Keyword Generation for Search Engine Advertising Amruta Joshi
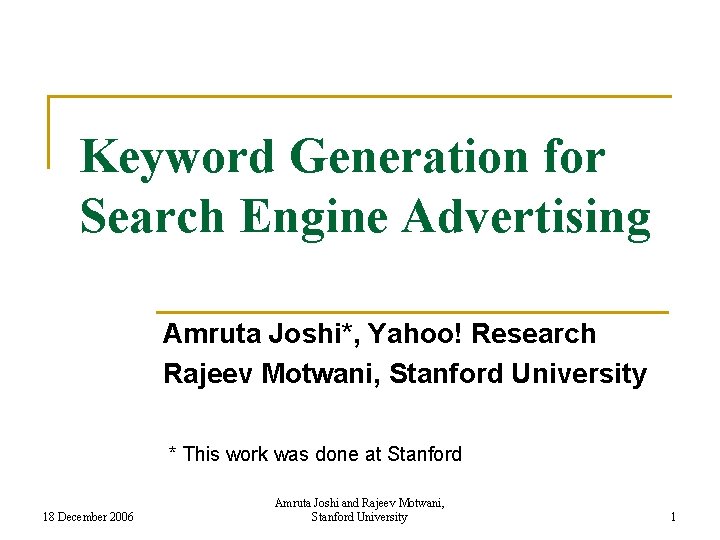
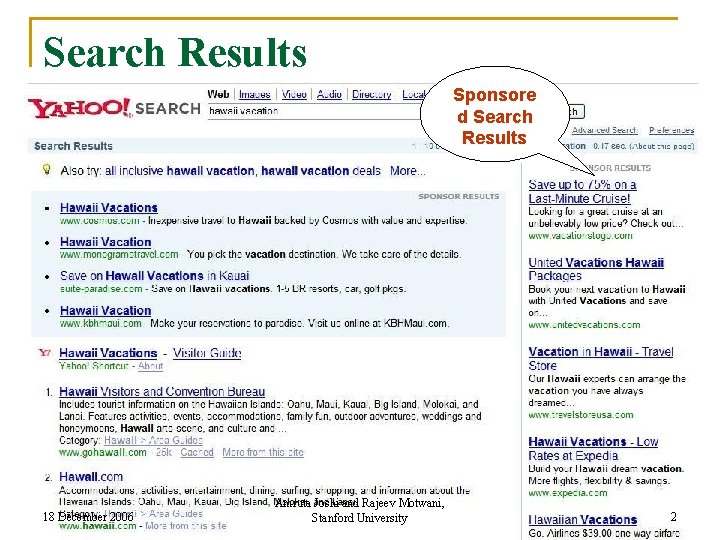
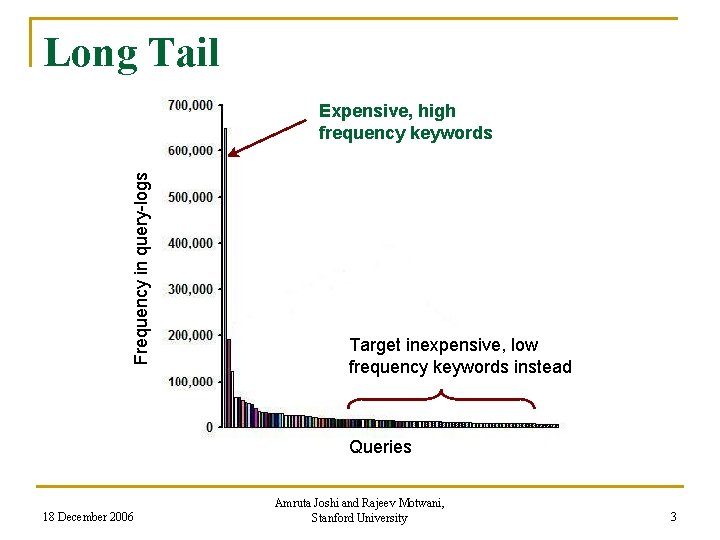
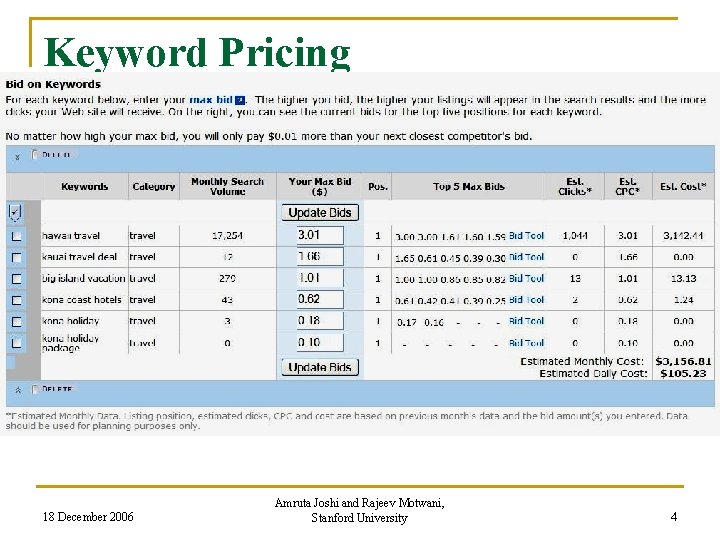
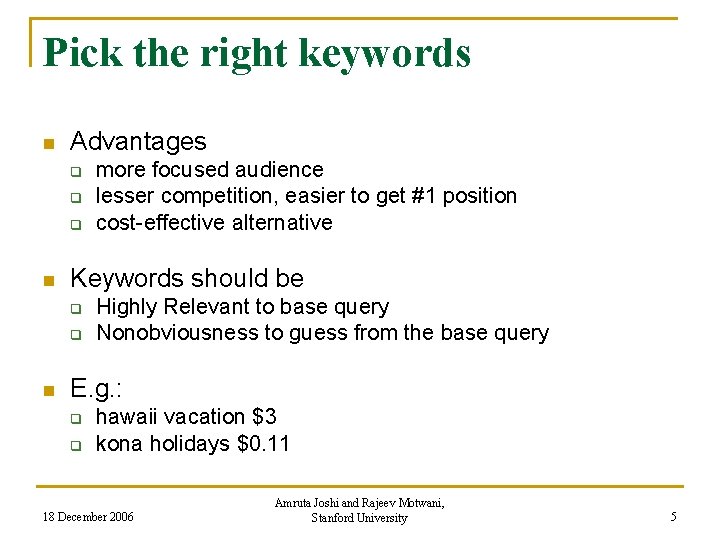
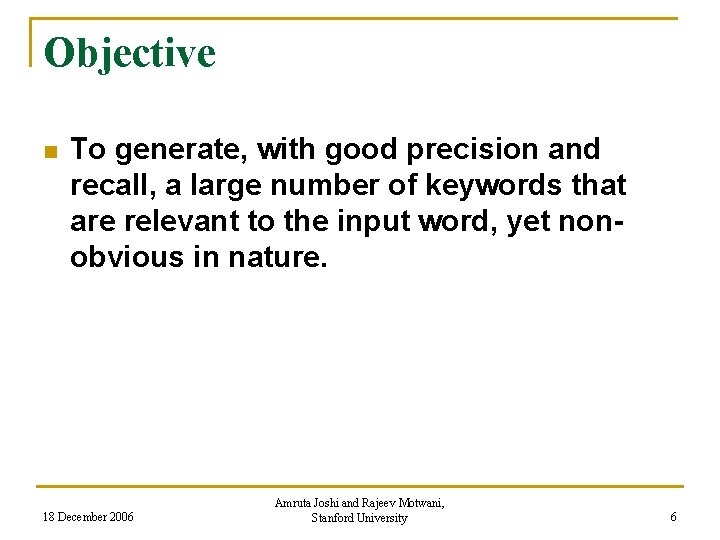
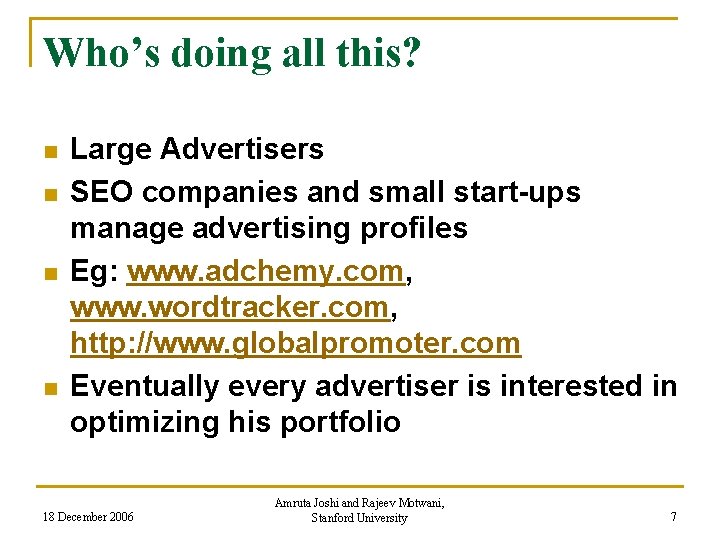
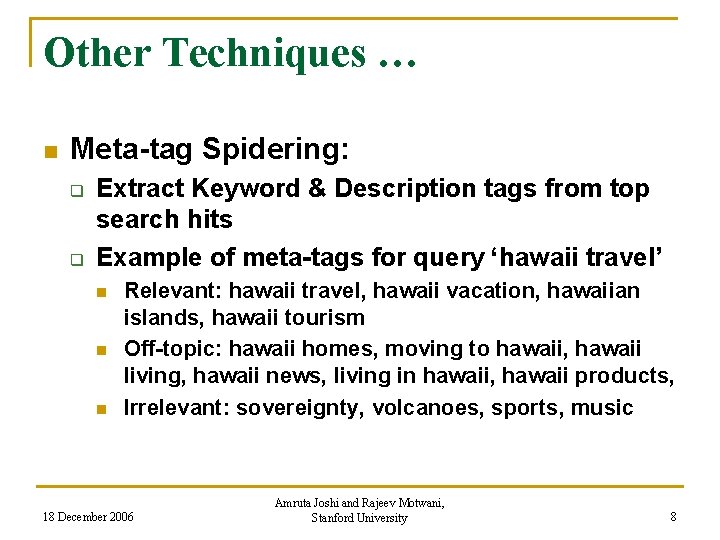
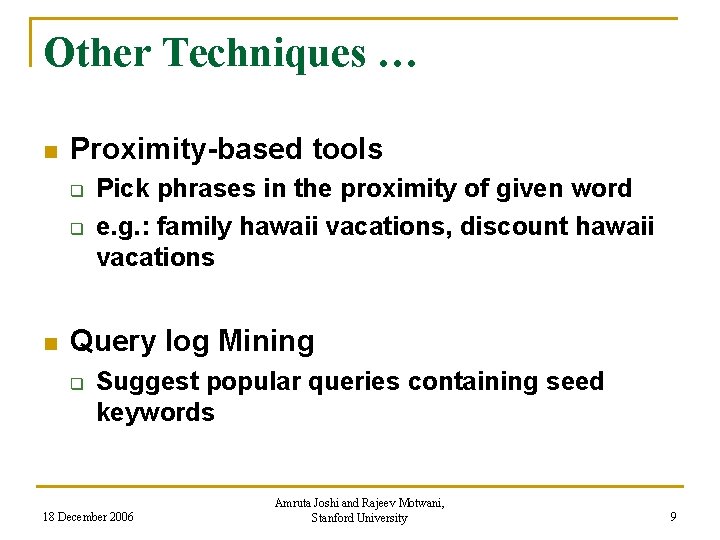
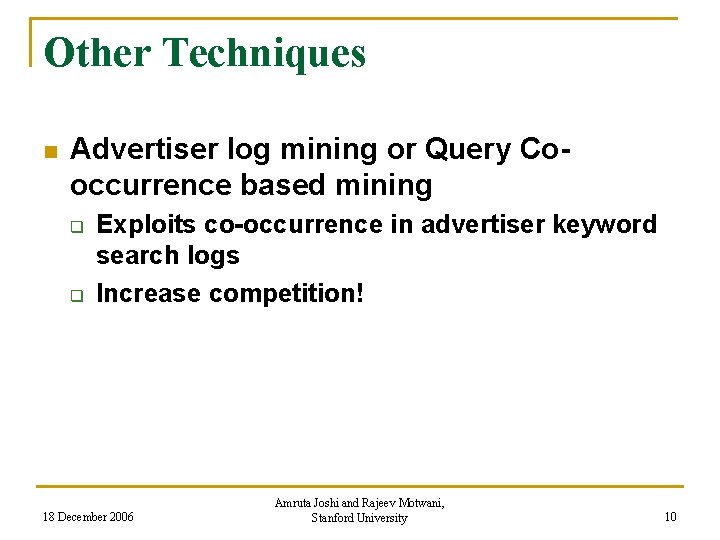
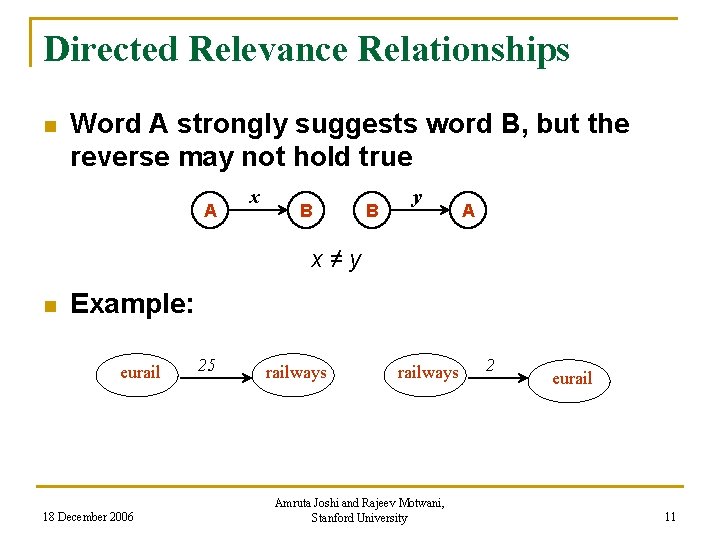
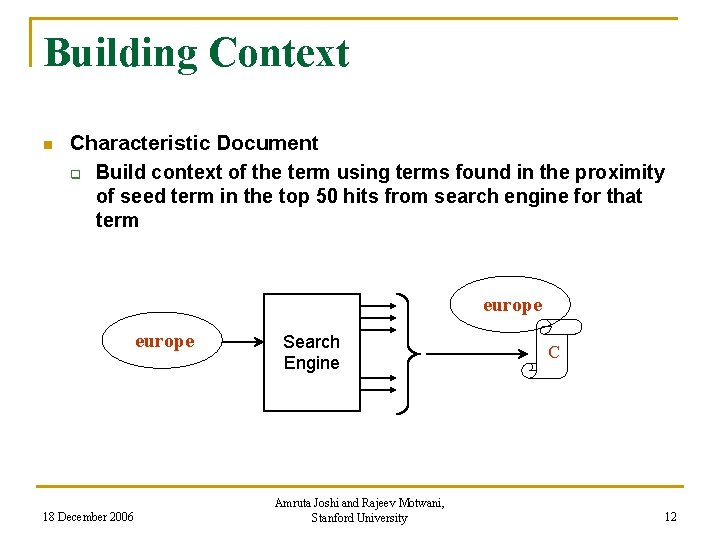
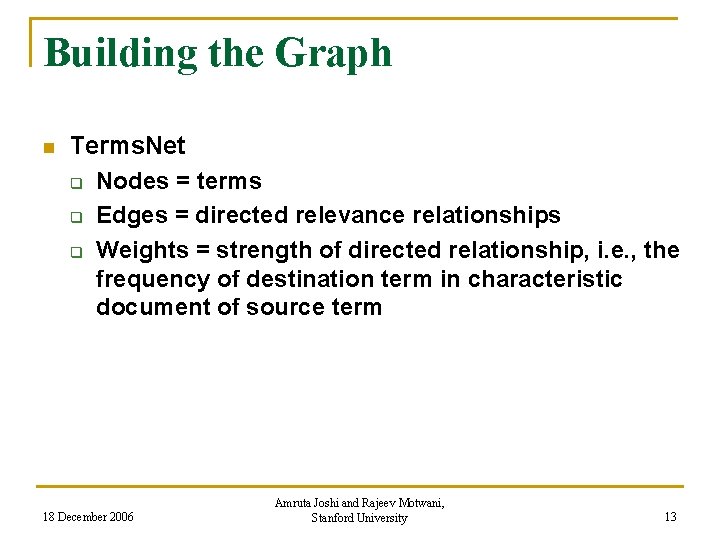
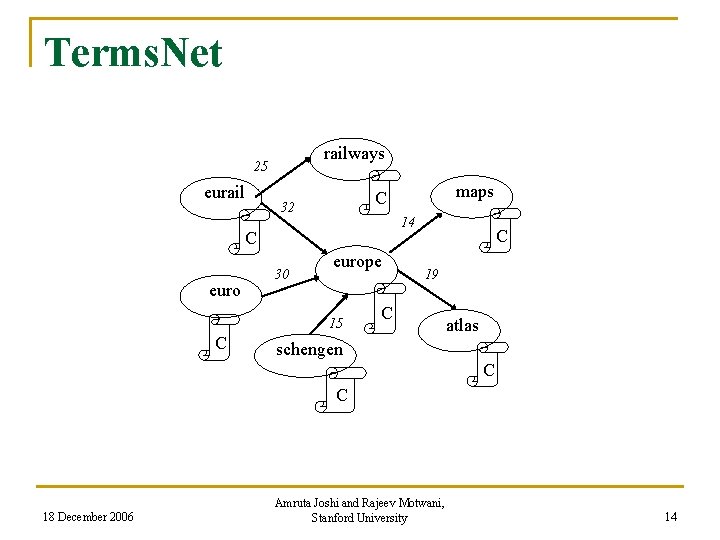
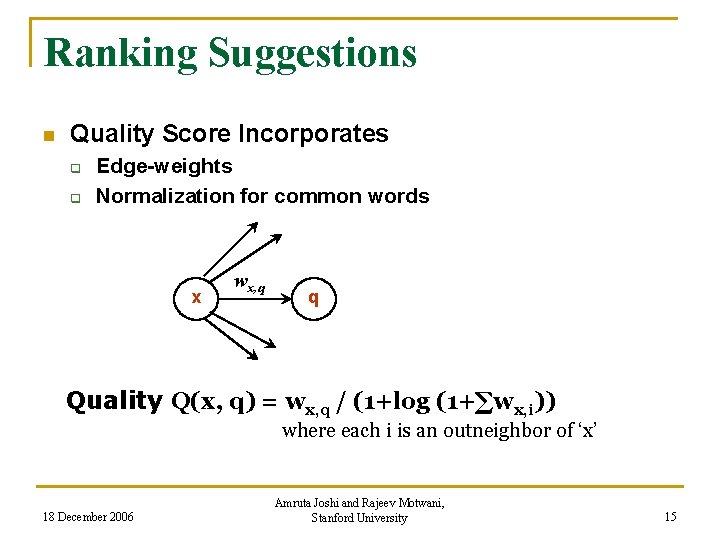
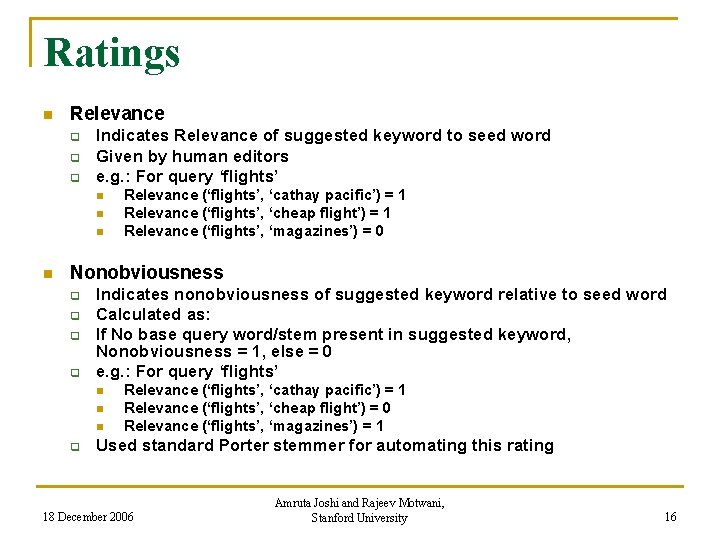
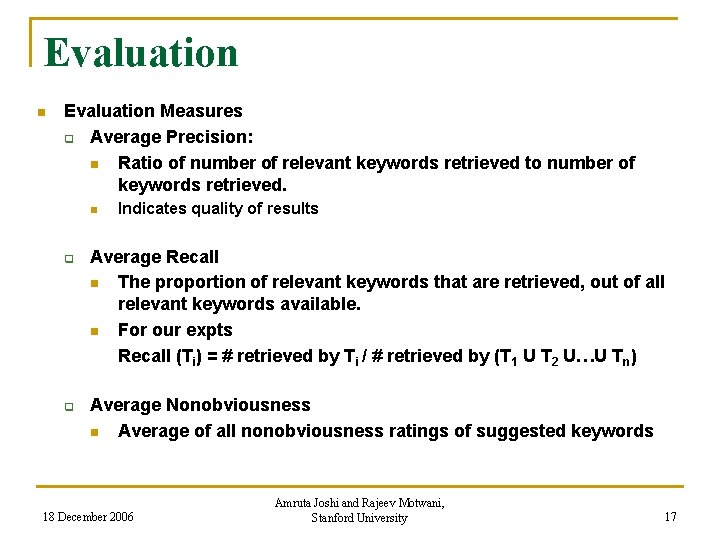
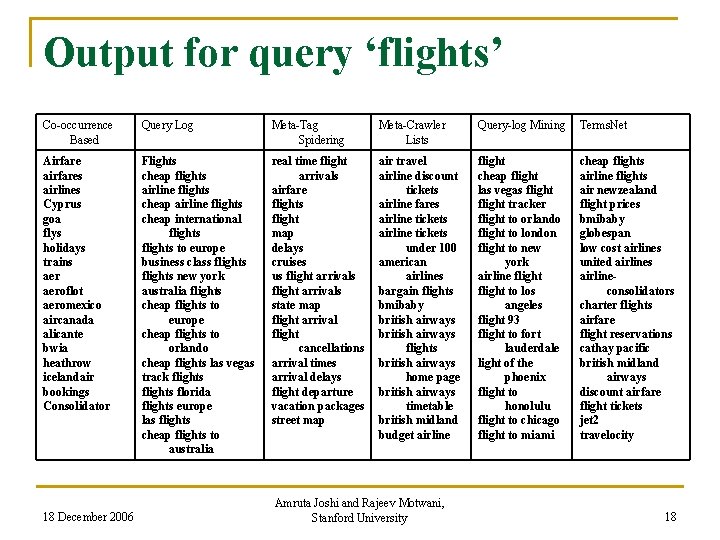
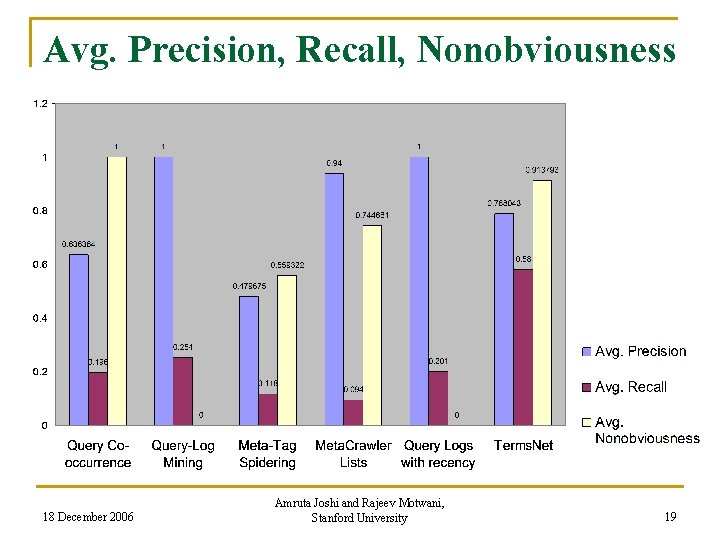
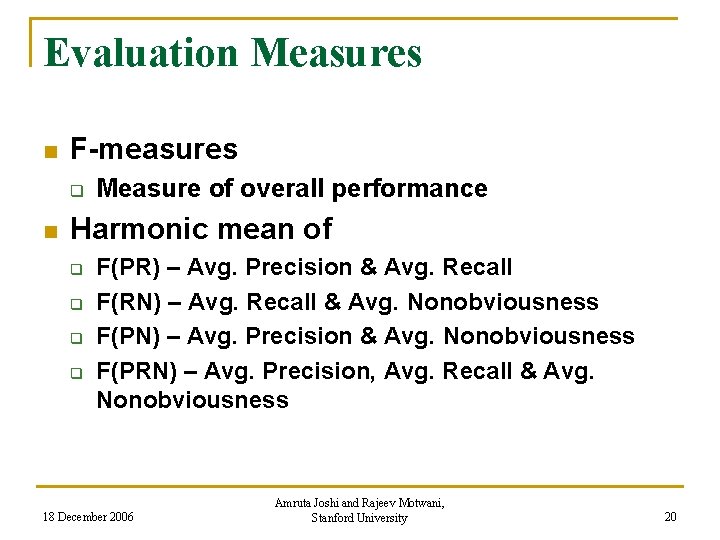
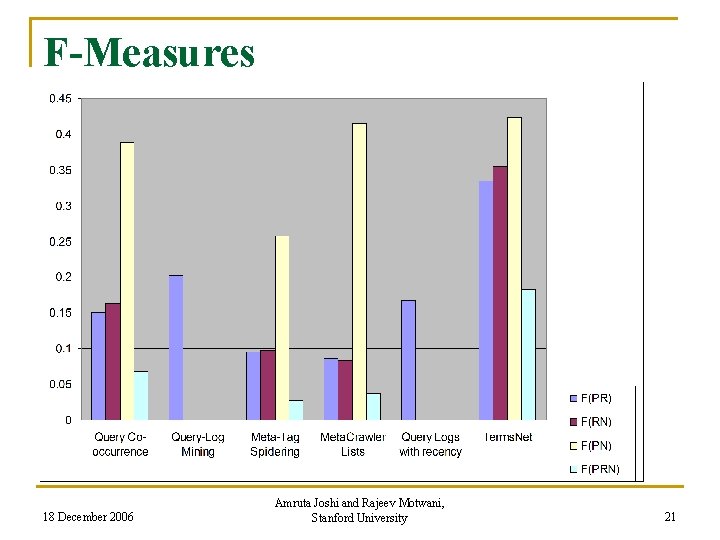
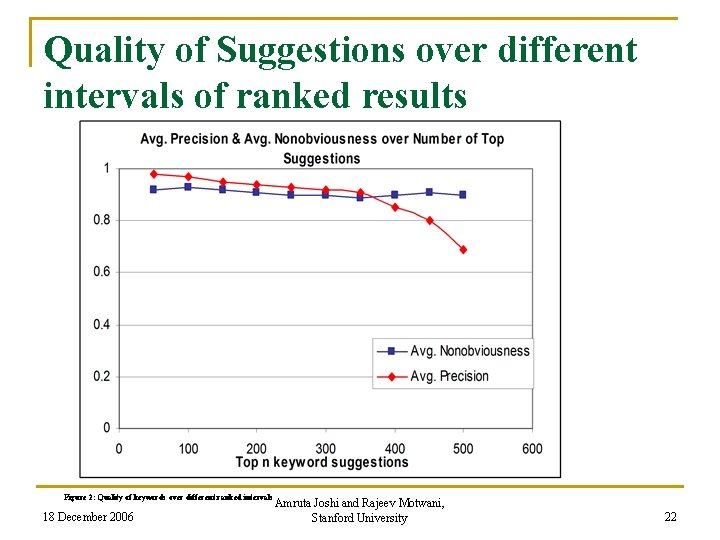
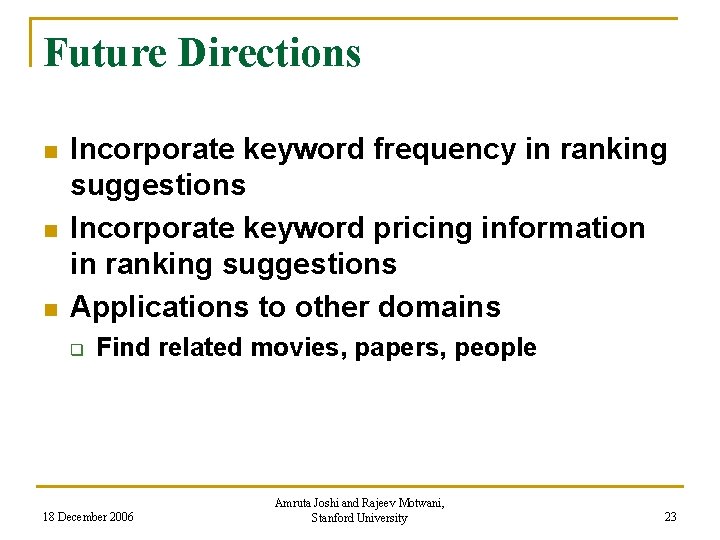
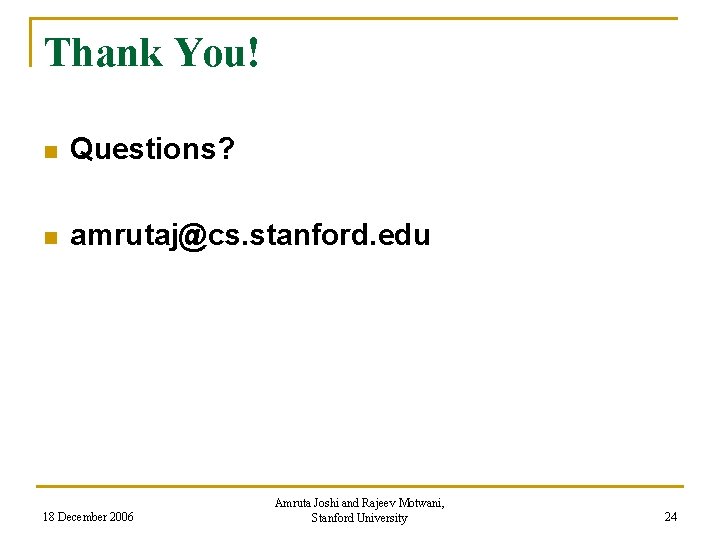
- Slides: 24
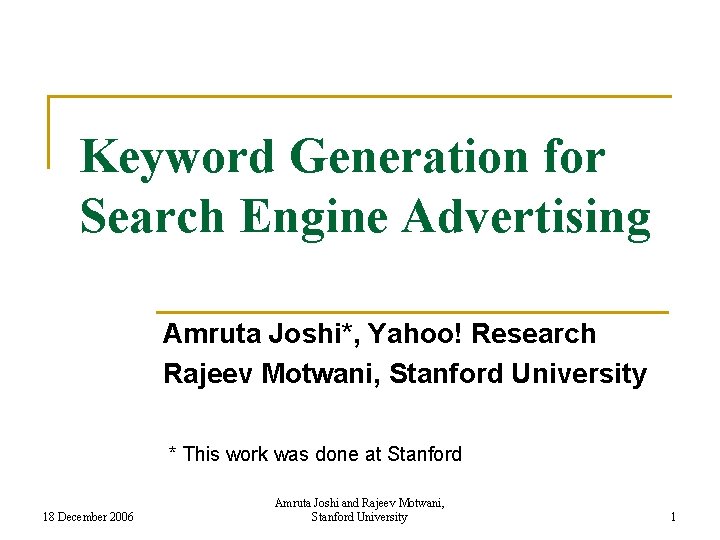
Keyword Generation for Search Engine Advertising Amruta Joshi*, Yahoo! Research Rajeev Motwani, Stanford University * This work was done at Stanford 18 December 2006 Amruta Joshi and Rajeev Motwani, Stanford University 1
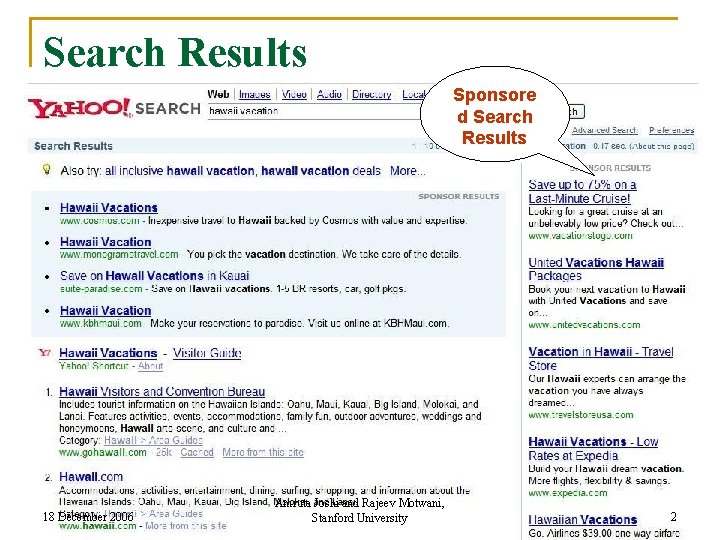
Search Results Sponsore d Search Results 18 December 2006 Amruta Joshi and Rajeev Motwani, Stanford University 2
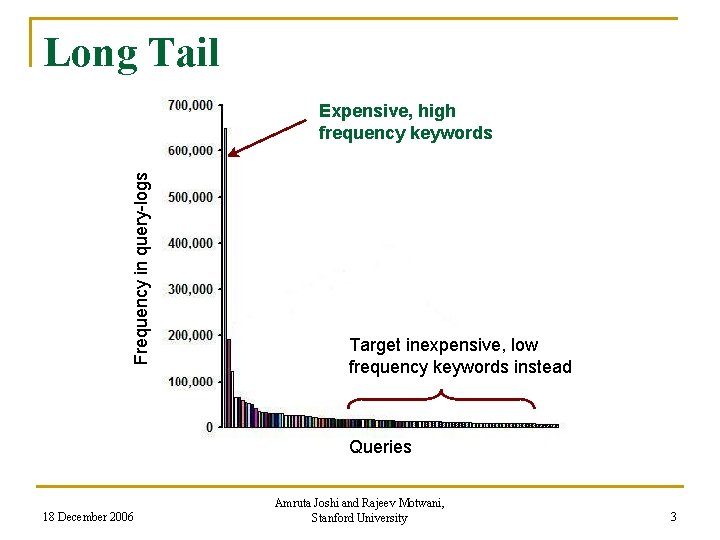
Long Tail Frequency in query-logs Expensive, high frequency keywords Target inexpensive, low frequency keywords instead Queries 18 December 2006 Amruta Joshi and Rajeev Motwani, Stanford University 3
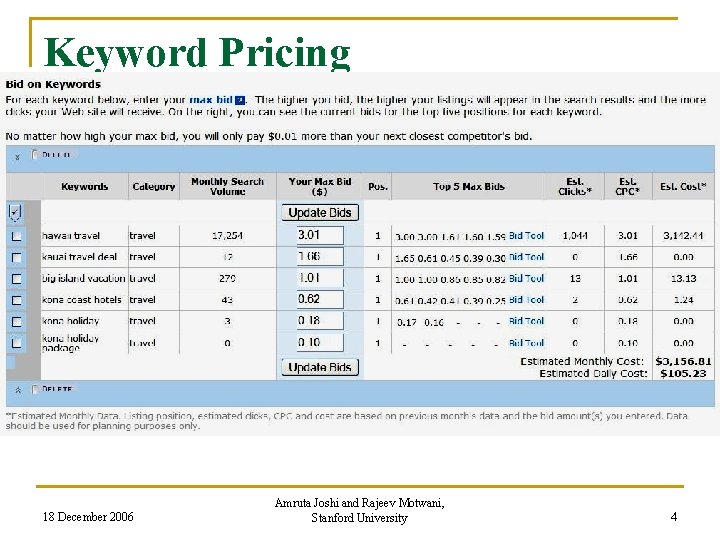
Keyword Pricing 18 December 2006 Amruta Joshi and Rajeev Motwani, Stanford University 4
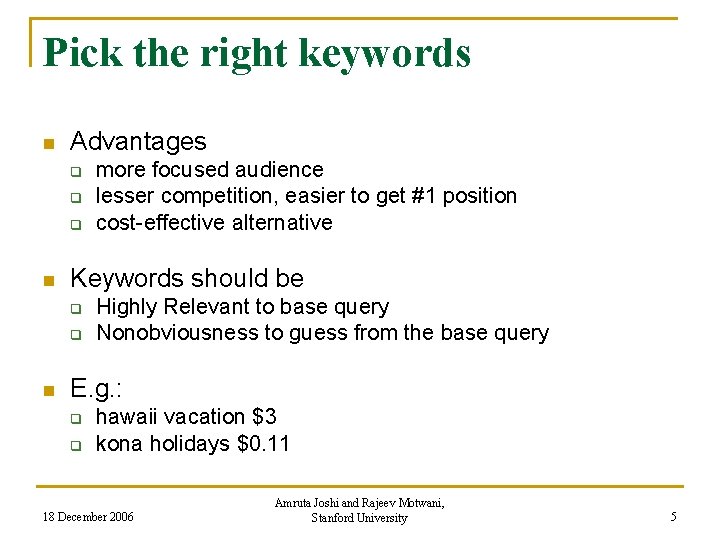
Pick the right keywords n Advantages q q q n Keywords should be q q n more focused audience lesser competition, easier to get #1 position cost-effective alternative Highly Relevant to base query Nonobviousness to guess from the base query E. g. : q q hawaii vacation $3 kona holidays $0. 11 18 December 2006 Amruta Joshi and Rajeev Motwani, Stanford University 5
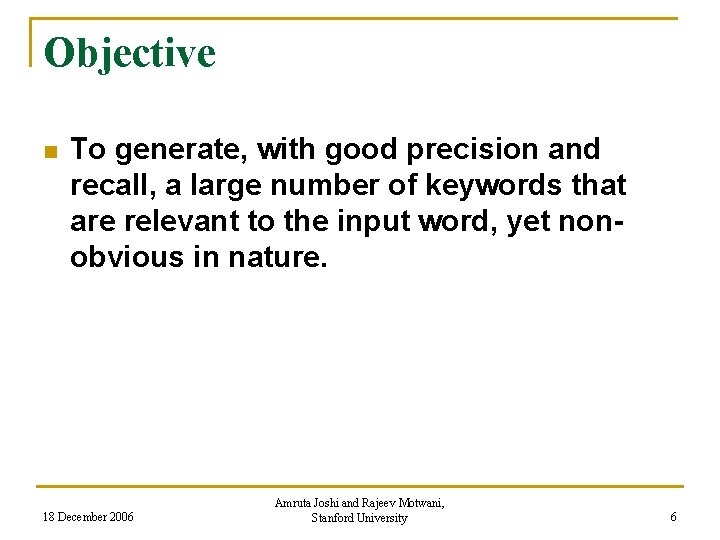
Objective n To generate, with good precision and recall, a large number of keywords that are relevant to the input word, yet nonobvious in nature. 18 December 2006 Amruta Joshi and Rajeev Motwani, Stanford University 6
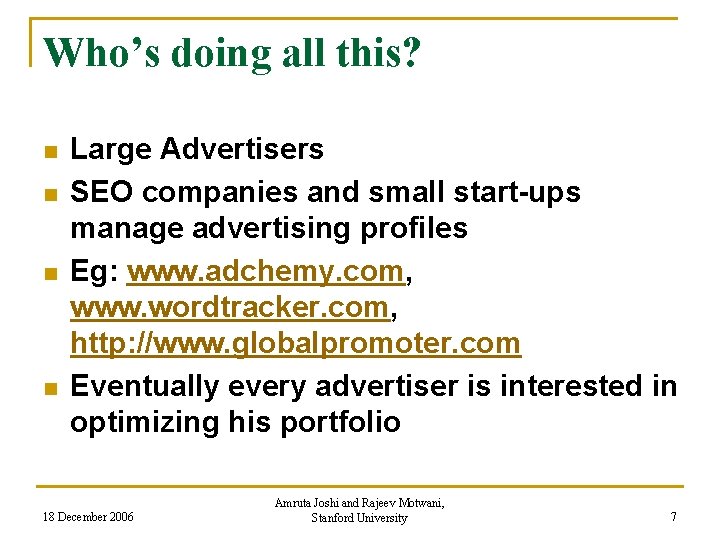
Who’s doing all this? n n Large Advertisers SEO companies and small start-ups manage advertising profiles Eg: www. adchemy. com, www. wordtracker. com, http: //www. globalpromoter. com Eventually every advertiser is interested in optimizing his portfolio 18 December 2006 Amruta Joshi and Rajeev Motwani, Stanford University 7
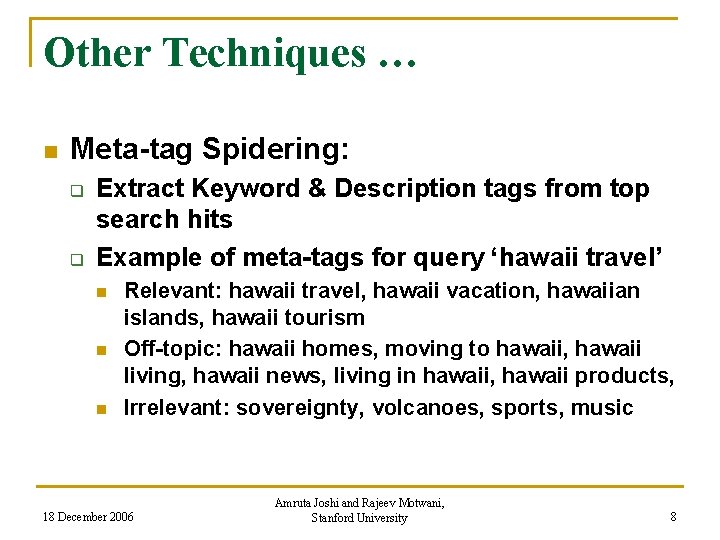
Other Techniques … n Meta-tag Spidering: q q Extract Keyword & Description tags from top search hits Example of meta-tags for query ‘hawaii travel’ n n n Relevant: hawaii travel, hawaii vacation, hawaiian islands, hawaii tourism Off-topic: hawaii homes, moving to hawaii, hawaii living, hawaii news, living in hawaii, hawaii products, Irrelevant: sovereignty, volcanoes, sports, music 18 December 2006 Amruta Joshi and Rajeev Motwani, Stanford University 8
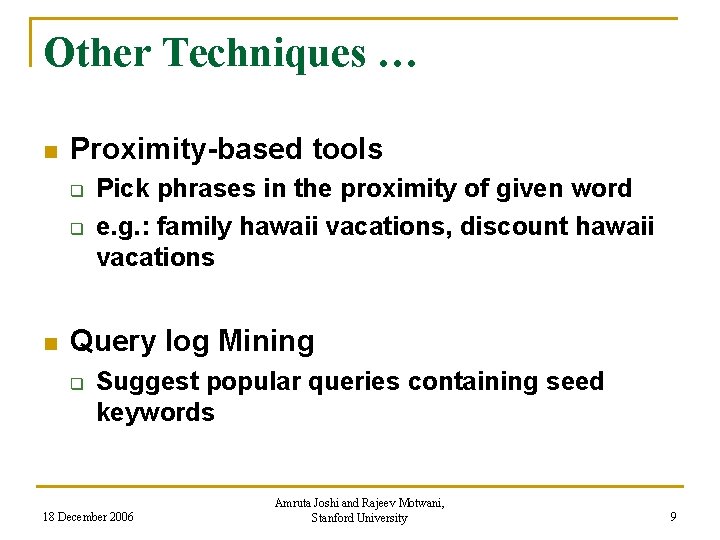
Other Techniques … n Proximity-based tools q q n Pick phrases in the proximity of given word e. g. : family hawaii vacations, discount hawaii vacations Query log Mining q Suggest popular queries containing seed keywords 18 December 2006 Amruta Joshi and Rajeev Motwani, Stanford University 9
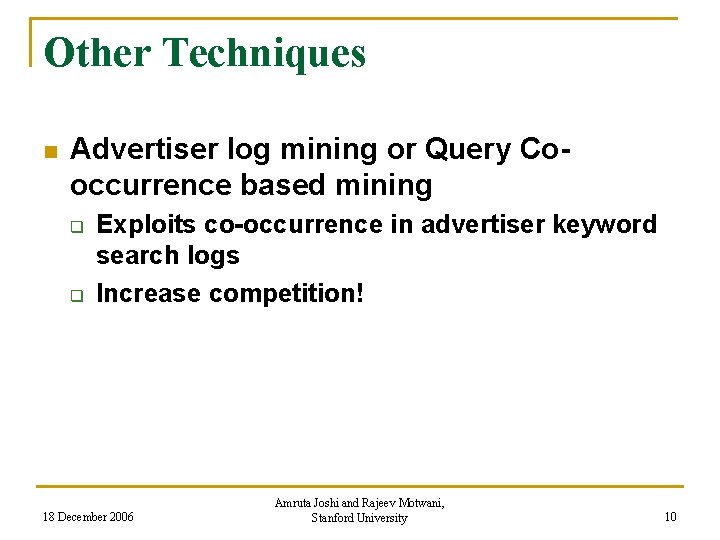
Other Techniques n Advertiser log mining or Query Cooccurrence based mining q q Exploits co-occurrence in advertiser keyword search logs Increase competition! 18 December 2006 Amruta Joshi and Rajeev Motwani, Stanford University 10
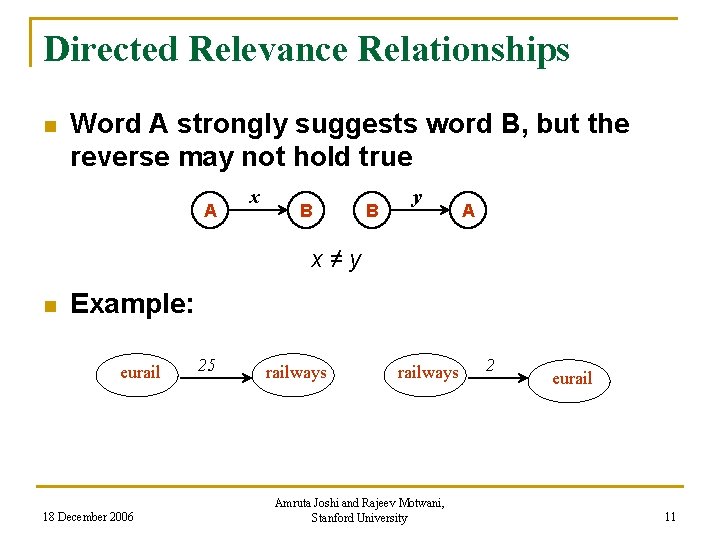
Directed Relevance Relationships n Word A strongly suggests word B, but the reverse may not hold true A x B B y A x≠y n Example: eurail 18 December 2006 25 railways Amruta Joshi and Rajeev Motwani, Stanford University 2 eurail 11
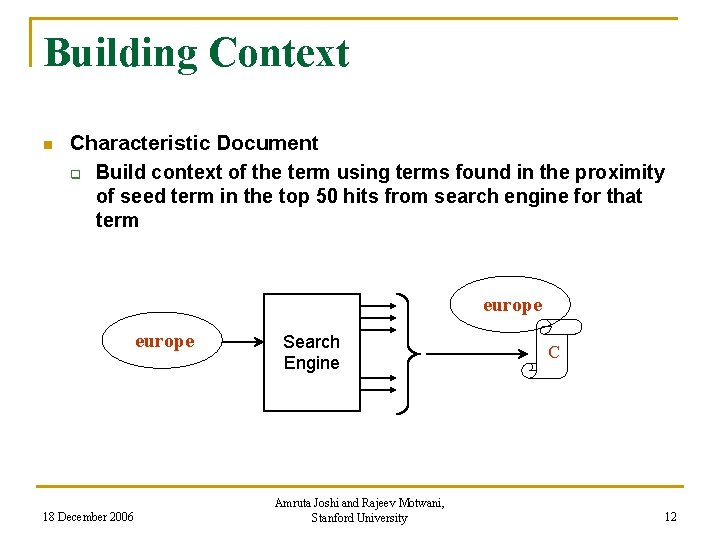
Building Context n Characteristic Document q Build context of the term using terms found in the proximity of seed term in the top 50 hits from search engine for that term europe 18 December 2006 . Search Engine Amruta Joshi and Rajeev Motwani, Stanford University . C 12
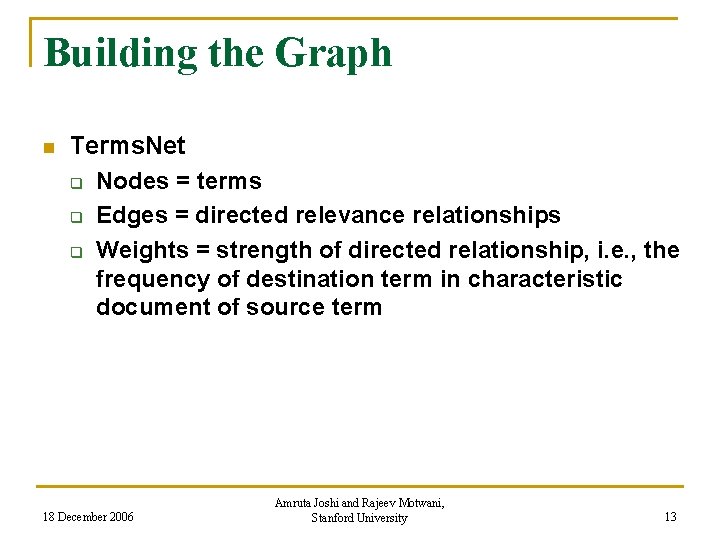
Building the Graph n Terms. Net q Nodes = terms q Edges = directed relevance relationships q Weights = strength of directed relationship, i. e. , the frequency of destination term in characteristic document of source term 18 December 2006 Amruta Joshi and Rajeev Motwani, Stanford University 13
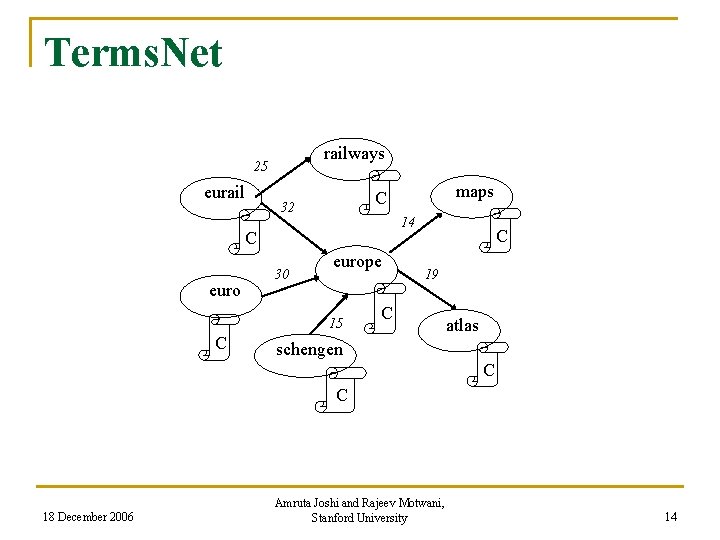
Terms. Net railways 25 eurail 14 C euro 30 europe. 15 C maps C 32 C 19 C atlas schengen C C 18 December 2006 Amruta Joshi and Rajeev Motwani, Stanford University 14
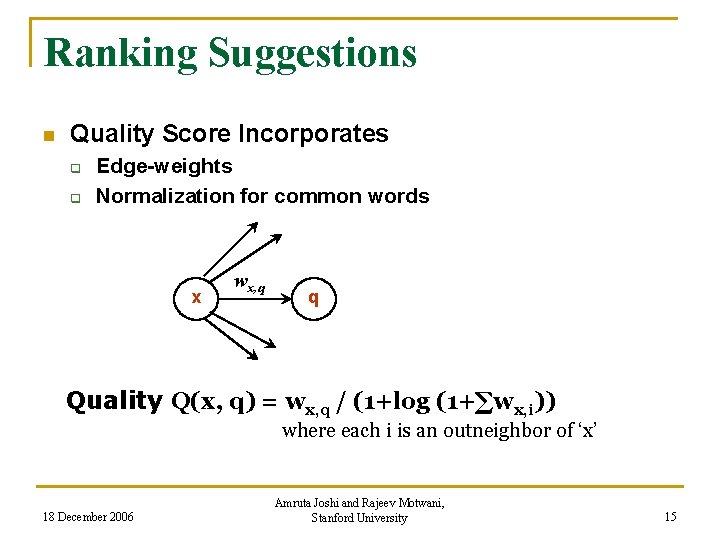
Ranking Suggestions n Quality Score Incorporates q q Edge-weights Normalization for common words x wx, q q Quality Q(x, q) = wx, q / (1+log (1+∑wx, i)) where each i is an outneighbor of ‘x’ 18 December 2006 Amruta Joshi and Rajeev Motwani, Stanford University 15
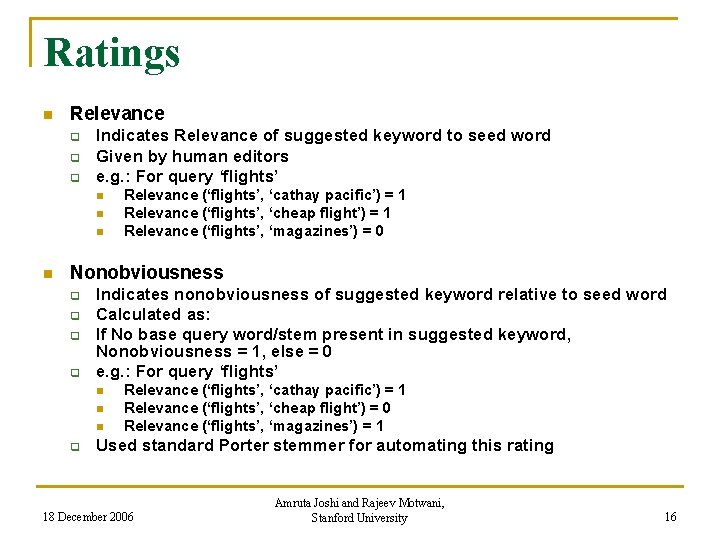
Ratings n Relevance q q q Indicates Relevance of suggested keyword to seed word Given by human editors e. g. : For query ‘flights’ n n Relevance (‘flights’, ‘cathay pacific’) = 1 Relevance (‘flights’, ‘cheap flight’) = 1 Relevance (‘flights’, ‘magazines’) = 0 Nonobviousness q q Indicates nonobviousness of suggested keyword relative to seed word Calculated as: If No base query word/stem present in suggested keyword, Nonobviousness = 1, else = 0 e. g. : For query ‘flights’ n n n q Relevance (‘flights’, ‘cathay pacific’) = 1 Relevance (‘flights’, ‘cheap flight’) = 0 Relevance (‘flights’, ‘magazines’) = 1 Used standard Porter stemmer for automating this rating 18 December 2006 Amruta Joshi and Rajeev Motwani, Stanford University 16
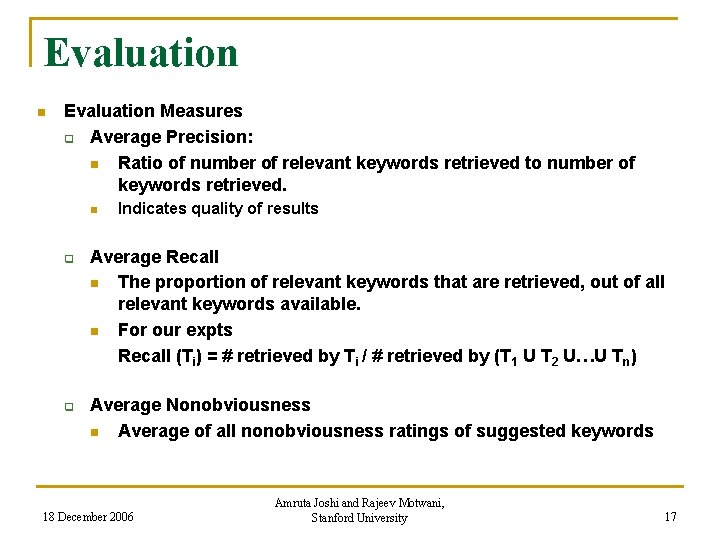
Evaluation n Evaluation Measures q Average Precision: n Ratio of number of relevant keywords retrieved to number of keywords retrieved. n q q Indicates quality of results Average Recall n The proportion of relevant keywords that are retrieved, out of all relevant keywords available. n For our expts Recall (Ti) = # retrieved by Ti / # retrieved by (T 1 U T 2 U…U Tn) Average Nonobviousness n Average of all nonobviousness ratings of suggested keywords 18 December 2006 Amruta Joshi and Rajeev Motwani, Stanford University 17
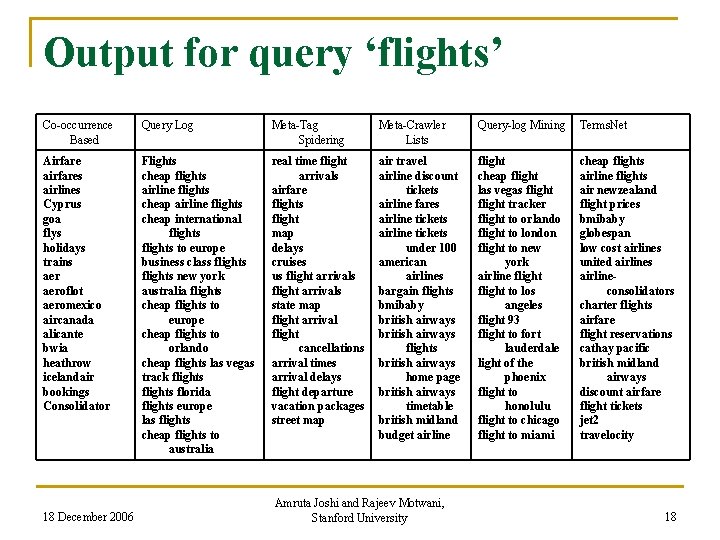
Output for query ‘flights’ Co-occurrence Based Query Log Meta-Tag Spidering Meta-Crawler Lists Query-log Mining Terms. Net Airfare airfares airlines Cyprus goa flys holidays trains aeroflot aeromexico aircanada alicante bwia heathrow icelandair bookings Consolidator Flights cheap flights airline flights cheap international flights to europe business class flights new york australia flights cheap flights to europe cheap flights to orlando cheap flights las vegas track flights florida flights europe las flights cheap flights to australia real time flight arrivals airfare flights flight map delays cruises us flight arrivals state map flight arrival flight cancellations arrival times arrival delays flight departure vacation packages street map air travel airline discount tickets airline fares airline tickets under 100 american airlines bargain flights bmibaby british airways flights british airways home page british airways timetable british midland budget airline flight cheap flight las vegas flight tracker flight to orlando flight to london flight to new york airline flight to los angeles flight 93 flight to fort lauderdale light of the phoenix flight to honolulu flight to chicago flight to miami cheap flights airline flights air newzealand flight prices bmibaby globespan low cost airlines united airlines airlineconsolidators charter flights airfare flight reservations cathay pacific british midland airways discount airfare flight tickets jet 2 travelocity 18 December 2006 Amruta Joshi and Rajeev Motwani, Stanford University 18
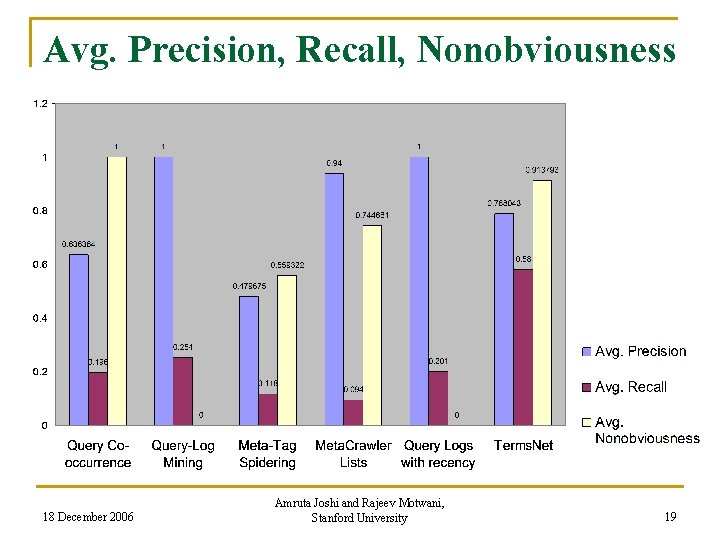
Avg. Precision, Recall, Nonobviousness 18 December 2006 Amruta Joshi and Rajeev Motwani, Stanford University 19
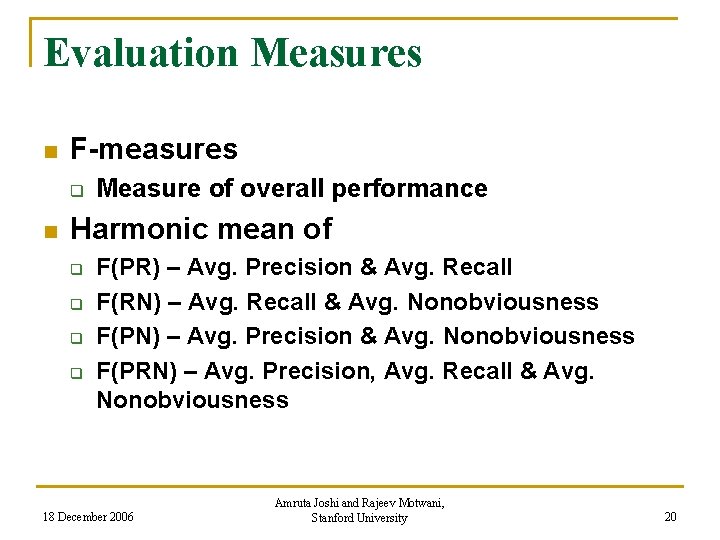
Evaluation Measures n F-measures q n Measure of overall performance Harmonic mean of q q F(PR) – Avg. Precision & Avg. Recall F(RN) – Avg. Recall & Avg. Nonobviousness F(PN) – Avg. Precision & Avg. Nonobviousness F(PRN) – Avg. Precision, Avg. Recall & Avg. Nonobviousness 18 December 2006 Amruta Joshi and Rajeev Motwani, Stanford University 20
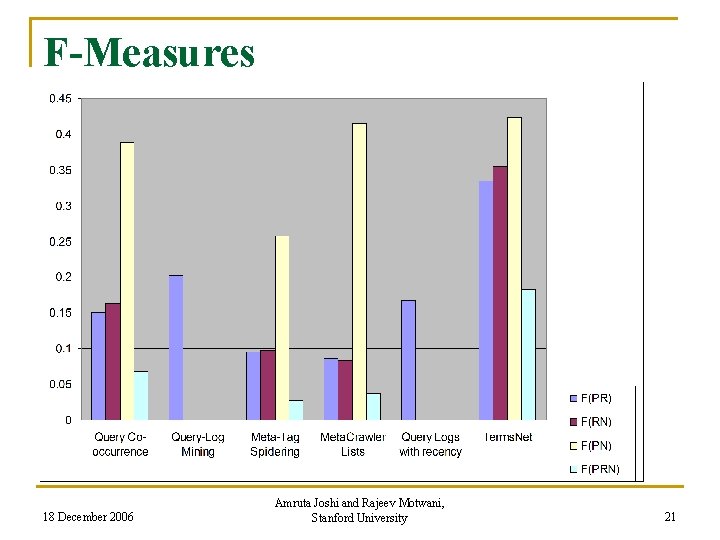
F-Measures 18 December 2006 Amruta Joshi and Rajeev Motwani, Stanford University 21
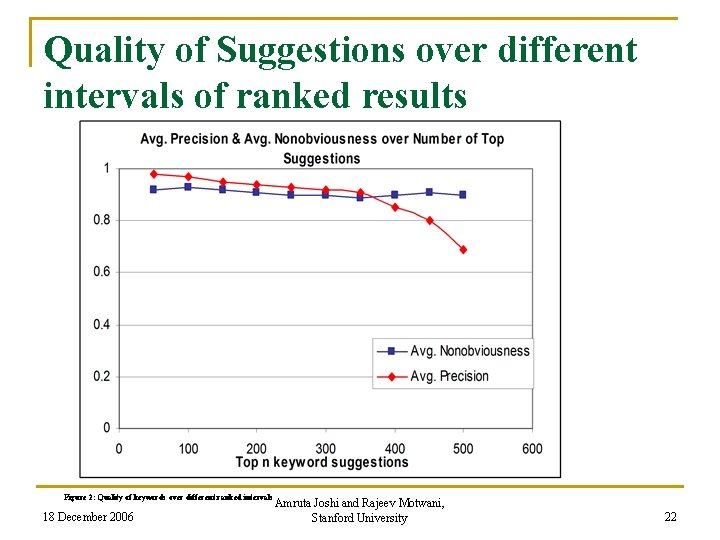
Quality of Suggestions over different intervals of ranked results Figure 2: Quality of keywords over different ranked intervals 18 December 2006 Amruta Joshi and Rajeev Motwani, Stanford University 22
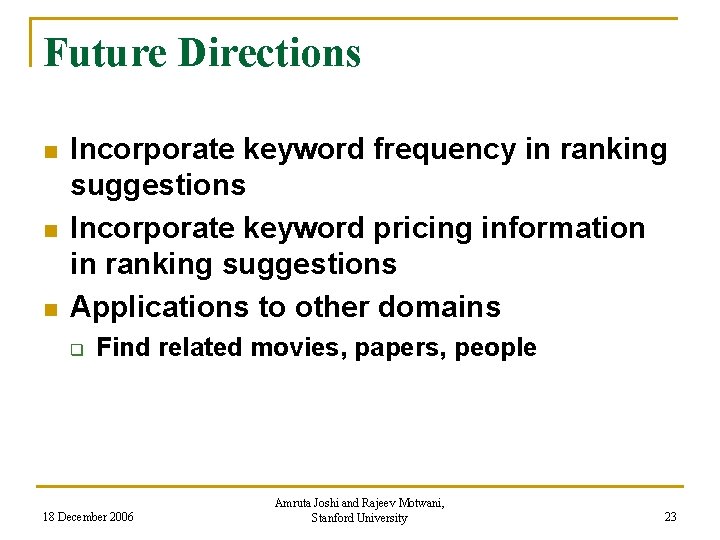
Future Directions n n n Incorporate keyword frequency in ranking suggestions Incorporate keyword pricing information in ranking suggestions Applications to other domains q Find related movies, papers, people 18 December 2006 Amruta Joshi and Rajeev Motwani, Stanford University 23
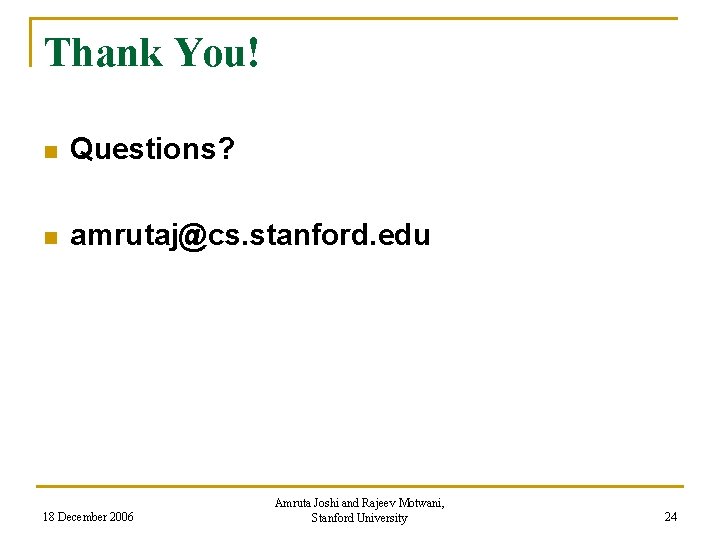
Thank You! n Questions? n amrutaj@cs. stanford. edu 18 December 2006 Amruta Joshi and Rajeev Motwani, Stanford University 24