Parallel Programming From Chapter 2 of Culler Singh
![Parallel Programming [From Chapter 2 of Culler, Singh, Gupta] Parallel Programming [From Chapter 2 of Culler, Singh, Gupta]](https://slidetodoc.com/presentation_image_h2/22670296ad10c718c04c034be8a4b63f/image-1.jpg)
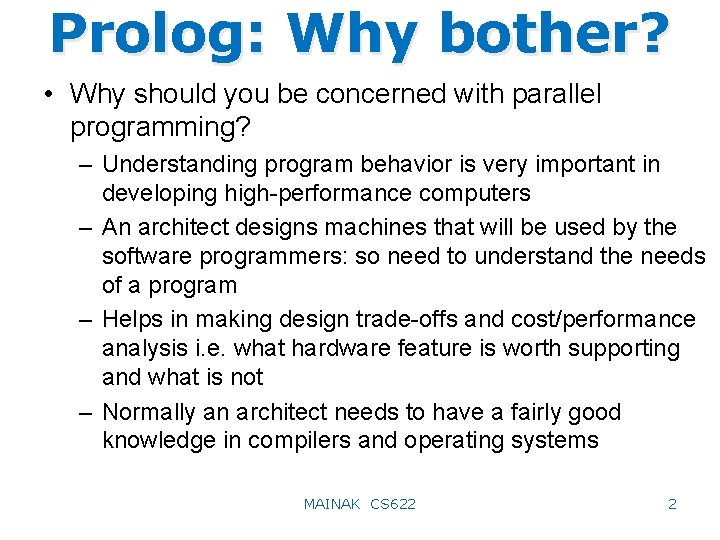
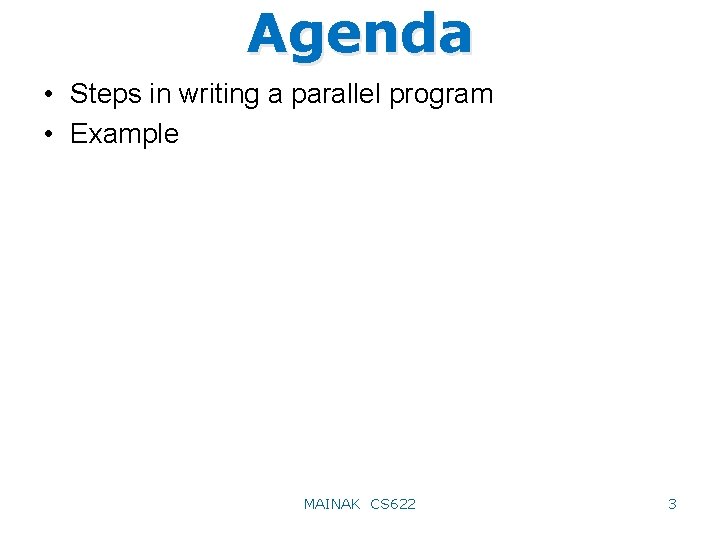
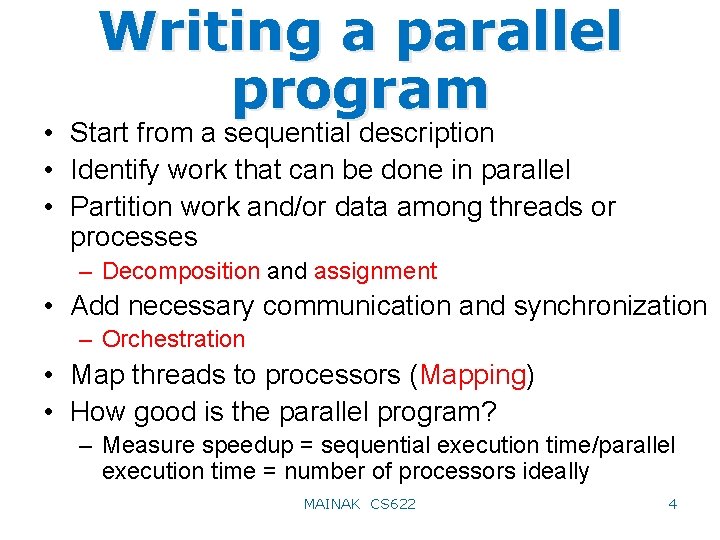
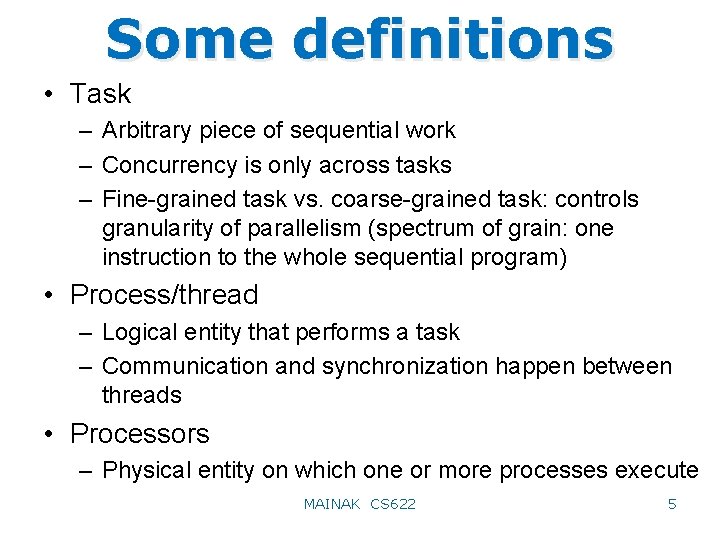
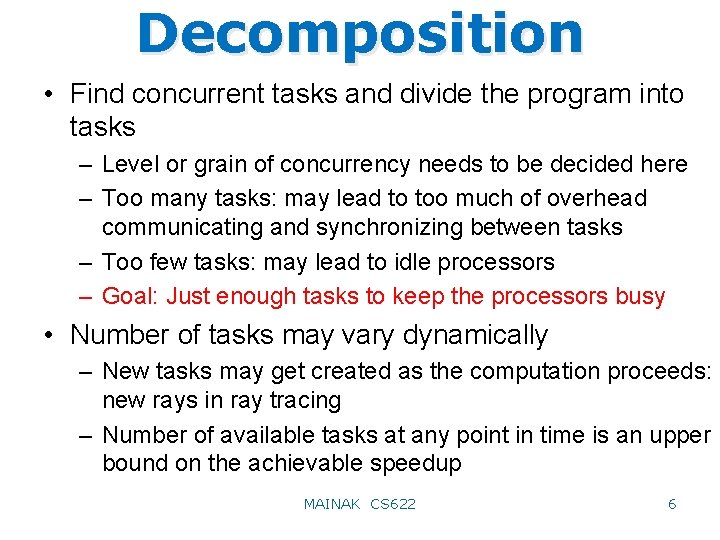
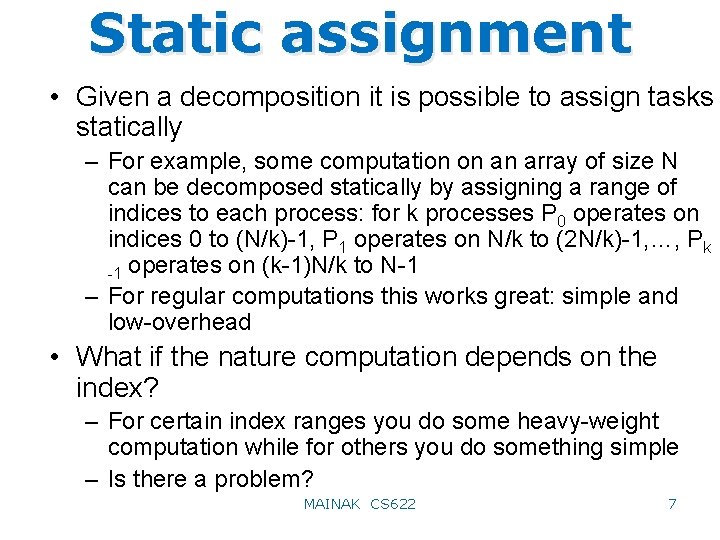
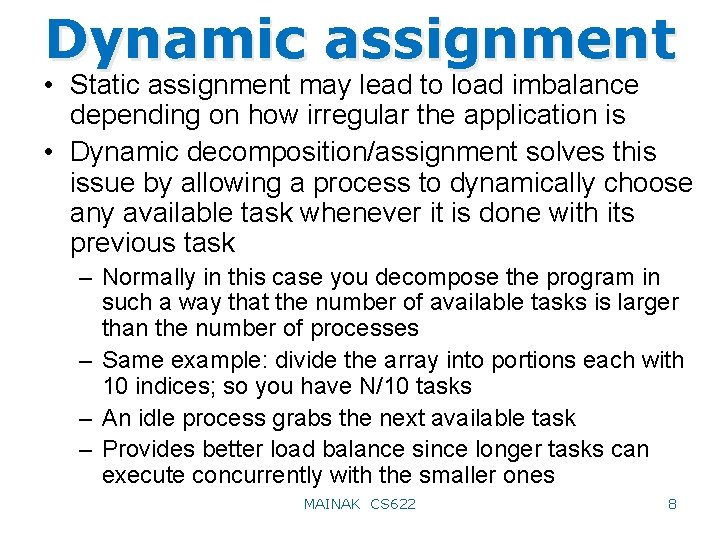
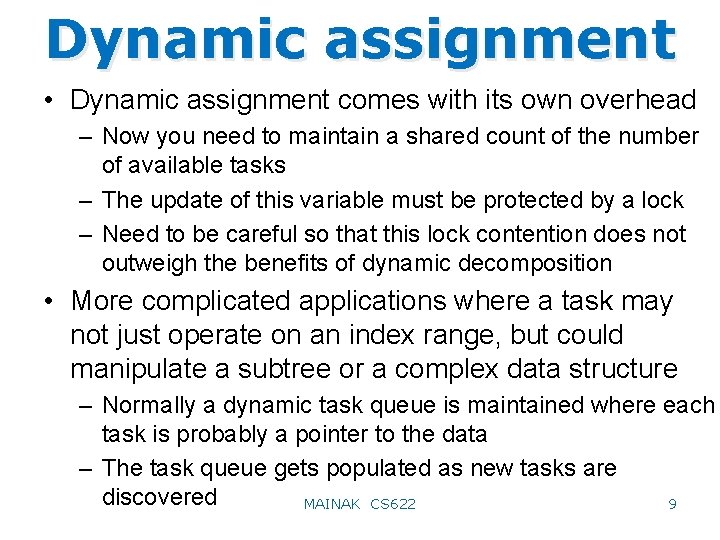
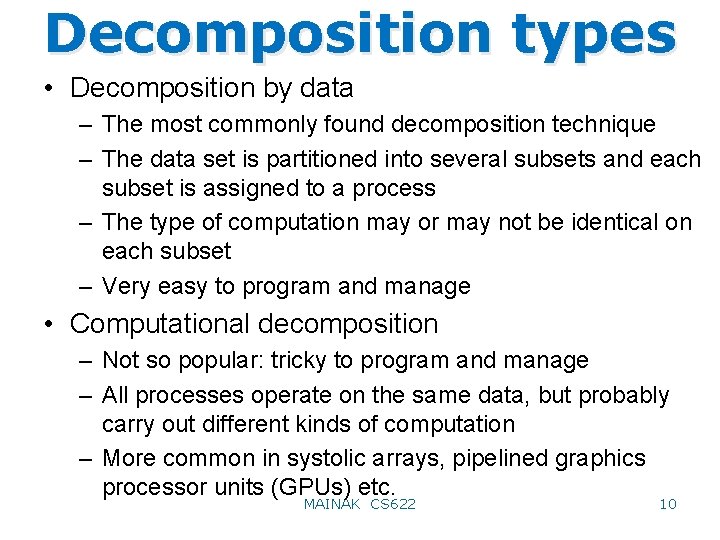
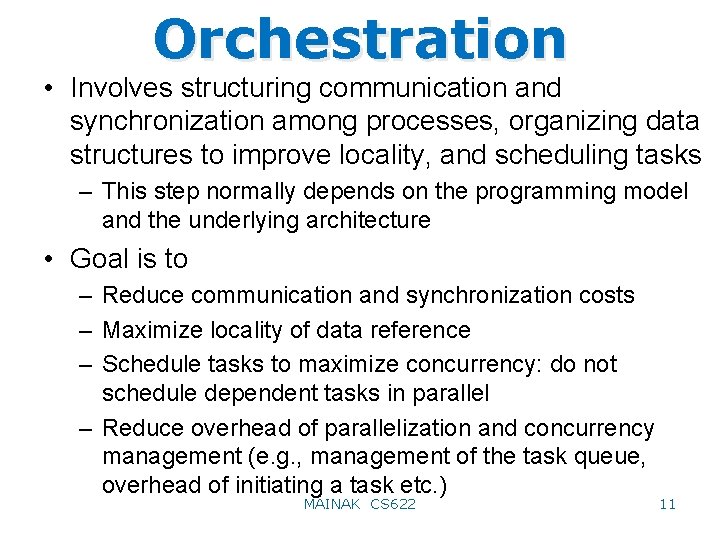
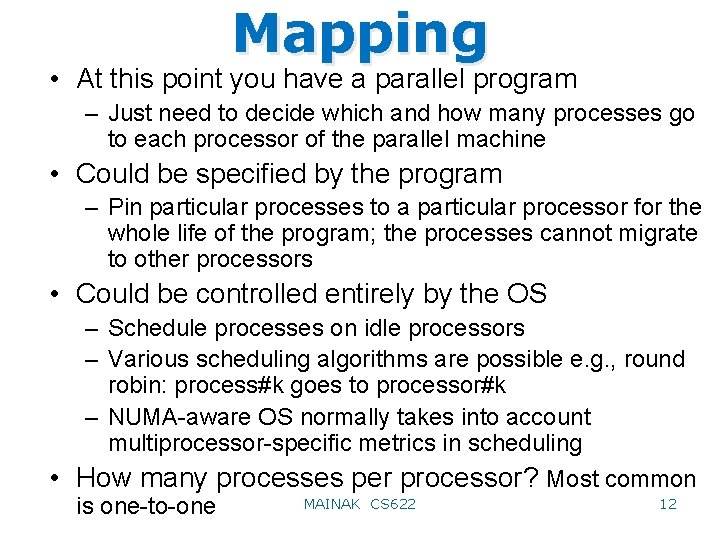
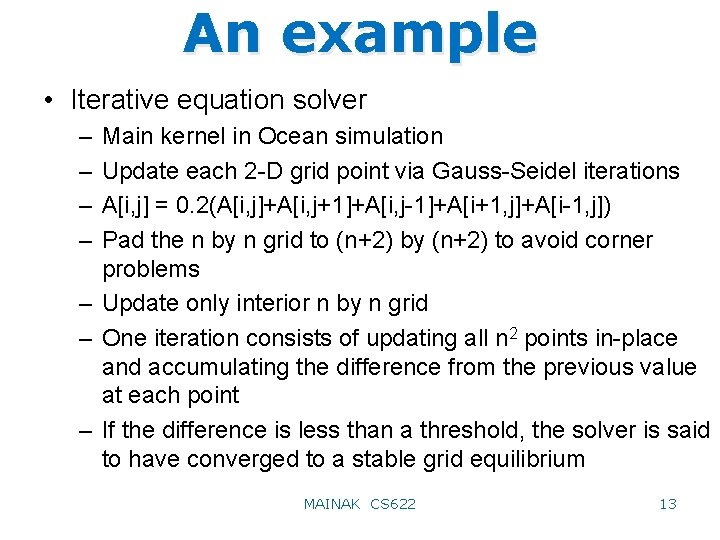
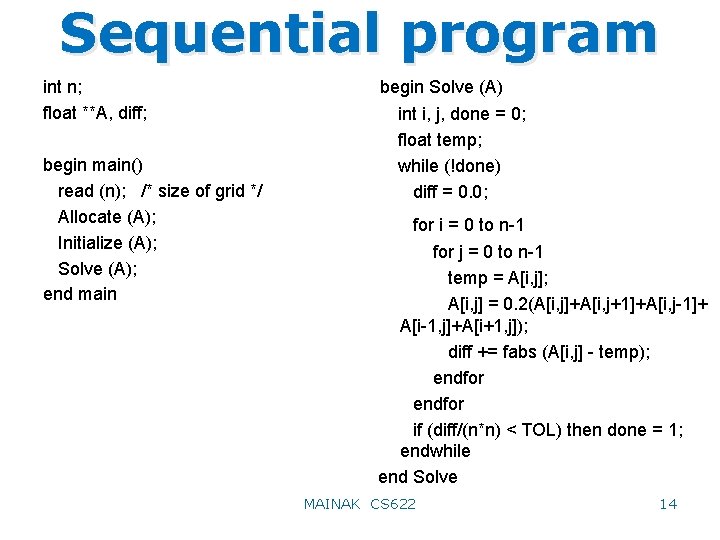
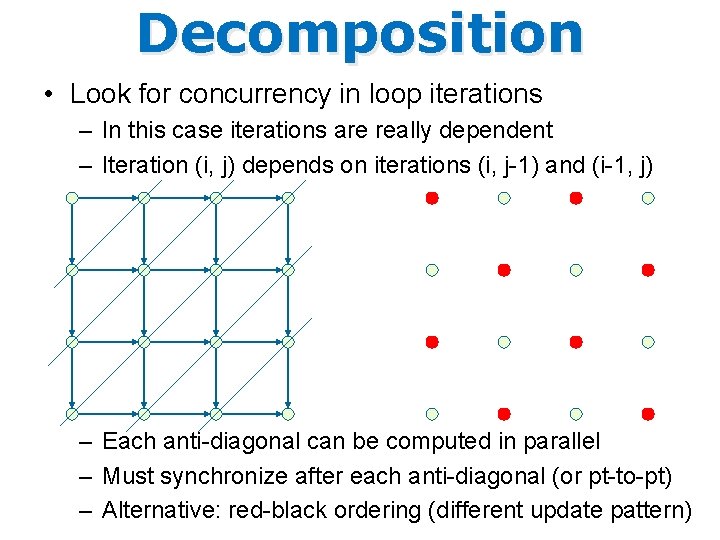
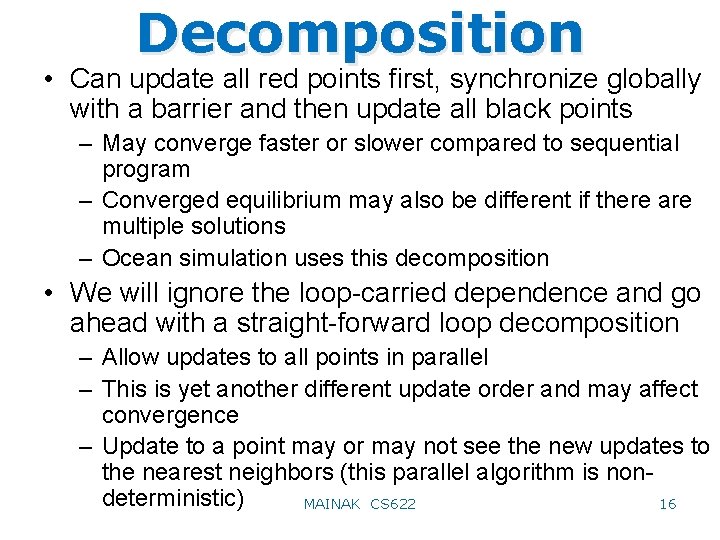
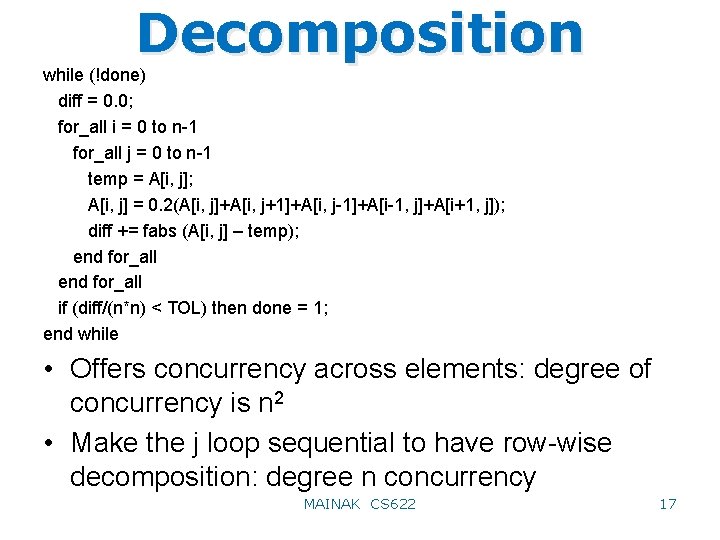
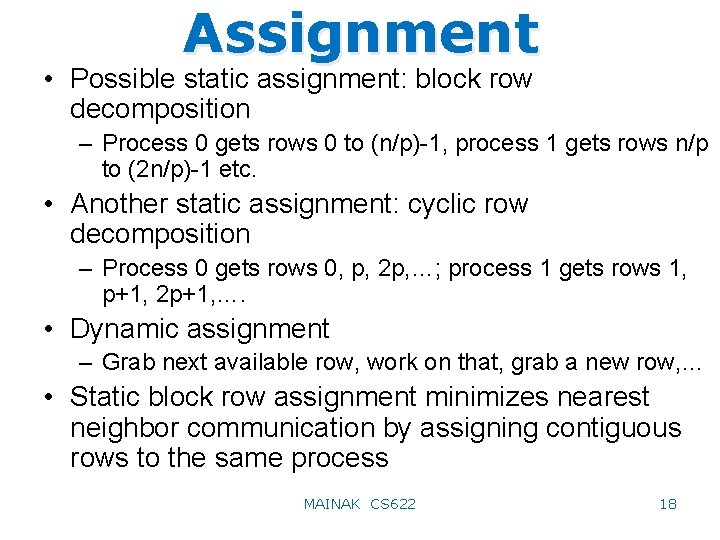
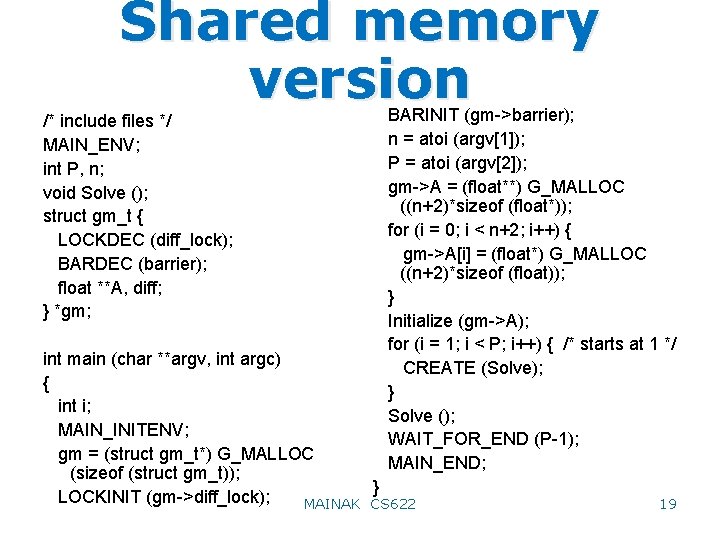
![Shared memory version local_diff += fabs (gm->A[i] [j] – void Solve (void) temp); { Shared memory version local_diff += fabs (gm->A[i] [j] – void Solve (void) temp); {](https://slidetodoc.com/presentation_image_h2/22670296ad10c718c04c034be8a4b63f/image-20.jpg)
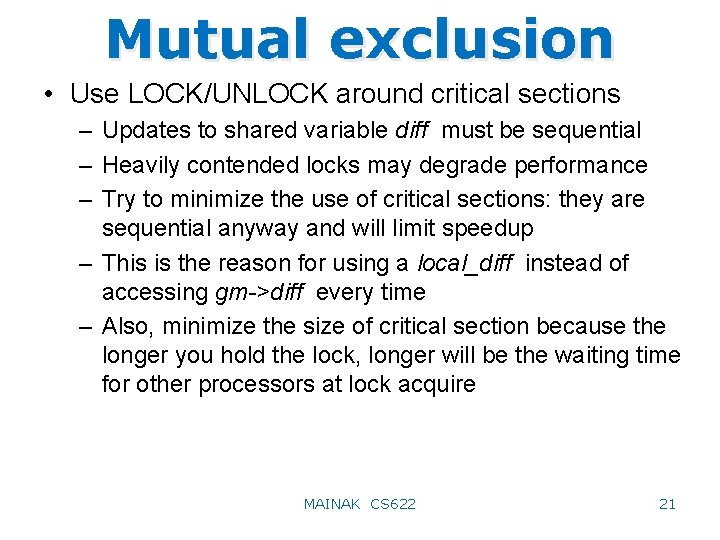
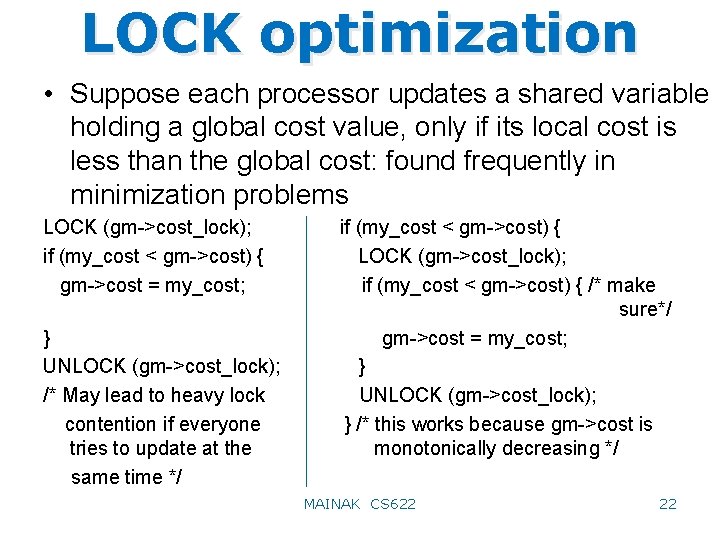
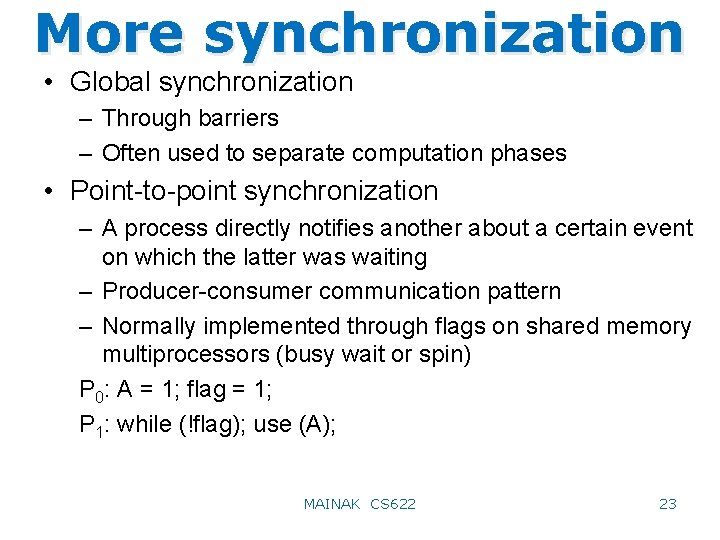
- Slides: 23
![Parallel Programming From Chapter 2 of Culler Singh Gupta Parallel Programming [From Chapter 2 of Culler, Singh, Gupta]](https://slidetodoc.com/presentation_image_h2/22670296ad10c718c04c034be8a4b63f/image-1.jpg)
Parallel Programming [From Chapter 2 of Culler, Singh, Gupta]
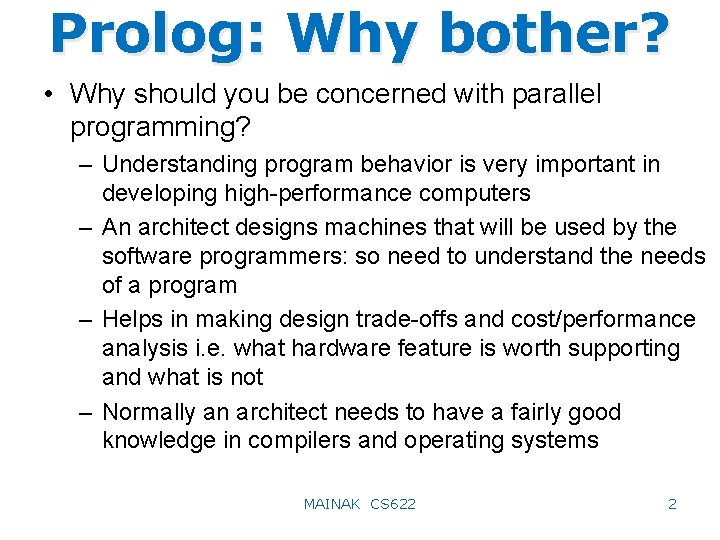
Prolog: Why bother? • Why should you be concerned with parallel programming? – Understanding program behavior is very important in developing high-performance computers – An architect designs machines that will be used by the software programmers: so need to understand the needs of a program – Helps in making design trade-offs and cost/performance analysis i. e. what hardware feature is worth supporting and what is not – Normally an architect needs to have a fairly good knowledge in compilers and operating systems MAINAK CS 622 2
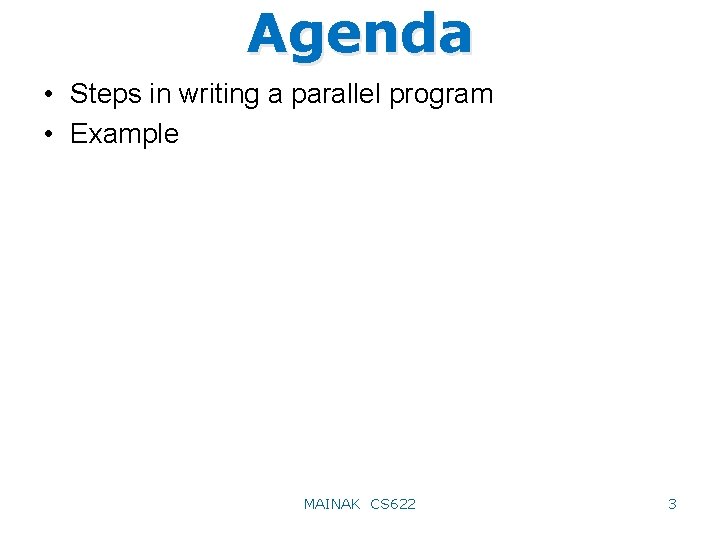
Agenda • Steps in writing a parallel program • Example MAINAK CS 622 3
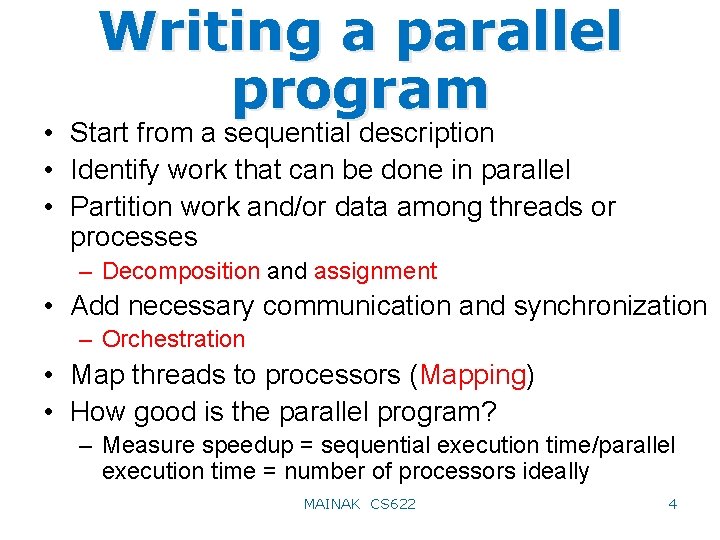
Writing a parallel program • Start from a sequential description • Identify work that can be done in parallel • Partition work and/or data among threads or processes – Decomposition and assignment • Add necessary communication and synchronization – Orchestration • Map threads to processors (Mapping) • How good is the parallel program? – Measure speedup = sequential execution time/parallel execution time = number of processors ideally MAINAK CS 622 4
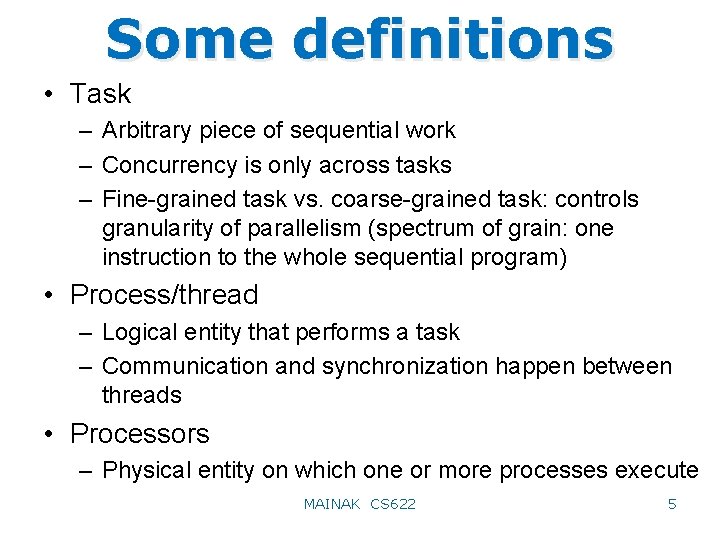
Some definitions • Task – Arbitrary piece of sequential work – Concurrency is only across tasks – Fine-grained task vs. coarse-grained task: controls granularity of parallelism (spectrum of grain: one instruction to the whole sequential program) • Process/thread – Logical entity that performs a task – Communication and synchronization happen between threads • Processors – Physical entity on which one or more processes execute MAINAK CS 622 5
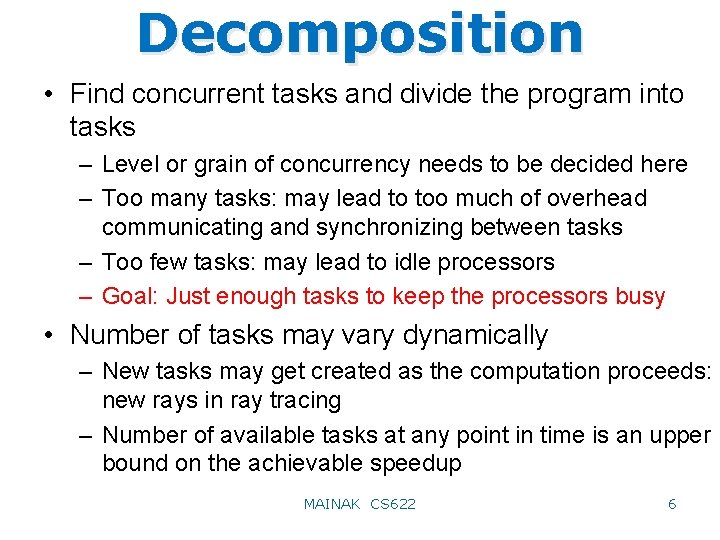
Decomposition • Find concurrent tasks and divide the program into tasks – Level or grain of concurrency needs to be decided here – Too many tasks: may lead to too much of overhead communicating and synchronizing between tasks – Too few tasks: may lead to idle processors – Goal: Just enough tasks to keep the processors busy • Number of tasks may vary dynamically – New tasks may get created as the computation proceeds: new rays in ray tracing – Number of available tasks at any point in time is an upper bound on the achievable speedup MAINAK CS 622 6
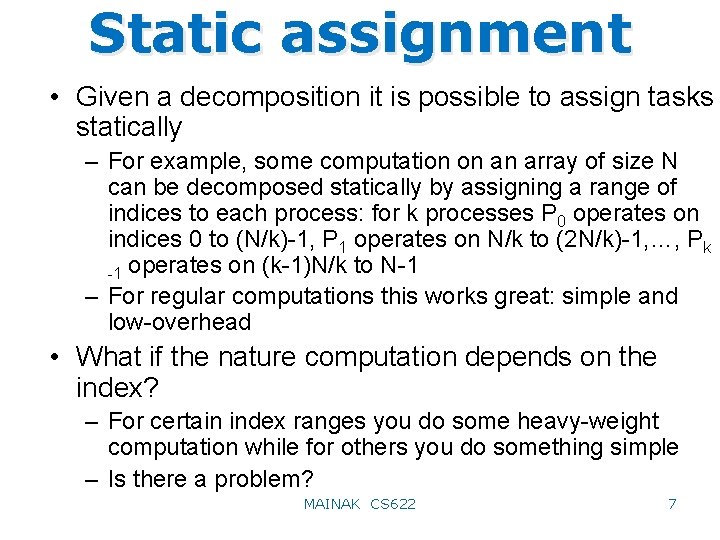
Static assignment • Given a decomposition it is possible to assign tasks statically – For example, some computation on an array of size N can be decomposed statically by assigning a range of indices to each process: for k processes P 0 operates on indices 0 to (N/k)-1, P 1 operates on N/k to (2 N/k)-1, …, Pk -1 operates on (k-1)N/k to N-1 – For regular computations this works great: simple and low-overhead • What if the nature computation depends on the index? – For certain index ranges you do some heavy-weight computation while for others you do something simple – Is there a problem? MAINAK CS 622 7
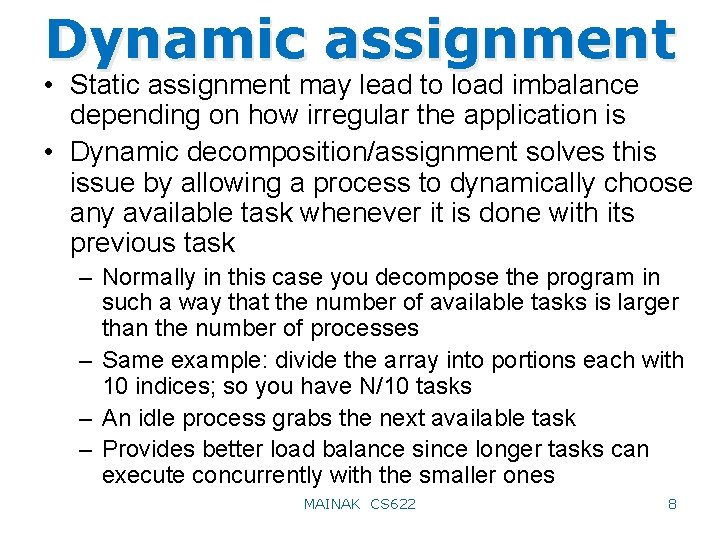
Dynamic assignment • Static assignment may lead to load imbalance depending on how irregular the application is • Dynamic decomposition/assignment solves this issue by allowing a process to dynamically choose any available task whenever it is done with its previous task – Normally in this case you decompose the program in such a way that the number of available tasks is larger than the number of processes – Same example: divide the array into portions each with 10 indices; so you have N/10 tasks – An idle process grabs the next available task – Provides better load balance since longer tasks can execute concurrently with the smaller ones MAINAK CS 622 8
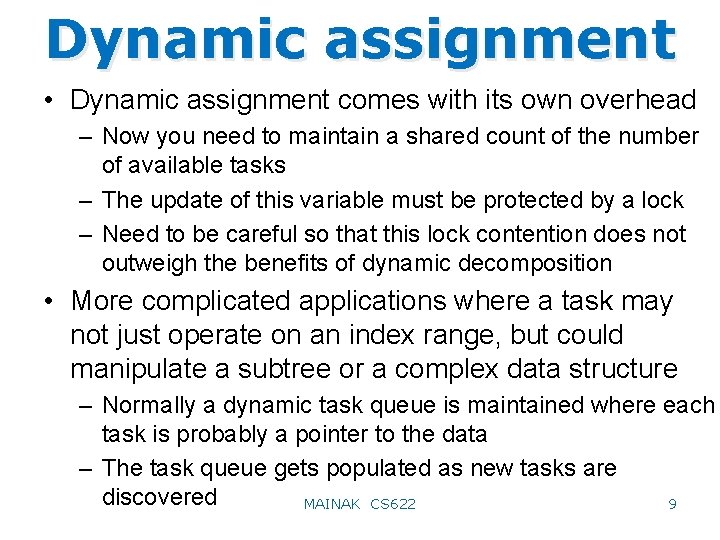
Dynamic assignment • Dynamic assignment comes with its own overhead – Now you need to maintain a shared count of the number of available tasks – The update of this variable must be protected by a lock – Need to be careful so that this lock contention does not outweigh the benefits of dynamic decomposition • More complicated applications where a task may not just operate on an index range, but could manipulate a subtree or a complex data structure – Normally a dynamic task queue is maintained where each task is probably a pointer to the data – The task queue gets populated as new tasks are discovered 9 MAINAK CS 622
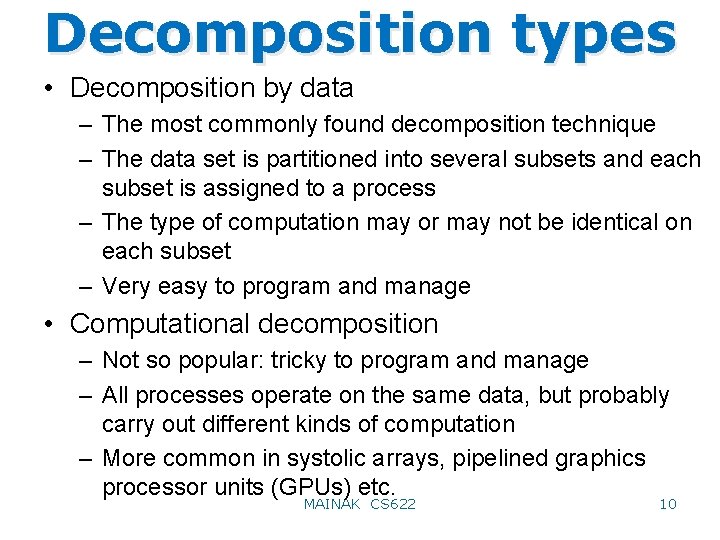
Decomposition types • Decomposition by data – The most commonly found decomposition technique – The data set is partitioned into several subsets and each subset is assigned to a process – The type of computation may or may not be identical on each subset – Very easy to program and manage • Computational decomposition – Not so popular: tricky to program and manage – All processes operate on the same data, but probably carry out different kinds of computation – More common in systolic arrays, pipelined graphics processor units (GPUs) etc. MAINAK CS 622 10
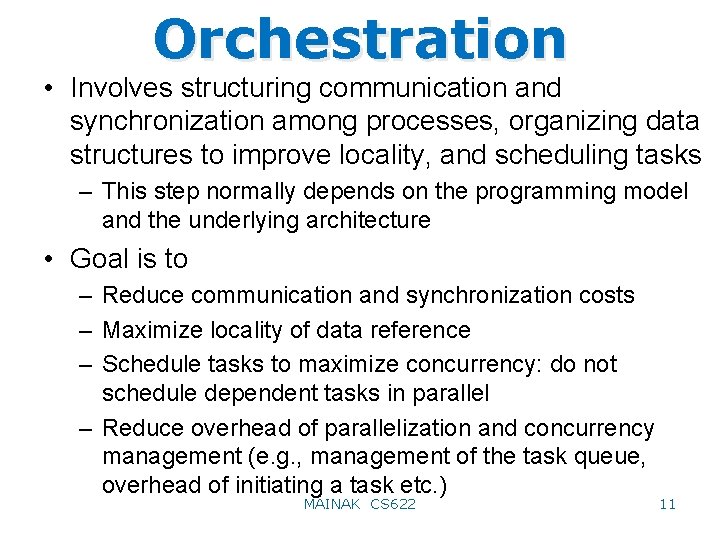
Orchestration • Involves structuring communication and synchronization among processes, organizing data structures to improve locality, and scheduling tasks – This step normally depends on the programming model and the underlying architecture • Goal is to – Reduce communication and synchronization costs – Maximize locality of data reference – Schedule tasks to maximize concurrency: do not schedule dependent tasks in parallel – Reduce overhead of parallelization and concurrency management (e. g. , management of the task queue, overhead of initiating a task etc. ) MAINAK CS 622 11
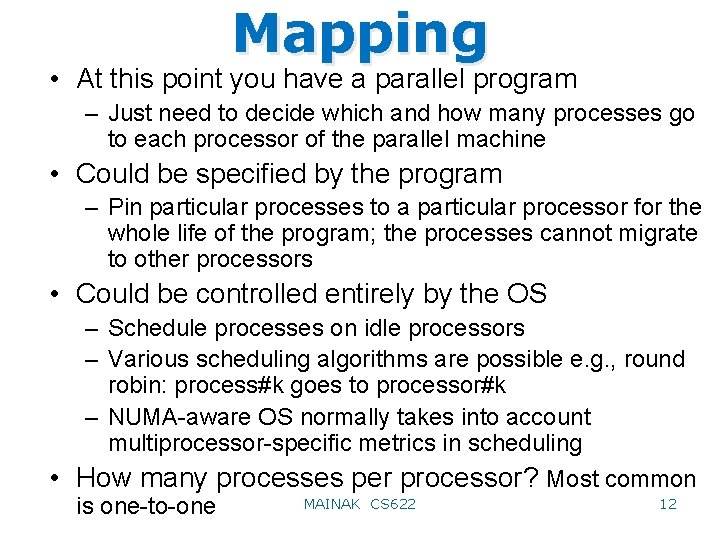
Mapping • At this point you have a parallel program – Just need to decide which and how many processes go to each processor of the parallel machine • Could be specified by the program – Pin particular processes to a particular processor for the whole life of the program; the processes cannot migrate to other processors • Could be controlled entirely by the OS – Schedule processes on idle processors – Various scheduling algorithms are possible e. g. , round robin: process#k goes to processor#k – NUMA-aware OS normally takes into account multiprocessor-specific metrics in scheduling • How many processes per processor? Most common 12 MAINAK CS 622 is one-to-one
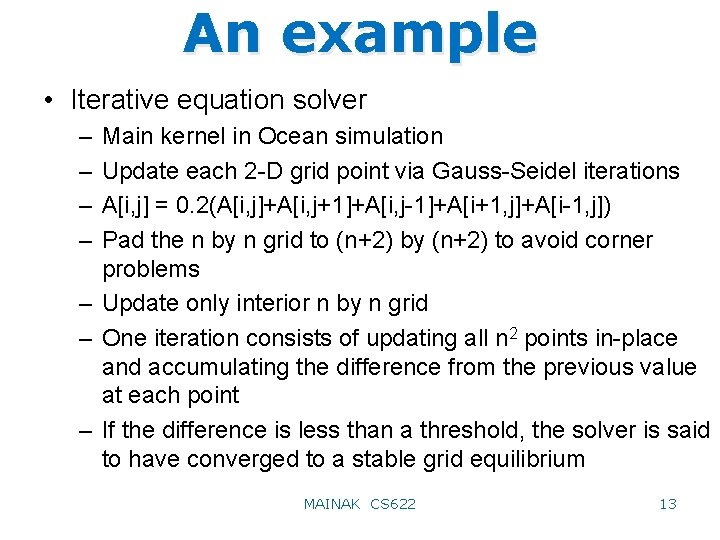
An example • Iterative equation solver – – Main kernel in Ocean simulation Update each 2 -D grid point via Gauss-Seidel iterations A[i, j] = 0. 2(A[i, j]+A[i, j+1]+A[i, j-1]+A[i+1, j]+A[i-1, j]) Pad the n by n grid to (n+2) by (n+2) to avoid corner problems – Update only interior n by n grid – One iteration consists of updating all n 2 points in-place and accumulating the difference from the previous value at each point – If the difference is less than a threshold, the solver is said to have converged to a stable grid equilibrium MAINAK CS 622 13
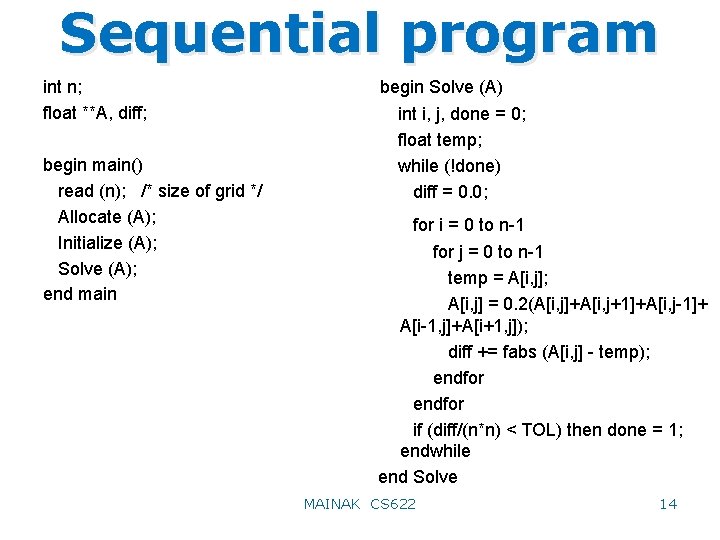
Sequential program int n; float **A, diff; begin main() read (n); /* size of grid */ Allocate (A); Initialize (A); Solve (A); end main begin Solve (A) int i, j, done = 0; float temp; while (!done) diff = 0. 0; for i = 0 to n-1 for j = 0 to n-1 temp = A[i, j]; A[i, j] = 0. 2(A[i, j]+A[i, j+1]+A[i, j-1]+ A[i-1, j]+A[i+1, j]); diff += fabs (A[i, j] - temp); endfor if (diff/(n*n) < TOL) then done = 1; endwhile end Solve MAINAK CS 622 14
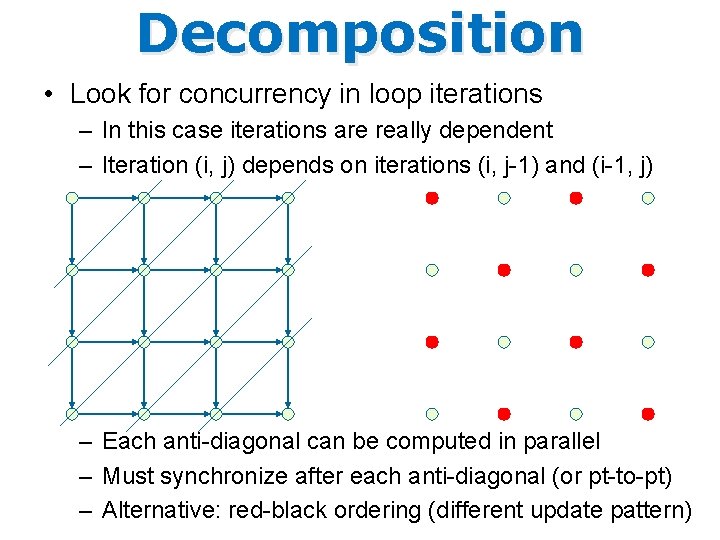
Decomposition • Look for concurrency in loop iterations – In this case iterations are really dependent – Iteration (i, j) depends on iterations (i, j-1) and (i-1, j) – Each anti-diagonal can be computed in parallel – Must synchronize after each anti-diagonal (or pt-to-pt) – Alternative: red-black ordering (different update pattern)
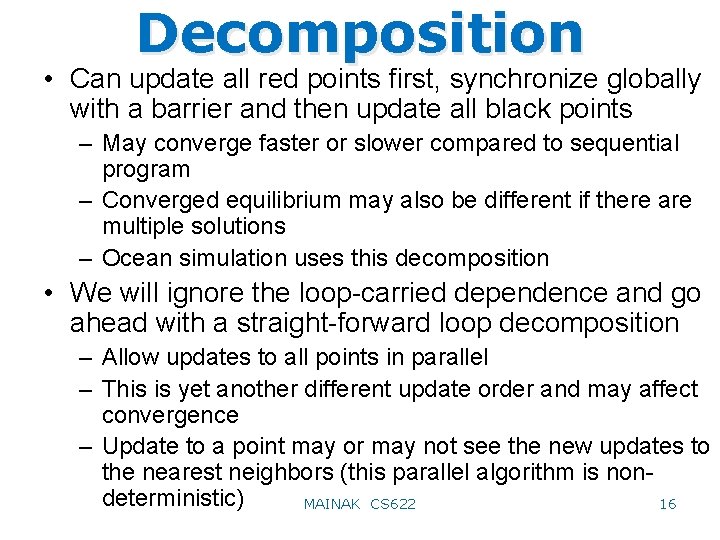
Decomposition • Can update all red points first, synchronize globally with a barrier and then update all black points – May converge faster or slower compared to sequential program – Converged equilibrium may also be different if there are multiple solutions – Ocean simulation uses this decomposition • We will ignore the loop-carried dependence and go ahead with a straight-forward loop decomposition – Allow updates to all points in parallel – This is yet another different update order and may affect convergence – Update to a point may or may not see the new updates to the nearest neighbors (this parallel algorithm is nondeterministic) 16 MAINAK CS 622
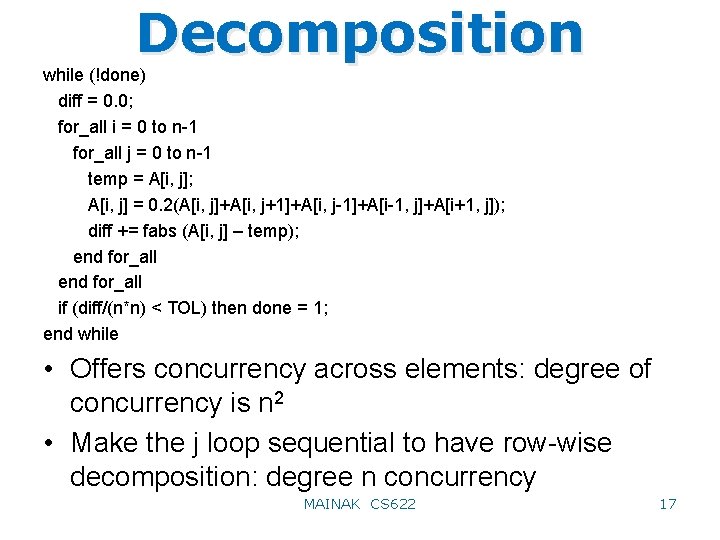
Decomposition while (!done) diff = 0. 0; for_all i = 0 to n-1 for_all j = 0 to n-1 temp = A[i, j]; A[i, j] = 0. 2(A[i, j]+A[i, j+1]+A[i, j-1]+A[i-1, j]+A[i+1, j]); diff += fabs (A[i, j] – temp); end for_all if (diff/(n*n) < TOL) then done = 1; end while • Offers concurrency across elements: degree of concurrency is n 2 • Make the j loop sequential to have row-wise decomposition: degree n concurrency MAINAK CS 622 17
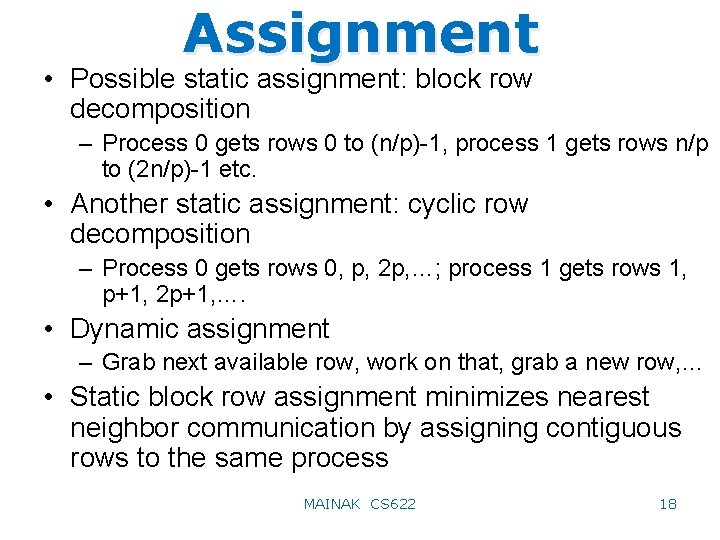
Assignment • Possible static assignment: block row decomposition – Process 0 gets rows 0 to (n/p)-1, process 1 gets rows n/p to (2 n/p)-1 etc. • Another static assignment: cyclic row decomposition – Process 0 gets rows 0, p, 2 p, …; process 1 gets rows 1, p+1, 2 p+1, …. • Dynamic assignment – Grab next available row, work on that, grab a new row, … • Static block row assignment minimizes nearest neighbor communication by assigning contiguous rows to the same process MAINAK CS 622 18
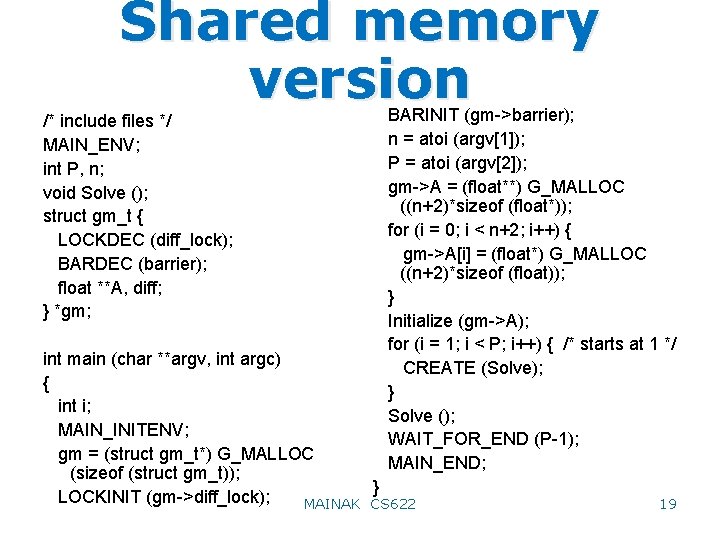
Shared memory version /* include files */ MAIN_ENV; int P, n; void Solve (); struct gm_t { LOCKDEC (diff_lock); BARDEC (barrier); float **A, diff; } *gm; BARINIT (gm->barrier); n = atoi (argv[1]); P = atoi (argv[2]); gm->A = (float**) G_MALLOC ((n+2)*sizeof (float*)); for (i = 0; i < n+2; i++) { gm->A[i] = (float*) G_MALLOC ((n+2)*sizeof (float)); } Initialize (gm->A); for (i = 1; i < P; i++) { /* starts at 1 */ CREATE (Solve); } Solve (); WAIT_FOR_END (P-1); MAIN_END; int main (char **argv, int argc) { int i; MAIN_INITENV; gm = (struct gm_t*) G_MALLOC (sizeof (struct gm_t)); } LOCKINIT (gm->diff_lock); MAINAK CS 622 19
![Shared memory version localdiff fabs gmAi j void Solve void temp Shared memory version local_diff += fabs (gm->A[i] [j] – void Solve (void) temp); {](https://slidetodoc.com/presentation_image_h2/22670296ad10c718c04c034be8a4b63f/image-20.jpg)
Shared memory version local_diff += fabs (gm->A[i] [j] – void Solve (void) temp); { } /* end for */ int i, j, pid, done = 0; } /* end for */ float temp, local_diff; LOCK (gm->diff_lock); GET_PID (pid); gm->diff += local_diff; while (!done) { UNLOCK (gm->diff_lock); local_diff = 0. 0; BARRIER (gm->barrier, P); if (!pid) gm->diff = 0. 0; if (gm->diff/(n*n) < TOL) done = 1; BARRIER (gm->barrier, P); /*why? */ BARRIER (gm->barrier, P); /* why? */ for (i = pid*(n/P); i < (pid+1)*(n/P); } /* end while */ i++) { } for (j = 0; j < n; j++) { temp = gm->A[i] [j]; gm->A[i] [j] = 0. 2*(gm->A[i] [j] + gm->A[i] [j-1] + gm->A[i] [j+1] + gm>A[i+1] [j] + gm->A[i-1] [j]); MAINAK CS 622 20
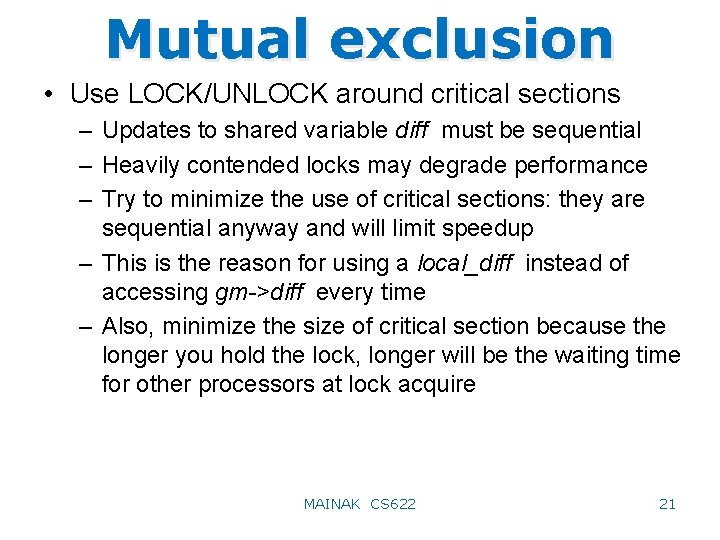
Mutual exclusion • Use LOCK/UNLOCK around critical sections – Updates to shared variable diff must be sequential – Heavily contended locks may degrade performance – Try to minimize the use of critical sections: they are sequential anyway and will limit speedup – This is the reason for using a local_diff instead of accessing gm->diff every time – Also, minimize the size of critical section because the longer you hold the lock, longer will be the waiting time for other processors at lock acquire MAINAK CS 622 21
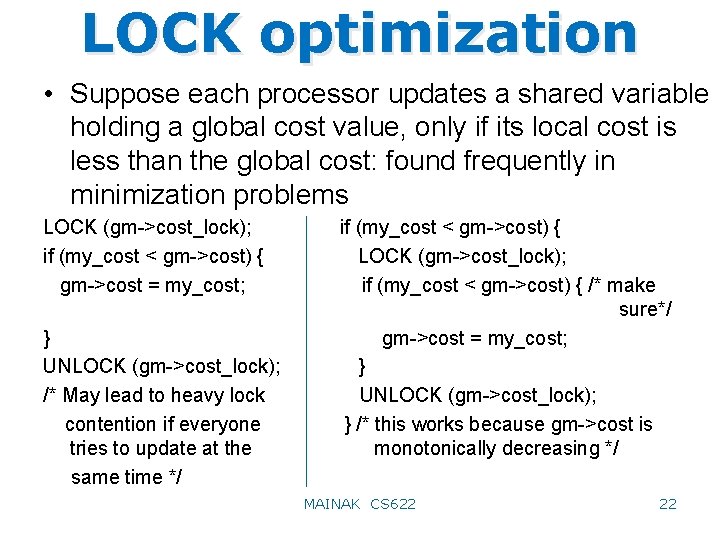
LOCK optimization • Suppose each processor updates a shared variable holding a global cost value, only if its local cost is less than the global cost: found frequently in minimization problems LOCK (gm->cost_lock); if (my_cost < gm->cost) { gm->cost = my_cost; } UNLOCK (gm->cost_lock); /* May lead to heavy lock contention if everyone tries to update at the same time */ if (my_cost < gm->cost) { LOCK (gm->cost_lock); if (my_cost < gm->cost) { /* make sure*/ gm->cost = my_cost; } UNLOCK (gm->cost_lock); } /* this works because gm->cost is monotonically decreasing */ MAINAK CS 622 22
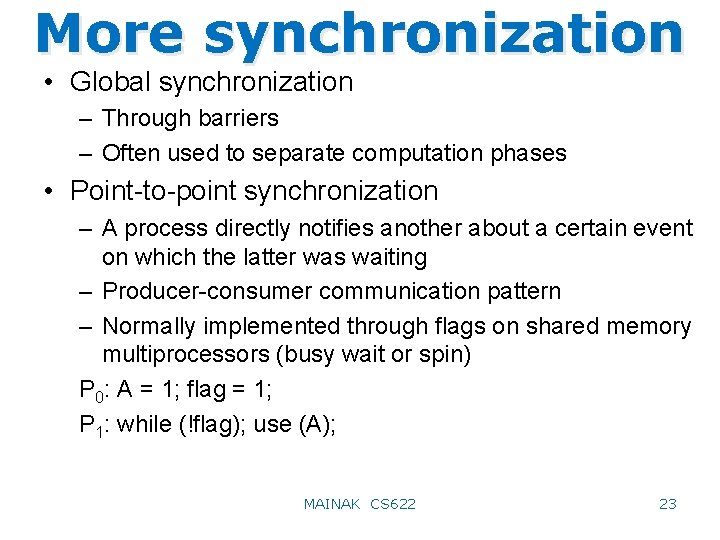
More synchronization • Global synchronization – Through barriers – Often used to separate computation phases • Point-to-point synchronization – A process directly notifies another about a certain event on which the latter was waiting – Producer-consumer communication pattern – Normally implemented through flags on shared memory multiprocessors (busy wait or spin) P 0: A = 1; flag = 1; P 1: while (!flag); use (A); MAINAK CS 622 23
David e. culler
Cullers meaning
Sarwan singh kundan singh
Perbedaan linear programming dan integer programming
Greedy programming vs dynamic programming
Definition of system programming
Integer programming vs linear programming
Definisi linear
Programming massively parallel processors
Parallel programming patterns
What is parallel programming in java
An introduction to parallel programming peter pacheco
Bubble sort parallel programming
Mpi parallel programming in c
Programming massively parallel processors
David kirk nvidia
Parallel programming platforms
F# parallel programming
Parallel programming
Programming massively parallel processors, kirk et al.
Define like and unlike parallel forces with examples
The inner terminus of the fingerprint pattern
Non parallel sentence
Grammatically parallel