The Multiway Relay Channel Deniz Gndz Aylin Yener
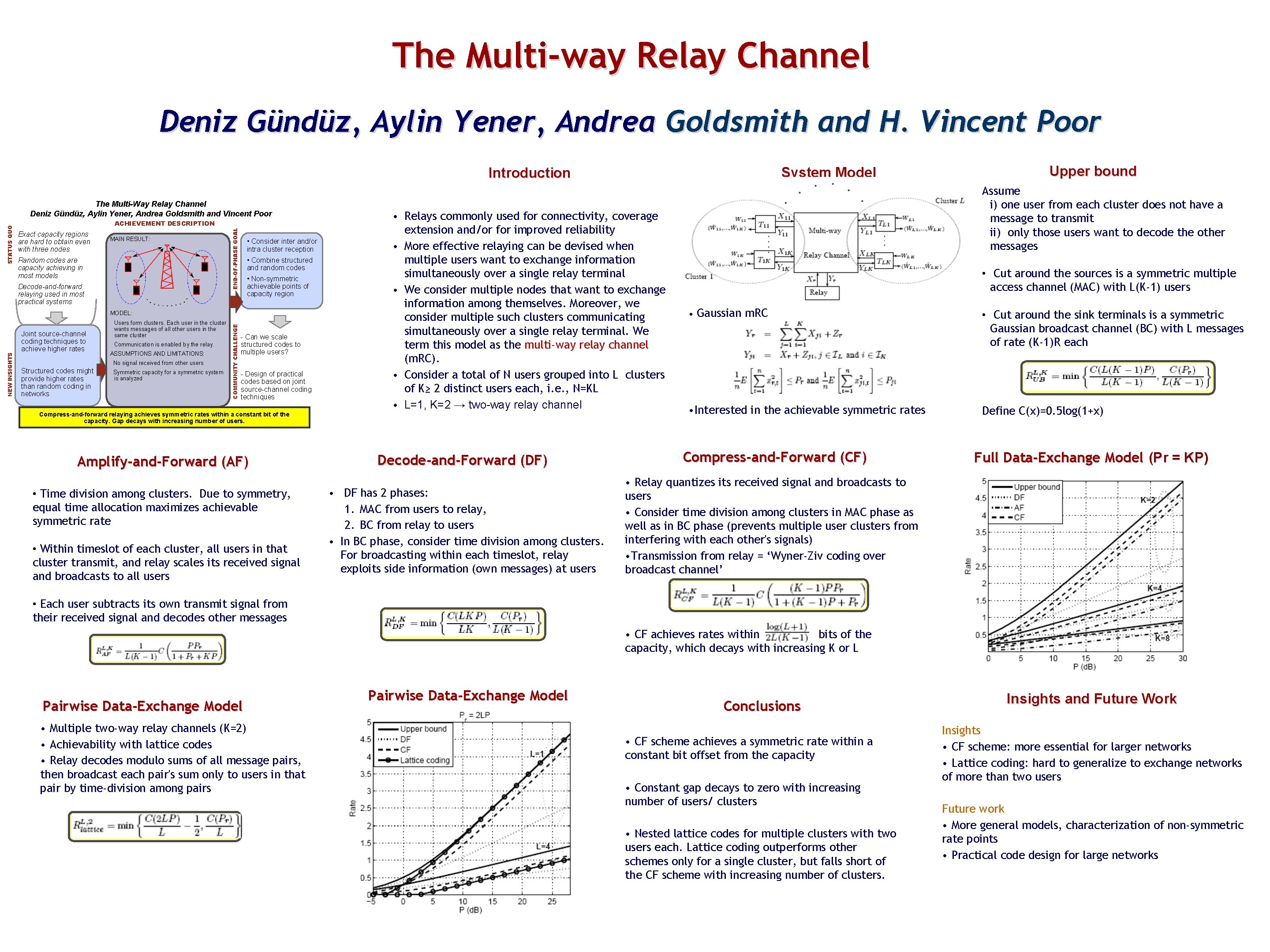
- Slides: 1
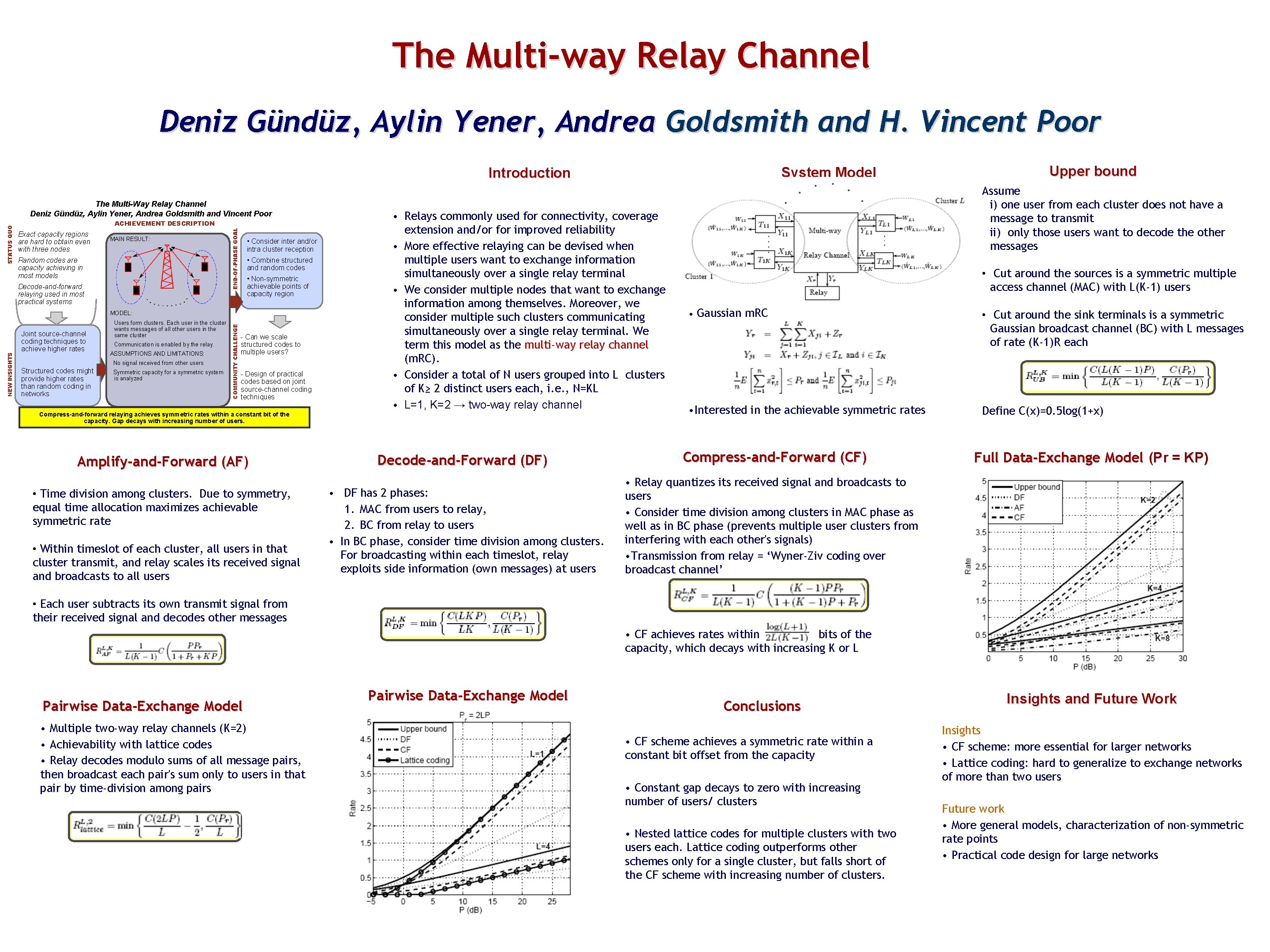
The Multi-way Relay Channel Deniz Gündüz, Aylin Yener, Andrea Goldsmith and H. Vincent Poor System Model Introduction ACHIEVEMENT DESCRIPTION Exact capacity regions are hard to obtain even with three nodes MAIN RESULT: Random codes are capacity achieving in most models Decode-and-forward relaying used in most practical systems END-OF-PHASE GOAL STATUS QUO The Multi-Way Relay Channel Deniz Gündüz, Aylin Yener, Andrea Goldsmith and Vincent Poor • Consider inter and/or intra cluster reception • Combine structured and random codes • Non-symmetric achievable points of capacity region Joint source-channel coding techniques to achieve higher rates Users form clusters. Each user in the cluster wants messages of all other users in the same cluster Communication is enabled by the relay. ASSUMPTIONS AND LIMITATIONS: No signal received from other users Structured codes might provide higher rates than random coding in networks Symmetric capacity for a symmetric system is analyzed COMMUNITY CHALLENGE NEW INSIGHTS MODEL: - Can we scale structured codes to multiple users? - Design of practical codes based on joint source-channel coding techniques • Relays commonly used for connectivity, coverage extension and/or for improved reliability • More effective relaying can be devised when multiple users want to exchange information simultaneously over a single relay terminal • We consider multiple nodes that want to exchange information among themselves. Moreover, we consider multiple such clusters communicating simultaneously over a single relay terminal. We term this model as the multi-way relay channel (m. RC). • Consider a total of N users grouped into L clusters of K≥ 2 distinct users each, i. e. , N=KL • L=1, K=2 → two-way relay channel Compress-and-forward relaying achieves symmetric rates within a constant bit of the capacity. Gap decays with increasing number of users. Amplify-and-Forward (AF) • Time division among clusters. Due to symmetry, equal time allocation maximizes achievable symmetric rate • Within timeslot of each cluster, all users in that cluster transmit, and relay scales its received signal and broadcasts to all users Decode-and-Forward (DF) • DF has 2 phases: 1. MAC from users to relay, 2. BC from relay to users • In BC phase, consider time division among clusters. For broadcasting within each timeslot, relay exploits side information (own messages) at users Upper bound Assume i) one user from each cluster does not have a message to transmit ii) only those users want to decode the other messages • Cut around the sources is a symmetric multiple access channel (MAC) with L(K-1) users • Gaussian m. RC • Cut around the sink terminals is a symmetric Gaussian broadcast channel (BC) with L messages of rate (K-1)R each • Interested in the achievable symmetric rates Define C(x)=0. 5 log(1+x) Compress-and-Forward (CF) Full Data-Exchange Model (Pr = KP) • Relay quantizes its received signal and broadcasts to users • Consider time division among clusters in MAC phase as well as in BC phase (prevents multiple user clusters from interfering with each other's signals) • Transmission from relay = ‘Wyner-Ziv coding over broadcast channel’ • Each user subtracts its own transmit signal from their received signal and decodes other messages • CF achieves rates within bits of the capacity, which decays with increasing K or L Pairwise Data-Exchange Model • Multiple two-way relay channels (K=2) • Achievability with lattice codes • Relay decodes modulo sums of all message pairs, then broadcast each pair's sum only to users in that pair by time-division among pairs Pairwise Data-Exchange Model Conclusions • CF scheme achieves a symmetric rate within a constant bit offset from the capacity • Constant gap decays to zero with increasing number of users/ clusters • Nested lattice codes for multiple clusters with two users each. Lattice coding outperforms other schemes only for a single cluster, but falls short of the CF scheme with increasing number of clusters. Insights and Future Work Insights • CF scheme: more essential for larger networks • Lattice coding: hard to generalize to exchange networks of more than two users Future work • More general models, characterization of non-symmetric rate points • Practical code design for large networks