Introduction to Inference Statistical Hypotheses Statistical Hypotheses are
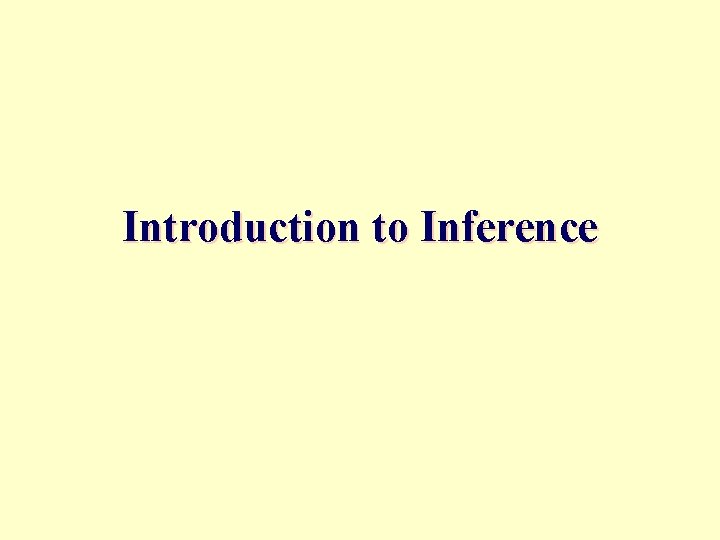
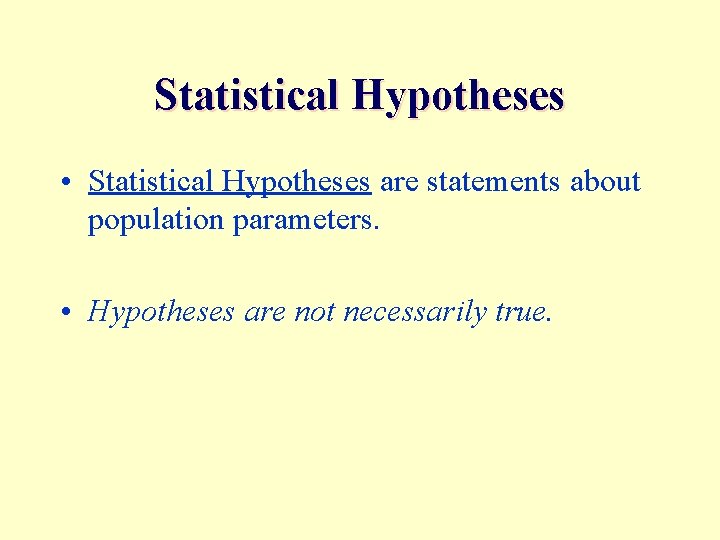
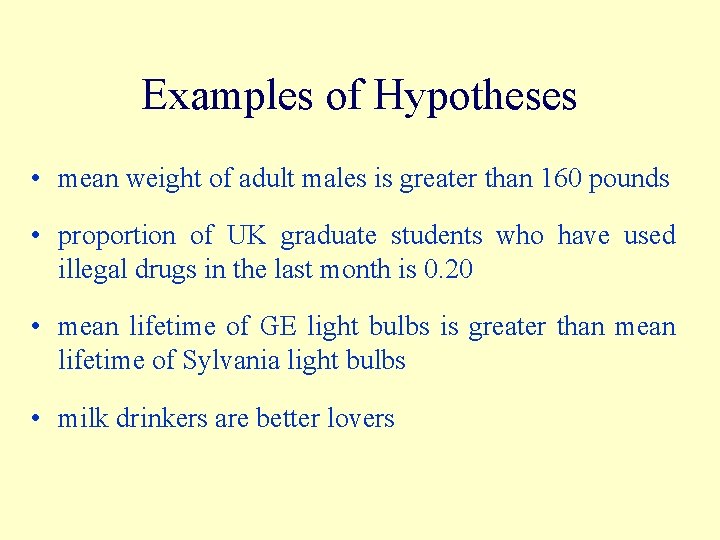
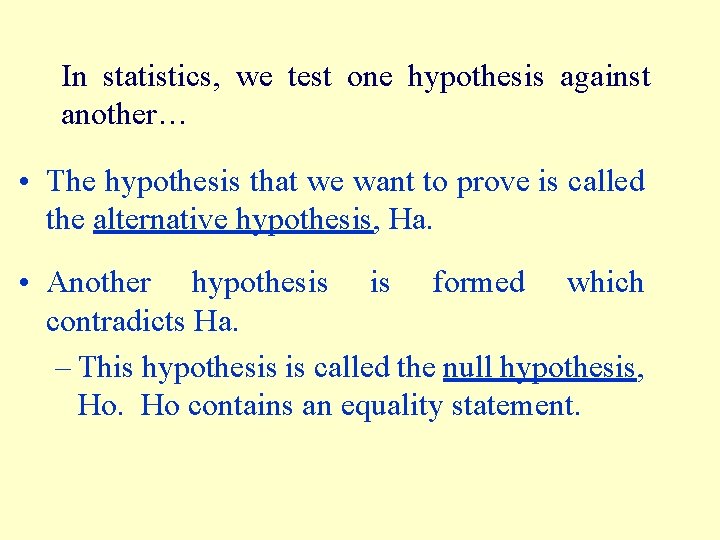
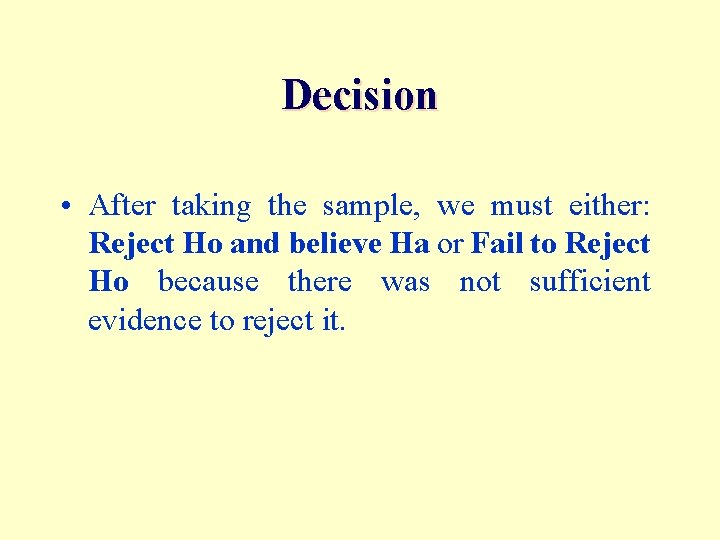
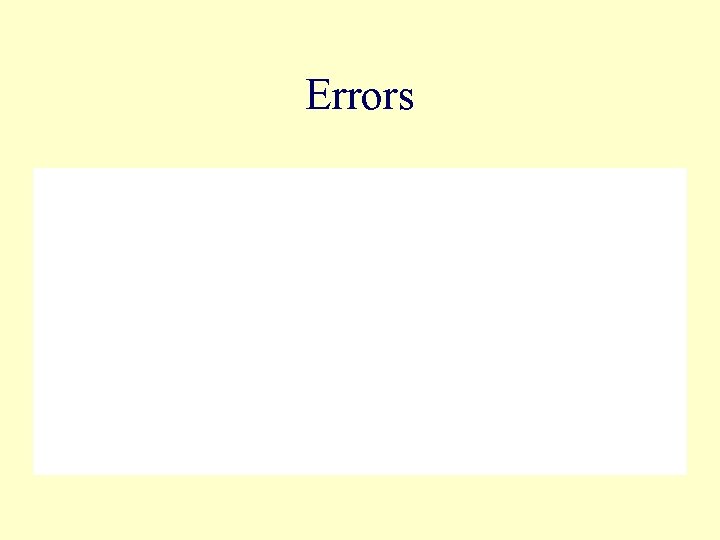
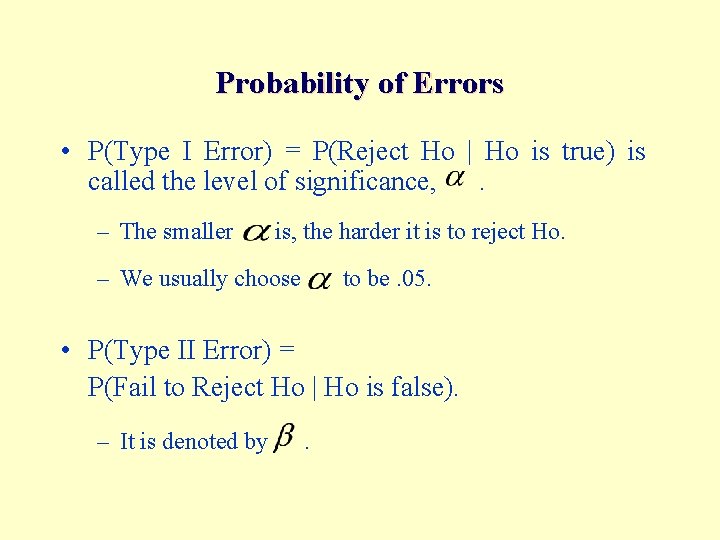
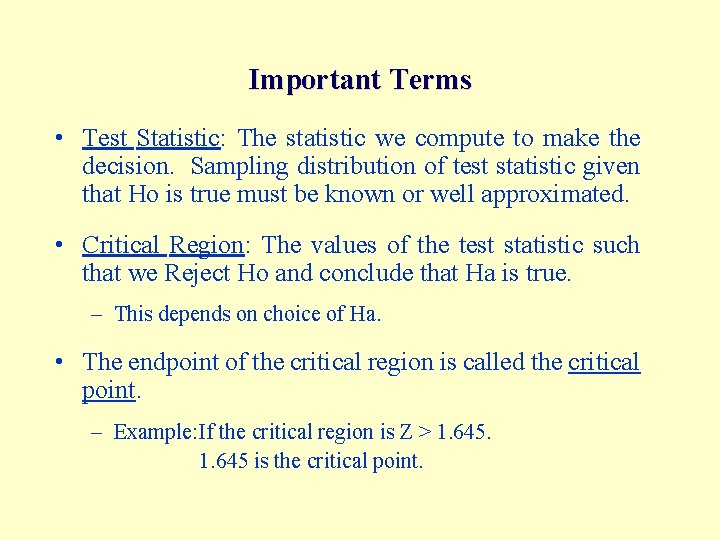
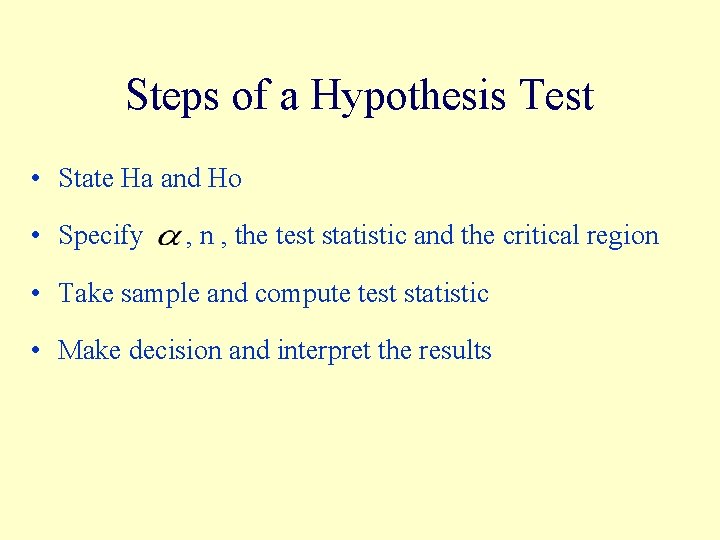
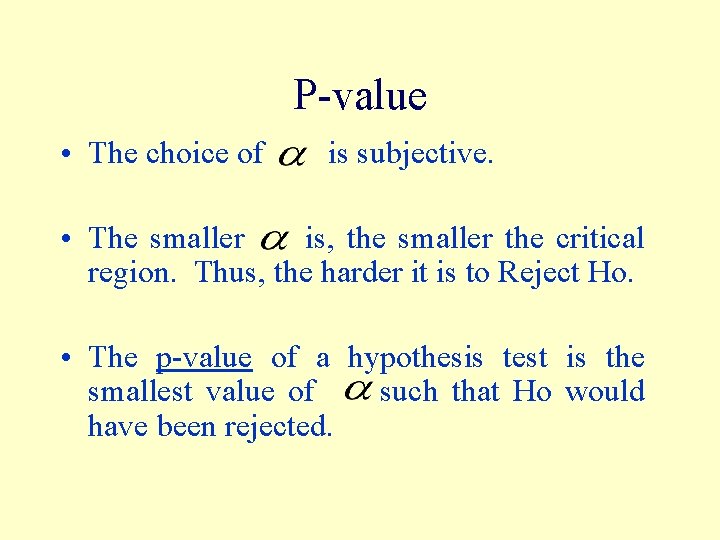
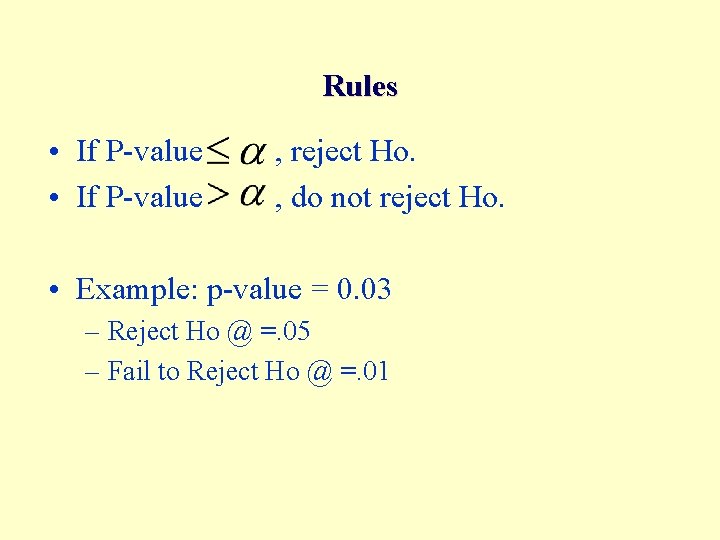
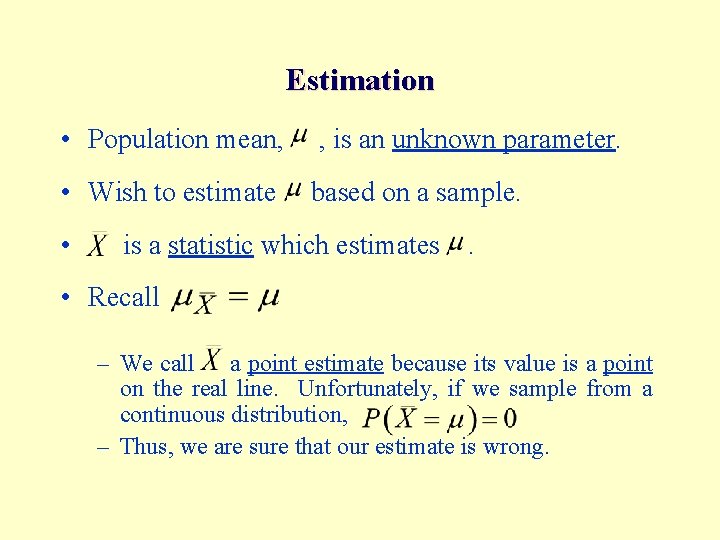
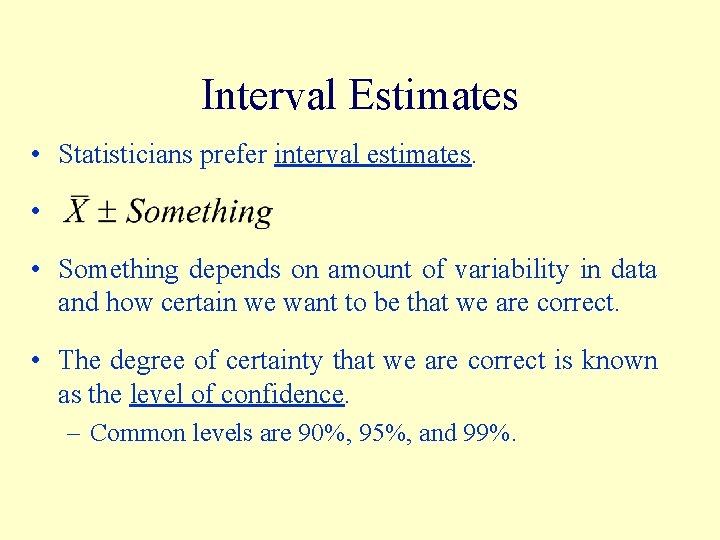
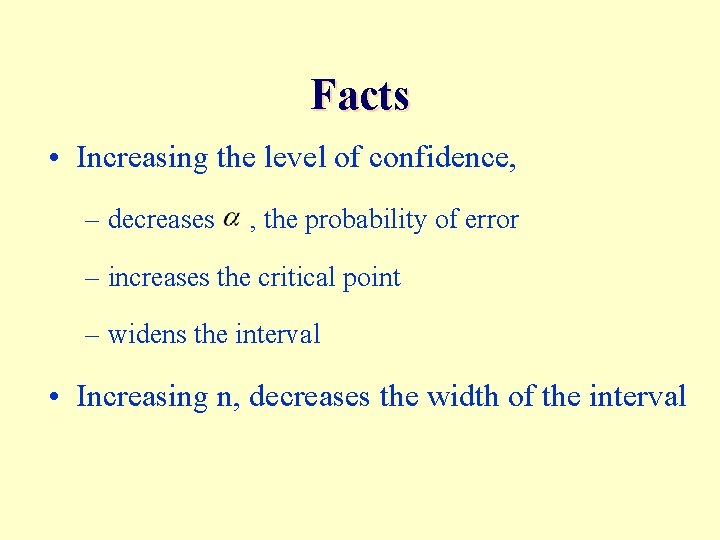
- Slides: 14
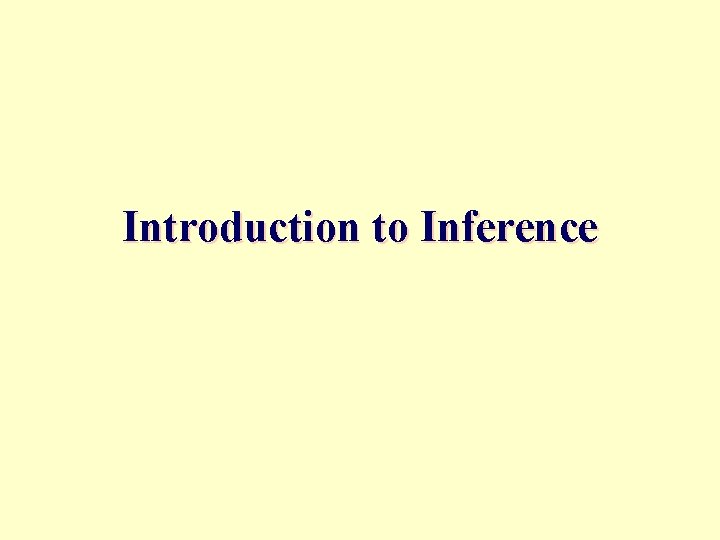
Introduction to Inference
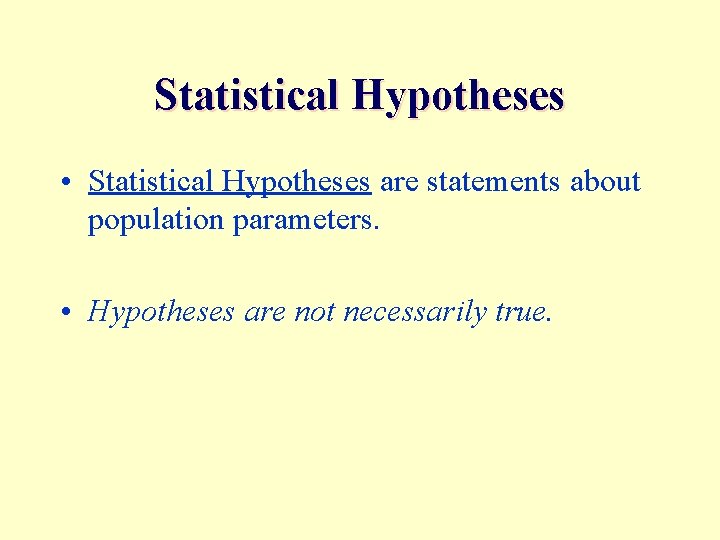
Statistical Hypotheses • Statistical Hypotheses are statements about population parameters. • Hypotheses are not necessarily true.
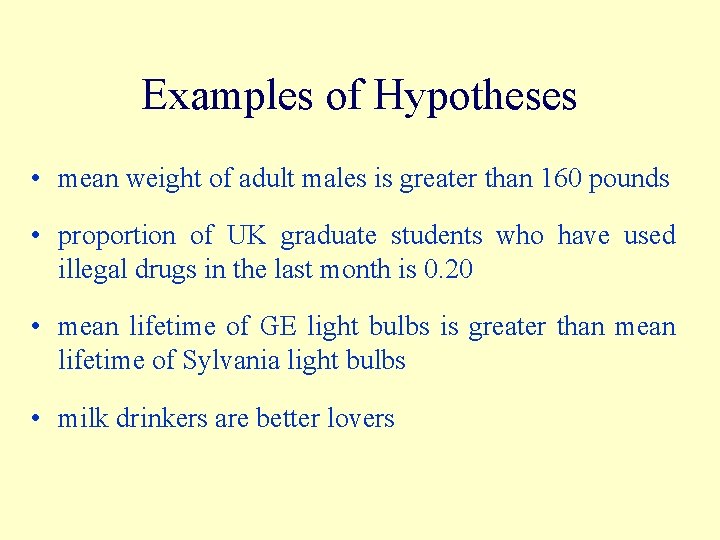
Examples of Hypotheses • mean weight of adult males is greater than 160 pounds • proportion of UK graduate students who have used illegal drugs in the last month is 0. 20 • mean lifetime of GE light bulbs is greater than mean lifetime of Sylvania light bulbs • milk drinkers are better lovers
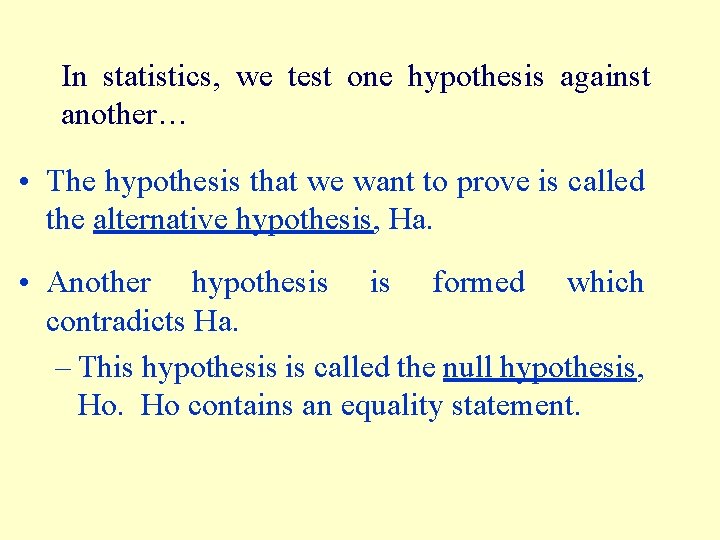
In statistics, we test one hypothesis against another… • The hypothesis that we want to prove is called the alternative hypothesis, Ha. • Another hypothesis is formed which contradicts Ha. – This hypothesis is called the null hypothesis, Ho contains an equality statement.
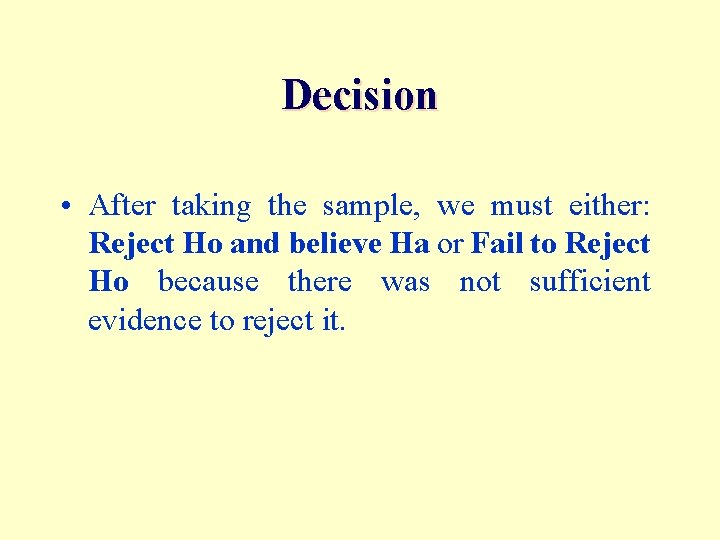
Decision • After taking the sample, we must either: Reject Ho and believe Ha or Fail to Reject Ho because there was not sufficient evidence to reject it.
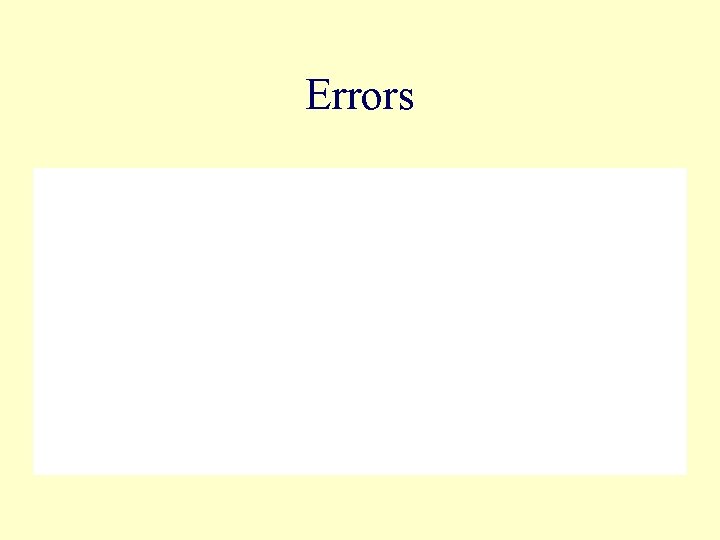
Errors
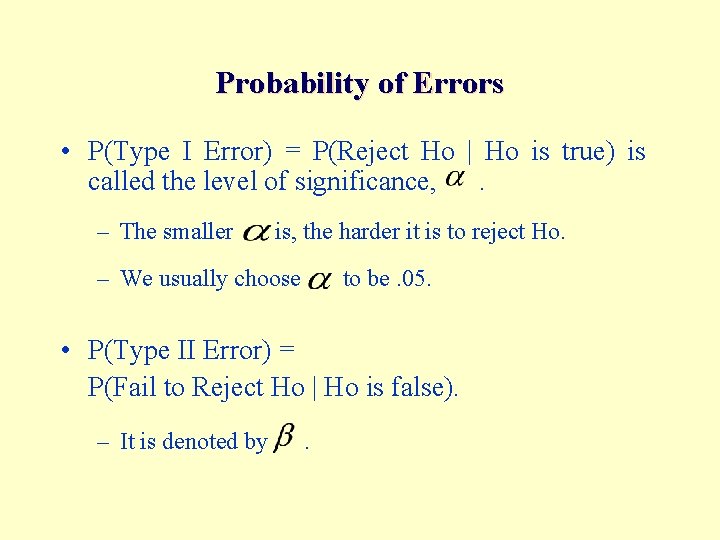
Probability of Errors • P(Type I Error) = P(Reject Ho | Ho is true) is called the level of significance, . – The smaller is, the harder it is to reject Ho. – We usually choose to be. 05. • P(Type II Error) = P(Fail to Reject Ho | Ho is false). – It is denoted by .
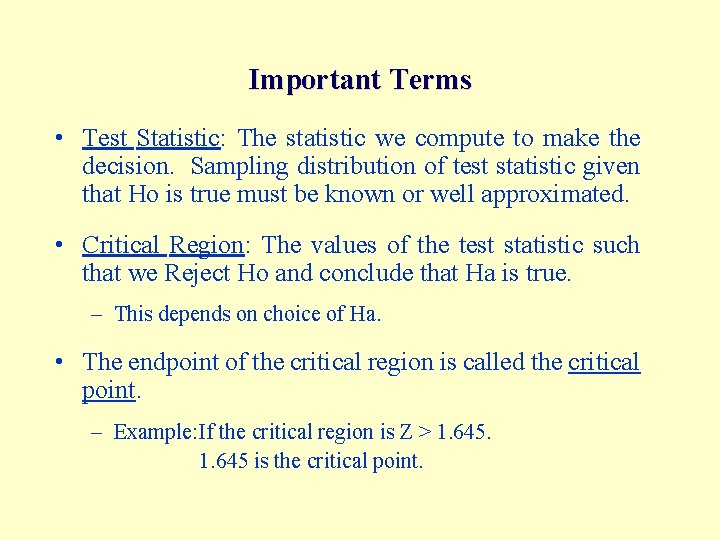
Important Terms • Test Statistic: The statistic we compute to make the decision. Sampling distribution of test statistic given that Ho is true must be known or well approximated. • Critical Region: The values of the test statistic such that we Reject Ho and conclude that Ha is true. – This depends on choice of Ha. • The endpoint of the critical region is called the critical point. – Example: If the critical region is Z > 1. 645 is the critical point.
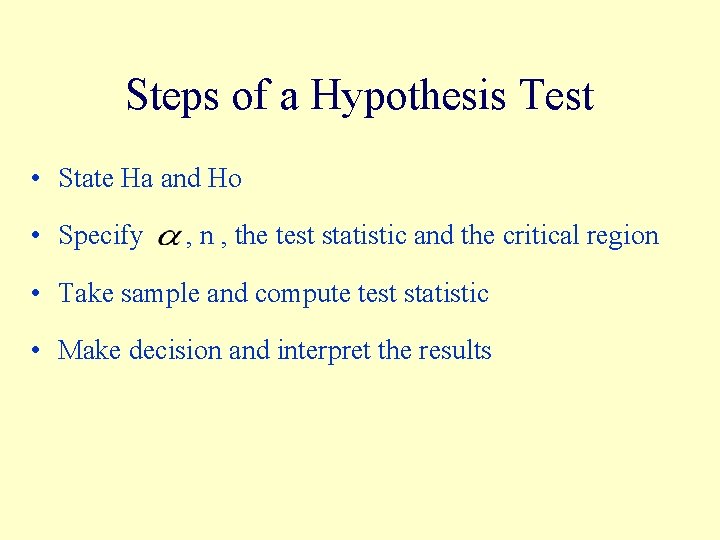
Steps of a Hypothesis Test • State Ha and Ho • Specify , n , the test statistic and the critical region • Take sample and compute test statistic • Make decision and interpret the results
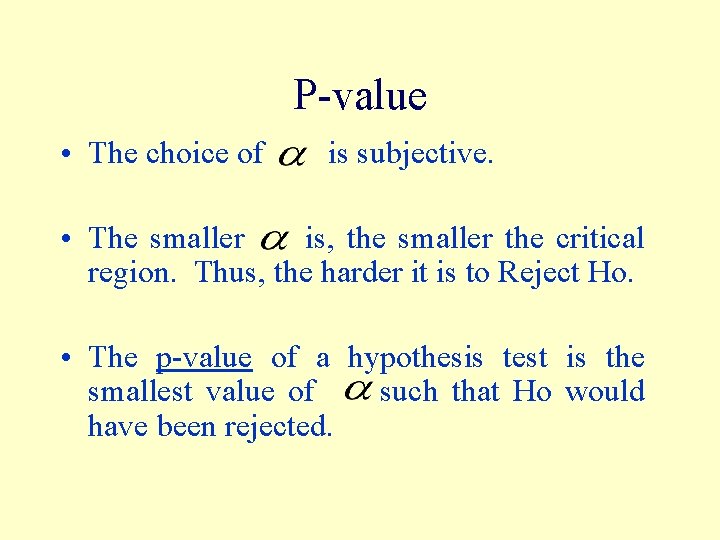
P-value • The choice of is subjective. • The smaller is, the smaller the critical region. Thus, the harder it is to Reject Ho. • The p-value of a hypothesis test is the smallest value of such that Ho would have been rejected.
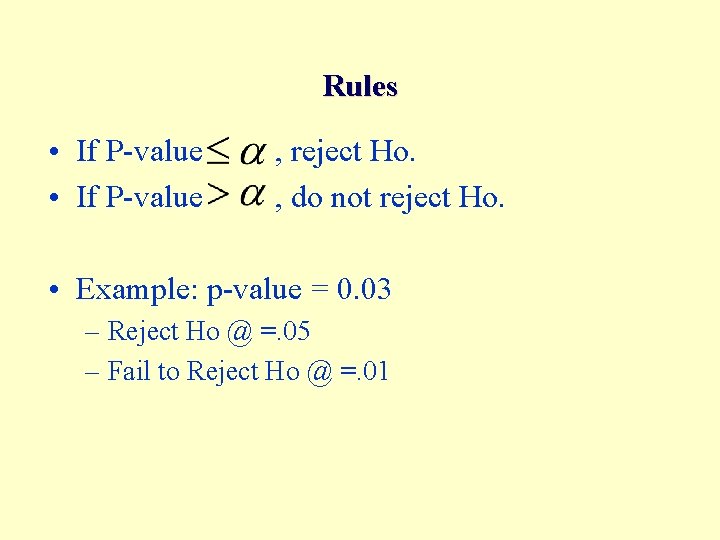
Rules • If P-value , reject Ho. • If P-value , do not reject Ho. • Example: p-value = 0. 03 – Reject Ho @ =. 05 – Fail to Reject Ho @ =. 01
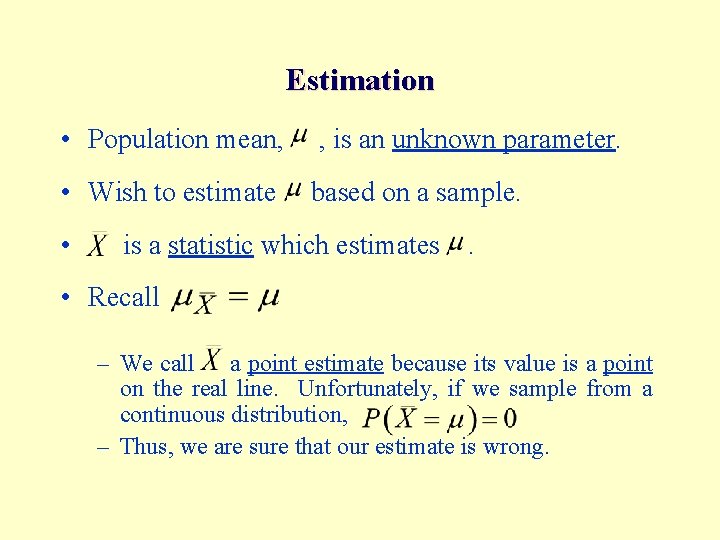
Estimation • Population mean, , is an unknown parameter. • Wish to estimate based on a sample. • is a statistic which estimates . • Recall – We call a point estimate because its value is a point on the real line. Unfortunately, if we sample from a continuous distribution, – Thus, we are sure that our estimate is wrong.
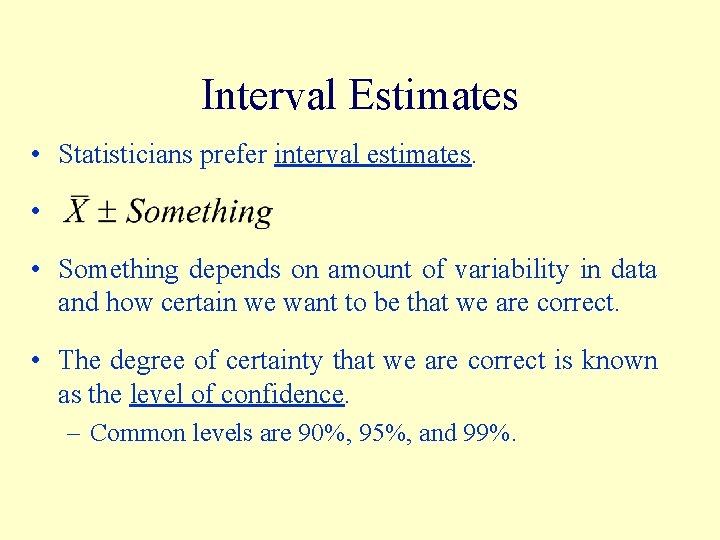
Interval Estimates • Statisticians prefer interval estimates. • • Something depends on amount of variability in data and how certain we want to be that we are correct. • The degree of certainty that we are correct is known as the level of confidence. – Common levels are 90%, 95%, and 99%.
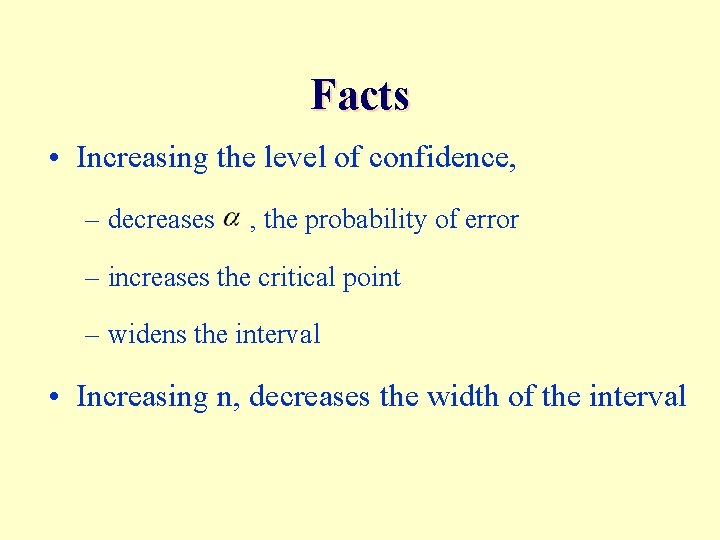
Facts • Increasing the level of confidence, – decreases , the probability of error – increases the critical point – widens the interval • Increasing n, decreases the width of the interval
Insidan region jh
Chebyshev inequality proof
Probability and statistical inference 9th solution pdf
Statistical inference
Inference point
Statistical inference is concerned with
Statistical inference is divided into
Theoretical framework independent dependent variable
Hypothesis in research example
Theoretical framework and hypothesis development
Chapter 20 testing hypotheses about proportions
Theoretical framework for business research
Ruling out rival hypotheses
Analysis of competing hypotheses template
Two types of hypotheses