature classes Snow in Shade More snow Vegetation Exposed Surface Water World. View MODSCAG Landsat Clouds 65. 5 sq km 86. 9 sq km 90. 4 sq km False color image over Washington N. Cascades Run by ● Analyze with canopy- and viewing angle-corrected MODSCAG ● Use VHR maps to filter snow depth maps and calculate water storage Output random forest classification For further information: 2. Calculating VHR snow covered area jmhu@uw. edu https: //jmichellehu. github. io/ Snow in Shade Washington N. Cascades - separate collection Classified map from RF model trained in Washington N. Cascades Σ Snow pixels * pixel area = direct SCA Empirical and theoretical cumulative distribution functions illustrate World. View-3’s distinct difference in f. SCA identification compared with MODSCAG and Landsat f. SCA. https: //github. com/jmichellehu Image Sources MODSCAG and TMSCAG image chips: https: //modis. gsfc. nasa. gov/sci_team/meetings/201810/presentations/plenary/bair. pdf WV-3 color-infrared imagery © MAXAR/Digital. Globe Inc. , 2020 WV-3 satellite: https: //www. digitalglobe. com/company/about-us