Logical Hypothesis Testing Eliwid Medellin James W Grice
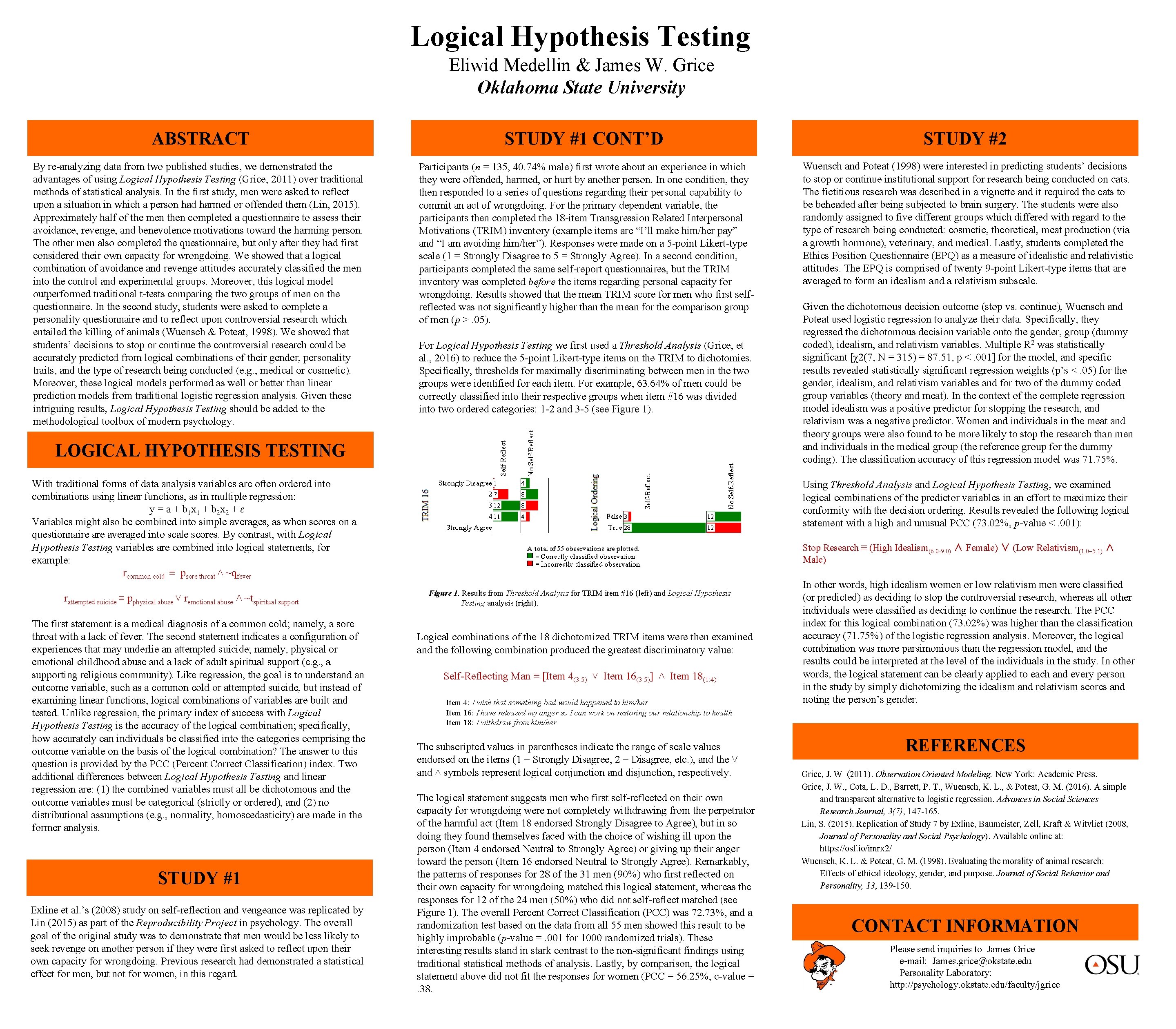
- Slides: 1
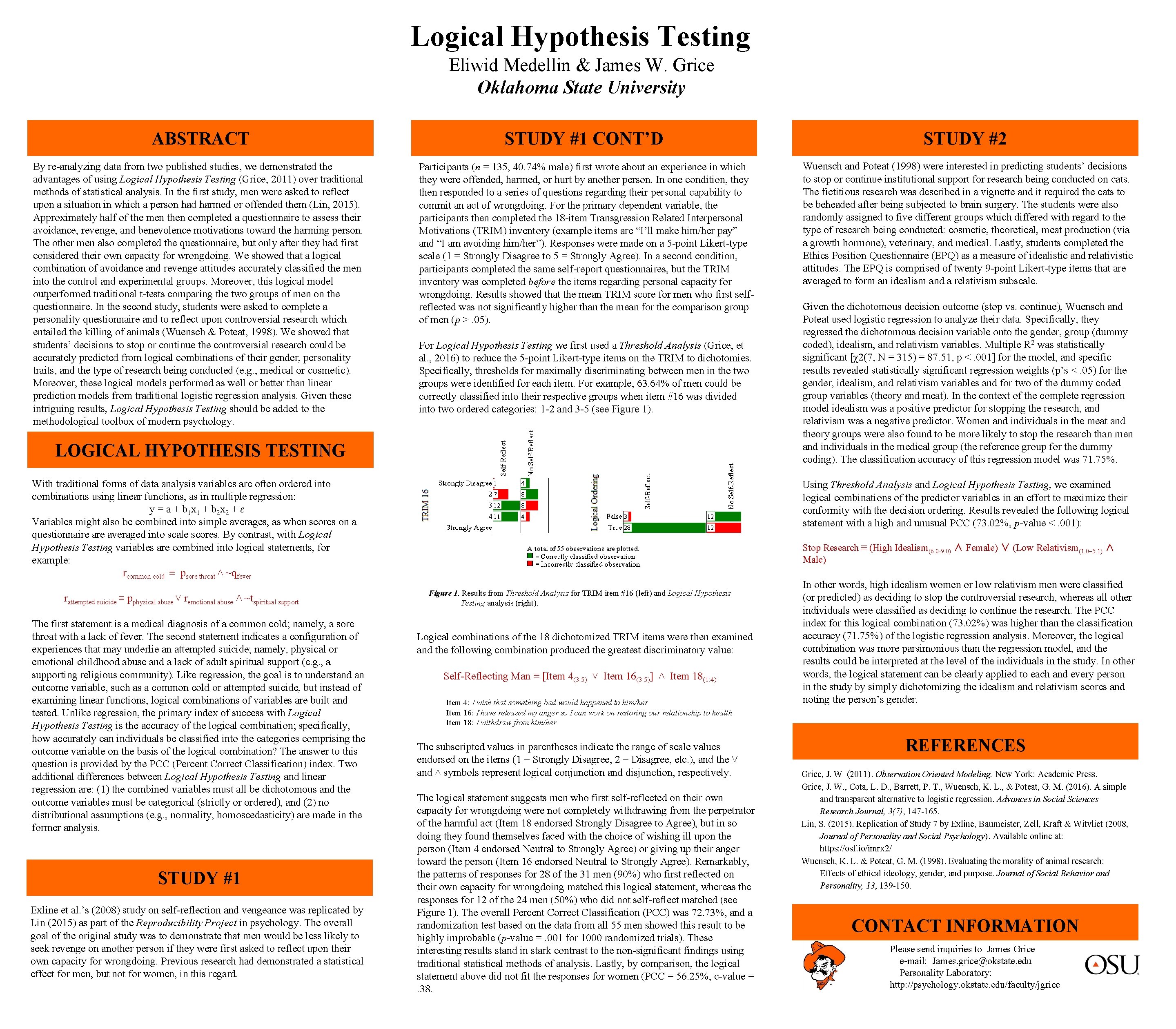
Logical Hypothesis Testing Eliwid Medellin & James W. Grice Oklahoma State University ABSTRACT STUDY #1 CONT’D STUDY #2 By re-analyzing data from two published studies, we demonstrated the advantages of using Logical Hypothesis Testing (Grice, 2011) over traditional methods of statistical analysis. In the first study, men were asked to reflect upon a situation in which a person had harmed or offended them (Lin, 2015). Approximately half of the men then completed a questionnaire to assess their avoidance, revenge, and benevolence motivations toward the harming person. The other men also completed the questionnaire, but only after they had first considered their own capacity for wrongdoing. We showed that a logical combination of avoidance and revenge attitudes accurately classified the men into the control and experimental groups. Moreover, this logical model outperformed traditional t-tests comparing the two groups of men on the questionnaire. In the second study, students were asked to complete a personality questionnaire and to reflect upon controversial research which entailed the killing of animals (Wuensch & Poteat, 1998). We showed that students’ decisions to stop or continue the controversial research could be accurately predicted from logical combinations of their gender, personality traits, and the type of research being conducted (e. g. , medical or cosmetic). Moreover, these logical models performed as well or better than linear prediction models from traditional logistic regression analysis. Given these intriguing results, Logical Hypothesis Testing should be added to the methodological toolbox of modern psychology. Participants (n = 135, 40. 74% male) first wrote about an experience in which they were offended, harmed, or hurt by another person. In one condition, they then responded to a series of questions regarding their personal capability to commit an act of wrongdoing. For the primary dependent variable, the participants then completed the 18 -item Transgression Related Interpersonal Motivations (TRIM) inventory (example items are “I’ll make him/her pay” and “I am avoiding him/her”). Responses were made on a 5 -point Likert-type scale (1 = Strongly Disagree to 5 = Strongly Agree). In a secondition, participants completed the same self-report questionnaires, but the TRIM inventory was completed before the items regarding personal capacity for wrongdoing. Results showed that the mean TRIM score for men who first selfreflected was not significantly higher than the mean for the comparison group of men (p >. 05). Wuensch and Poteat (1998) were interested in predicting students’ decisions to stop or continue institutional support for research being conducted on cats. The fictitious research was described in a vignette and it required the cats to be beheaded after being subjected to brain surgery. The students were also randomly assigned to five different groups which differed with regard to the type of research being conducted: cosmetic, theoretical, meat production (via a growth hormone), veterinary, and medical. Lastly, students completed the Ethics Position Questionnaire (EPQ) as a measure of idealistic and relativistic attitudes. The EPQ is comprised of twenty 9 -point Likert-type items that are averaged to form an idealism and a relativism subscale. For Logical Hypothesis Testing we first used a Threshold Analysis (Grice, et al. , 2016) to reduce the 5 -point Likert-type items on the TRIM to dichotomies. Specifically, thresholds for maximally discriminating between men in the two groups were identified for each item. For example, 63. 64% of men could be correctly classified into their respective groups when item #16 was divided into two ordered categories: 1 -2 and 3 -5 (see Figure 1). LOGICAL HYPOTHESIS TESTING With traditional forms of data analysis variables are often ordered into combinations using linear functions, as in multiple regression: y = a + b 1 x 1 + b 2 x 2 + ɛ Variables might also be combined into simple averages, as when scores on a questionnaire averaged into scale scores. By contrast, with Logical Hypothesis Testing variables are combined into logical statements, for example: rcommon cold ≡ psore throat ˄ ~qfever rattempted suicide ≡ pphysical abuse ˅ remotional abuse ˄ ~tspiritual support The first statement is a medical diagnosis of a common cold; namely, a sore throat with a lack of fever. The second statement indicates a configuration of experiences that may underlie an attempted suicide; namely, physical or emotional childhood abuse and a lack of adult spiritual support (e. g. , a supporting religious community). Like regression, the goal is to understand an outcome variable, such as a common cold or attempted suicide, but instead of examining linear functions, logical combinations of variables are built and tested. Unlike regression, the primary index of success with Logical Hypothesis Testing is the accuracy of the logical combination; specifically, how accurately can individuals be classified into the categories comprising the outcome variable on the basis of the logical combination? The answer to this question is provided by the PCC (Percent Correct Classification) index. Two additional differences between Logical Hypothesis Testing and linear regression are: (1) the combined variables must all be dichotomous and the outcome variables must be categorical (strictly or ordered), and (2) no distributional assumptions (e. g. , normality, homoscedasticity) are made in the former analysis. STUDY #1 Exline et al. ’s (2008) study on self-reflection and vengeance was replicated by Lin (2015) as part of the Reproducibility Project in psychology. The overall goal of the original study was to demonstrate that men would be less likely to seek revenge on another person if they were first asked to reflect upon their own capacity for wrongdoing. Previous research had demonstrated a statistical effect for men, but not for women, in this regard. Given the dichotomous decision outcome (stop vs. continue), Wuensch and Poteat used logistic regression to analyze their data. Specifically, they regressed the dichotomous decision variable onto the gender, group (dummy coded), idealism, and relativism variables. Multiple R 2 was statistically significant [χ2(7, N = 315) = 87. 51, p <. 001] for the model, and specific results revealed statistically significant regression weights (p’s <. 05) for the gender, idealism, and relativism variables and for two of the dummy coded group variables (theory and meat). In the context of the complete regression model idealism was a positive predictor for stopping the research, and relativism was a negative predictor. Women and individuals in the meat and theory groups were also found to be more likely to stop the research than men and individuals in the medical group (the reference group for the dummy coding). The classification accuracy of this regression model was 71. 75%. Using Threshold Analysis and Logical Hypothesis Testing, we examined logical combinations of the predictor variables in an effort to maximize their conformity with the decision ordering. Results revealed the following logical statement with a high and unusual PCC (73. 02%, p-value <. 001): Stop Research ≡ (High Idealism(6. 0 -9. 0) ∧ Female) ∨ (Low Relativism(1. 0– 5. 1) ∧ Male) Figure 1. Results from Threshold Analysis for TRIM item #16 (left) and Logical Hypothesis Testing analysis (right). Logical combinations of the 18 dichotomized TRIM items were then examined and the following combination produced the greatest discriminatory value: Self-Reflecting Man ≡ [Item 4(3: 5) ˅ Item 16(3: 5)] ˄ Item 18(1: 4) Item 4: I wish that something bad would happened to him/her Item 16: I have released my anger so I can work on restoring our relationship to health Item 18: I withdraw from him/her The subscripted values in parentheses indicate the range of scale values endorsed on the items (1 = Strongly Disagree, 2 = Disagree, etc. ), and the ˅ and ˄ symbols represent logical conjunction and disjunction, respectively. The logical statement suggests men who first self-reflected on their own capacity for wrongdoing were not completely withdrawing from the perpetrator of the harmful act (Item 18 endorsed Strongly Disagree to Agree), but in so doing they found themselves faced with the choice of wishing ill upon the person (Item 4 endorsed Neutral to Strongly Agree) or giving up their anger toward the person (Item 16 endorsed Neutral to Strongly Agree). Remarkably, the patterns of responses for 28 of the 31 men (90%) who first reflected on their own capacity for wrongdoing matched this logical statement, whereas the responses for 12 of the 24 men (50%) who did not self-reflect matched (see Figure 1). The overall Percent Correct Classification (PCC) was 72. 73%, and a randomization test based on the data from all 55 men showed this result to be highly improbable (p-value =. 001 for 1000 randomized trials). These interesting results stand in stark contrast to the non-significant findings using traditional statistical methods of analysis. Lastly, by comparison, the logical statement above did not fit the responses for women (PCC = 56. 25%, c-value =. 38. In other words, high idealism women or low relativism men were classified (or predicted) as deciding to stop the controversial research, whereas all other individuals were classified as deciding to continue the research. The PCC index for this logical combination (73. 02%) was higher than the classification accuracy (71. 75%) of the logistic regression analysis. Moreover, the logical combination was more parsimonious than the regression model, and the results could be interpreted at the level of the individuals in the study. In other words, the logical statement can be clearly applied to each and every person in the study by simply dichotomizing the idealism and relativism scores and noting the person’s gender. REFERENCES Grice, J. W (2011). Observation Oriented Modeling. New York: Academic Press. Grice, J. W. , Cota, L. D. , Barrett, P. T. , Wuensch, K. L. , & Poteat, G. M. (2016). A simple and transparent alternative to logistic regression. Advances in Social Sciences Research Journal, 3(7), 147 -165. Lin, S. (2015). Replication of Study 7 by Exline, Baumeister, Zell, Kraft & Witvliet (2008, Journal of Personality and Social Psychology). Available online at: https: //osf. io/imrx 2/ Wuensch, K. L. & Poteat, G. M. (1998). Evaluating the morality of animal research: Effects of ethical ideology, gender, and purpose. Journal of Social Behavior and Personality, 139 -150. CONTACT INFORMATION Please send inquiries to James Grice e-mail: James. grice@okstate. edu Personality Laboratory: http: //psychology. okstate. edu/faculty/jgrice