Modularized Transcriptional Regulatory Networks of Pathogenic Fungus Fusarium
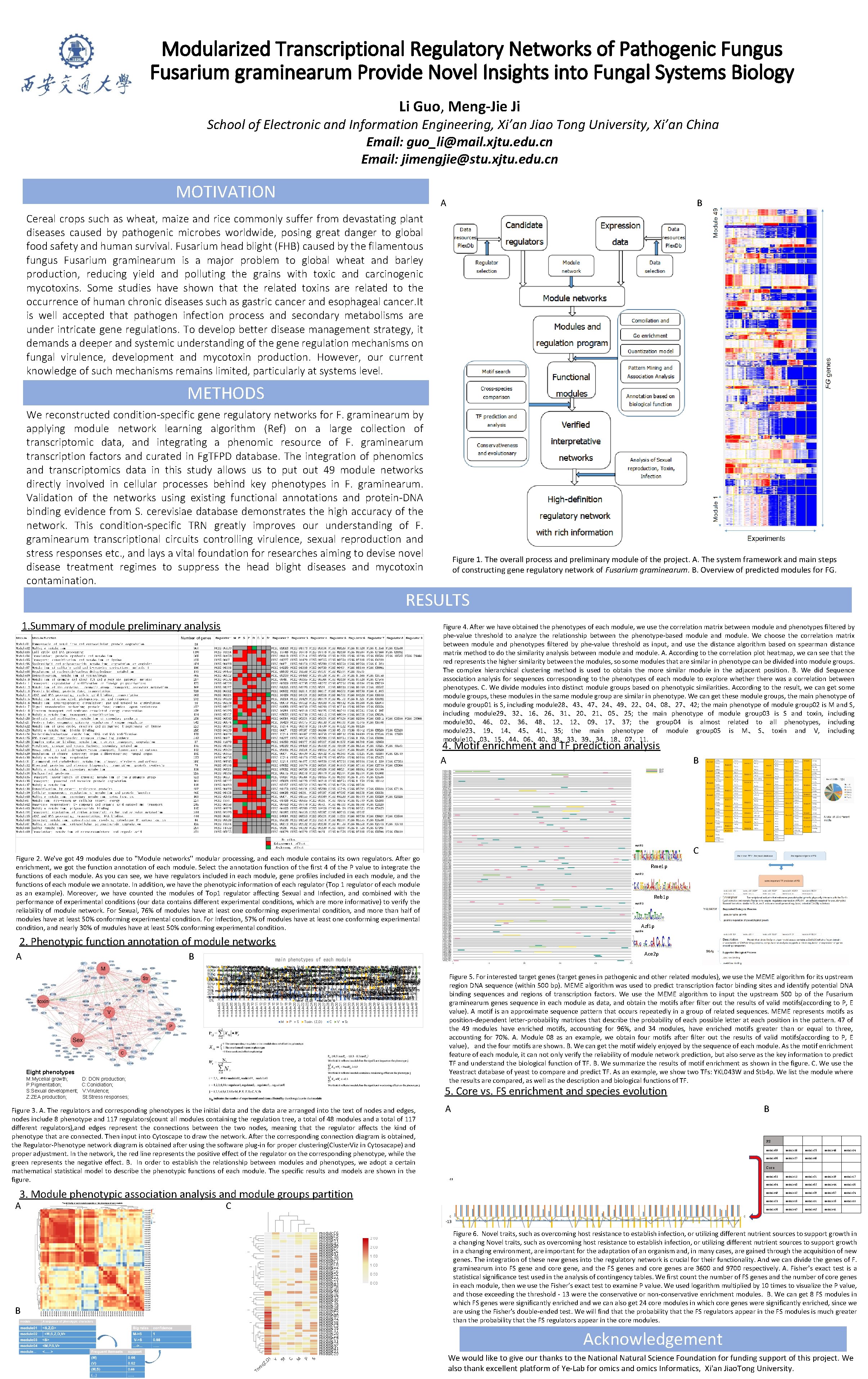
- Slides: 1
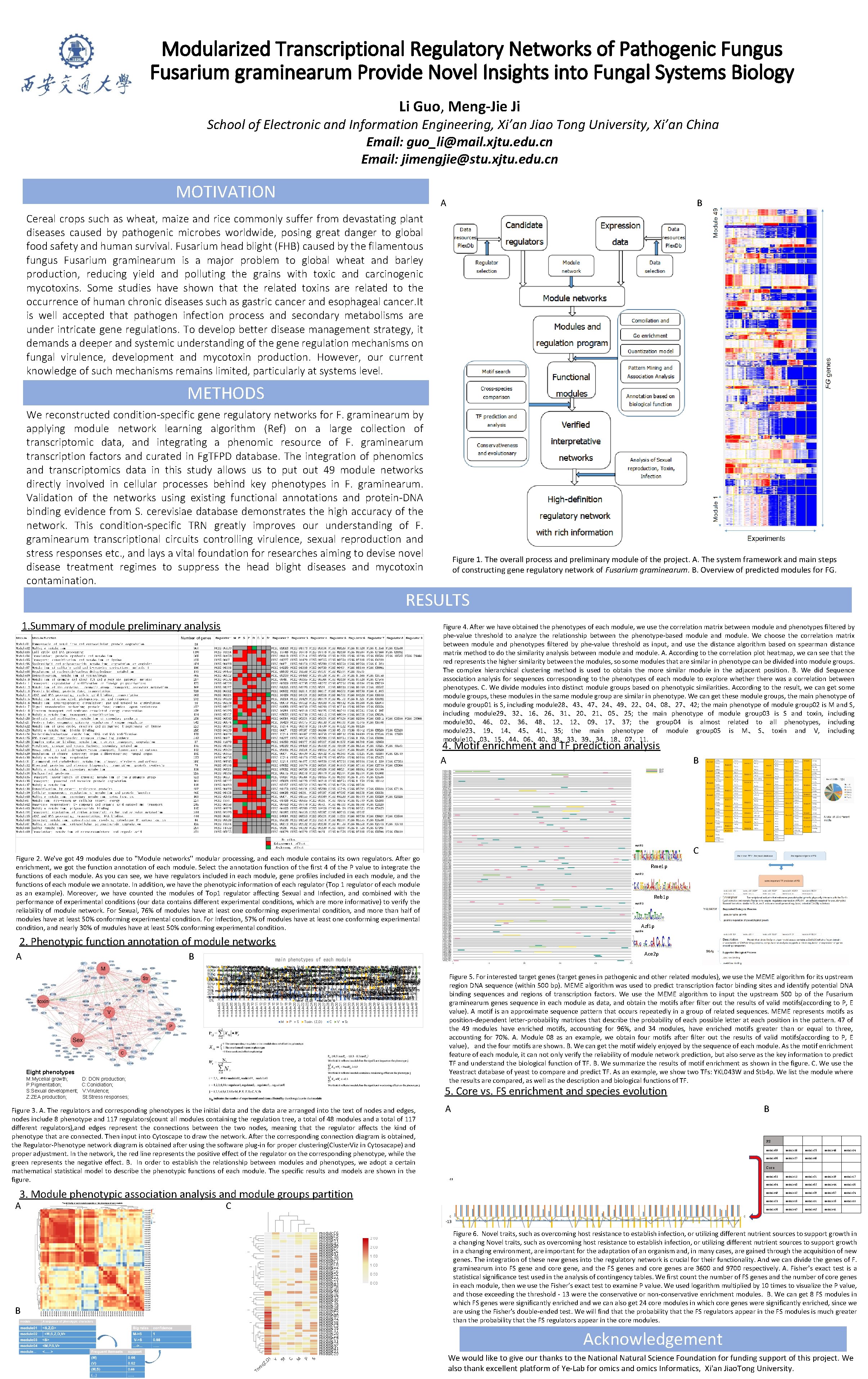
Modularized Transcriptional Regulatory Networks of Pathogenic Fungus Fusarium graminearum Provide Novel Insights into Fungal Systems Biology Li Guo, Meng-Jie Ji School of Electronic and Information Engineering, Xi’an Jiao Tong University, Xi’an China Email: guo_li@mail. xjtu. edu. cn Email: jimengjie@stu. xjtu. edu. cn MOTIVATION B A Cereal crops such as wheat, maize and rice commonly suffer from devastating plant diseases caused by pathogenic microbes worldwide, posing great danger to global food safety and human survival. Fusarium head blight (FHB) caused by the filamentous fungus Fusarium graminearum is a major problem to global wheat and barley production, reducing yield and polluting the grains with toxic and carcinogenic mycotoxins. Some studies have shown that the related toxins are related to the occurrence of human chronic diseases such as gastric cancer and esophageal cancer. It is well accepted that pathogen infection process and secondary metabolisms are under intricate gene regulations. To develop better disease management strategy, it demands a deeper and systemic understanding of the gene regulation mechanisms on fungal virulence, development and mycotoxin production. However, our current knowledge of such mechanisms remains limited, particularly at systems level. METHODS We reconstructed condition-specific gene regulatory networks for F. graminearum by applying module network learning algorithm (Ref) on a large collection of transcriptomic data, and integrating a phenomic resource of F. graminearum transcription factors and curated in Fg. TFPD database. The integration of phenomics and transcriptomics data in this study allows us to put out 49 module networks directly involved in cellular processes behind key phenotypes in F. graminearum. Validation of the networks using existing functional annotations and protein-DNA binding evidence from S. cerevisiae database demonstrates the high accuracy of the network. This condition-specific TRN greatly improves our understanding of F. graminearum transcriptional circuits controlling virulence, sexual reproduction and stress responses etc. , and lays a vital foundation for researches aiming to devise novel disease treatment regimes to suppress the head blight diseases and mycotoxin contamination. Figure 1. The overall process and preliminary module of the project. A. The system framework and main steps of constructing gene regulatory network of Fusarium graminearum. B. Overview of predicted modules for FG. RESULTS 1. Summary of module preliminary analysis Figure 4. After we have obtained the phenotypes of each module, we use the correlation matrix between module and phenotypes filtered by phe-value threshold to analyze the relationship between the phenotype-based module and module. We choose the correlation matrix between module and phenotypes filtered by phe-value threshold as input, and use the distance algorithm based on spearman distance matrix method to do the similarity analysis between module and module. A. According to the correlation plot heatmap, we can see that the red represents the higher similarity between the modules, so some modules that are similar in phenotype can be divided into module groups. The complex hierarchical clustering method is used to obtain the more similar module in the adjacent position. B. We did Sequence association analysis for sequences corresponding to the phenotypes of each module to explore whethere was a correlation between phenotypes. C. We divide modules into distinct module groups based on phenotypic similarities. According to the result, we can get some module groups, these modules in the same module group are similar in phenotype. We can get these module groups, the main phenotype of module group 01 is S, including module 28、43、47、24、49、22、04、08、27、42; the main phenotype of module group 02 is M and S, including module 29、 32、 16、 26、 31、 20、 21、 05、 25; the main phenotype of module group 03 is S and toxin, including module 30、 46、 02、 36、 48、 12、 09、 17、 37; the group 04 is almost related to all phenotypes, including module 23、 19、 14、 45、 41、 35; the main phenotype of module group 05 is M、 S、 toxin and V, including module 10、03、15、44、06、40、38、33、39、34、18、07、11. 4. Motif enrichment and TF prediction analysis A B motif 1 Figure 2. We've got 49 modules due to ''Module networks'' modular processing, and each module contains its own regulators. After go enrichment, we got the function annotation of each module. Select the annotation function of the first 4 of the P value to integrate the functions of each module. As you can see, we have regulators included in each module, gene profiles included in each module, and the functions of each module we annotate. In addition, we have the phenotypic information of each regulator (Top 1 regulator of each module as an example). Moreover, we have counted the modules of Top 1 regulator affecting Sexual and Infection, and combined with the performance of experimental conditions (our data contains different experimental conditions, which are more informative) to verify the reliability of module network. For Sexual, 76% of modules have at least one conforming experimental condition, and more than half of modules have at least 50% conforming experimental condition. For Infection, 57% of modules have at least one conforming experimental condition, and nearly 30% of mudules have at least 50% conforming experimental condition. C Rme 1 p motif 2 Reb 1 p motif 3 Azf 1 p motif 4 2. Phenotypic function annotation of module networks A B main phenotypes of each module 02 module 03 module 04 module 05 module 06 module 07 module 08 module 09 module 10 module 11 module 12 module 13 module 14 module 15 module 16 module 17 module 18 module 19 module 20 module 21 module 22 module 23 module 24 module 25 module 26 module 27 module 28 module 29 module 30 module 31 module 32 module 33 module 34 module 35 module 36 module 37 module 38 module 39 module 40 module 41 module 42 module 43 module 44 module 45 module 46 module 47 module 48 module 49 Toxin(Z, D) module 04 module 07 0 module 08 00, 75 0 Toxin(Z, D) module 22 Toxin(Z, D) 0 module 27 module 28 Toxin(Z, D) module 30 0 0 0 module 34 Toxin(Z, D) 0 Toxin(Z, D) module 42 module 47 module 48 0 0 100% module 10 St module 45 1 St module 35 1, 1875 St 1 Vmodule 14 1, 125 Vmodule 39 module 40 0, 8125 V module 41 1, 5625 CToxin(Z, D) 1 Vmodule 19 1, 75 St. St V module 05 Vmodule 17 module 18 1, 5 module 15 1, 6875 90%V module 03 St module 21 1, 375 module 23 1, 6875 St module 26 1 V 1 module 09 module 10 0, 75 V 1, 25 V module 29 0, 875 V module 43 1 V module 25 1 Toxin(Z, D) module 13 V module 44 1, 5 V module 38 1, 3125 Toxin(Z, D) module 37 module 20 1, 25 V module 16 module 32 St. V module 31 10, 75 1, 375 module 45 0, 875 1 V module 35 VV module 49 module 11 V module 14 1, 25 St 1, 75 80%C module 03 0, 75 Toxin(Z, D) 1, 0625 C module 41 1, 0625 Toxin(Z, D) module 26 Toxin(Z, D) module 17 Toxin(Z, D) module 25 0 0 Vmodule 05 module 06 1, 5 0, 875 C 1 module 10 1, 875 C module 23 0, 75 2, 5 C C module 45 1, 0625 V module 21 1 70% S module 42 1, 1875 Toxin(Z, D) module 33 Toxin(Z, D) module 12 Toxin(Z, D) module 18 1, 75 module 16 0 module 21 Toxin(Z, D) module 15 01, 625 C 1 Toxin(Z, D) module 14 1, 25 C module 32 module 35 C module 09 Toxin(Z, D) module 02 module 31 0 Toxin(Z, D) module 40 Toxin(Z, D) 0 C Toxin(Z, D) module 29 0, 875 S module 25 1 Toxin(Z, D) module 41 Toxin(Z, D) module 05 0 Toxin(Z, D) module 03 Toxin(Z, D) module 23 module 49 20 Toxin(Z, D) module 39 module 14 60% Toxin(Z, D) module 38 P 1, 5 module 19 1, 125 Toxin(Z, D) module 10 Toxin(Z, D) module 20 Toxin(Z, D) module 44 S module 26 1, 9375 module 29 CToxin(Z, D) module 43 1, 5625 Toxin(Z, D) module 36 Toxin(Z, D) module 462 2, 625 2, 5 3, 0625 S module 21 1, 125 Toxin(Z, D) module 35 3, 375 Toxin(Z, D) module 45 3, 5 S module 05 1 Toxin(Z, D) 0 2, 875 S module 31 0, 9375 3, 125 Toxin(Z, D) SToxin(Z, D) 1, 4375 module 08 module 06 1, 8125 0 S module 16 1, 9375 S module 22 V module 24 1, 8125 S S module 27 1, 3125 module 28 S module 30 1, 875 1, 4375 S 1, 9375 module 34 1, 9375 S module 47 module 48 1, 9375 1, 3125 50% S module 04 3, 5 S module 07 2, 125 2, 25 S module 37 1, 4375 1, 25 1, 875 module 09 S module 17 1, 625 2, 5 1, 5 2, 5 0, 8125 2, 25 40% S module 14 1, 3125 P module 21 1, 125 P module 25 1 Toxin(Z, D) module 43 0 S module 06 0, 75 S module 45 1, 125 M module 32 1, 5625 1, 375 P module 05 1, 5625 S module 41 S module 15 1, 6875 1, 9375 S module 23 1, 6875 S module 13 1, 4375 S 1, 5625 module 49 1, 4375 S module 35 S module 18 30% S module 44 1, 25 S Smodule 10 S module 03 1, 81251, 125 S module 29 1, 875 P 1, 75 module 14 1, 25 S module 38 0, 8125 module 11 1, 6875 P module 37 1, 5 S module 40 0, 8125 M module 19 1, 6875 module 45 20% MSmodule 42 0, 75 module 43 1, 5625 1, 75 module 35 S 1, 125 M module 26 1, 625 M module 31 P 1, 0625 P module 41 module 39 1, 75 P 1, 25 M module 20 1, 3125 M 0, 8125 1, 75 M module 21 1, 9375 Pmodule 16 module 17 1, 625 M module 25 module 06 0, 75 0, 875 S module 12 MM module 05 1, 25 module 15 1, 9375 module 09 M 1, 5 module 23 1, 68751, 0625 M module 33 SM module 02 0, 9375 10% module 10 1, 875 module 03 1, 875 PM M 1 M module 14 M 1, 75 module 18 M 0, 8125 module 44 1, 06251 module 38 0, 8125 M module 40 M module 35 1, 375 MMmodule 37 0, 75 M module 41 1, 0625 Toxin(Z, D) module 24 0 0% M P S Toxin(Z, D) C V St Eight phenotypes M: Mycelial growth; P: Pigmentation; S: Sexual development; Z: ZEA production; Ace 2 p D: DON production; C: Conidiation; V: Virulence; St: Stress responses; Figure 5. For interested target genes (target genes in pathogenic and other related modules), we use the MEME algorithm for its upstream region DNA sequence (within 500 bp). MEME algorithm was used to predict transcription factor binding sites and identify potential DNA binding sequences and regions of transcription factors. We use the MEME algorithm to input the upstream 500 bp of the Fusarium graminearum genes sequence in each module as data, and obtain the motifs after filter out the results of valid motifs(according to P, E value). A motif is an approximate sequence pattern that occurs repeatedly in a group of related sequences. MEME represents motifs as position-dependent letter-probability matrices that describe the probability of each possible letter at each position in the pattern. 47 of the 49 modules have enriched motifs, accounting for 96%, and 34 modules, have enriched motifs greater than or equal to three, accounting for 70%. A. Module 08 as an example, we obtain four motifs after filter out the results of valid motifs(according to P, E value),and the four motifs are shown. B. We can get the motif widely enjoyed by the sequence of each module. As the motif enrichment feature of each module, it can not only verify the reliability of module network prediction, but also serve as the key information to predict TF and understand the biological function of TF. B. We summarize the results of motif enrichment as shown in the figure. C. We use the Yeastract database of yeast to compare and predict TF. As an example, we show two TFs: YKL 043 W and Stb 4 p. We list the module where the results are compared, as well as the description and biological functions of TF. 5. Core vs. FS enrichment and species evolution Figure 3. A. The regulators and corresponding phenotypes is the initial data and the data are arranged into the text of nodes and edges, nodes include 8 phenotype and 117 regulators(count all modules containing the regulation tree, a total of 48 modules and a total of 117 different regulators), and edges represent the connections between the two nodes, meaning that the regulator affects the kind of phenotype that are connected. Then input into Cytoscape to draw the network. After the corresponding connection diagram is obtained, the Regulator-Phenotype network diagram is obtained after using the software plug-in for proper clustering(Cluster. Viz in Cytosacape) and proper adjustment. In the network, the red line represents the positive effect of the regulator on the corresponding phenotype, while the green represents the negative effect. B. In order to establish the relationship between modules and phenotypes, we adopt a certain mathematical statistical model to describe the phenotypic functions of each module. The specific results and models are shown in the figure. A B FS module 38 module 25 module 48 module 34 module 06 module 27 module 46 module 03 module 13 module 21 module 39 module 17 module 04 module 45 module 02 module 44 module 30 module 49 module 32 module 29 module 07 module 24 module 23 module 10 module 31 module 35 module 33 module 36 module 47 module 42 module 41 Core -13 3. Module phenotypic association analysis and module groups partition A module 09 C -13 B Figure 6. Novel traits, such as overcoming host resistance to establish infection, or utilizing different nutrient sources to support growth in a changing environment, are important for the adaptation of an organism and, in many cases, are gained through the acquisition of new genes. The integration of these new genes into the regulatory network is crucial for their functionality. And we can divide the genes of F. graminearum into FS gene and core gene, and the FS genes and core genes are 3600 and 9700 respectively. A. Fisher's exact test is a statistical significance test used in the analysis of contingency tables. We first count the number of FS genes and the number of core genes in each module, then we use the Fisher’s exact test to examine P value. We used logarithm multiplied by 10 times to visualize the P value, and those exceeding the threshold - 13 were the conservative or non-conservative enrichment modules. B. We can get 8 FS modules in which FS genes were significantly enriched and we can also get 24 core modules in which core genes were significantly enriched, since we are using the Fisher's double-ended test. We will find that the probability that the FS regulators appear in the FS modules is much greater than the probability that the FS regulators appear in the core modules. Acknowledgement We would like to give our thanks to the National Natural Science Foundation for funding support of this project. We also thank excellent platform of Ye-Lab for omics and omics Informatics, Xi'an Jiao. Tong University.