PIT 2017 County of Riverside Continuum of Care
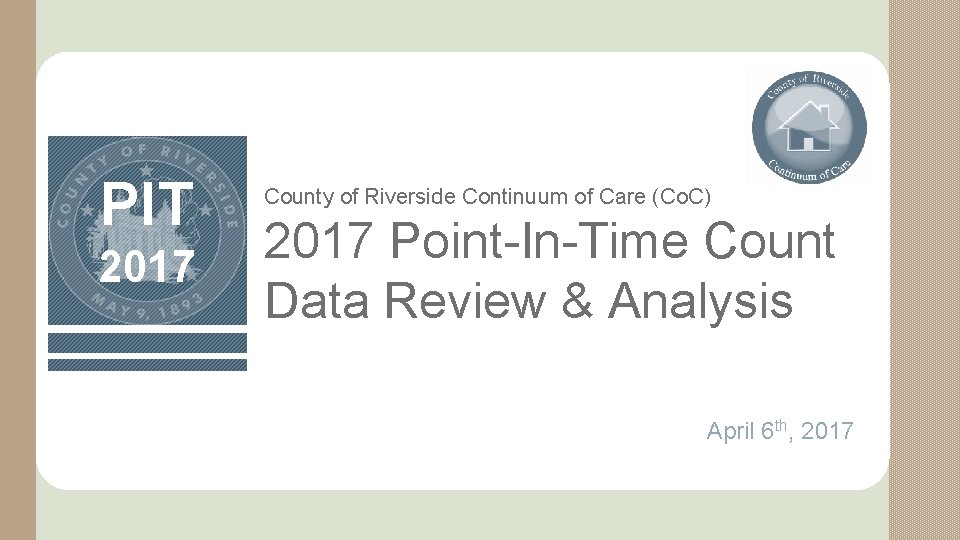
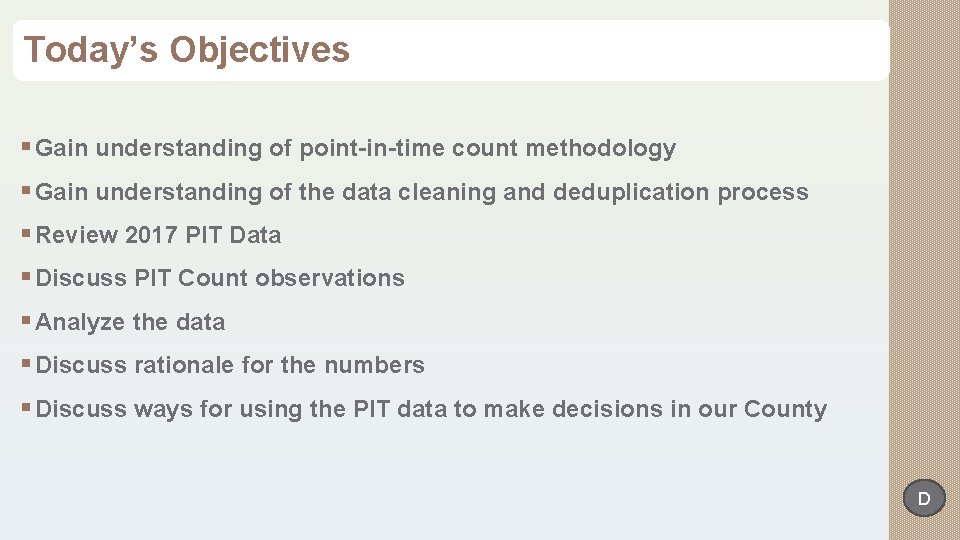
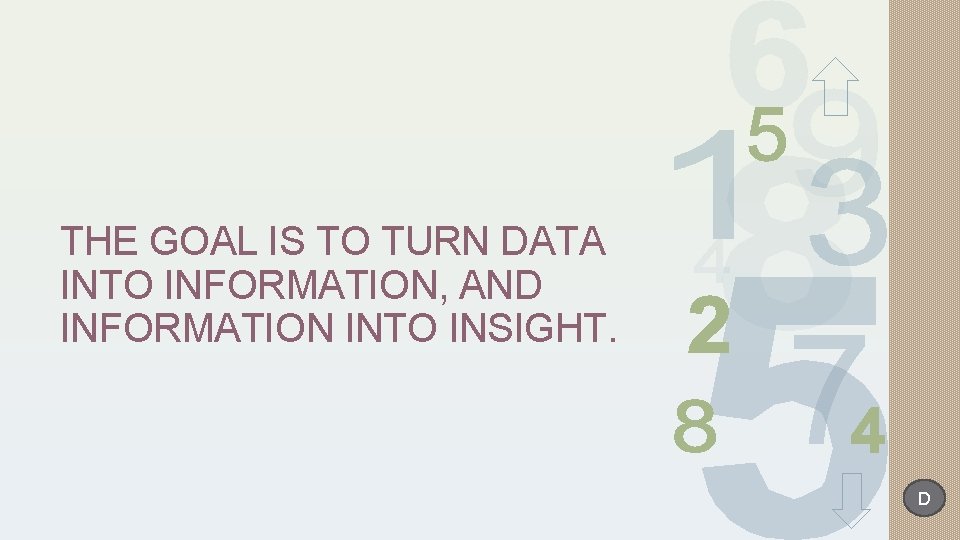
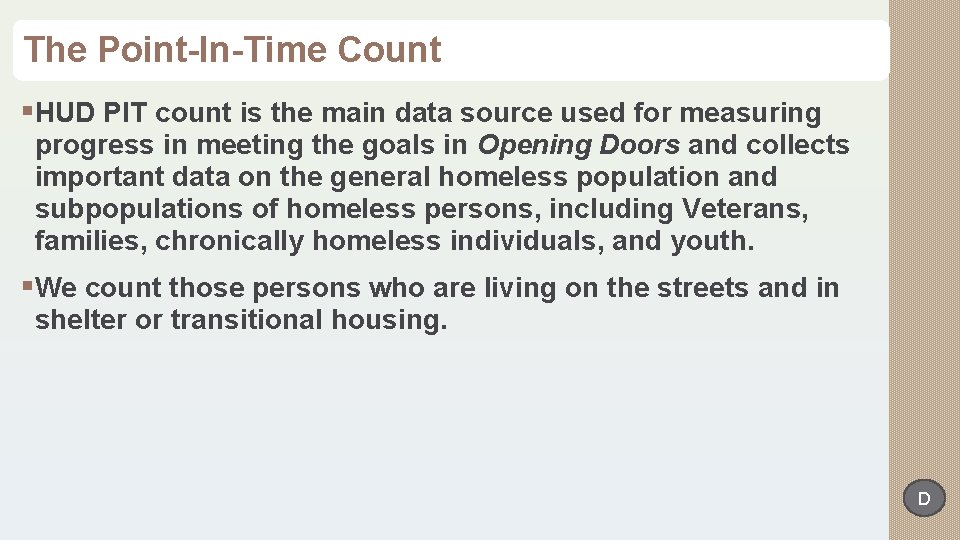
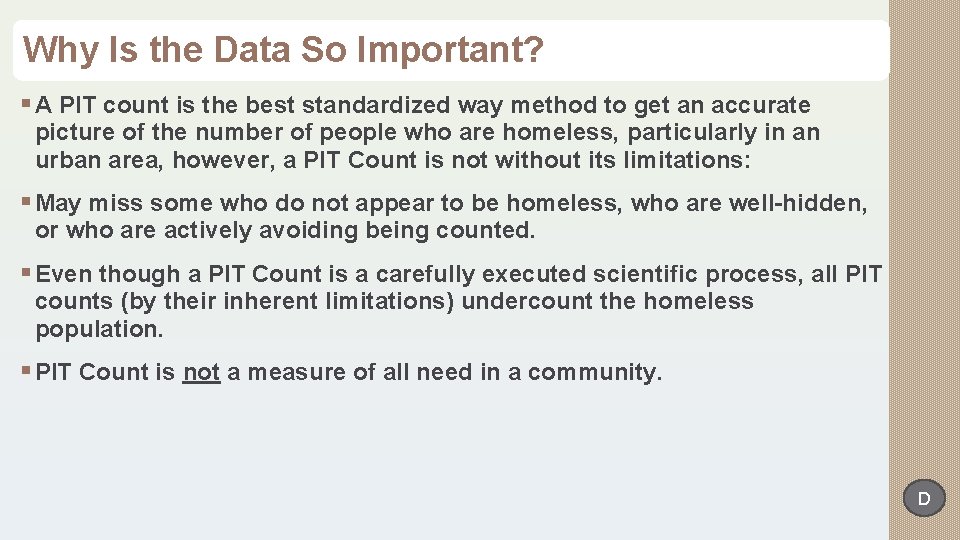
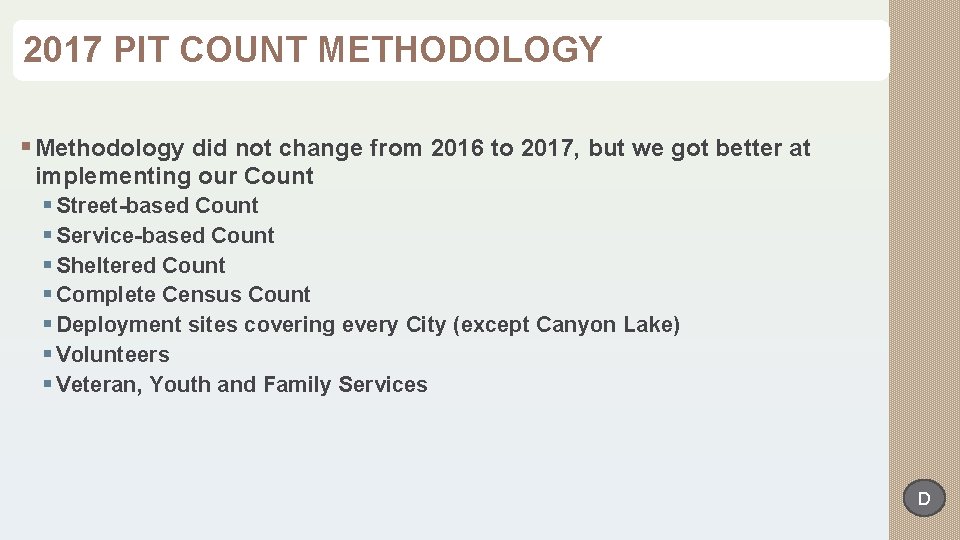
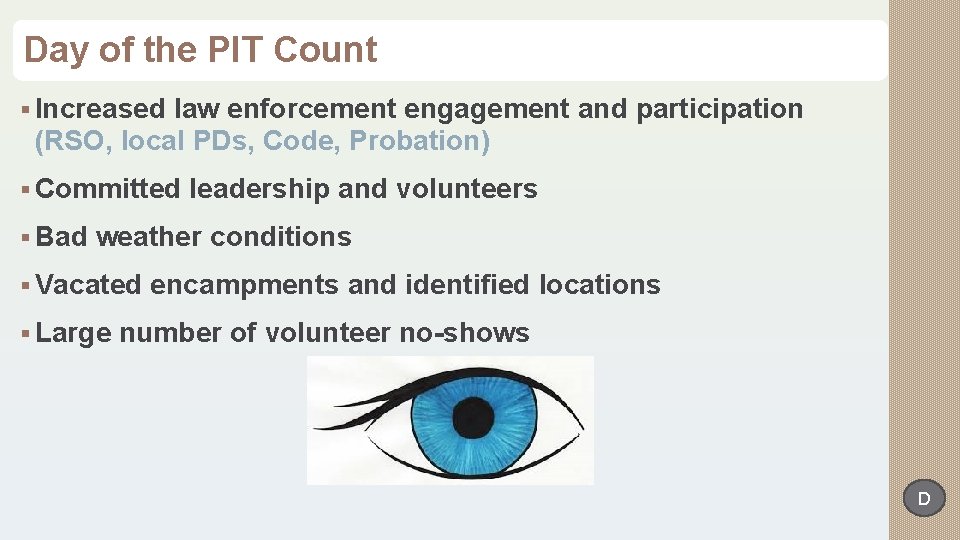
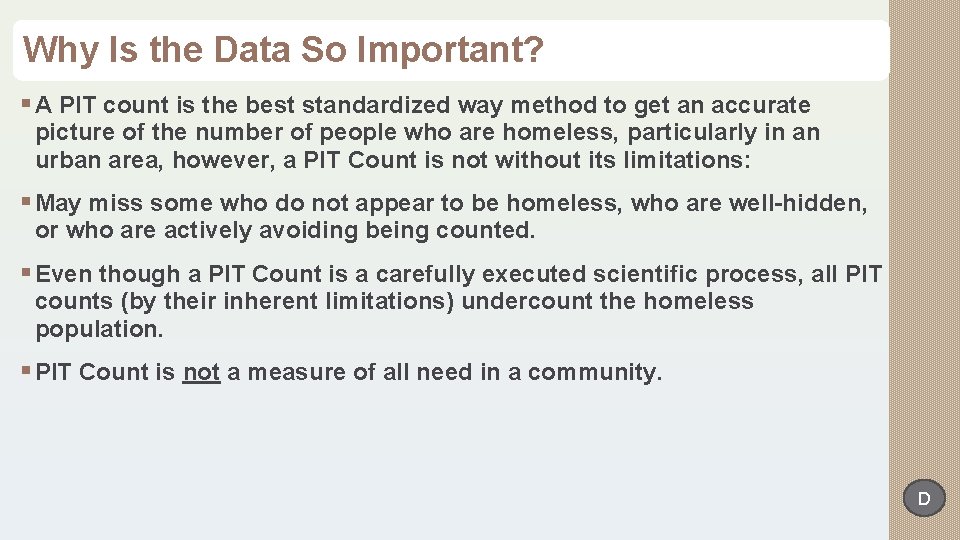
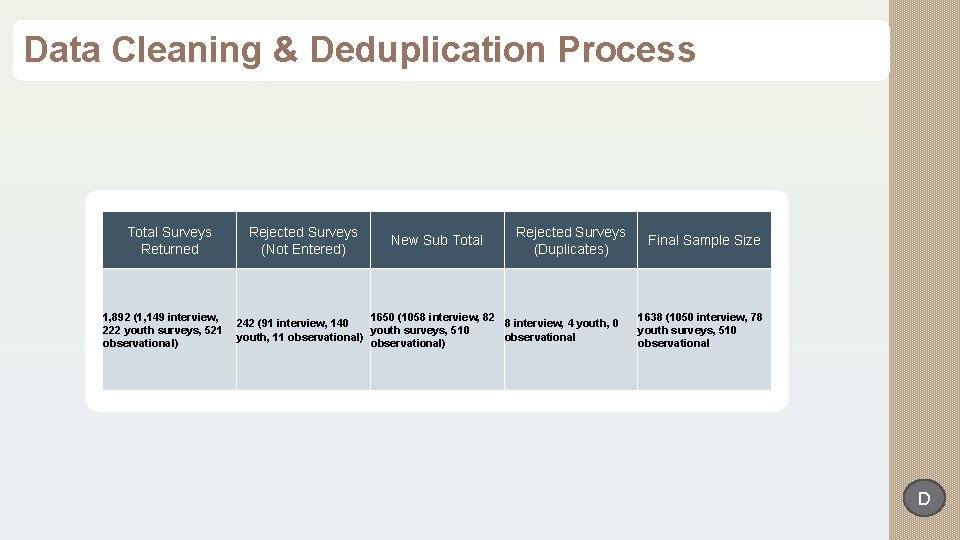
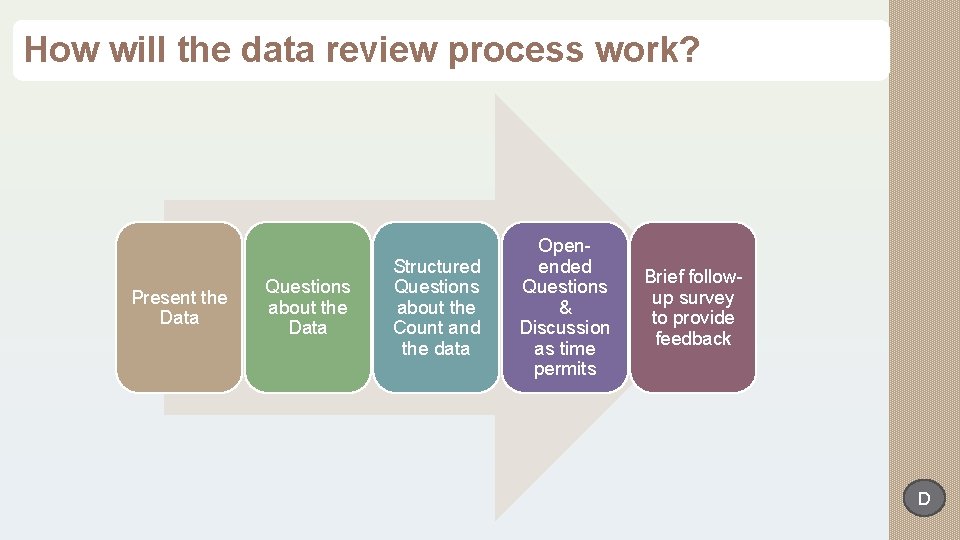
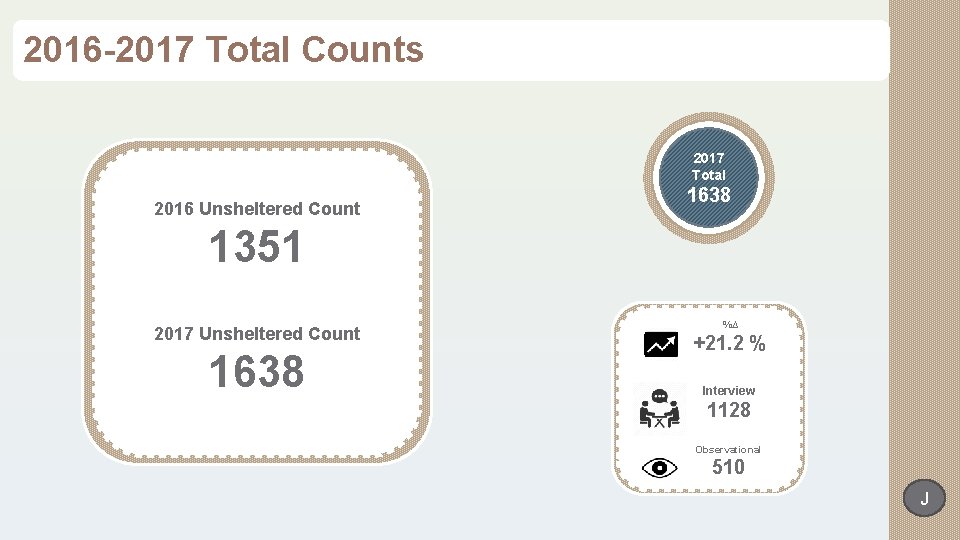
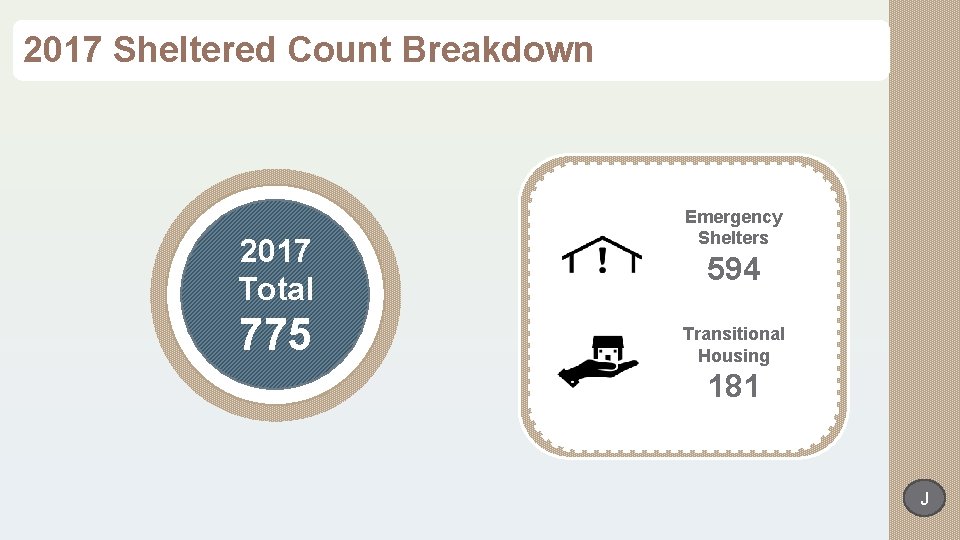
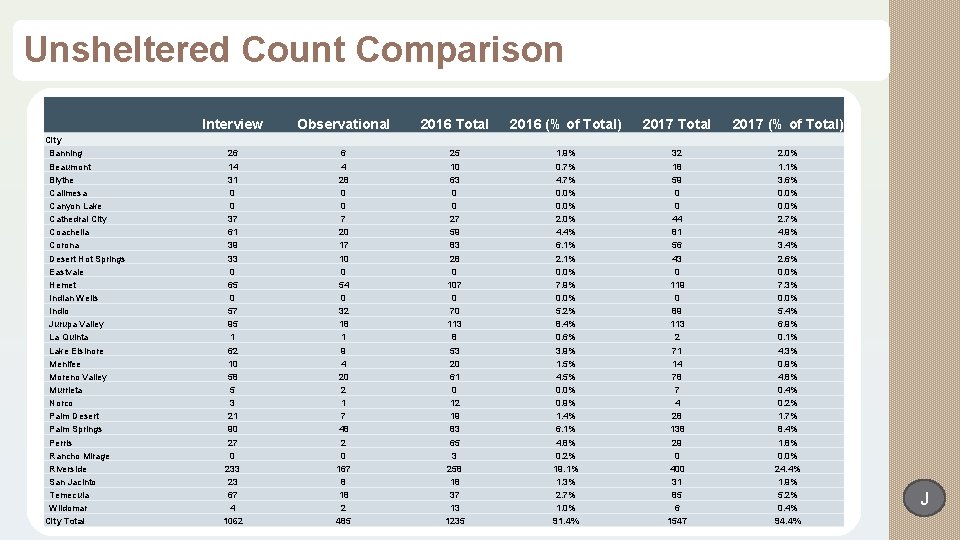
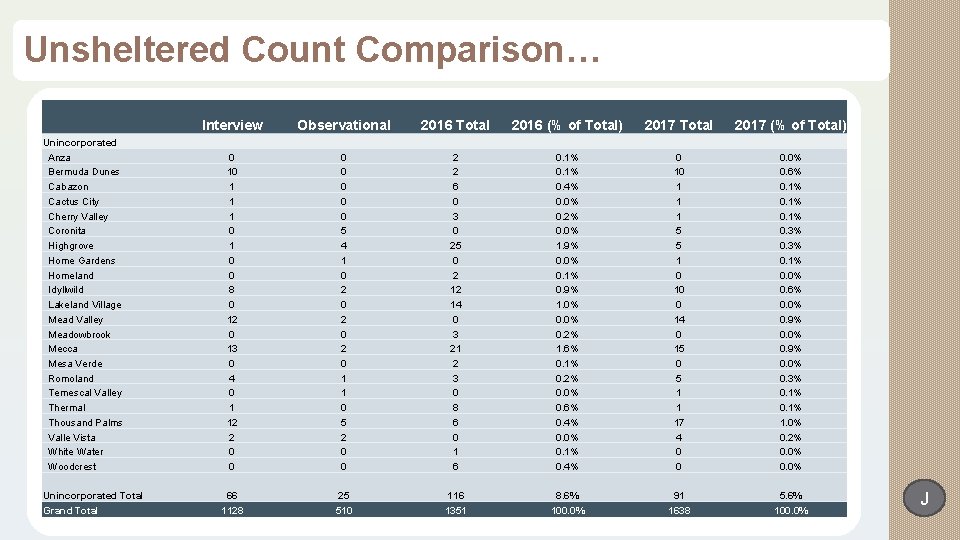
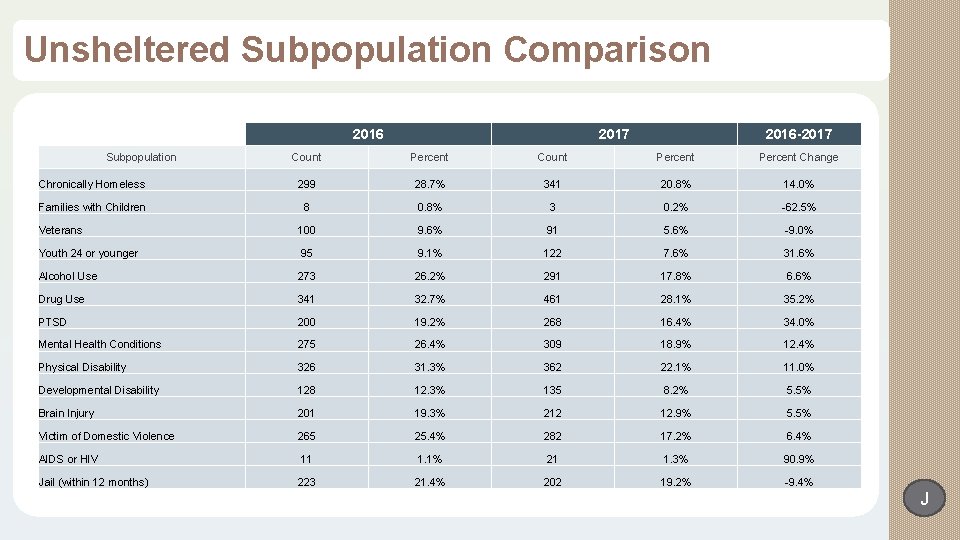
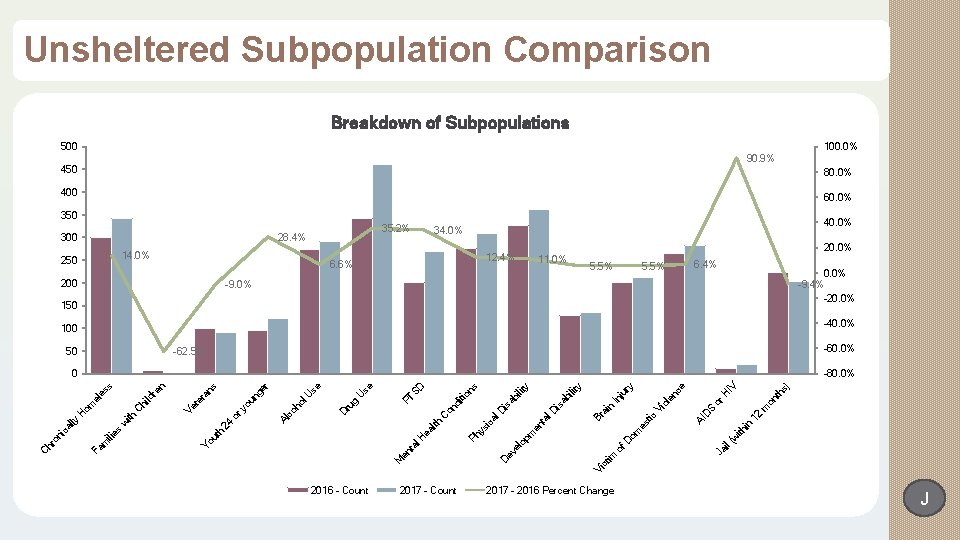
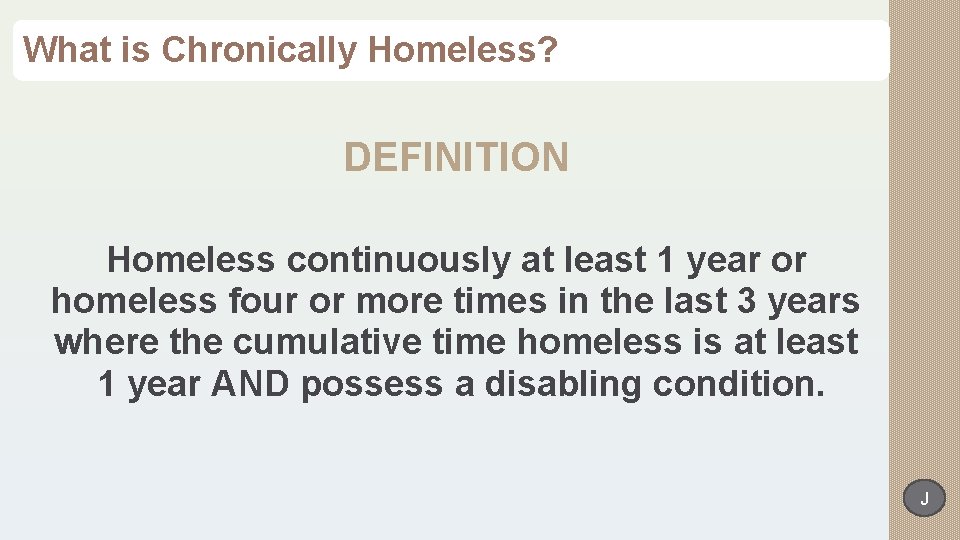
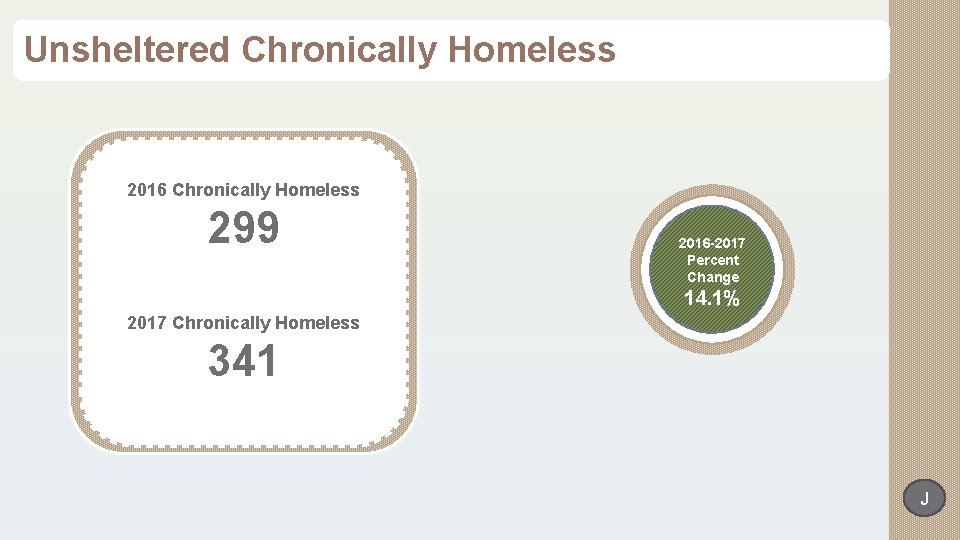
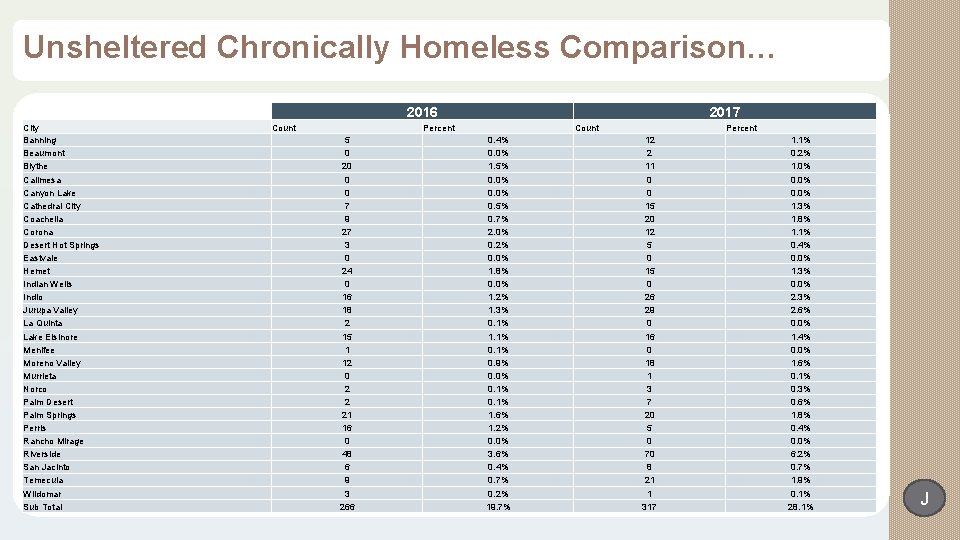
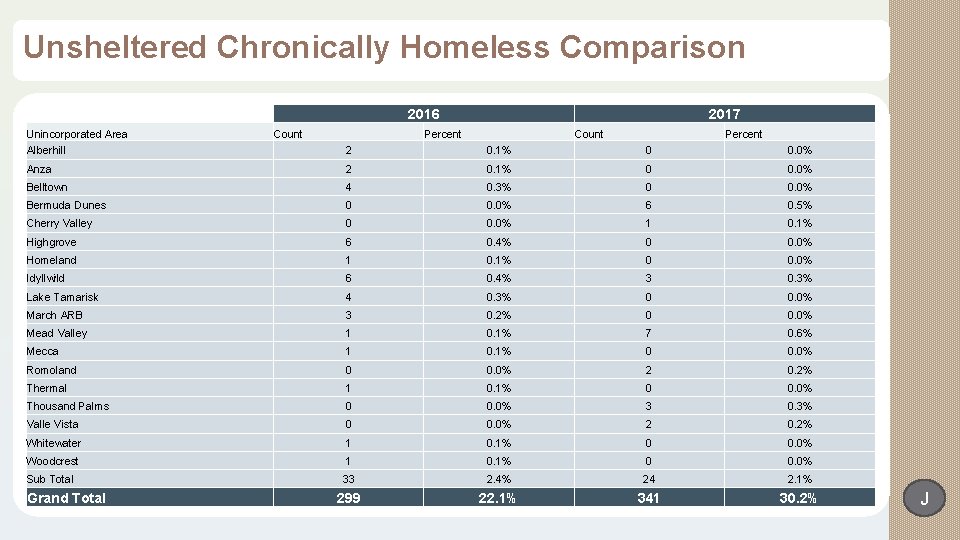
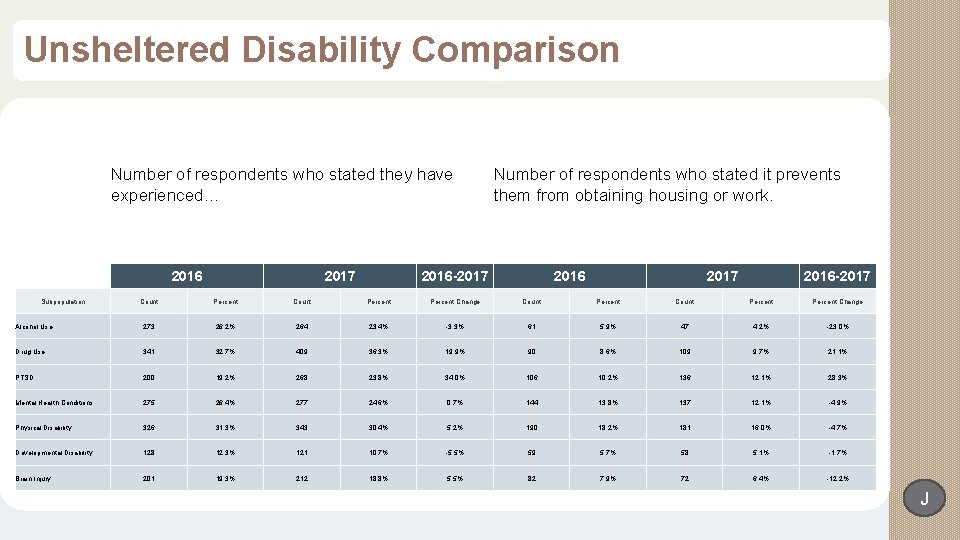
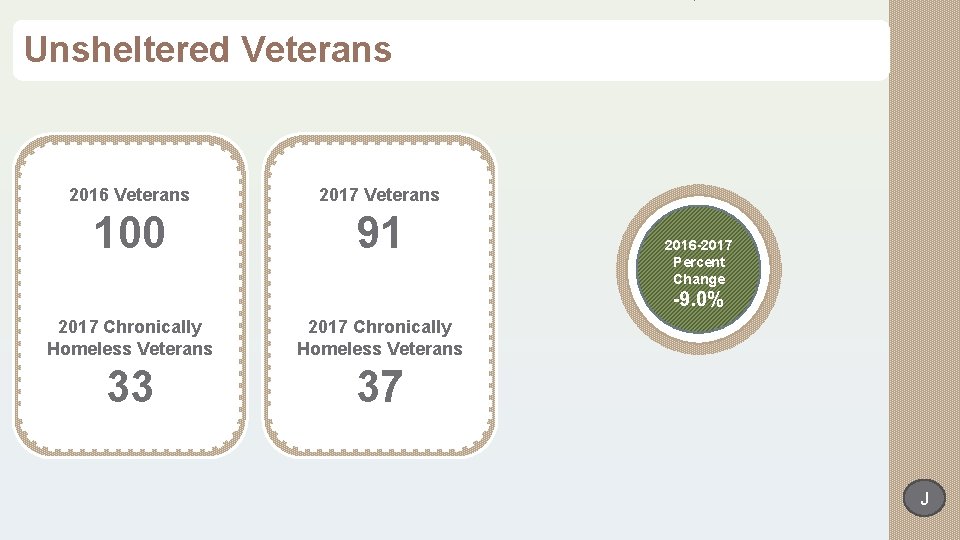
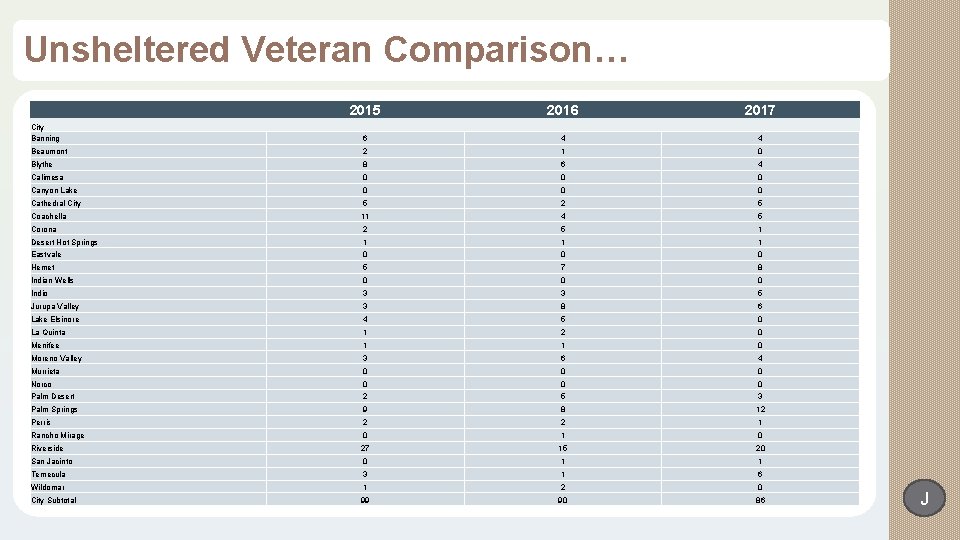
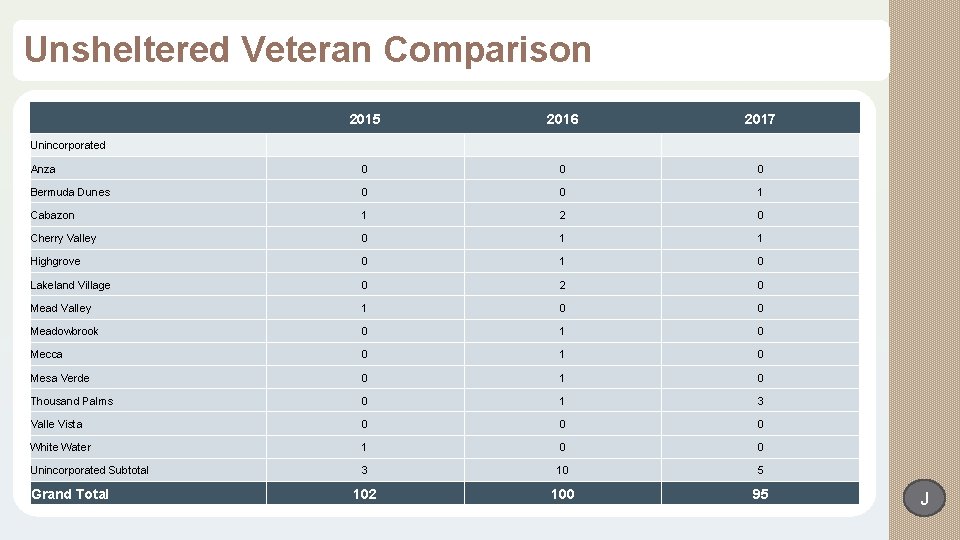
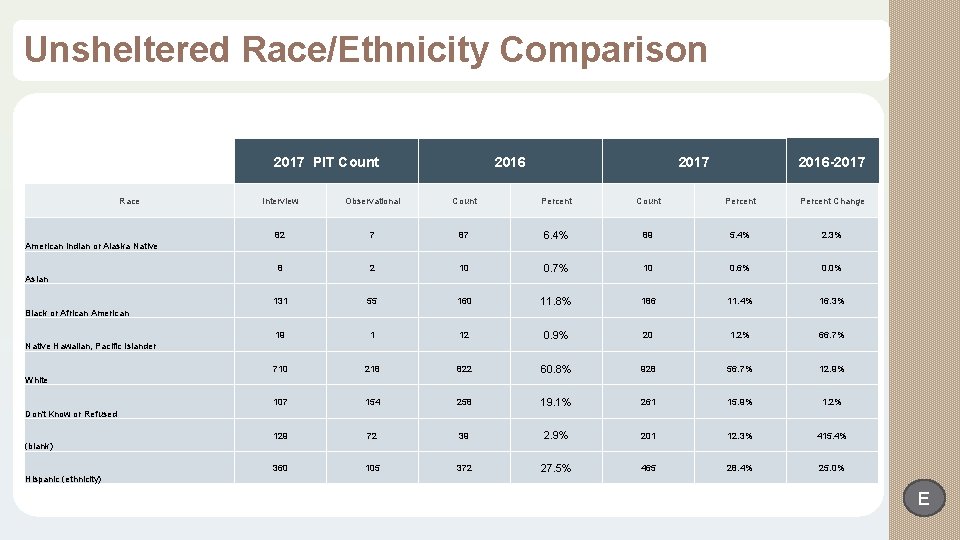
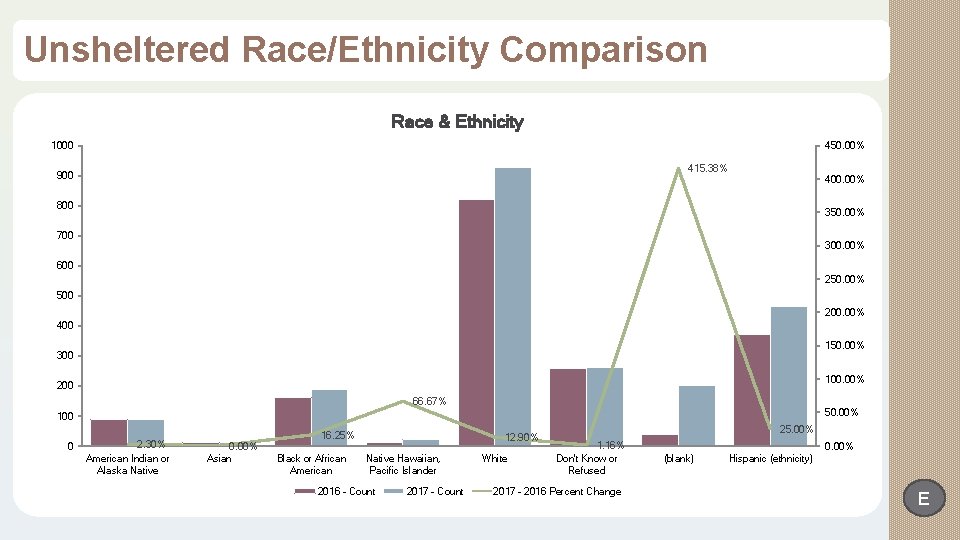
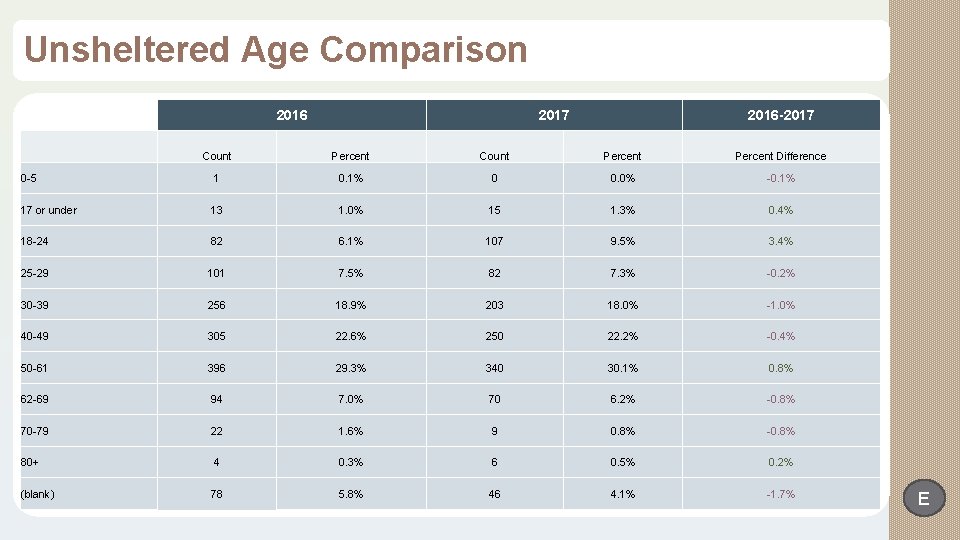
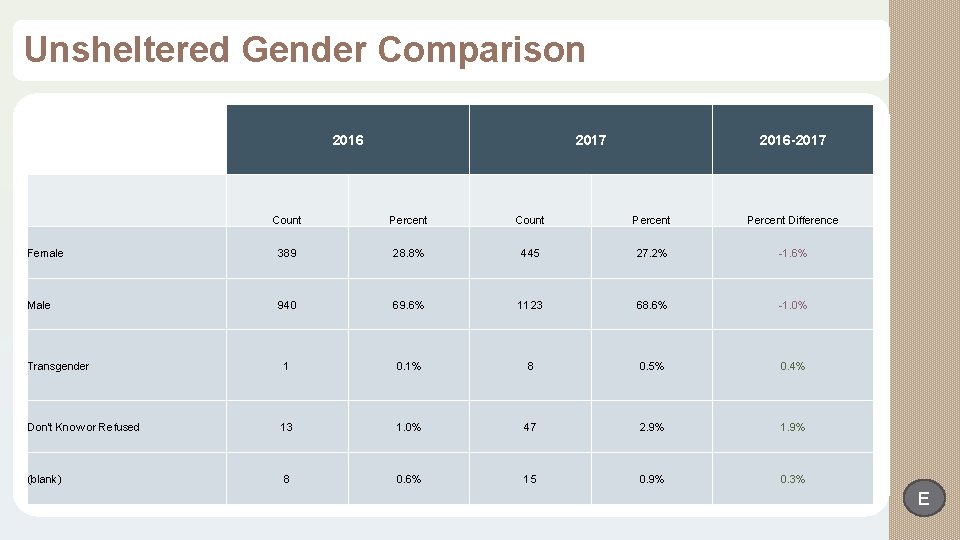
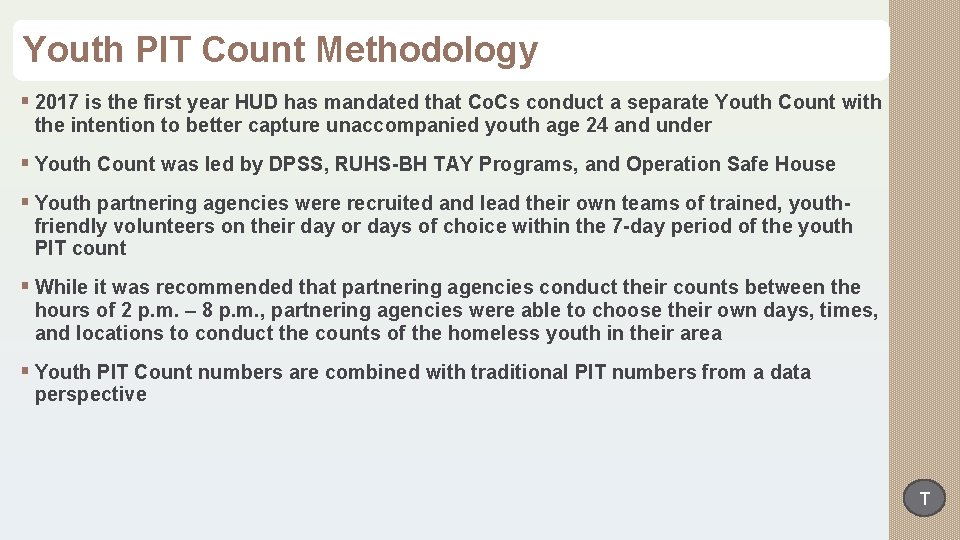
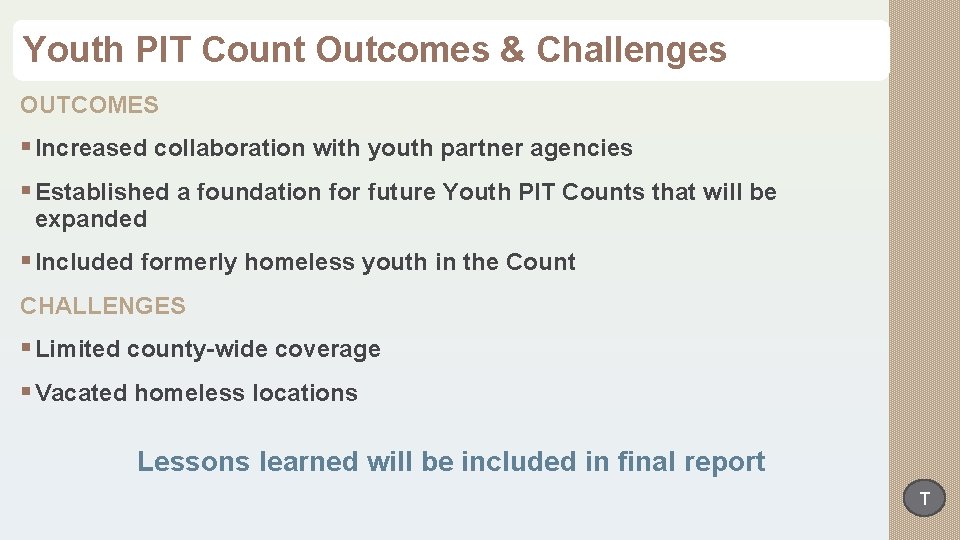
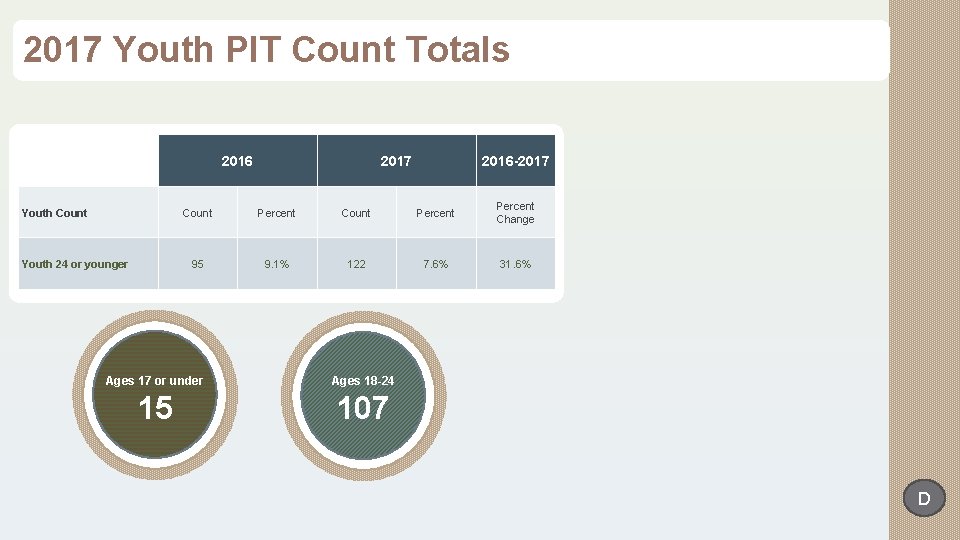
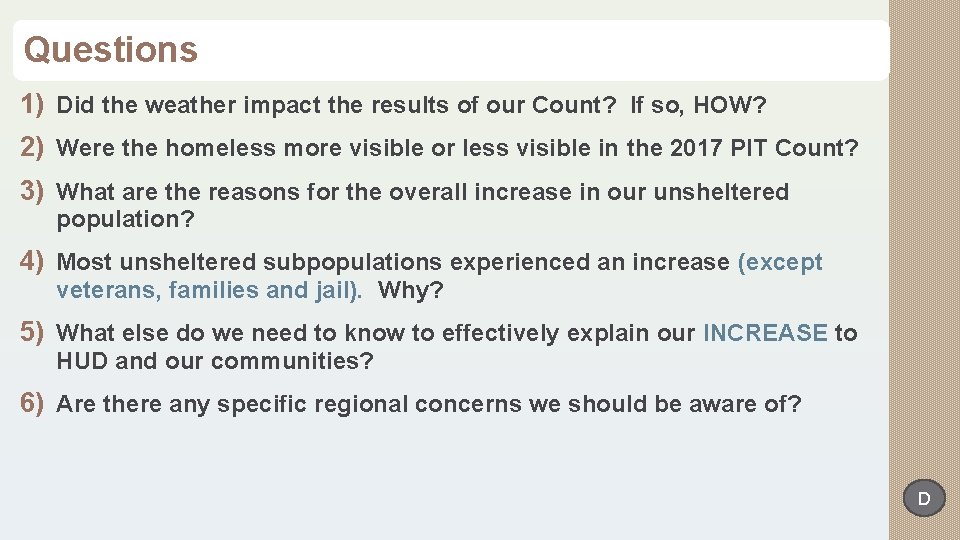
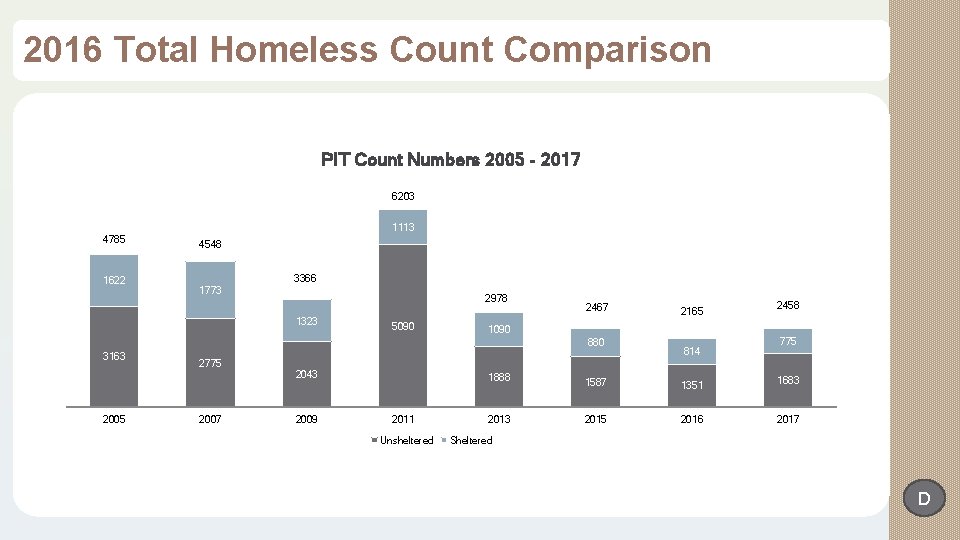
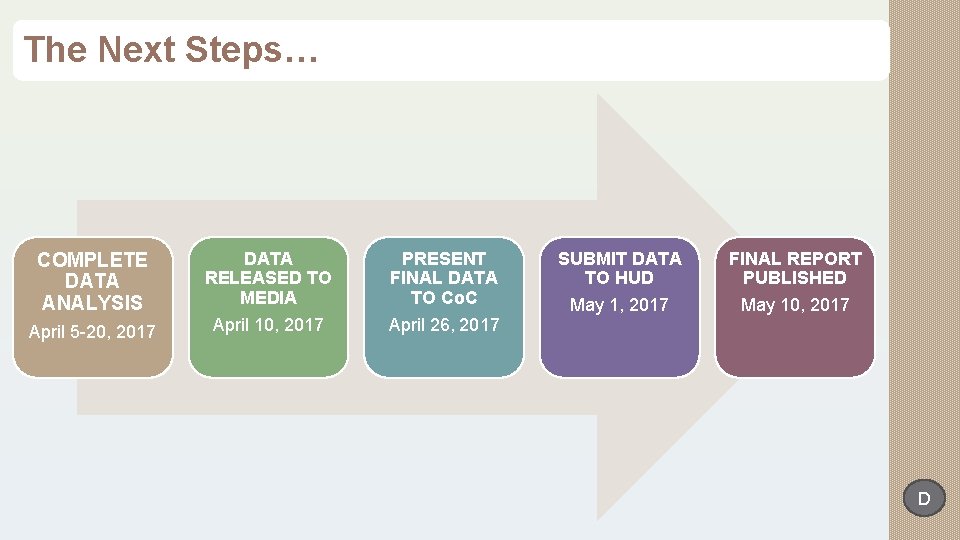
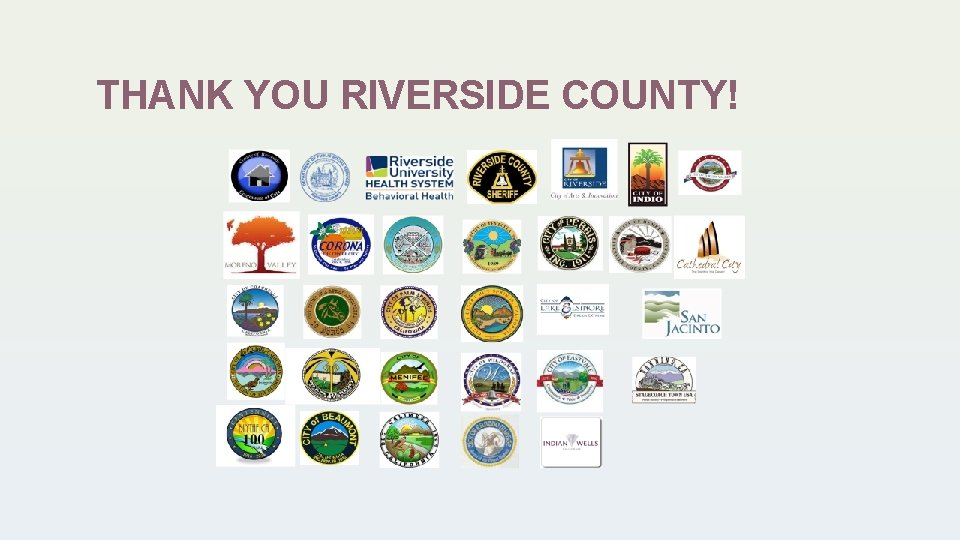
- Slides: 35
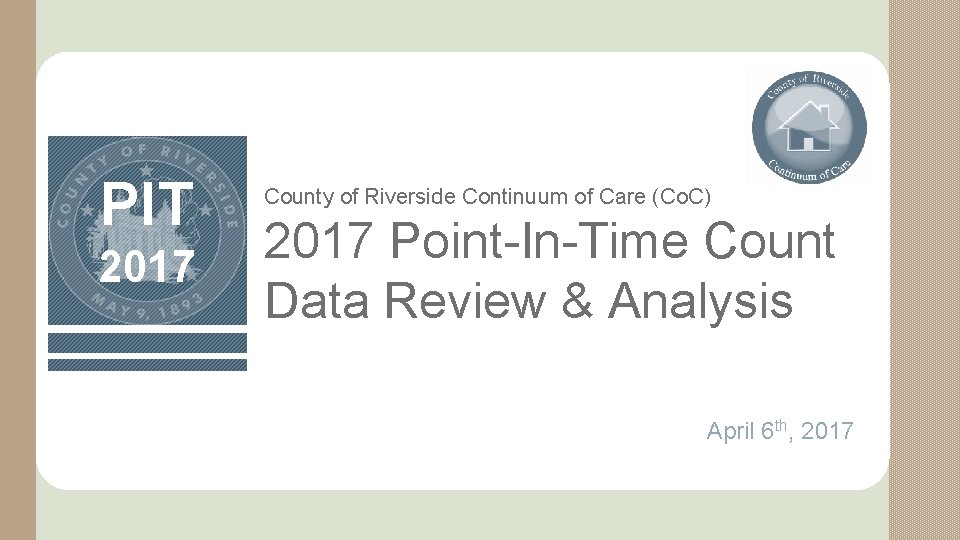
PIT 2017 County of Riverside Continuum of Care (Co. C) 2017 Point-In-Time Count Data Review & Analysis April 6 th, 2017
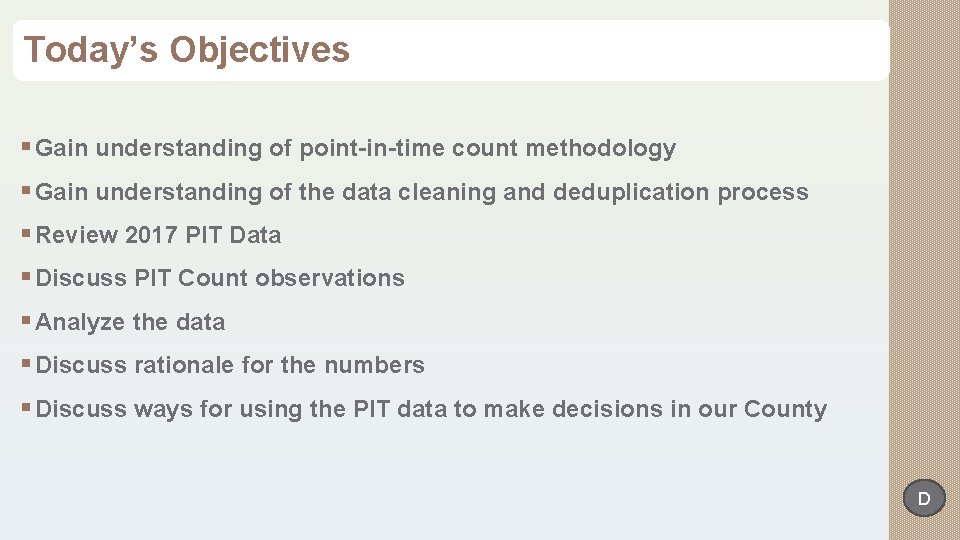
Today’s Objectives § Gain understanding of point-in-time count methodology § Gain understanding of the data cleaning and deduplication process § Review 2017 PIT Data § Discuss PIT Count observations § Analyze the data § Discuss rationale for the numbers § Discuss ways for using the PIT data to make decisions in our County D
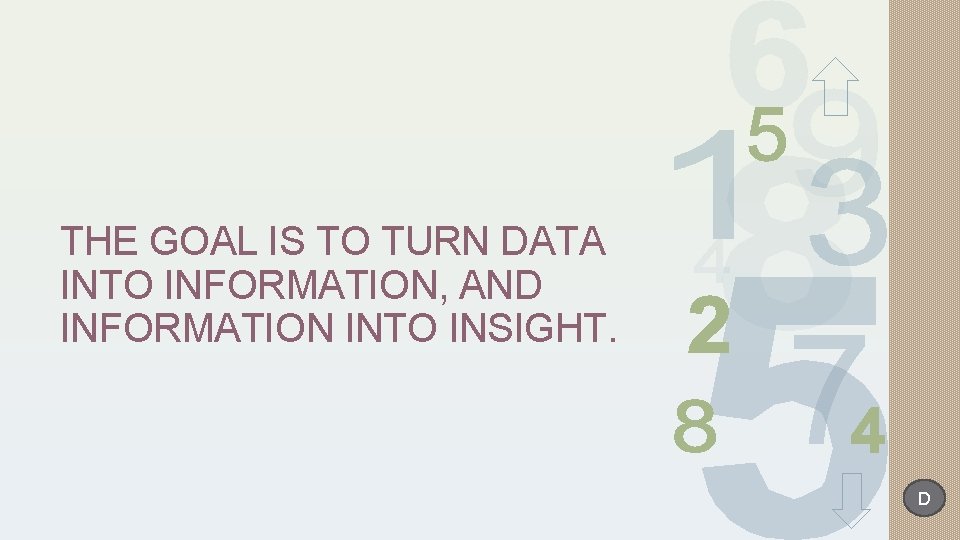
THE GOAL IS TO TURN DATA INTO INFORMATION, AND INFORMATION INTO INSIGHT. D
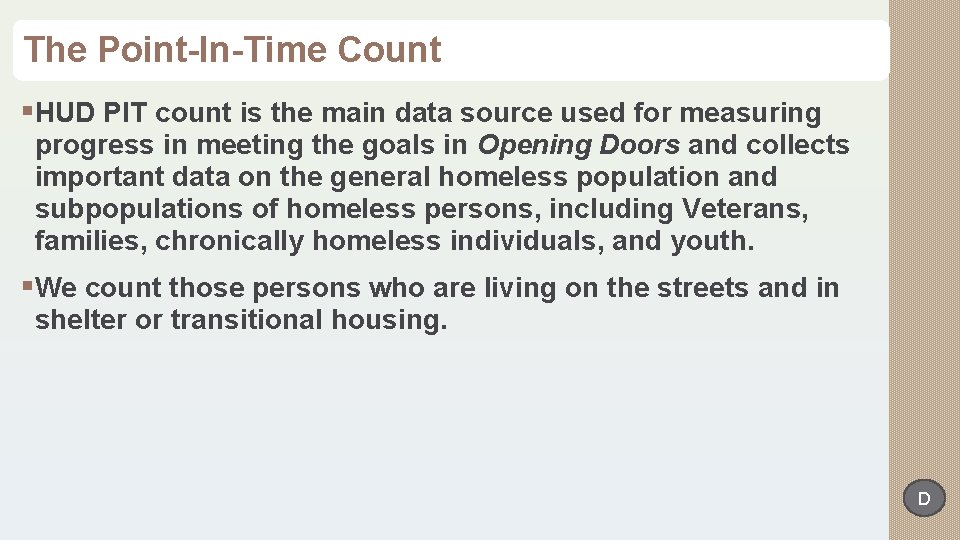
The Point-In-Time Count §HUD PIT count is the main data source used for measuring progress in meeting the goals in Opening Doors and collects important data on the general homeless population and subpopulations of homeless persons, including Veterans, families, chronically homeless individuals, and youth. §We count those persons who are living on the streets and in shelter or transitional housing. D
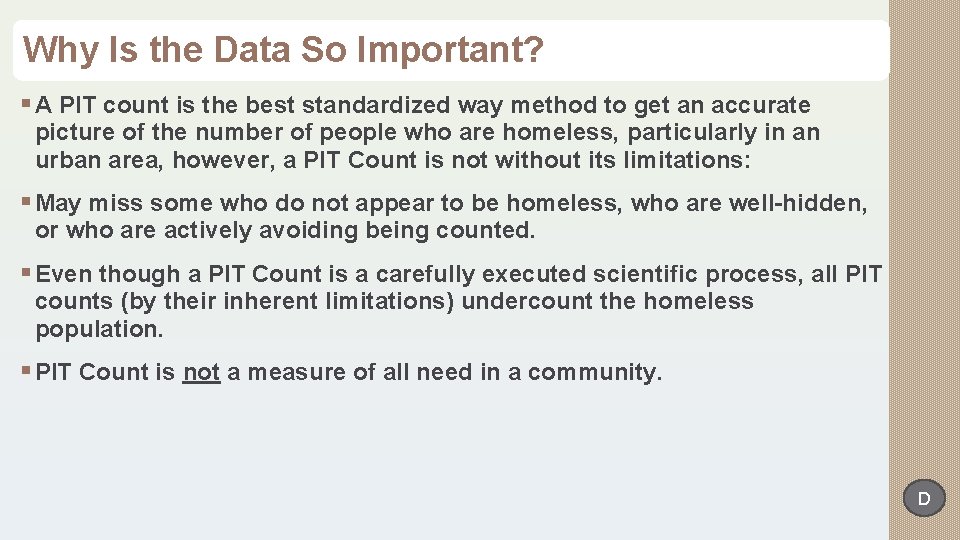
Why Is the Data So Important? § A PIT count is the best standardized way method to get an accurate picture of the number of people who are homeless, particularly in an urban area, however, a PIT Count is not without its limitations: § May miss some who do not appear to be homeless, who are well-hidden, or who are actively avoiding being counted. § Even though a PIT Count is a carefully executed scientific process, all PIT counts (by their inherent limitations) undercount the homeless population. § PIT Count is not a measure of all need in a community. D
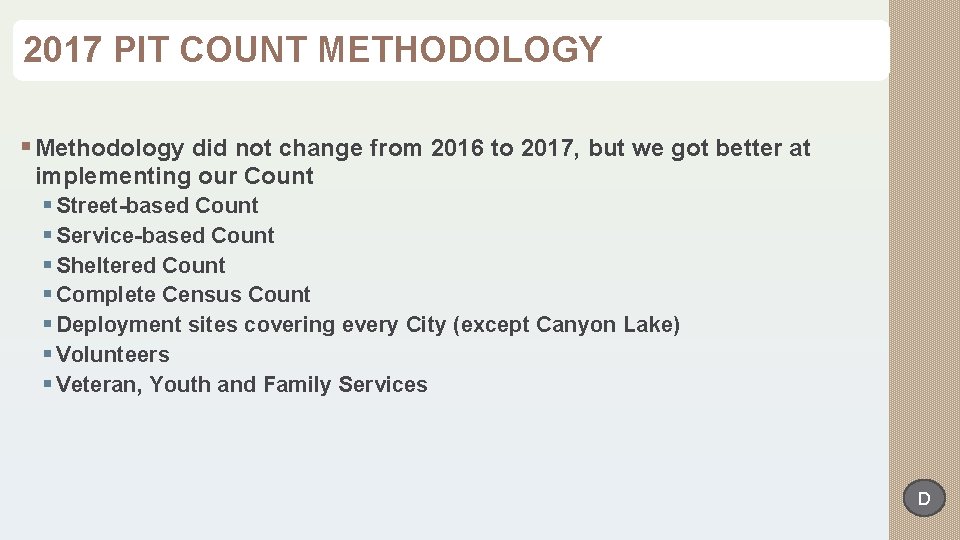
2017 PIT COUNT METHODOLOGY § Methodology did not change from 2016 to 2017, but we got better at implementing our Count § Street-based Count § Service-based Count § Sheltered Count § Complete Census Count § Deployment sites covering every City (except Canyon Lake) § Volunteers § Veteran, Youth and Family Services D
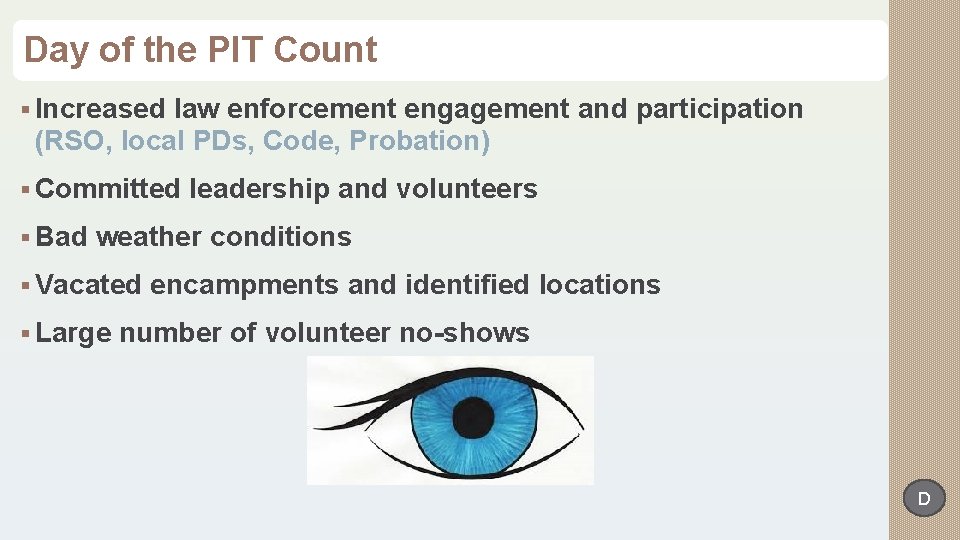
Day of the PIT Count § Increased law enforcement engagement and participation (RSO, local PDs, Code, Probation) § Committed leadership and volunteers § Bad weather conditions § Vacated encampments and identified locations § Large number of volunteer no-shows D
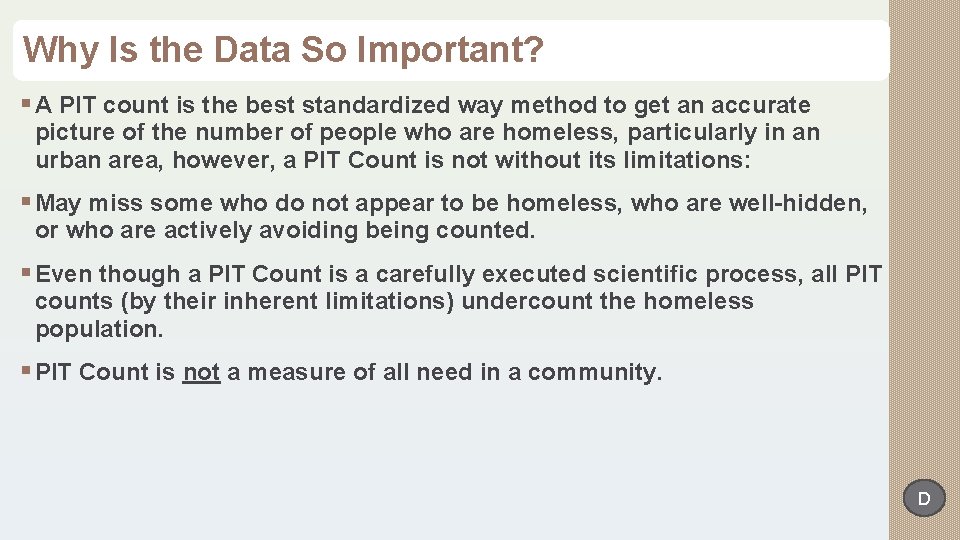
Why Is the Data So Important? § A PIT count is the best standardized way method to get an accurate picture of the number of people who are homeless, particularly in an urban area, however, a PIT Count is not without its limitations: § May miss some who do not appear to be homeless, who are well-hidden, or who are actively avoiding being counted. § Even though a PIT Count is a carefully executed scientific process, all PIT counts (by their inherent limitations) undercount the homeless population. § PIT Count is not a measure of all need in a community. D
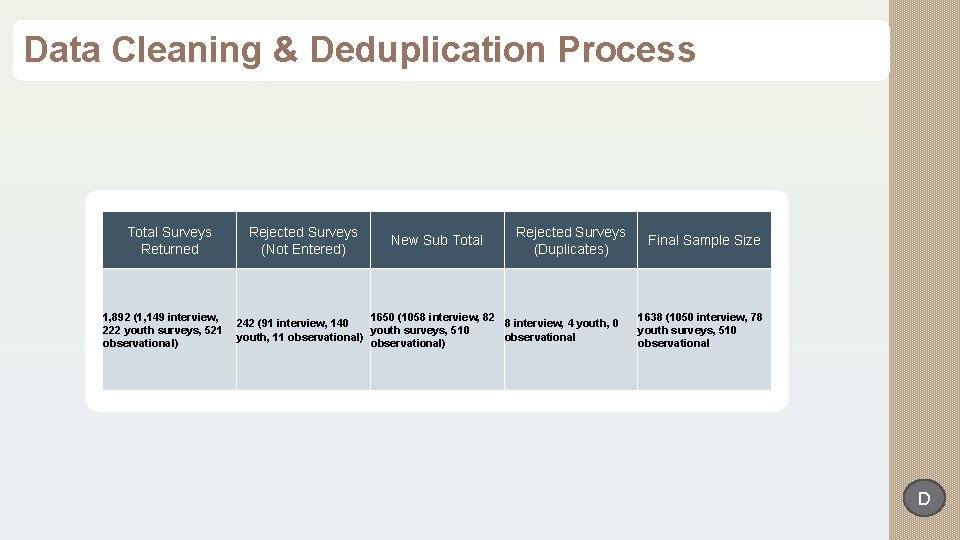
Data Cleaning & Deduplication Process Total Surveys Returned Rejected Surveys (Not Entered) New Sub Total Rejected Surveys (Duplicates) 1, 892 (1, 149 interview, 1650 (1058 interview, 82 242 (91 interview, 140 8 interview, 4 youth, 0 222 youth surveys, 521 youth surveys, 510 youth, 11 observational) Final Sample Size 1638 (1050 interview, 78 youth surveys, 510 observational D
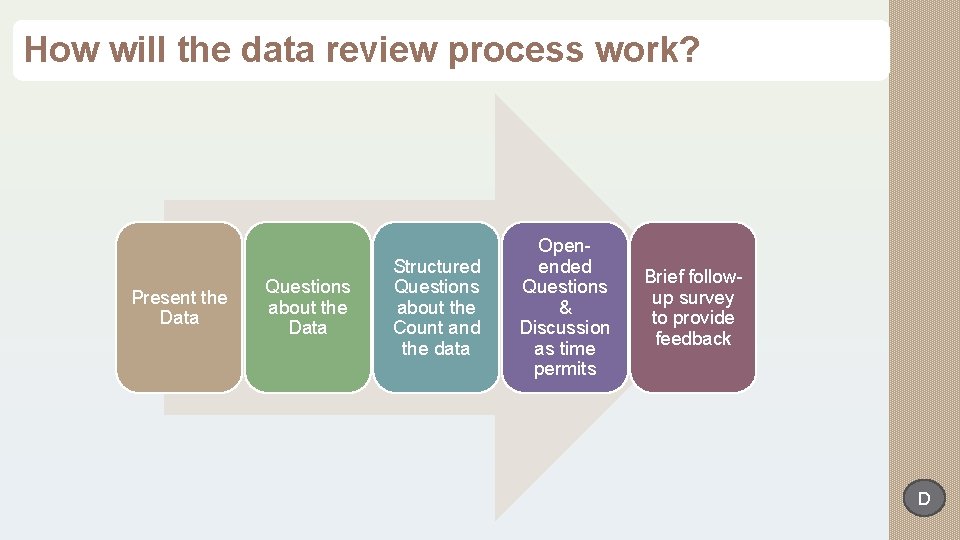
How will the data review process work? Present the Data Questions about the Data Structured Questions about the Count and the data Openended Questions & Discussion as time permits Brief followup survey to provide feedback D
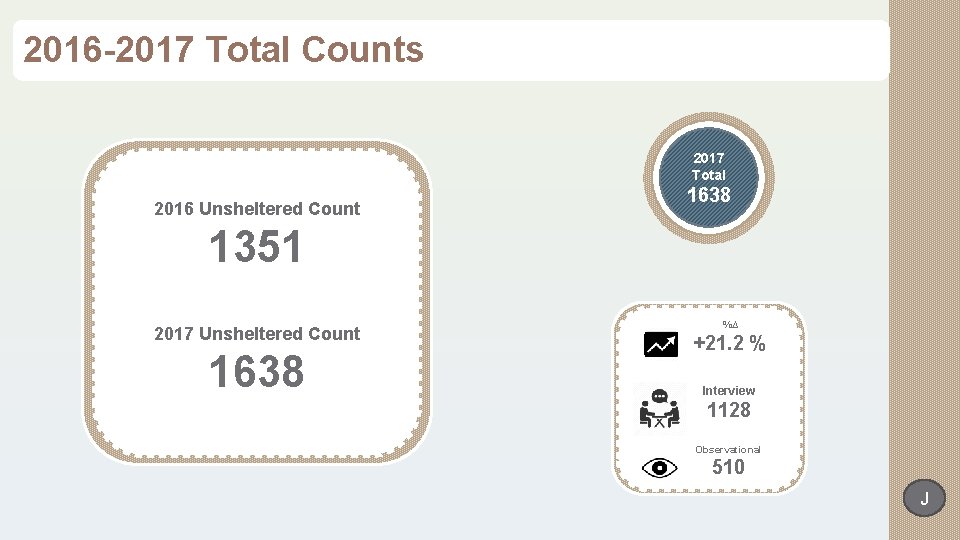
2016 -2017 Total Counts 2017 Total 2016 Unsheltered Count 1638 1351 2017 Unsheltered Count 1638 %∆ +21. 2 % Interview 1128 Observational 510 J
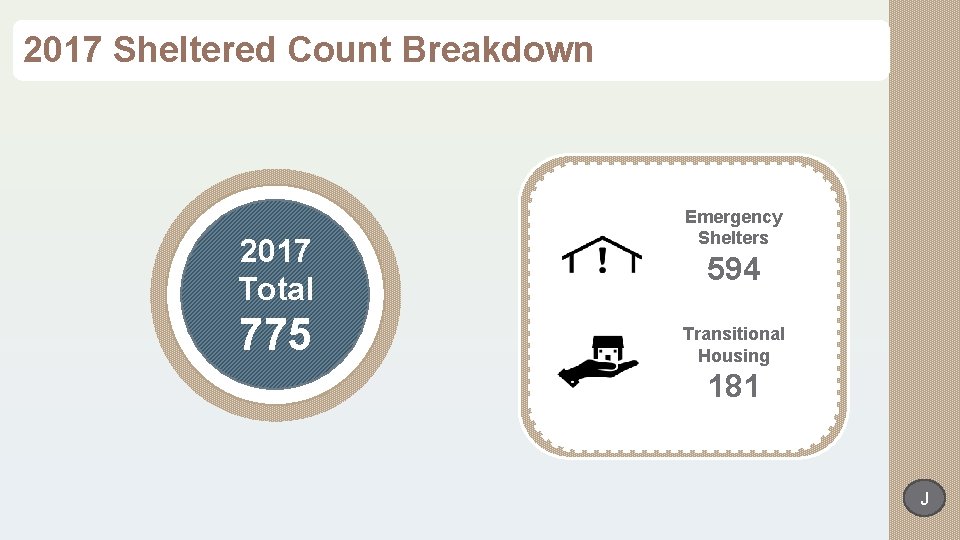
2017 Sheltered Count Breakdown 2017 Total 775 Emergency Shelters 594 Transitional Housing 181 J
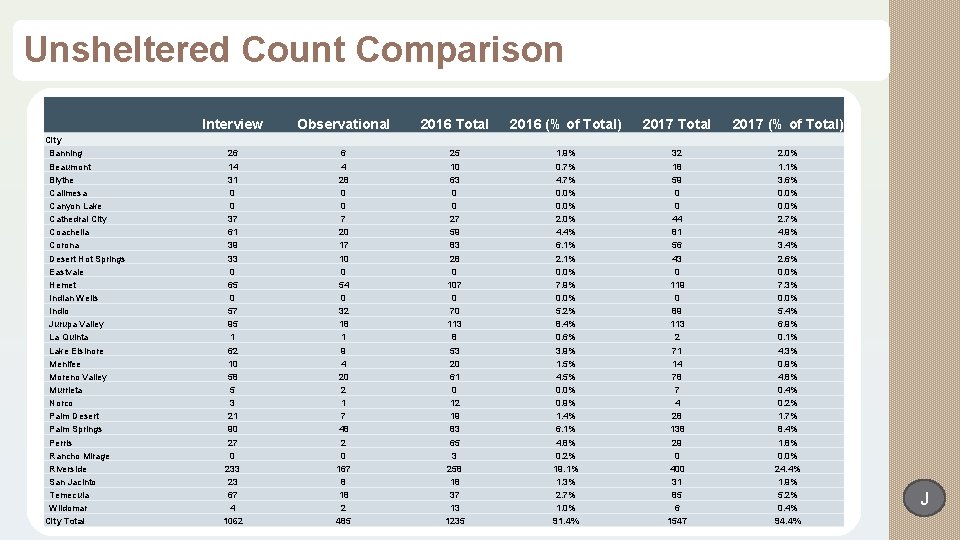
Unsheltered Count Comparison City Banning Beaumont Blythe Calimesa Canyon Lake Cathedral City Coachella Corona Desert Hot Springs Eastvale Hemet Indian Wells Indio Jurupa Valley La Quinta Lake Elsinore Menifee Moreno Valley Murrieta Norco Palm Desert Palm Springs Perris Rancho Mirage Riverside San Jacinto Temecula Wildomar City Total Interview Observational 2016 Total 2016 (% of Total) 2017 Total 2017 (% of Total) 26 14 31 0 0 37 61 39 33 0 65 0 57 95 1 62 10 58 5 3 21 90 27 0 233 23 67 4 1062 6 4 28 0 0 7 20 17 10 0 54 0 32 18 1 9 4 20 2 1 7 48 2 0 167 8 18 2 485 25 10 63 0 0 27 59 83 28 0 107 0 70 113 8 53 20 61 0 12 19 83 65 3 258 18 37 13 1235 1. 9% 0. 7% 4. 7% 0. 0% 2. 0% 4. 4% 6. 1% 2. 1% 0. 0% 7. 9% 0. 0% 5. 2% 8. 4% 0. 6% 3. 9% 1. 5% 4. 5% 0. 0% 0. 9% 1. 4% 6. 1% 4. 8% 0. 2% 19. 1% 1. 3% 2. 7% 1. 0% 91. 4% 32 18 59 0 0 44 81 56 43 0 119 0 89 113 2 71 14 78 7 4 28 138 29 0 400 31 85 6 1547 2. 0% 1. 1% 3. 6% 0. 0% 2. 7% 4. 9% 3. 4% 2. 6% 0. 0% 7. 3% 0. 0% 5. 4% 6. 9% 0. 1% 4. 3% 0. 9% 4. 8% 0. 4% 0. 2% 1. 7% 8. 4% 1. 8% 0. 0% 24. 4% 1. 9% 5. 2% 0. 4% 94. 4% J
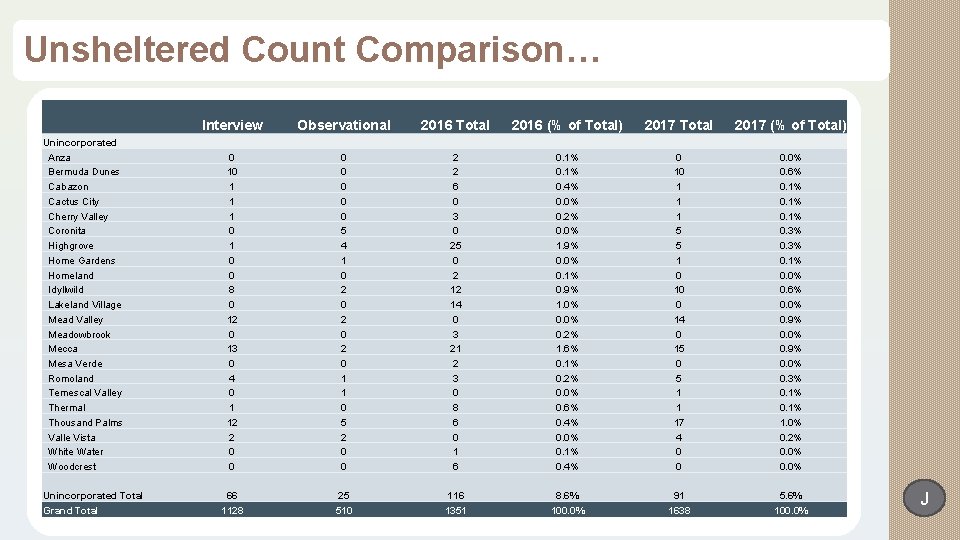
Unsheltered Count Comparison… Unincorporated Anza Bermuda Dunes Cabazon Cactus City Cherry Valley Coronita Highgrove Home Gardens Homeland Idyllwild Lakeland Village Mead Valley Meadowbrook Mecca Mesa Verde Romoland Temescal Valley Thermal Thousand Palms Valle Vista White Water Woodcrest Unincorporated Total Grand Total Interview Observational 2016 Total 2016 (% of Total) 2017 Total 2017 (% of Total) 0 10 1 1 1 0 0 8 0 12 0 13 0 4 0 1 12 2 0 0 0 0 0 5 4 1 0 2 0 2 0 1 1 0 5 2 0 0 2 2 6 0 3 0 25 0 2 12 14 0 3 21 2 3 0 8 6 0 1 6 0. 1% 0. 4% 0. 0% 0. 2% 0. 0% 1. 9% 0. 0% 0. 1% 0. 9% 1. 0% 0. 2% 1. 6% 0. 1% 0. 2% 0. 0% 0. 6% 0. 4% 0. 0% 0. 1% 0. 4% 0 10 1 1 1 5 5 1 0 10 0 14 0 15 0 5 1 1 17 4 0 0 0. 0% 0. 6% 0. 1% 0. 3% 0. 1% 0. 0% 0. 6% 0. 0% 0. 9% 0. 0% 0. 3% 0. 1% 1. 0% 0. 2% 0. 0% 66 1128 25 510 116 1351 8. 6% 100. 0% 91 1638 5. 6% 100. 0% J
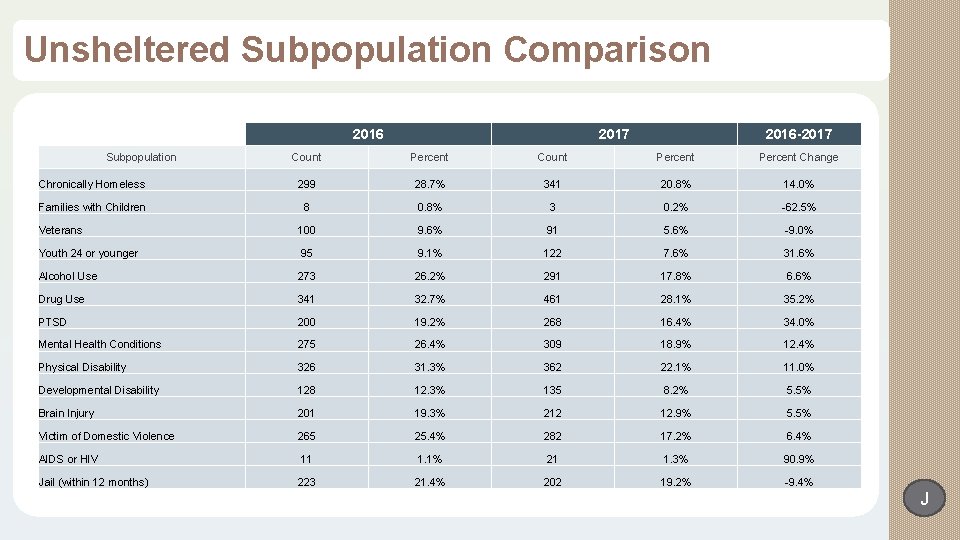
Unsheltered Subpopulation Comparison 2016 Subpopulation 2017 2016 -2017 Count Percent Change Chronically Homeless 299 28. 7% 341 20. 8% 14. 0% Families with Children 8 0. 8% 3 0. 2% -62. 5% Veterans 100 9. 6% 91 5. 6% -9. 0% Youth 24 or younger 95 9. 1% 122 7. 6% 31. 6% Alcohol Use 273 26. 2% 291 17. 8% 6. 6% Drug Use 341 32. 7% 461 28. 1% 35. 2% PTSD 200 19. 2% 268 16. 4% 34. 0% Mental Health Conditions 275 26. 4% 309 18. 9% 12. 4% Physical Disability 326 31. 3% 362 22. 1% 11. 0% Developmental Disability 128 12. 3% 135 8. 2% 5. 5% Brain Injury 201 19. 3% 212 12. 9% 5. 5% Victim of Domestic Violence 265 25. 4% 282 17. 2% 6. 4% AIDS or HIV 11 1. 1% 21 1. 3% 90. 9% Jail (within 12 months) 223 21. 4% 202 19. 2% -9. 4% J
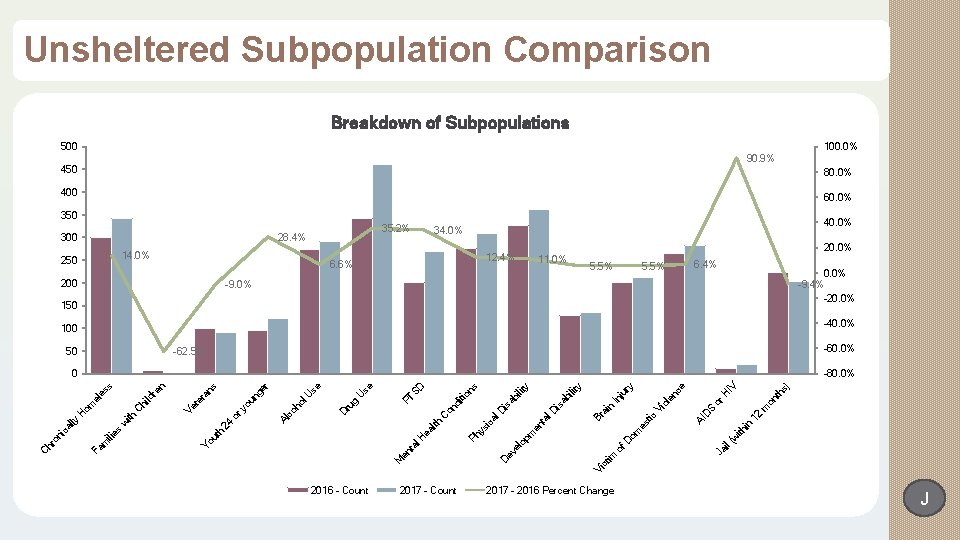
Unsheltered Subpopulation Comparison Breakdown of Subpopulations 500 100. 0% 90. 9% 450 80. 0% 400 60. 0% 350 35. 2% 28. 4% 300 14. 0% 250 40. 0% 34. 0% 6. 6% 200 20. 0% 12. 4% 11. 0% 6. 4% 5. 5% 0. 0% -9. 4% -20. 0% -9. 0% 150 -40. 0% 100 50 -60. 0% -62. 5% th s) m on H or Ja il (w ith in AI 12 D S ol Vi tic om es D of Vi ct im IV e ju In n ai Br el ev D en c ry ab ilit y op Ph ys m en ic a ta l. D is di on C lth ea ta l. H M en Yo ab ilit y ns tio SD PT D ru g U se se ho l. U co Al yo or 24 ut h w s ilie m Fa un g ns ra te re hi ld C ith Ve s el es om H lly ic a on hr C er -80. 0% n 0 2016 - Count 2017 - 2016 Percent Change J
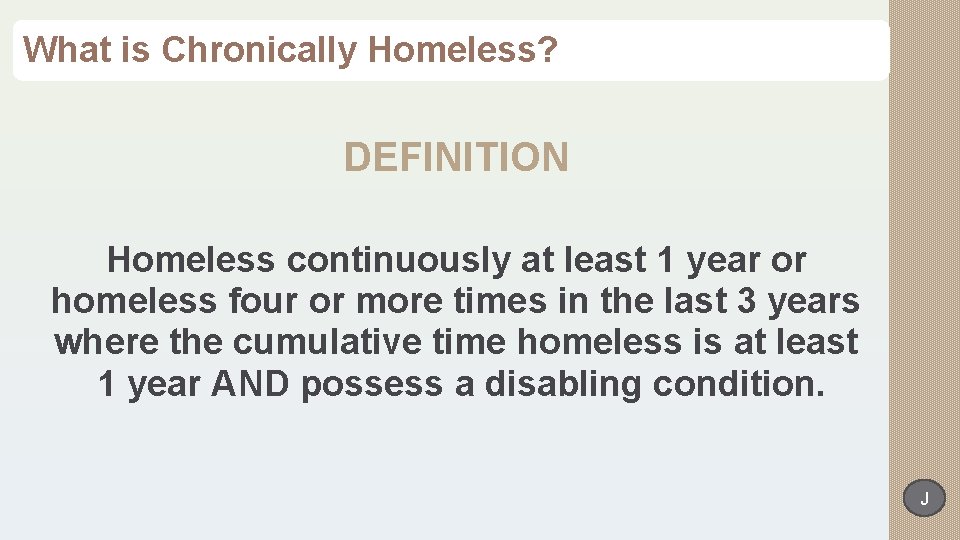
What is Chronically Homeless? DEFINITION Homeless continuously at least 1 year or homeless four or more times in the last 3 years where the cumulative time homeless is at least 1 year AND possess a disabling condition. J
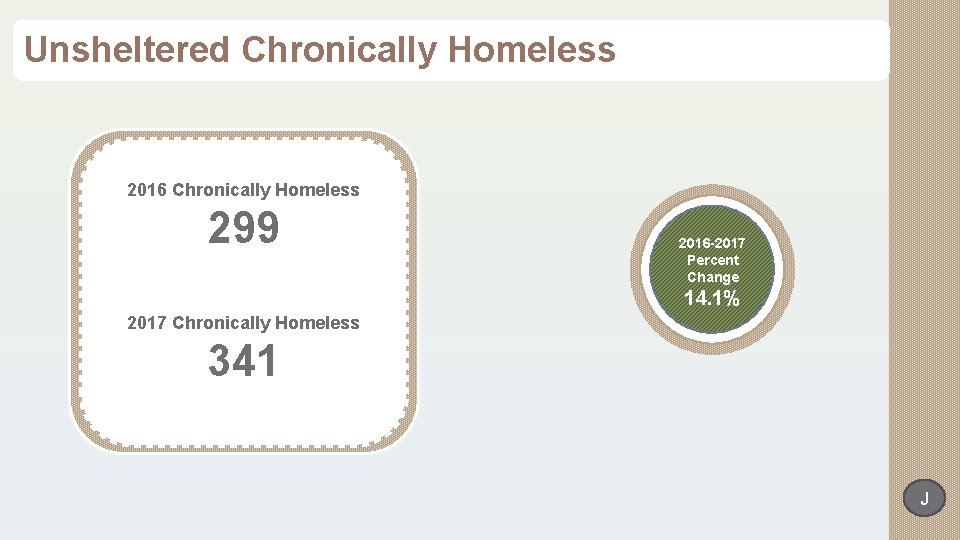
Unsheltered Chronically Homeless 2016 Chronically Homeless 299 2016 -2017 Percent Change 14. 1% 2017 Chronically Homeless 341 J
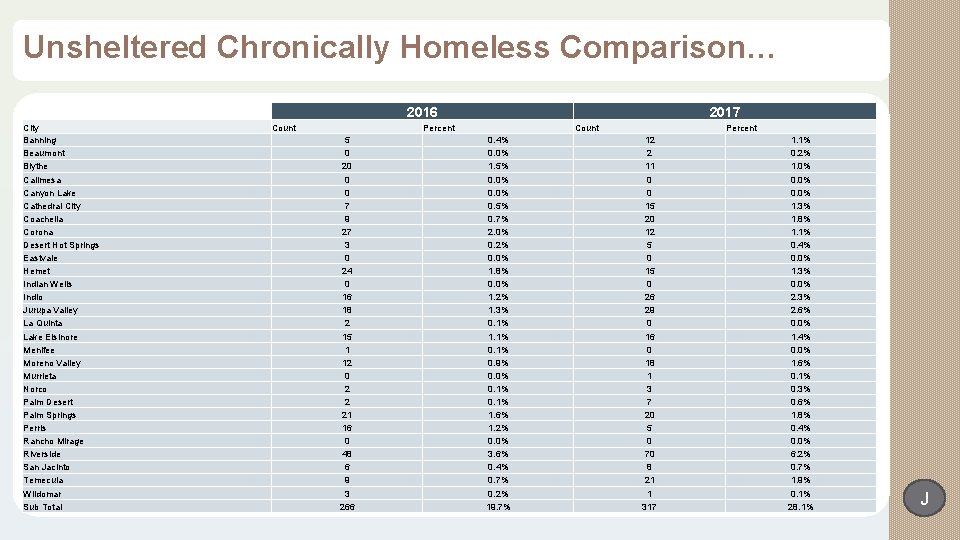
Unsheltered Chronically Homeless Comparison… 2016 City Banning Beaumont Blythe Calimesa Canyon Lake Cathedral City Coachella Corona Desert Hot Springs Eastvale Hemet Indian Wells Indio Jurupa Valley La Quinta Lake Elsinore Menifee Moreno Valley Murrieta Norco Palm Desert Palm Springs Perris Rancho Mirage Riverside San Jacinto Temecula Wildomar Sub Total Count 2017 Percent 5 0 20 0 0 7 9 27 3 0 24 0 16 18 2 15 1 12 0 2 2 21 16 0 48 6 9 3 266 Count 0. 4% 0. 0% 1. 5% 0. 0% 0. 5% 0. 7% 2. 0% 0. 2% 0. 0% 1. 8% 0. 0% 1. 2% 1. 3% 0. 1% 1. 1% 0. 9% 0. 0% 0. 1% 1. 6% 1. 2% 0. 0% 3. 6% 0. 4% 0. 7% 0. 2% 19. 7% Percent 12 2 11 0 0 15 20 12 5 0 15 0 26 29 0 16 0 18 1 3 7 20 5 0 70 8 21 1 317 1. 1% 0. 2% 1. 0% 0. 0% 1. 3% 1. 8% 1. 1% 0. 4% 0. 0% 1. 3% 0. 0% 2. 3% 2. 6% 0. 0% 1. 4% 0. 0% 1. 6% 0. 1% 0. 3% 0. 6% 1. 8% 0. 4% 0. 0% 6. 2% 0. 7% 1. 9% 0. 1% 28. 1% J
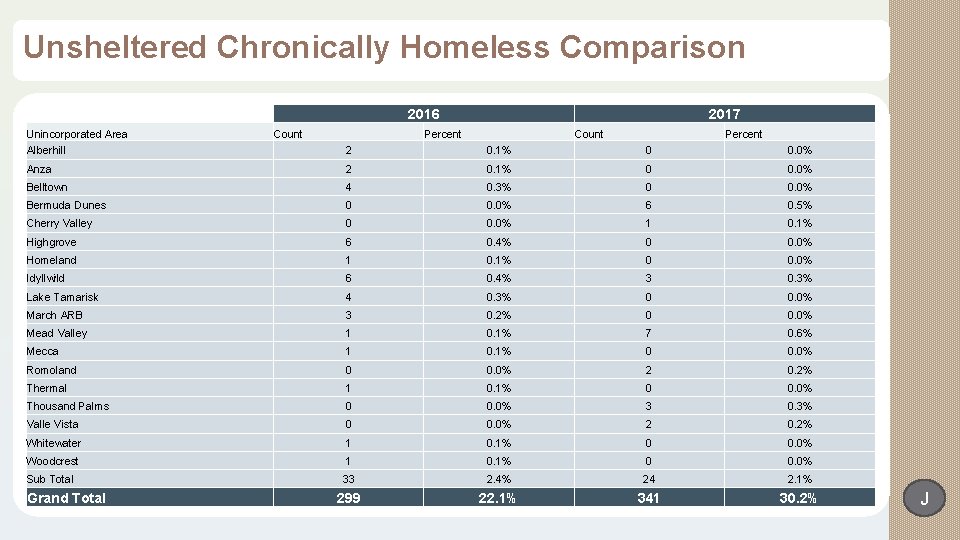
Unsheltered Chronically Homeless Comparison 2016 Unincorporated Area Alberhill Count 2017 Percent Count Percent 2 0. 1% 0 0. 0% Anza 2 0. 1% 0 0. 0% Belltown 4 0. 3% 0 0. 0% Bermuda Dunes 0 0. 0% 6 0. 5% Cherry Valley 0 0. 0% 1 0. 1% Highgrove 6 0. 4% 0 0. 0% Homeland 1 0. 1% 0 0. 0% Idyllwild 6 0. 4% 3 0. 3% Lake Tamarisk 4 0. 3% 0 0. 0% March ARB 3 0. 2% 0 0. 0% Mead Valley 1 0. 1% 7 0. 6% Mecca 1 0. 1% 0 0. 0% Romoland 0 0. 0% 2 0. 2% Thermal 1 0. 1% 0 0. 0% Thousand Palms 0 0. 0% 3 0. 3% Valle Vista 0 0. 0% 2 0. 2% Whitewater 1 0. 1% 0 0. 0% Woodcrest 1 0. 1% 0 0. 0% Sub Total 33 2. 4% 24 2. 1% 299 22. 1% 341 30. 2% Grand Total J
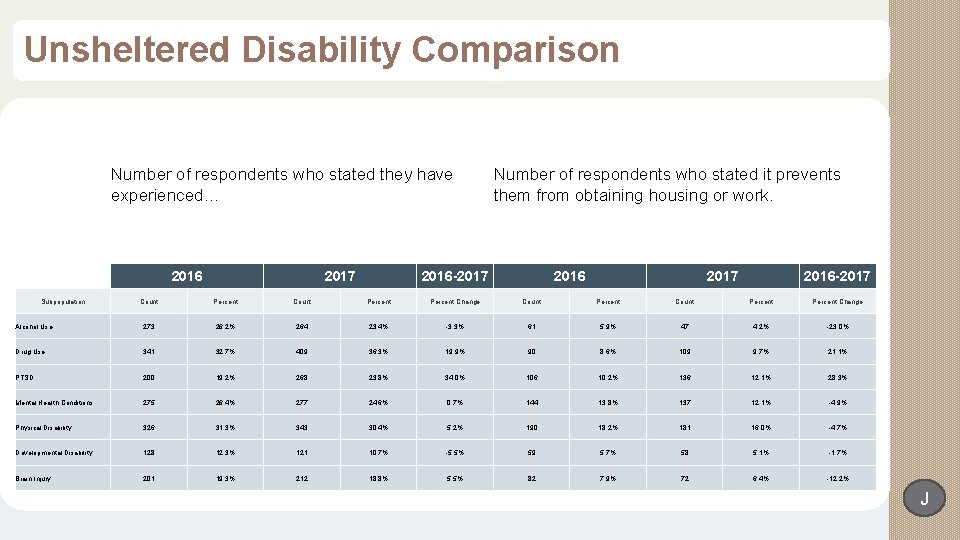
Unsheltered Disability Comparison Number of respondents who stated they have experienced… 2016 Subpopulation 2017 Number of respondents who stated it prevents them from obtaining housing or work. 2016 -2017 2016 -2017 Count Percent Count Percent Change Alcohol Use 273 26. 2% 264 23. 4% -3. 3% 61 5. 9% 47 4. 2% -23. 0% Drug Use 341 32. 7% 409 36. 3% 19. 9% 90 8. 6% 109 9. 7% 21. 1% PTSD 200 19. 2% 268 23. 8% 34. 0% 106 10. 2% 136 12. 1% 28. 3% Mental Health Conditions 275 26. 4% 277 24. 6% 0. 7% 144 13. 8% 137 12. 1% -4. 9% Physical Disability 326 31. 3% 343 30. 4% 5. 2% 190 18. 2% 181 16. 0% -4. 7% Developmental Disability 128 12. 3% 121 10. 7% -5. 5% 59 5. 7% 58 5. 1% -1. 7% Brain Injury 201 19. 3% 212 18. 8% 5. 5% 82 7. 9% 72 6. 4% -12. 2% J
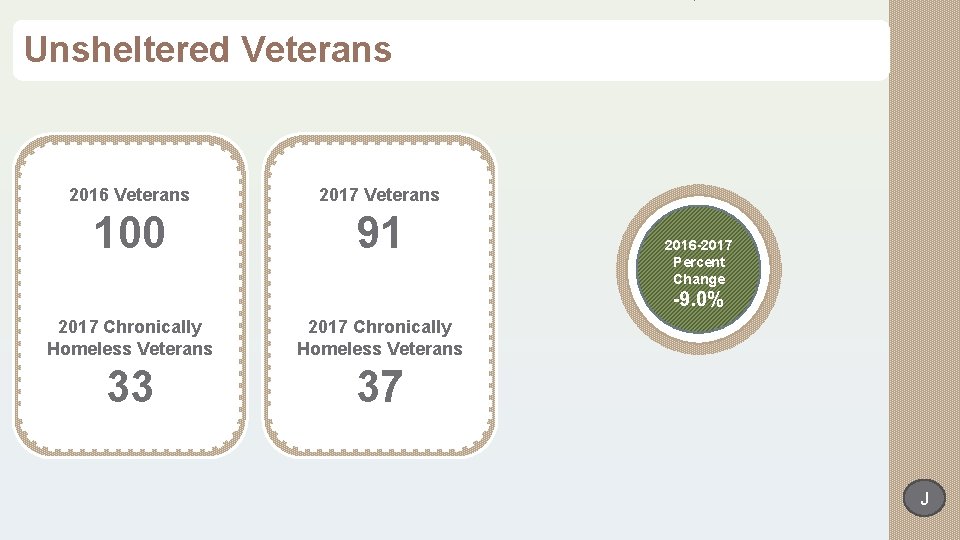
Unsheltered Veterans 2016 Veterans 2017 Veterans 100 91 2016 -2017 Percent Change -9. 0% 2017 Chronically Homeless Veterans 33 37 J
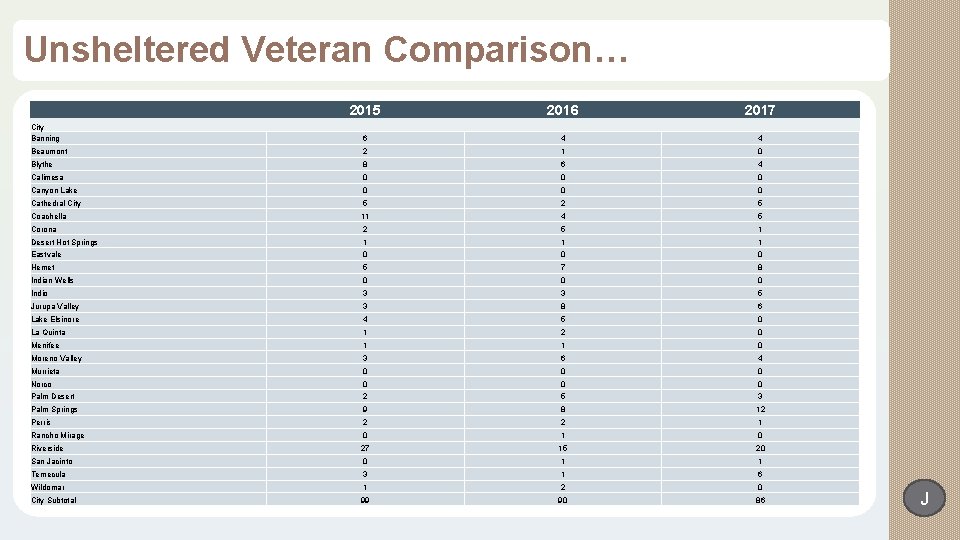
Unsheltered Veteran Comparison… 2015 2016 2017 City Banning 6 4 4 Beaumont 2 1 0 Blythe 8 6 4 Calimesa 0 0 0 Canyon Lake 0 0 0 Cathedral City 5 2 5 Coachella 11 4 5 Corona 2 5 1 Desert Hot Springs 1 1 1 Eastvale 0 0 0 Hemet 5 7 8 Indian Wells 0 0 0 Indio 3 3 5 Jurupa Valley 3 8 6 Lake Elsinore 4 5 0 La Quinta 1 2 0 Menifee 1 1 0 Moreno Valley 3 6 4 Murrieta 0 0 0 Norco 0 0 0 Palm Desert 2 5 3 Palm Springs 9 8 12 Perris 2 2 1 Rancho Mirage 0 1 0 Riverside 27 15 20 San Jacinto 0 1 1 Temecula 3 1 6 Wildomar 1 2 0 City Subtotal 99 90 86 J
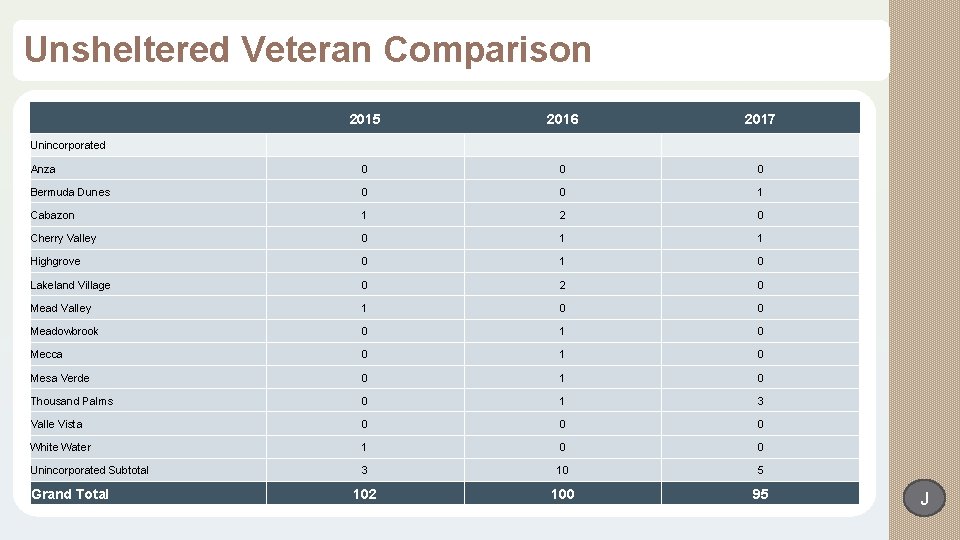
Unsheltered Veteran Comparison 2015 2016 2017 Unincorporated Anza 0 0 0 Bermuda Dunes 0 0 1 Cabazon 1 2 0 Cherry Valley 0 1 1 Highgrove 0 1 0 Lakeland Village 0 2 0 Mead Valley 1 0 0 Meadowbrook 0 1 0 Mecca 0 1 0 Mesa Verde 0 1 0 Thousand Palms 0 1 3 Valle Vista 0 0 0 White Water 1 0 0 Unincorporated Subtotal 3 10 5 102 100 95 Grand Total J
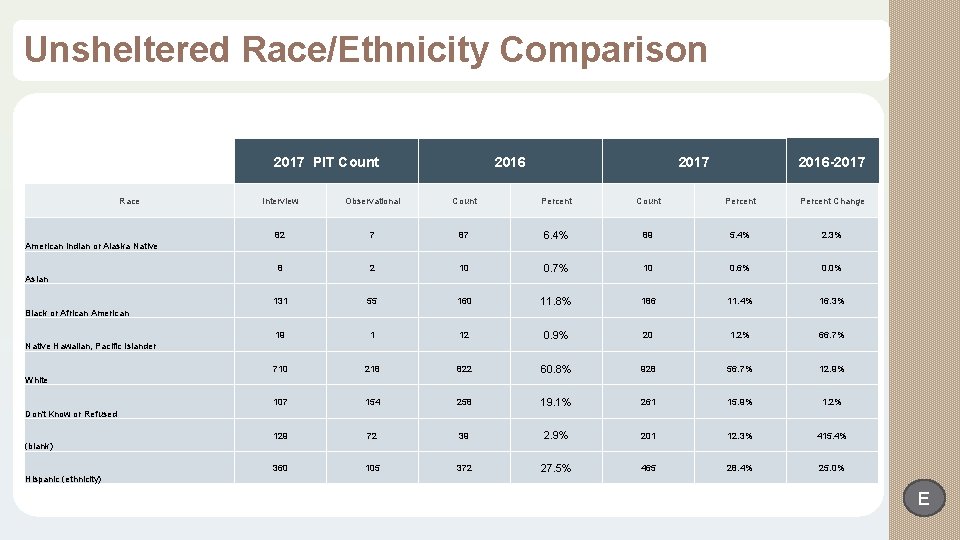
Unsheltered Race/Ethnicity Comparison 2017 PIT Count Race 2016 -2017 Interview Observational Count Percent Change 82 7 87 6. 4% 89 5. 4% 2. 3% 8 2 10 0. 7% 10 0. 6% 0. 0% 131 55 160 11. 8% 186 11. 4% 16. 3% 19 1 12 0. 9% 20 1. 2% 66. 7% 710 218 822 60. 8% 928 56. 7% 12. 9% 107 154 258 19. 1% 261 15. 9% 1. 2% 129 72 39 2. 9% 201 12. 3% 415. 4% 360 105 372 27. 5% 465 28. 4% 25. 0% American Indian or Alaska Native Asian Black or African American Native Hawaiian, Pacific Islander White Don't Know or Refused (blank) Hispanic (ethnicity) 2017 E
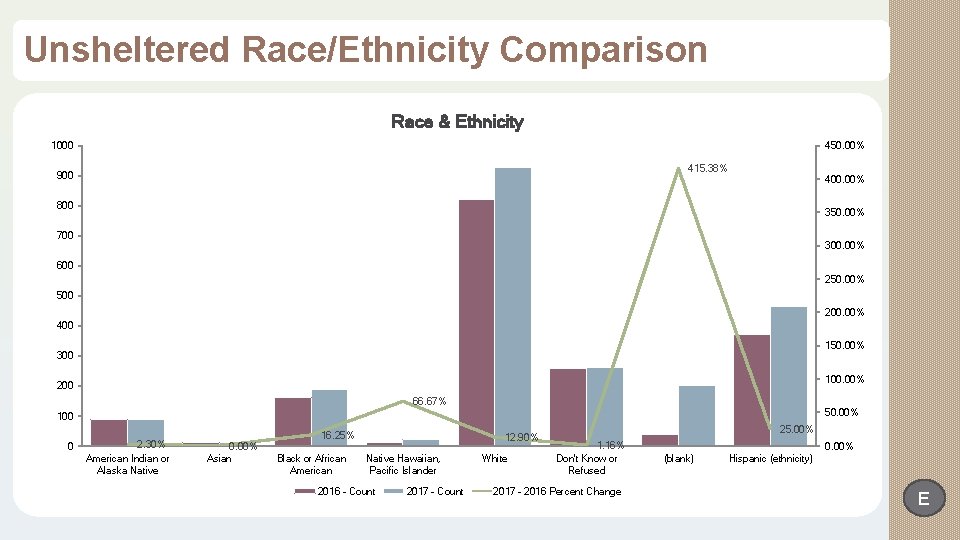
Unsheltered Race/Ethnicity Comparison Race & Ethnicity 1000 450. 00% 415. 38% 900 400. 00% 800 350. 00% 700 300. 00% 600 250. 00% 500 200. 00% 400 150. 00% 300 100. 00% 200 66. 67% 50. 00% 100 0 2. 30% American Indian or Alaska Native 0. 00% Asian 16. 25% Black or African American 12. 90% Native Hawaiian, Pacific Islander 2016 - Count 2017 - Count White 25. 00% 1. 16% Don't Know or Refused 2017 - 2016 Percent Change 0. 00% (blank) Hispanic (ethnicity) E
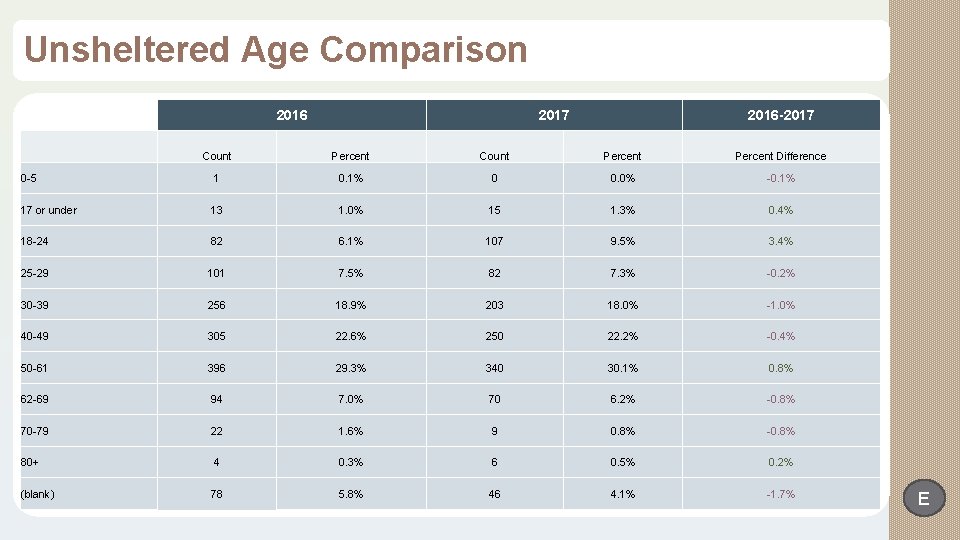
Unsheltered Age Comparison 2016 2017 2016 -2017 Count Percent Difference 0 -5 1 0. 1% 0 0. 0% -0. 1% 17 or under 13 1. 0% 15 1. 3% 0. 4% 18 -24 82 6. 1% 107 9. 5% 3. 4% 25 -29 101 7. 5% 82 7. 3% -0. 2% 30 -39 256 18. 9% 203 18. 0% -1. 0% 40 -49 305 22. 6% 250 22. 2% -0. 4% 50 -61 396 29. 3% 340 30. 1% 0. 8% 62 -69 94 7. 0% 70 6. 2% -0. 8% 70 -79 22 1. 6% 9 0. 8% -0. 8% 80+ 4 0. 3% 6 0. 5% 0. 2% (blank) 78 5. 8% 46 4. 1% -1. 7% E
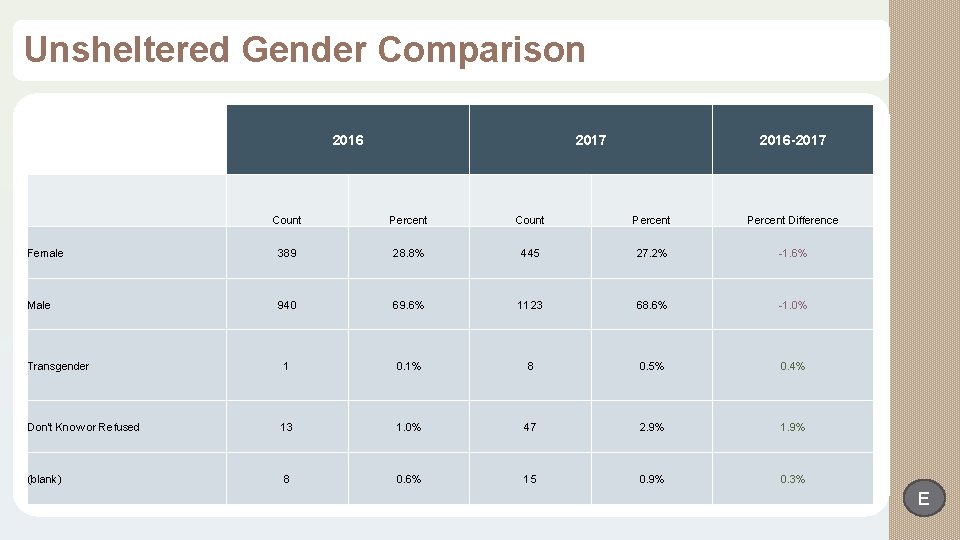
Unsheltered Gender Comparison 2016 2017 2016 -2017 Count Percent Difference Female 389 28. 8% 445 27. 2% -1. 6% Male 940 69. 6% 1123 68. 6% -1. 0% Transgender 1 0. 1% 8 0. 5% 0. 4% Don't Know or Refused 13 1. 0% 47 2. 9% 1. 9% (blank) 8 0. 6% 15 0. 9% 0. 3% E
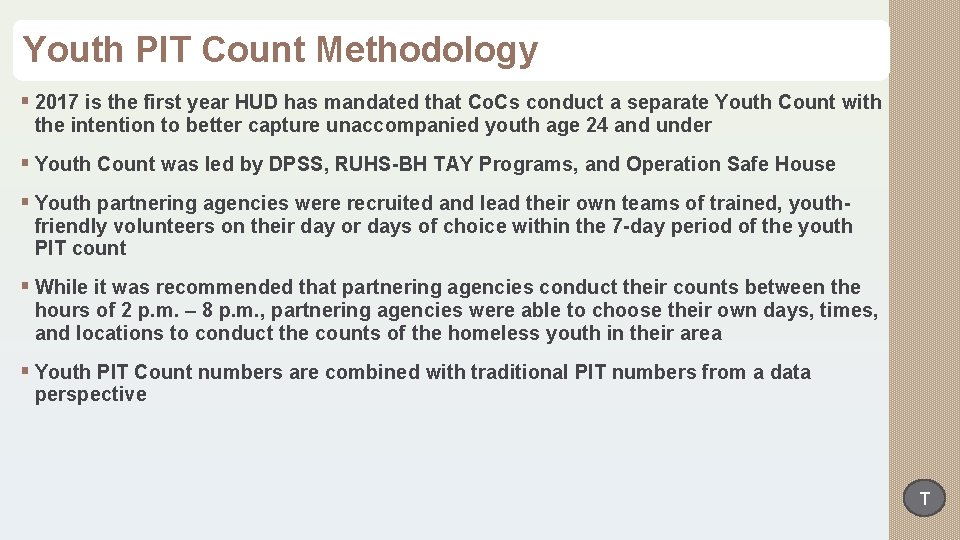
Youth PIT Count Methodology § 2017 is the first year HUD has mandated that Co. Cs conduct a separate Youth Count with the intention to better capture unaccompanied youth age 24 and under § Youth Count was led by DPSS, RUHS-BH TAY Programs, and Operation Safe House § Youth partnering agencies were recruited and lead their own teams of trained, youth- friendly volunteers on their day or days of choice within the 7 -day period of the youth PIT count § While it was recommended that partnering agencies conduct their counts between the hours of 2 p. m. – 8 p. m. , partnering agencies were able to choose their own days, times, and locations to conduct the counts of the homeless youth in their area § Youth PIT Count numbers are combined with traditional PIT numbers from a data perspective T
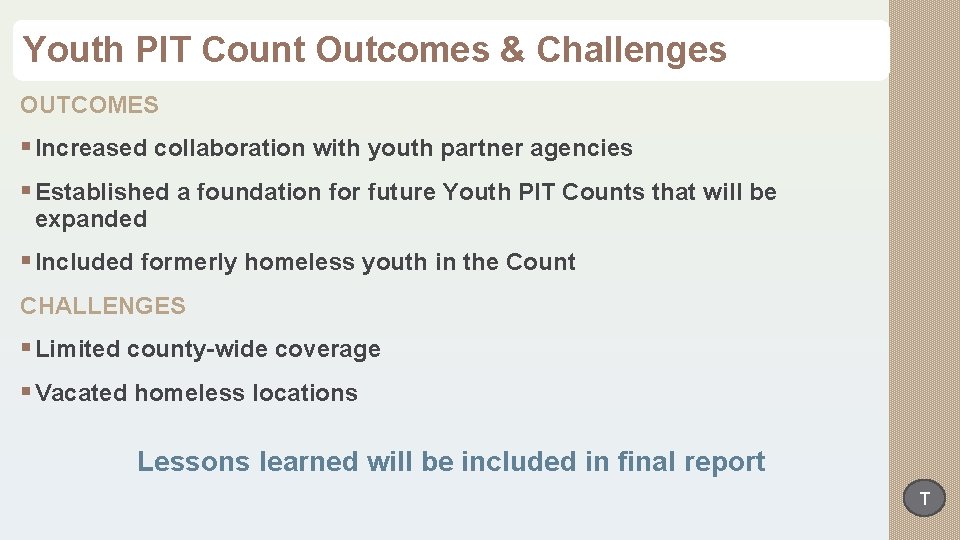
Youth PIT Count Outcomes & Challenges OUTCOMES § Increased collaboration with youth partner agencies § Established a foundation for future Youth PIT Counts that will be expanded § Included formerly homeless youth in the Count CHALLENGES § Limited county-wide coverage § Vacated homeless locations Lessons learned will be included in final report T
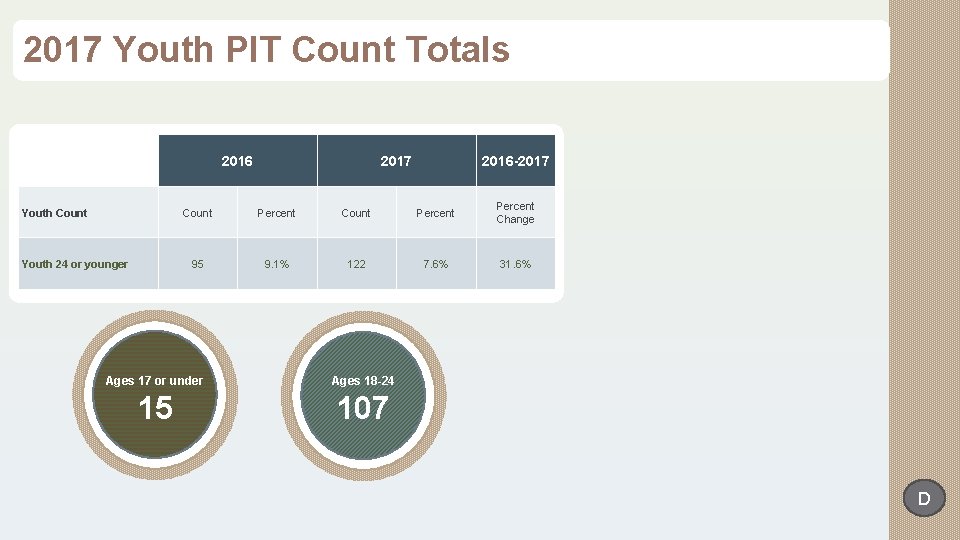
2017 Youth PIT Count Totals 2016 Youth Count Youth 24 or younger 2017 2016 -2017 Count Percent Change 95 9. 1% 122 7. 6% 31. 6% Ages 17 or under Ages 18 -24 15 107 D
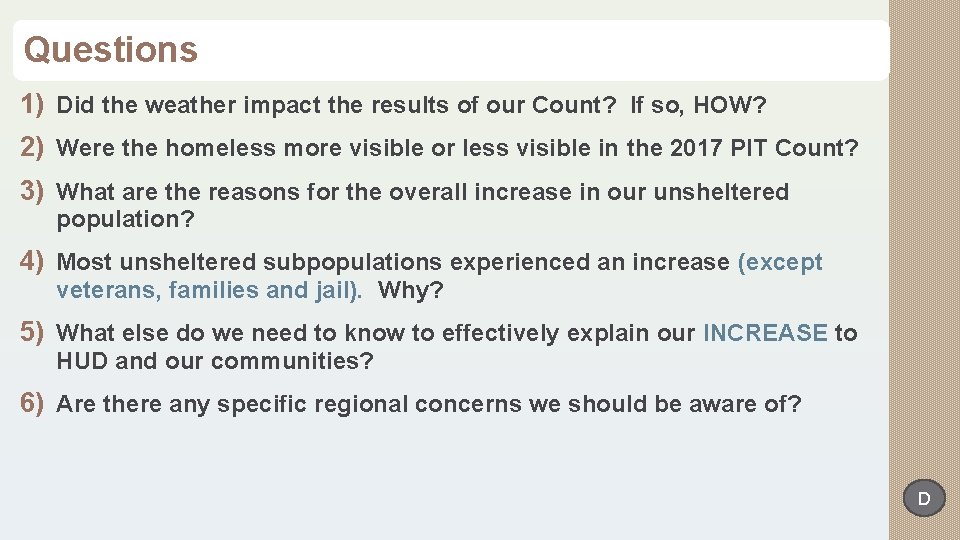
Questions 1) Did the weather impact the results of our Count? If so, HOW? 2) Were the homeless more visible or less visible in the 2017 PIT Count? 3) What are the reasons for the overall increase in our unsheltered population? 4) Most unsheltered subpopulations experienced an increase (except veterans, families and jail). Why? 5) What else do we need to know to effectively explain our INCREASE to HUD and our communities? 6) Are there any specific regional concerns we should be aware of? D
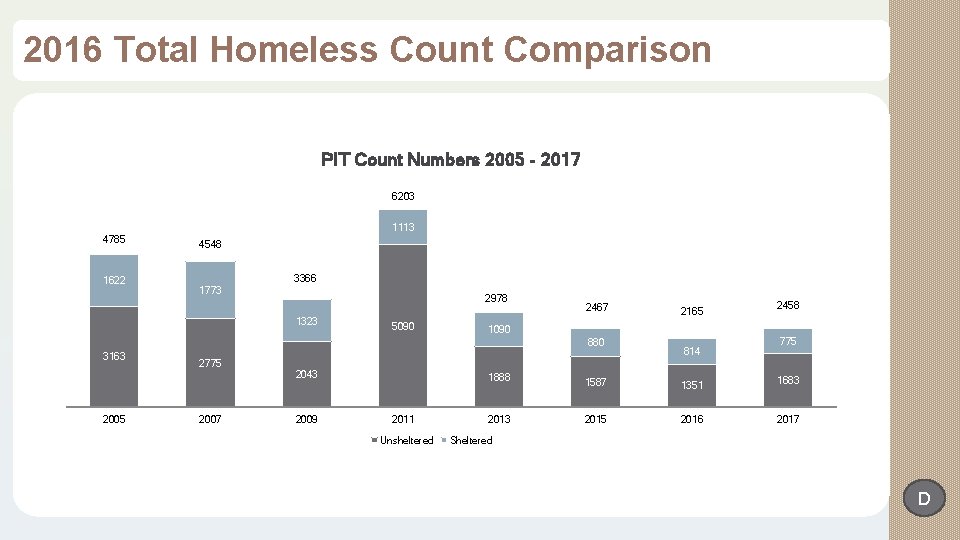
2016 Total Homeless Count Comparison PIT Count Numbers 2005 - 2017 6203 1113 4785 1622 4548 3366 1773 2978 1323 5090 2467 814 775 2043 2005 2458 1090 880 3163 2165 2007 2009 2011 Unsheltered 1888 1587 1351 2013 2015 2016 Sheltered 1683 2017 Total D
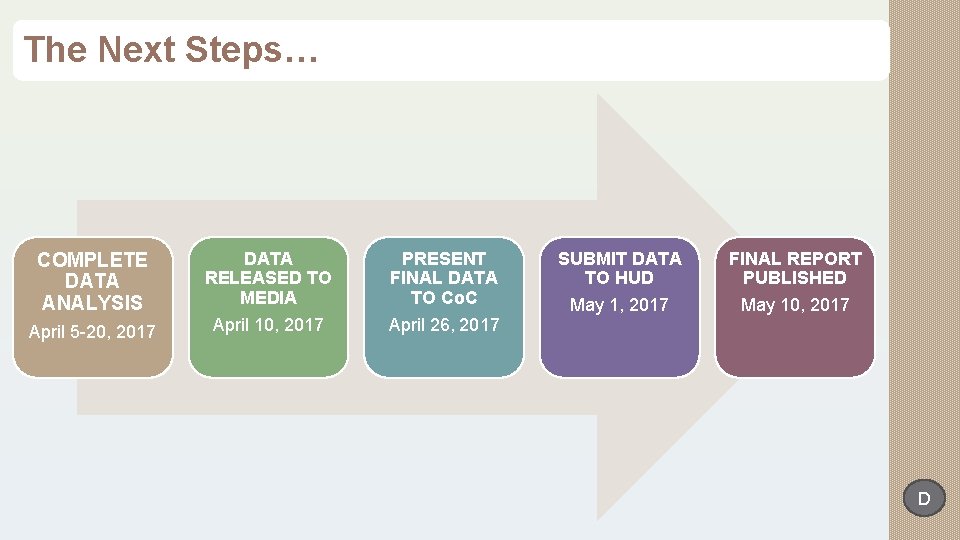
The Next Steps… COMPLETE DATA ANALYSIS April 5 -20, 2017 DATA RELEASED TO MEDIA April 10, 2017 PRESENT FINAL DATA TO Co. C April 26, 2017 SUBMIT DATA TO HUD May 1, 2017 FINAL REPORT PUBLISHED May 10, 2017 D
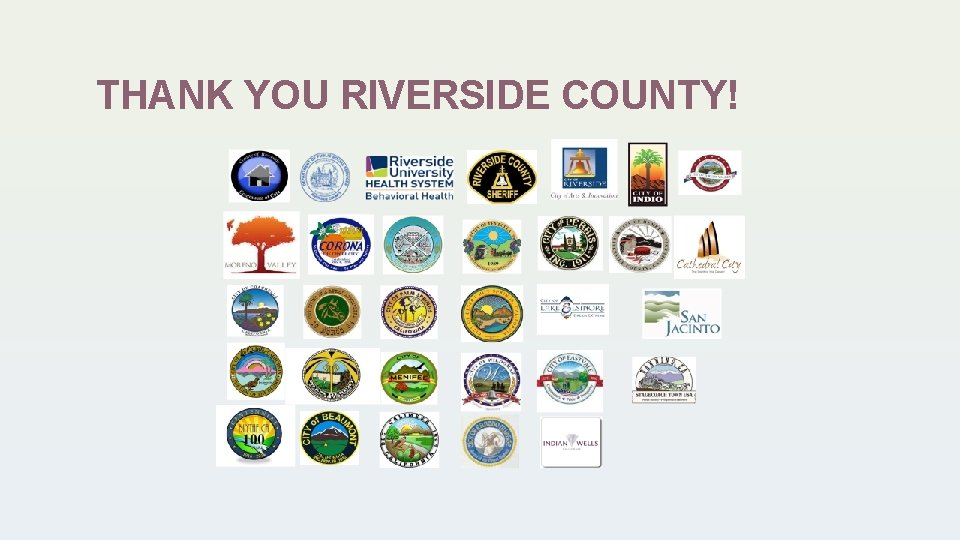
THANK YOU RIVERSIDE COUNTY!