Searching Large Scientific Data John Wu Scientific Data
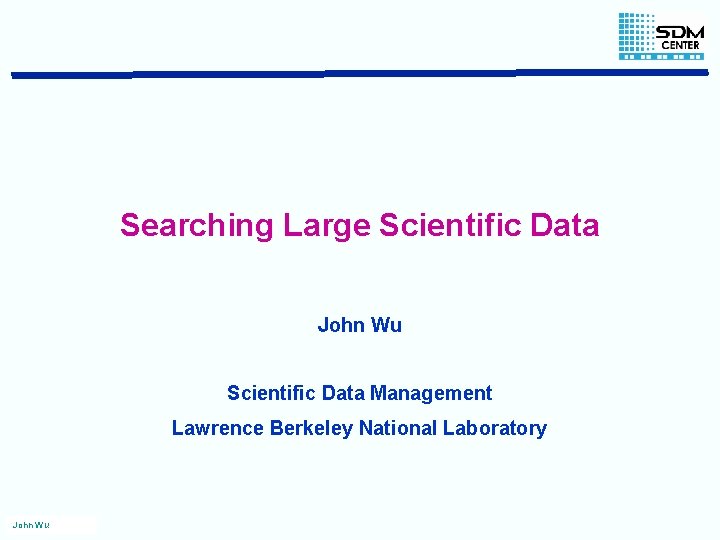
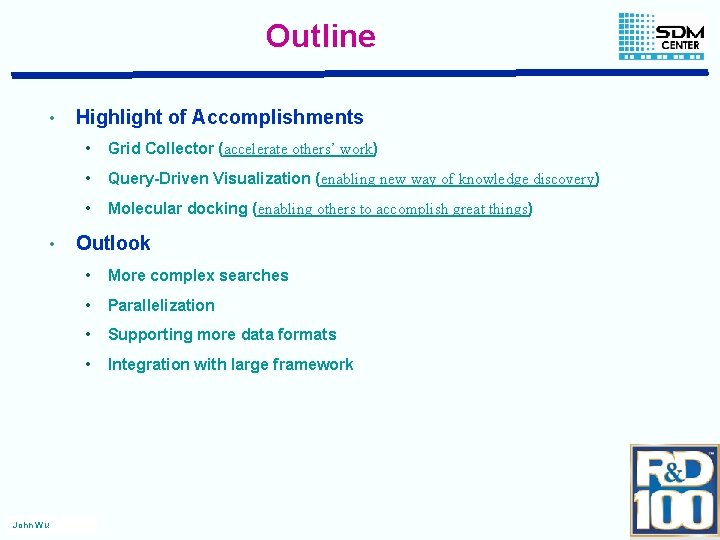
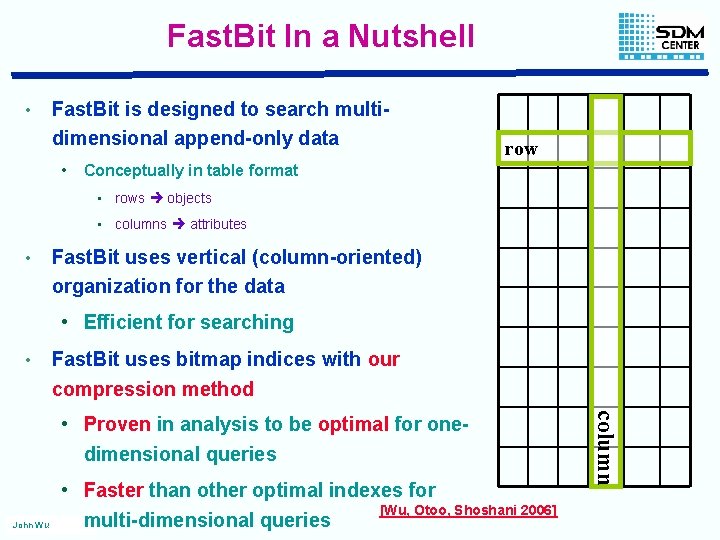
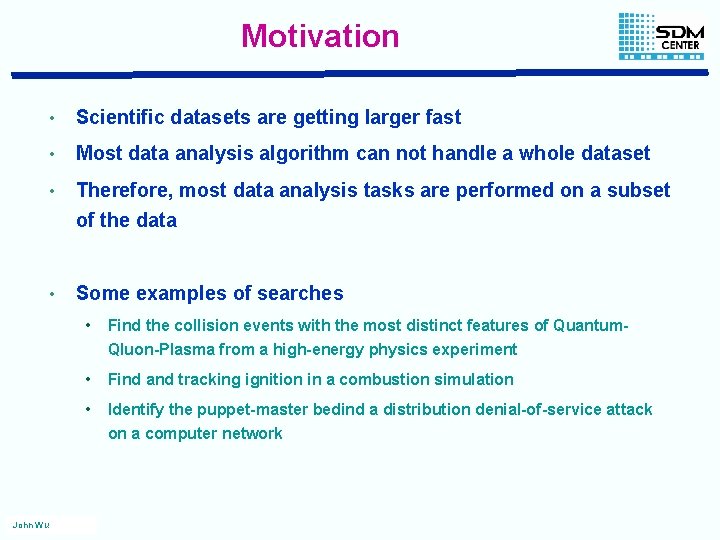
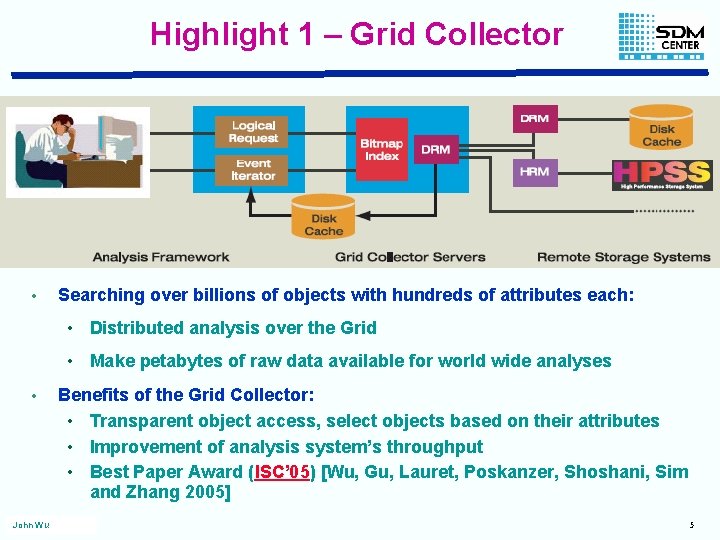
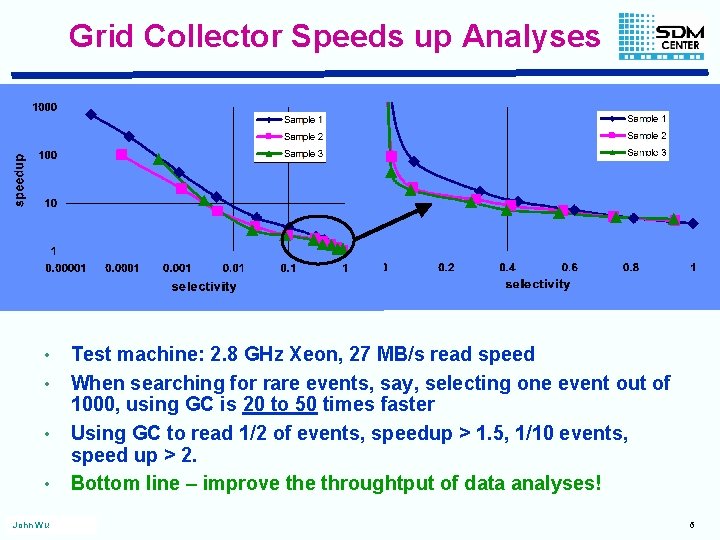
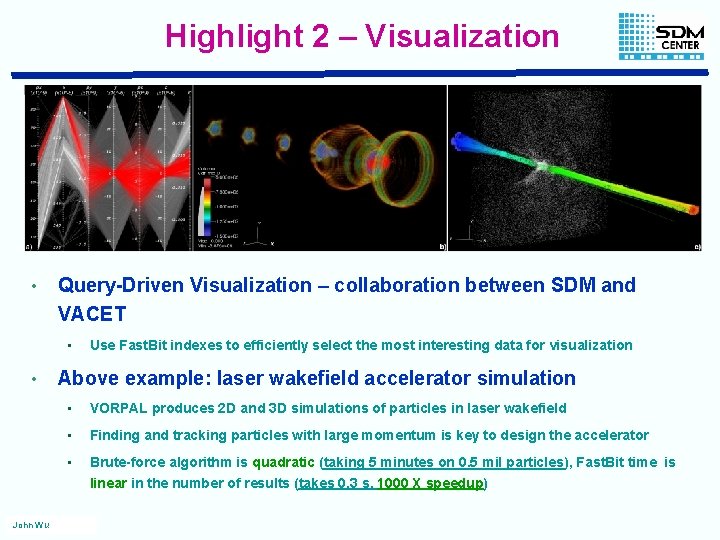
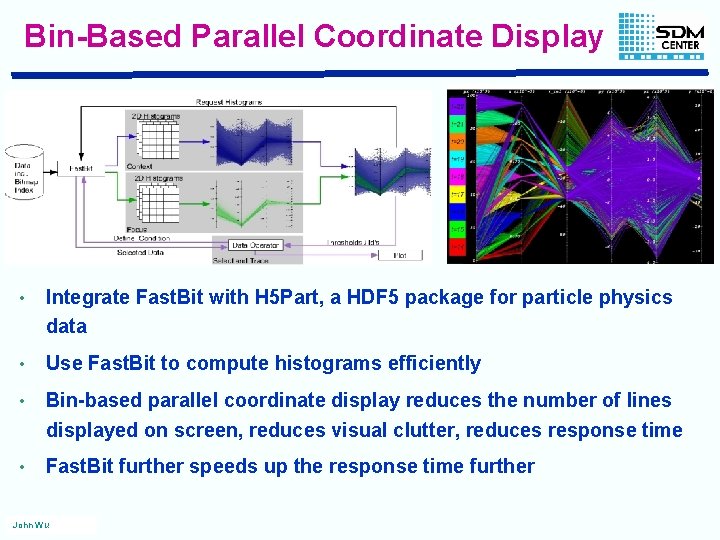
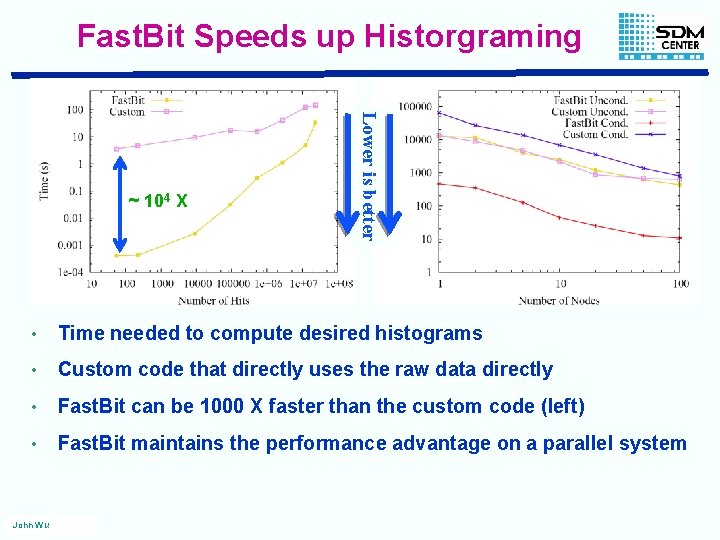
![Highlight 3 – Molecular Docking • • Jochen Schlosser [schlosser@zbh. uni-hamburg. de] Center for Highlight 3 – Molecular Docking • • Jochen Schlosser [schlosser@zbh. uni-hamburg. de] Center for](https://slidetodoc.com/presentation_image_h2/fbbedb90286b6e4a9ded91633401666e/image-10.jpg)
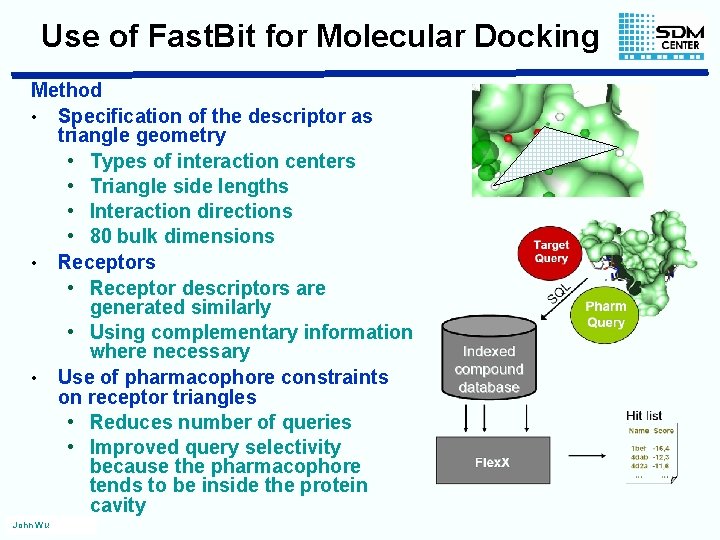
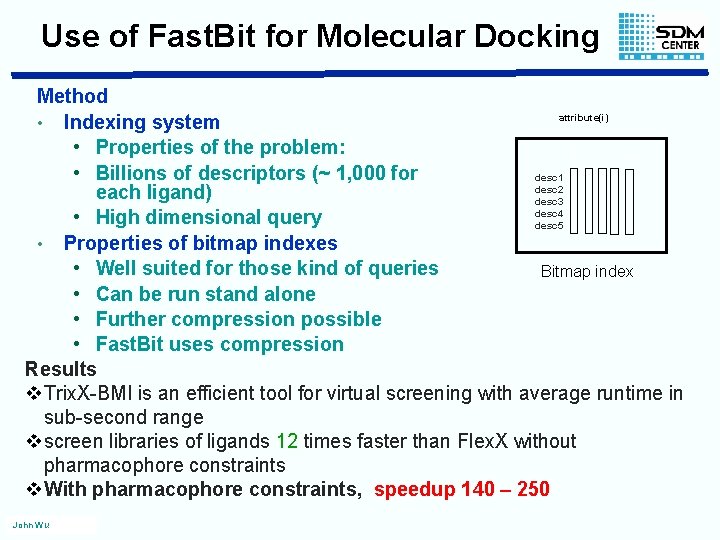
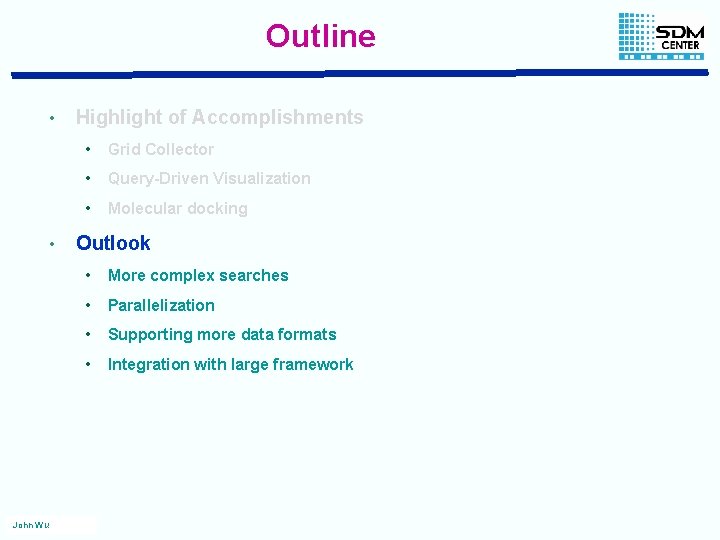
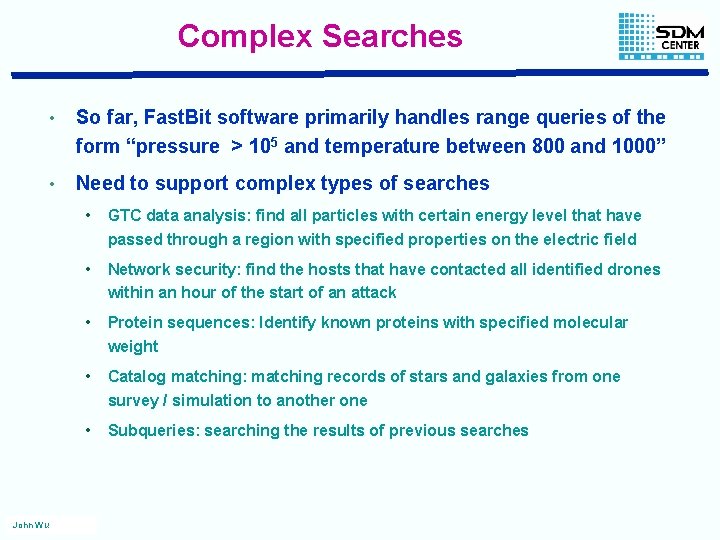
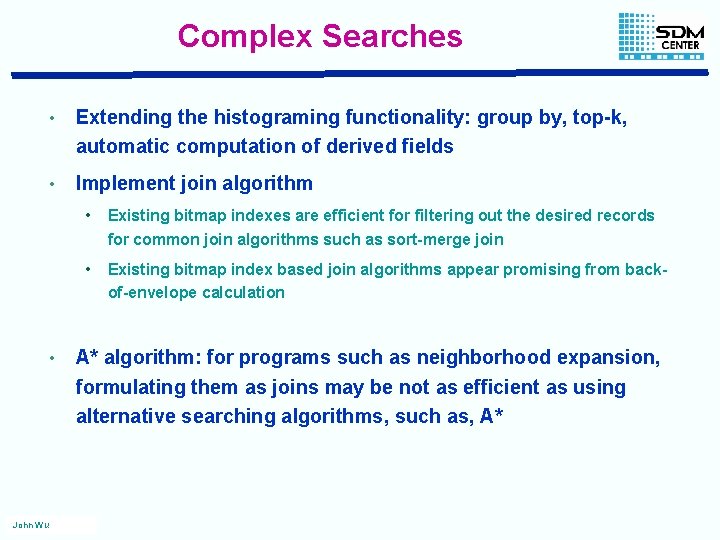
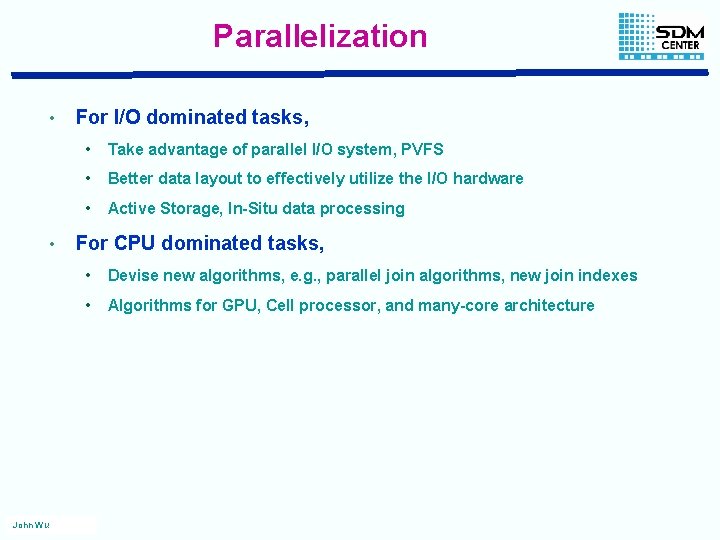
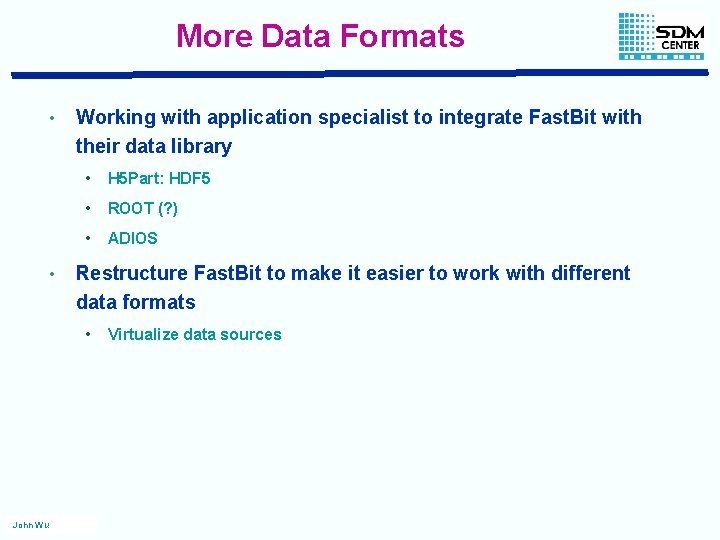
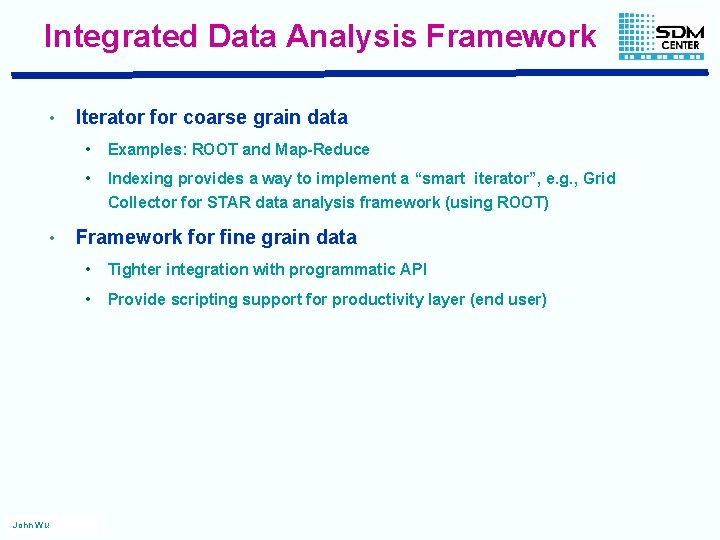
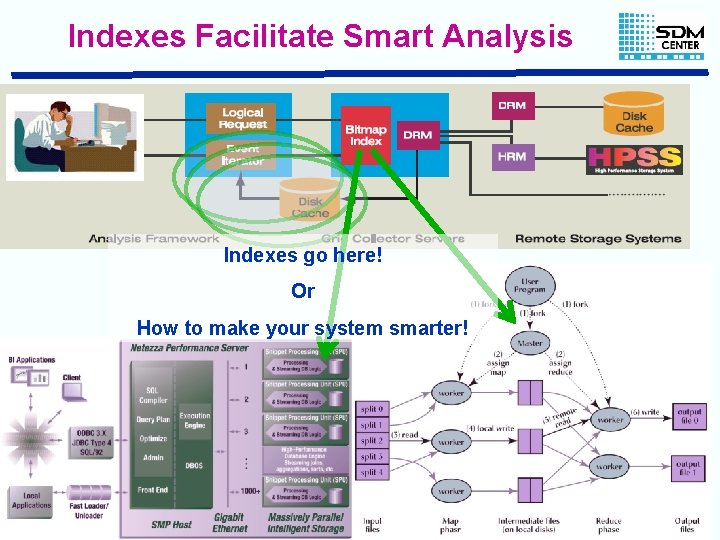
- Slides: 19
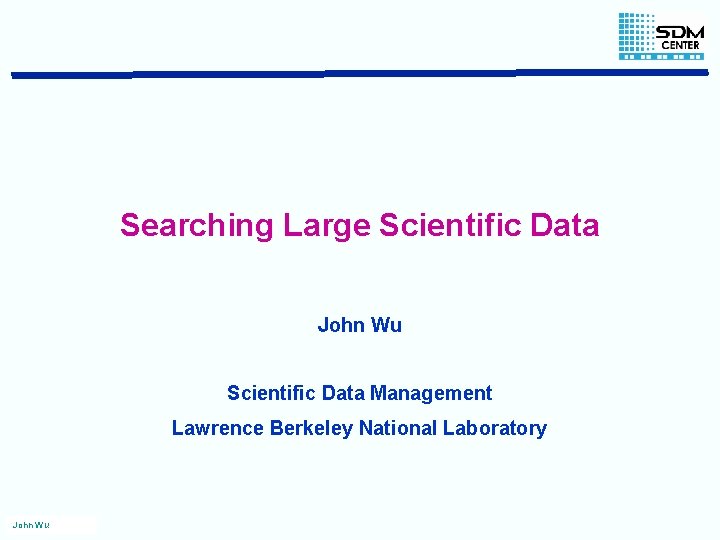
Searching Large Scientific Data John Wu Scientific Data Management Lawrence Berkeley National Laboratory John Wu
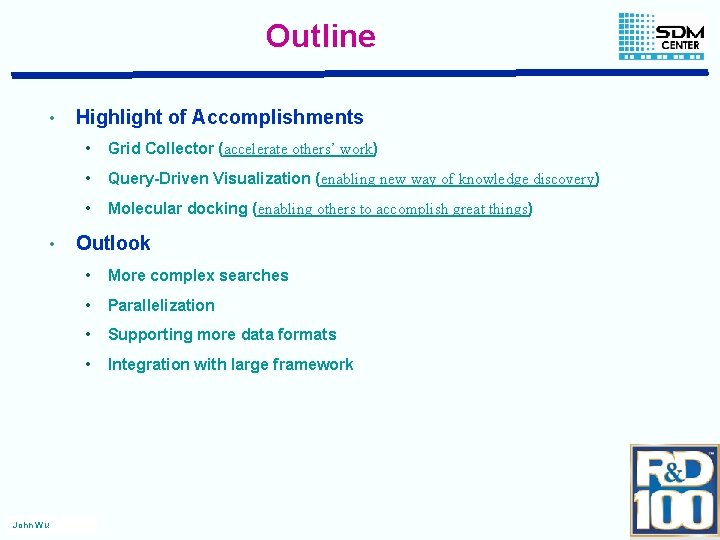
Outline • • John Wu Highlight of Accomplishments • Grid Collector (accelerate others’ work) • Query-Driven Visualization (enabling new way of knowledge discovery) • Molecular docking (enabling others to accomplish great things) Outlook • More complex searches • Parallelization • Supporting more data formats • Integration with large framework
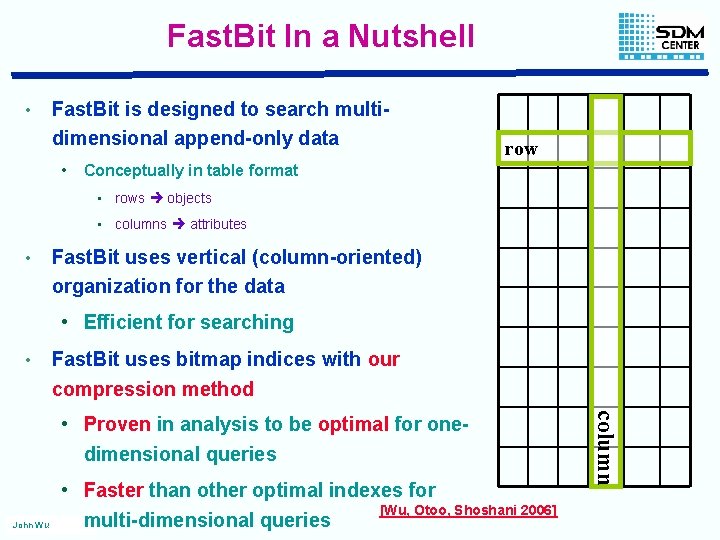
Fast. Bit In a Nutshell • Fast. Bit is designed to search multidimensional append-only data • row Conceptually in table format • rows objects • columns attributes • Fast. Bit uses vertical (column-oriented) organization for the data • Efficient for searching • Fast. Bit uses bitmap indices with our compression method John Wu • Faster than other optimal indexes for [Wu, Otoo, Shoshani 2006] multi-dimensional queries column • Proven in analysis to be optimal for onedimensional queries
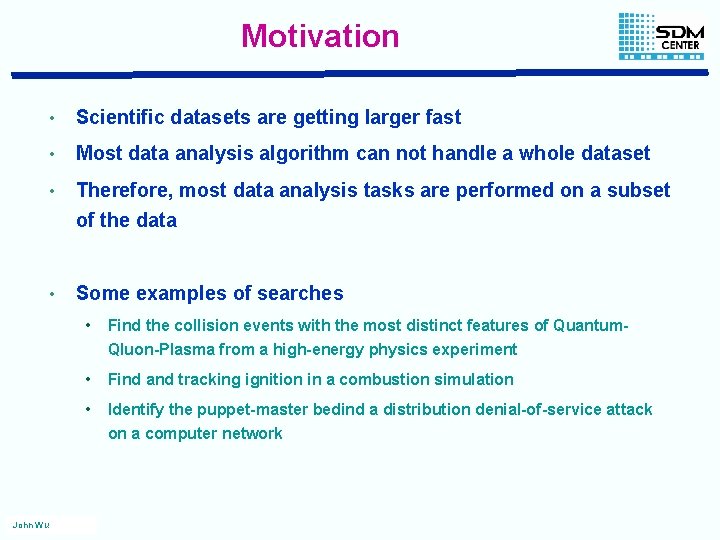
Motivation John Wu • Scientific datasets are getting larger fast • Most data analysis algorithm can not handle a whole dataset • Therefore, most data analysis tasks are performed on a subset of the data • Some examples of searches • Find the collision events with the most distinct features of Quantum. Qluon-Plasma from a high-energy physics experiment • Find and tracking ignition in a combustion simulation • Identify the puppet-master bedind a distribution denial-of-service attack on a computer network
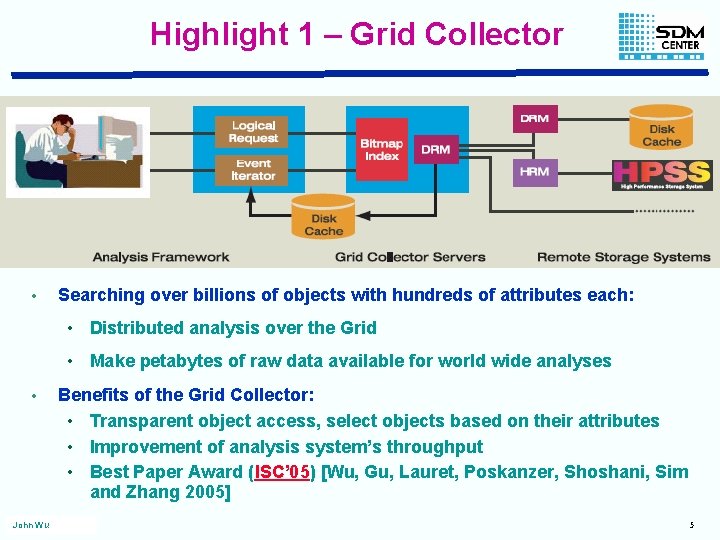
Highlight 1 – Grid Collector • Searching over billions of objects with hundreds of attributes each: • Distributed analysis over the Grid • Make petabytes of raw data available for world wide analyses • John Wu Benefits of the Grid Collector: • Transparent object access, select objects based on their attributes • Improvement of analysis system’s throughput • Best Paper Award (ISC’ 05) [Wu, Gu, Lauret, Poskanzer, Shoshani, Sim and Zhang 2005] 5
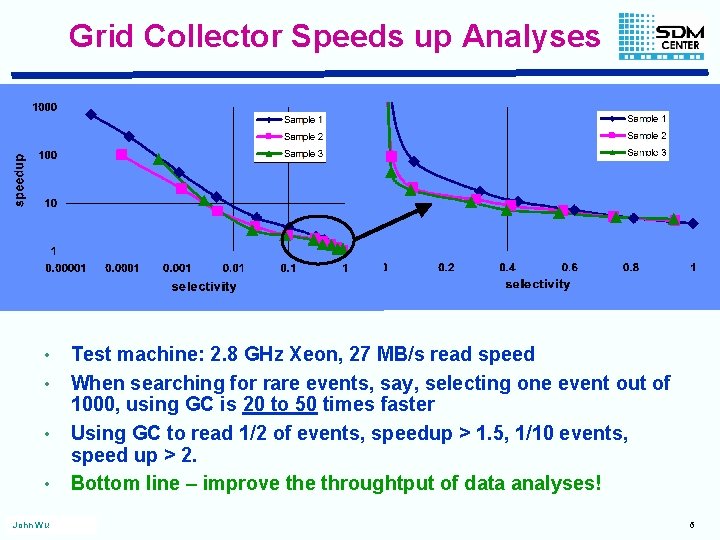
Grid Collector Speeds up Analyses • • John Wu Test machine: 2. 8 GHz Xeon, 27 MB/s read speed When searching for rare events, say, selecting one event out of 1000, using GC is 20 to 50 times faster Using GC to read 1/2 of events, speedup > 1. 5, 1/10 events, speed up > 2. Bottom line – improve throughtput of data analyses! 6
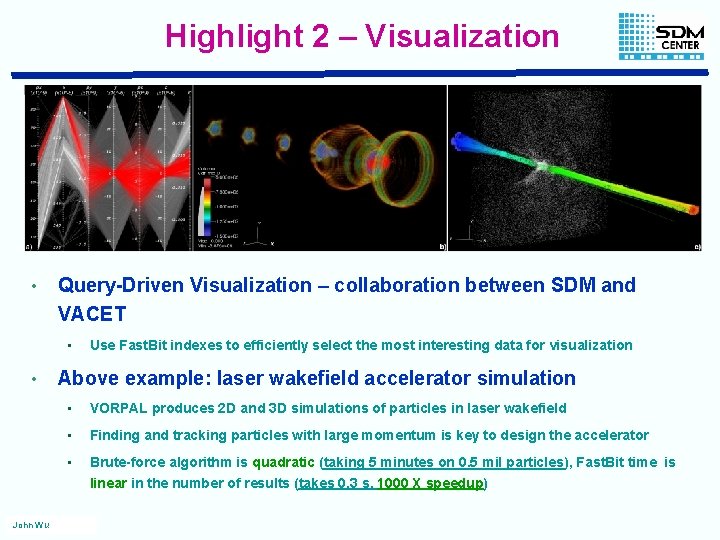
Highlight 2 – Visualization • Query-Driven Visualization – collaboration between SDM and VACET • • John Wu Use Fast. Bit indexes to efficiently select the most interesting data for visualization Above example: laser wakefield accelerator simulation • VORPAL produces 2 D and 3 D simulations of particles in laser wakefield • Finding and tracking particles with large momentum is key to design the accelerator • Brute-force algorithm is quadratic (taking 5 minutes on 0. 5 mil particles), Fast. Bit time is linear in the number of results (takes 0. 3 s, 1000 X speedup)
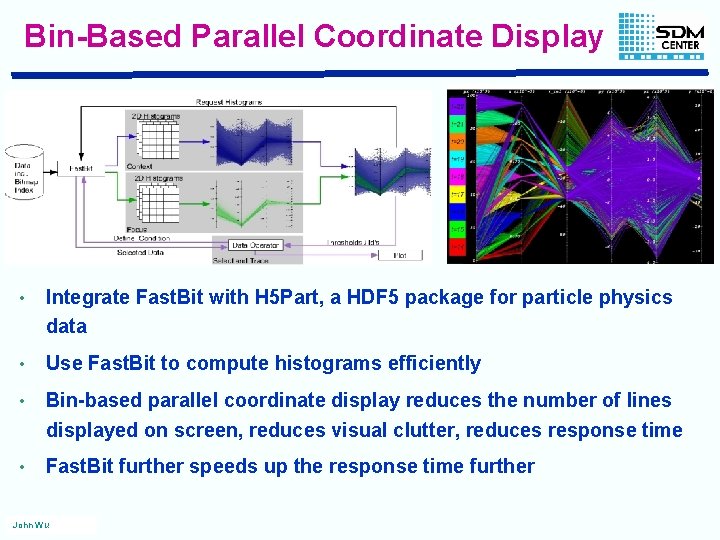
Bin-Based Parallel Coordinate Display • Integrate Fast. Bit with H 5 Part, a HDF 5 package for particle physics data • Use Fast. Bit to compute histograms efficiently • Bin-based parallel coordinate display reduces the number of lines displayed on screen, reduces visual clutter, reduces response time • Fast. Bit further speeds up the response time further John Wu
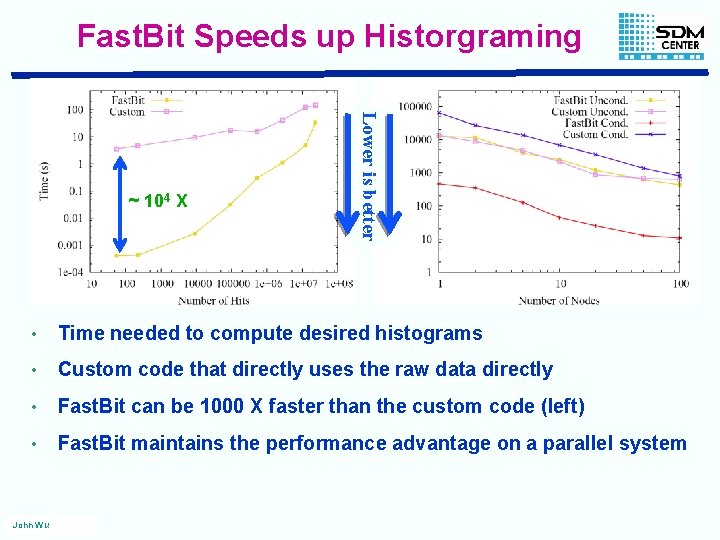
Fast. Bit Speeds up Historgraming Lower is better ~ 104 X • Time needed to compute desired histograms • Custom code that directly uses the raw data directly • Fast. Bit can be 1000 X faster than the custom code (left) • Fast. Bit maintains the performance advantage on a parallel system John Wu
![Highlight 3 Molecular Docking Jochen Schlosser schlosserzbh unihamburg de Center for Highlight 3 – Molecular Docking • • Jochen Schlosser [schlosser@zbh. uni-hamburg. de] Center for](https://slidetodoc.com/presentation_image_h2/fbbedb90286b6e4a9ded91633401666e/image-10.jpg)
Highlight 3 – Molecular Docking • • Jochen Schlosser [schlosser@zbh. uni-hamburg. de] Center for Bioinformatics, University of Hamburg Application: Structure-based virtual screening (ACS Fall 2007) n ligands One target protein n docking runs Hit list Name Score Match ligand with cavity 1 bef -16, 4 4 dab -12, 3 4 d 2 a -11, 6 …… Standard approach: match every ligand with every target protein New approach: using Fast. Bit indexes to avoid brute-force matching John Wu
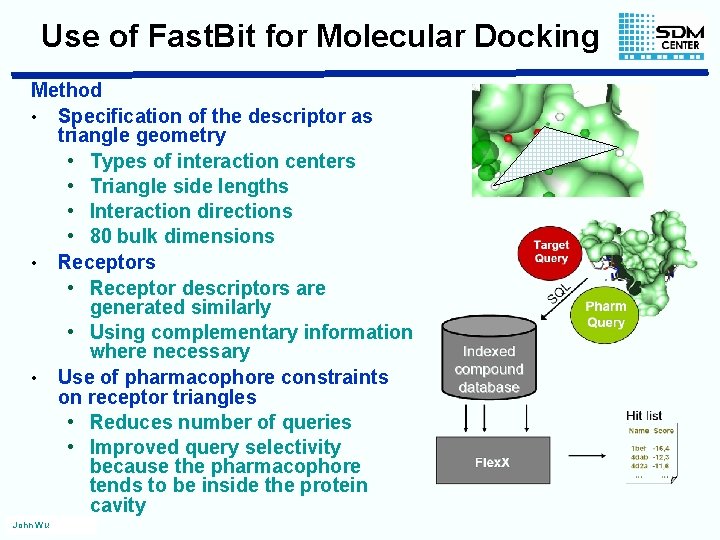
Use of Fast. Bit for Molecular Docking Method • Specification of the descriptor as triangle geometry • Types of interaction centers • Triangle side lengths • Interaction directions • 80 bulk dimensions • Receptor descriptors are generated similarly • Using complementary information where necessary • Use of pharmacophore constraints on receptor triangles • Reduces number of queries • Improved query selectivity because the pharmacophore tends to be inside the protein cavity John Wu
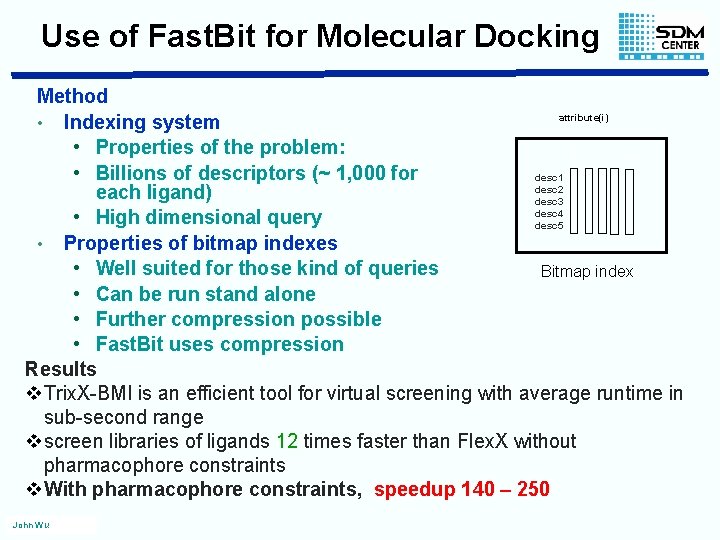
Use of Fast. Bit for Molecular Docking Method attribute(i) • Indexing system • Properties of the problem: [0]. . . … … [n] • Billions of descriptors (~ 1, 000 for desc 1 0 0 0 desc 2 0 0 0 1 0 each ligand) desc 3 0 1 0 0 0 desc 4 0 0 1 • High dimensional query desc 5 1 0 0 • Properties of bitmap indexes • Well suited for those kind of queries Bitmap index • Can be run stand alone • Further compression possible • Fast. Bit uses compression Results v. Trix. X-BMI is an efficient tool for virtual screening with average runtime in sub-second range vscreen libraries of ligands 12 times faster than Flex. X without pharmacophore constraints v. With pharmacophore constraints, speedup 140 – 250 John Wu
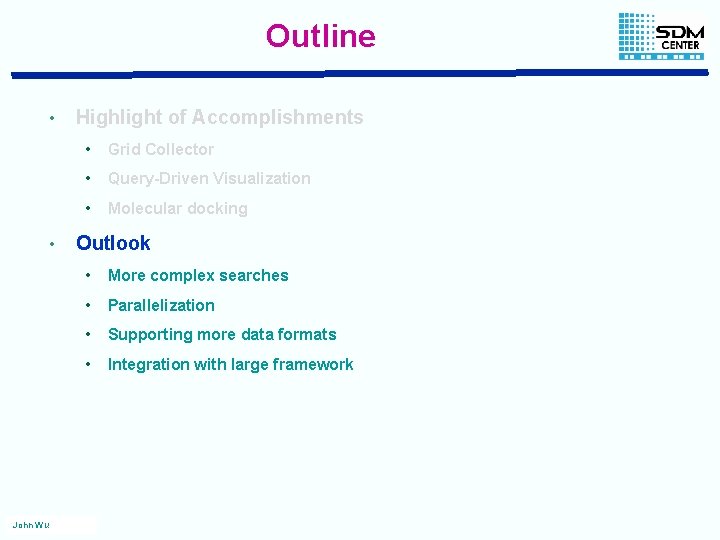
Outline • • John Wu Highlight of Accomplishments • Grid Collector • Query-Driven Visualization • Molecular docking Outlook • More complex searches • Parallelization • Supporting more data formats • Integration with large framework
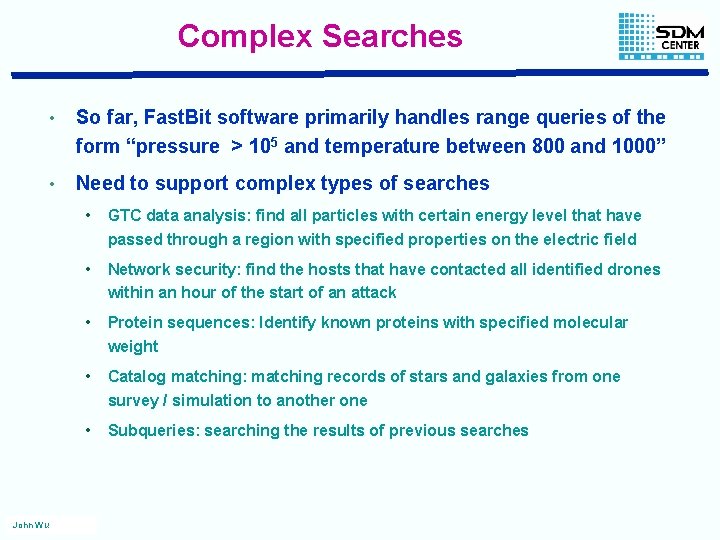
Complex Searches John Wu • So far, Fast. Bit software primarily handles range queries of the form “pressure > 105 and temperature between 800 and 1000” • Need to support complex types of searches • GTC data analysis: find all particles with certain energy level that have passed through a region with specified properties on the electric field • Network security: find the hosts that have contacted all identified drones within an hour of the start of an attack • Protein sequences: Identify known proteins with specified molecular weight • Catalog matching: matching records of stars and galaxies from one survey / simulation to another one • Subqueries: searching the results of previous searches
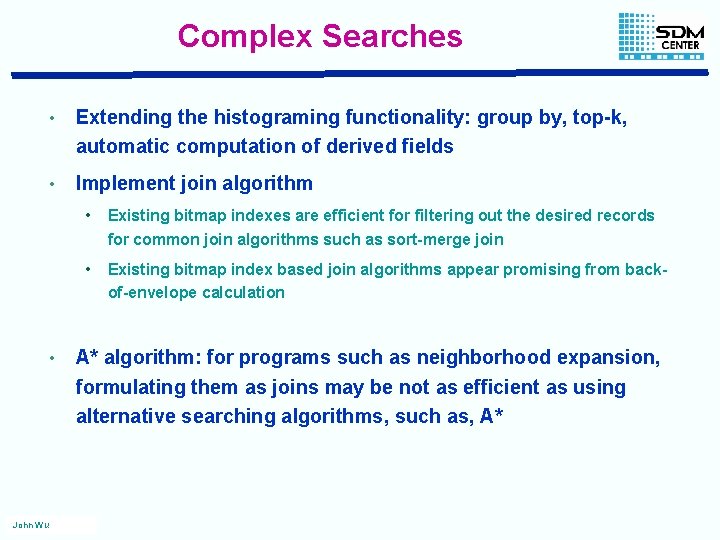
Complex Searches • Extending the histograming functionality: group by, top-k, automatic computation of derived fields • Implement join algorithm • John Wu • Existing bitmap indexes are efficient for filtering out the desired records for common join algorithms such as sort-merge join • Existing bitmap index based join algorithms appear promising from backof-envelope calculation A* algorithm: for programs such as neighborhood expansion, formulating them as joins may be not as efficient as using alternative searching algorithms, such as, A*
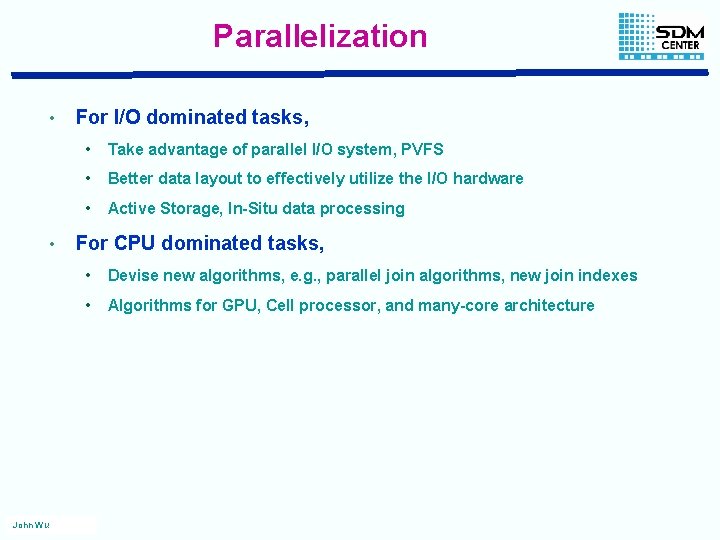
Parallelization • • John Wu For I/O dominated tasks, • Take advantage of parallel I/O system, PVFS • Better data layout to effectively utilize the I/O hardware • Active Storage, In-Situ data processing For CPU dominated tasks, • Devise new algorithms, e. g. , parallel join algorithms, new join indexes • Algorithms for GPU, Cell processor, and many-core architecture
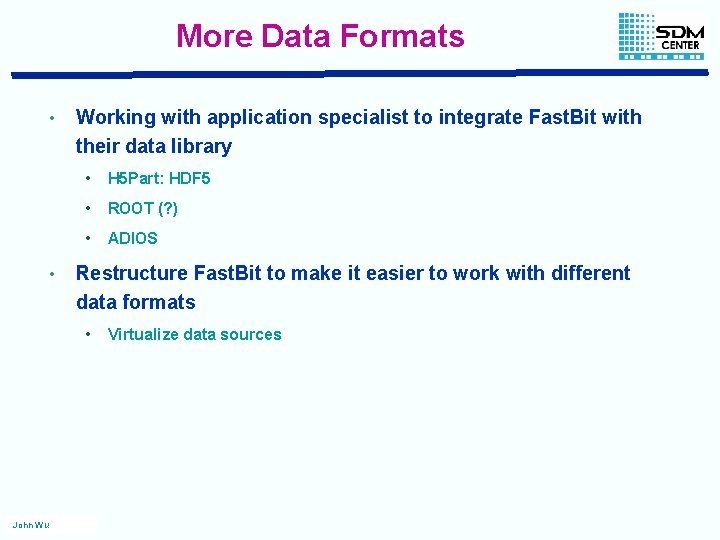
More Data Formats • • Working with application specialist to integrate Fast. Bit with their data library • H 5 Part: HDF 5 • ROOT (? ) • ADIOS Restructure Fast. Bit to make it easier to work with different data formats • John Wu Virtualize data sources
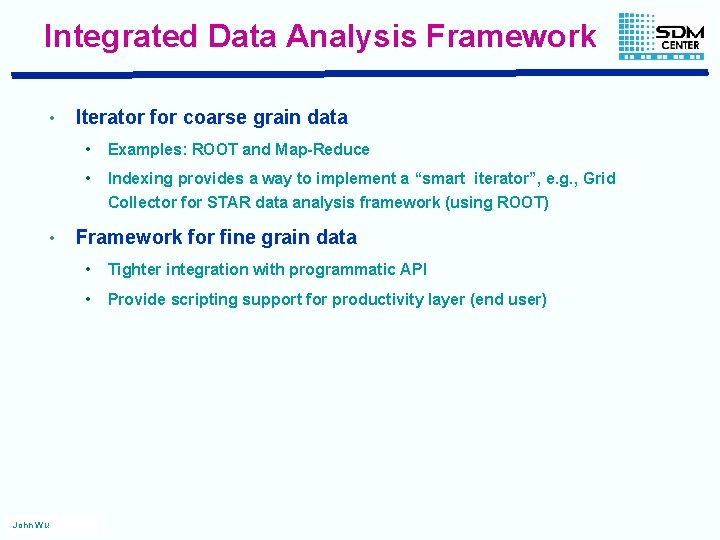
Integrated Data Analysis Framework • • John Wu Iterator for coarse grain data • Examples: ROOT and Map-Reduce • Indexing provides a way to implement a “smart iterator”, e. g. , Grid Collector for STAR data analysis framework (using ROOT) Framework for fine grain data • Tighter integration with programmatic API • Provide scripting support for productivity layer (end user)
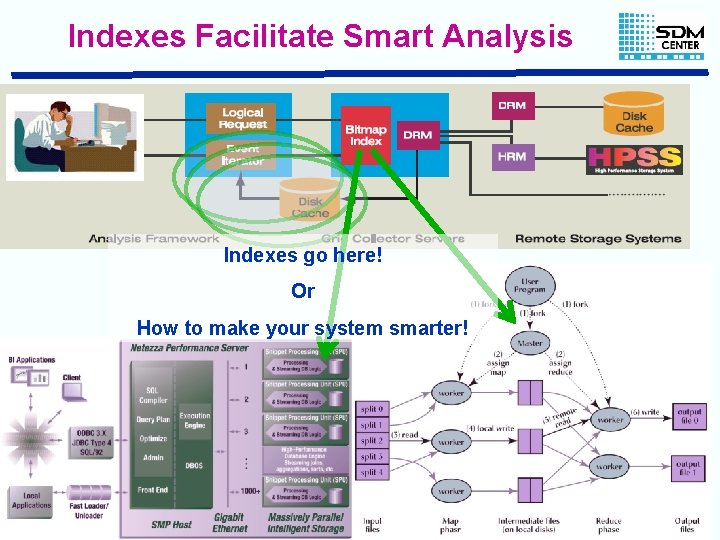
Indexes Facilitate Smart Analysis Indexes go here! Or How to make your system smarter! John Wu