SOIL THERMAL DIFFUSIVITY AS RELATED TO WATER CONTENT
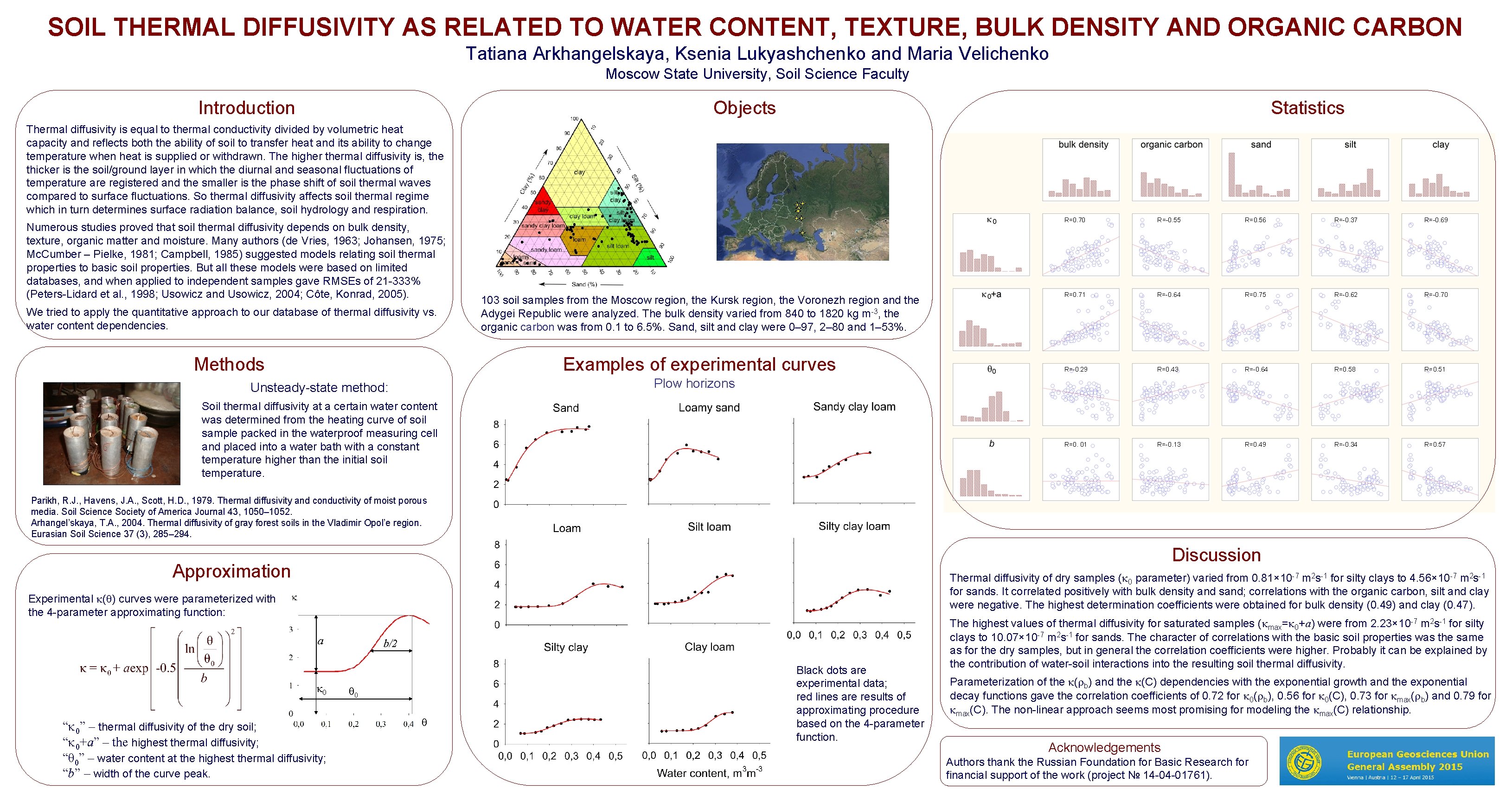
- Slides: 1
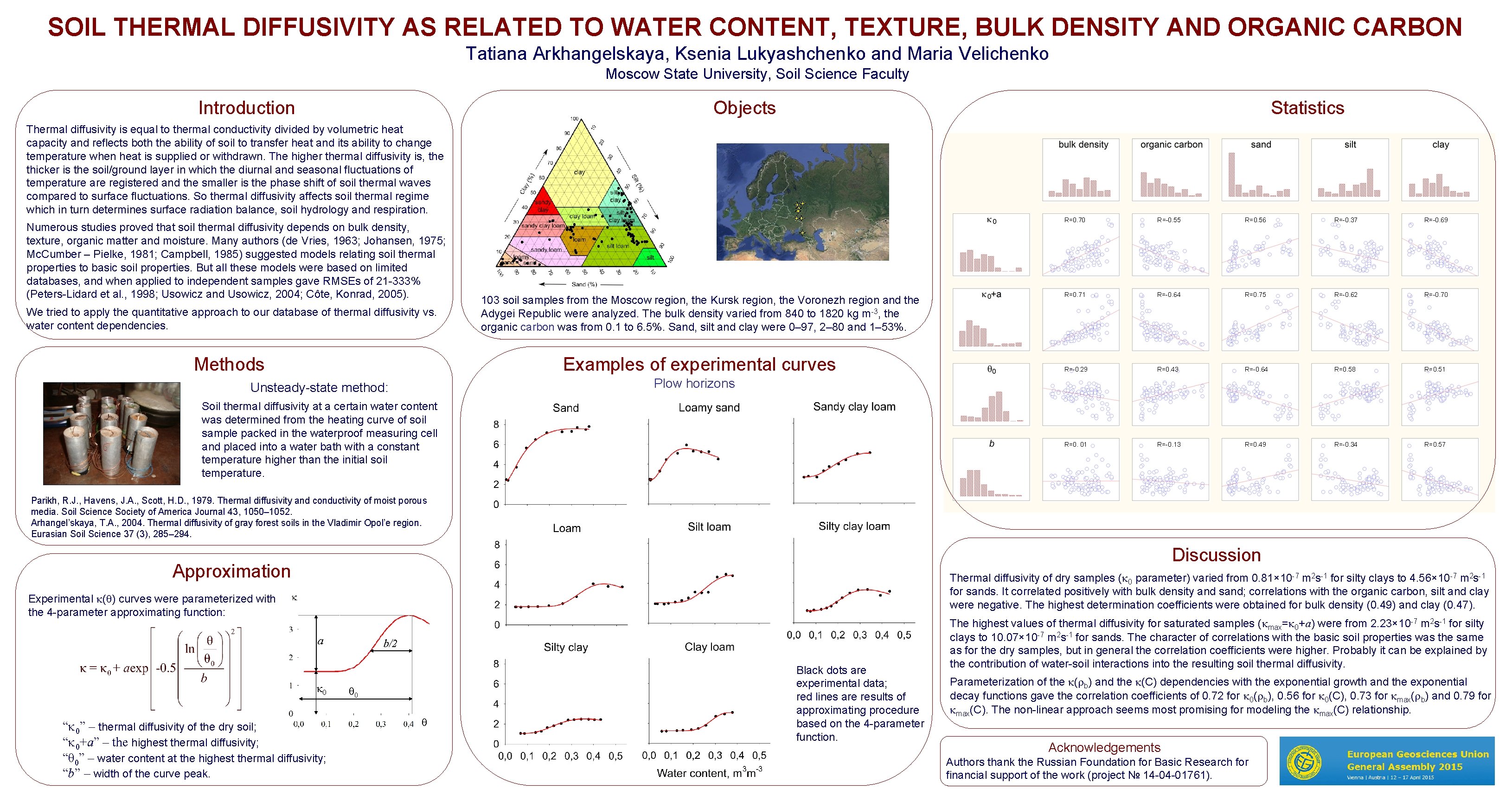
SOIL THERMAL DIFFUSIVITY AS RELATED TO WATER CONTENT, TEXTURE, BULK DENSITY AND ORGANIC CARBON Tatiana Arkhangelskaya, Ksenia Lukyashchenko and Maria Velichenko Moscow State University, Soil Science Faculty Introduction Objects Statistics Thermal diffusivity is equal to thermal conductivity divided by volumetric heat capacity and reflects both the ability of soil to transfer heat and its ability to change temperature when heat is supplied or withdrawn. The higher thermal diffusivity is, the thicker is the soil/ground layer in which the diurnal and seasonal fluctuations of temperature are registered and the smaller is the phase shift of soil thermal waves compared to surface fluctuations. So thermal diffusivity affects soil thermal regime which in turn determines surface radiation balance, soil hydrology and respiration. Numerous studies proved that soil thermal diffusivity depends on bulk density, texture, organic matter and moisture. Many authors (de Vries, 1963; Johansen, 1975; Mc. Cumber – Pielke, 1981; Campbell, 1985) suggested models relating soil thermal properties to basic soil properties. But all these models were based on limited databases, and when applied to independent samples gave RMSEs of 21 -333% (Peters-Lidard et al. , 1998; Usowicz and Usowicz, 2004; Côte, Konrad, 2005). We tried to apply the quantitative approach to our database of thermal diffusivity vs. water content dependencies. 103 soil samples from the Moscow region, the Kursk region, the Voronezh region and the Adygei Republic were analyzed. The bulk density varied from 840 to 1820 kg m-3, the organic carbon was from 0. 1 to 6. 5%. Sand, silt and clay were 0– 97, 2– 80 and 1– 53%. Methods Examples of experimental curves R=0. 70 R=-0. 55 R=0. 56 R=-0. 37 R=-0. 69 R=0. 71 R=-0. 64 R=0. 75 R=-0. 62 R=-0. 70 R=-0. 29 R=0. 43 R=-0. 64 R=0. 58 R=0. 51 R=0. 01 R=-0. 13 R=0. 49 R=-0. 34 R=0. 57 Plow horizons Unsteady-state method: Soil thermal diffusivity at a certain water content was determined from the heating curve of soil sample packed in the waterproof measuring cell and placed into a water bath with a constant temperature higher than the initial soil temperature. Parikh, R. J. , Havens, J. A. , Scott, H. D. , 1979. Thermal diffusivity and conductivity of moist porous media. Soil Science Society of America Journal 43, 1050– 1052. Arhangel’skaya, T. A. , 2004. Thermal diffusivity of gray forest soils in the Vladimir Opol’e region. Eurasian Soil Science 37 (3), 285– 294. Discussion Approximation Thermal diffusivity of dry samples (k 0 parameter) varied from 0. 81× 10 -7 m 2 s-1 for silty clays to 4. 56× 10 -7 m 2 s-1 for sands. It correlated positively with bulk density and sand; correlations with the organic carbon, silt and clay were negative. The highest determination coefficients were obtained for bulk density (0. 49) and clay (0. 47). Experimental k(q) curves were parameterized with the 4 -parameter approximating function: a k 0 “k 0” – thermal diffusivity of the dry soil; “k 0+a” – the highest thermal diffusivity; “θ 0” – water content at the highest thermal diffusivity; “b” – width of the curve peak. b/2 q 0 q Black dots are experimental data; red lines are results of approximating procedure based on the 4 -parameter function. The highest values of thermal diffusivity for saturated samples (kmax=k 0+a) were from 2. 23× 10 -7 m 2 s-1 for silty clays to 10. 07× 10 -7 m 2 s-1 for sands. The character of correlations with the basic soil properties was the same as for the dry samples, but in general the correlation coefficients were higher. Probably it can be explained by the contribution of water-soil interactions into the resulting soil thermal diffusivity. Parameterization of the k(rb) and the k(C) dependencies with the exponential growth and the exponential decay functions gave the correlation coefficients of 0. 72 for k 0(rb), 0. 56 for k 0(C), 0. 73 for kmax(rb) and 0. 79 for kmax(C). The non-linear approach seems most promising for modeling the kmax(C) relationship. Acknowledgements Authors thank the Russian Foundation for Basic Research for financial support of the work (project № 14 -04 -01761).