I Workhop PRIN Politiche dellUnione Europea processi di
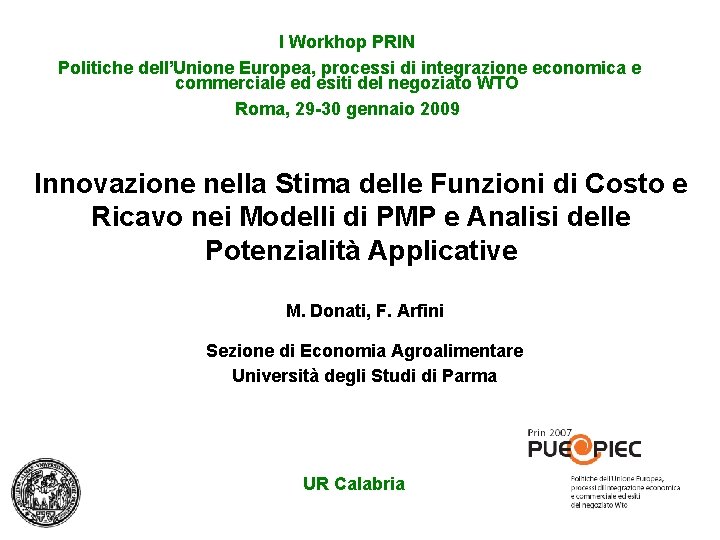
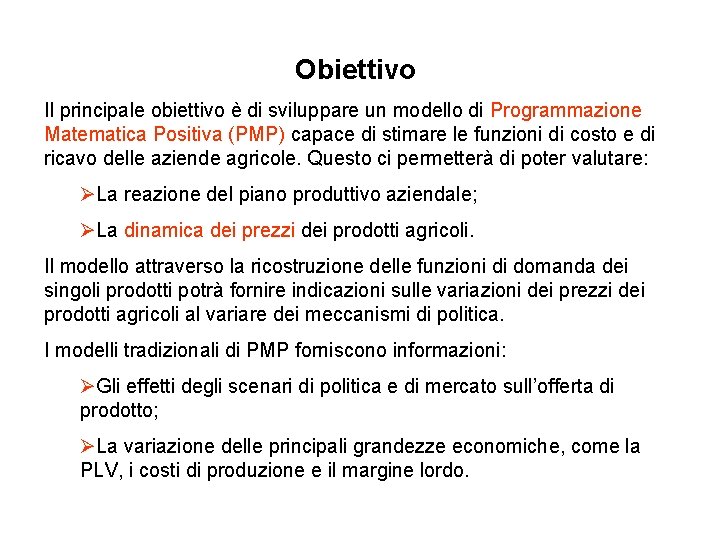
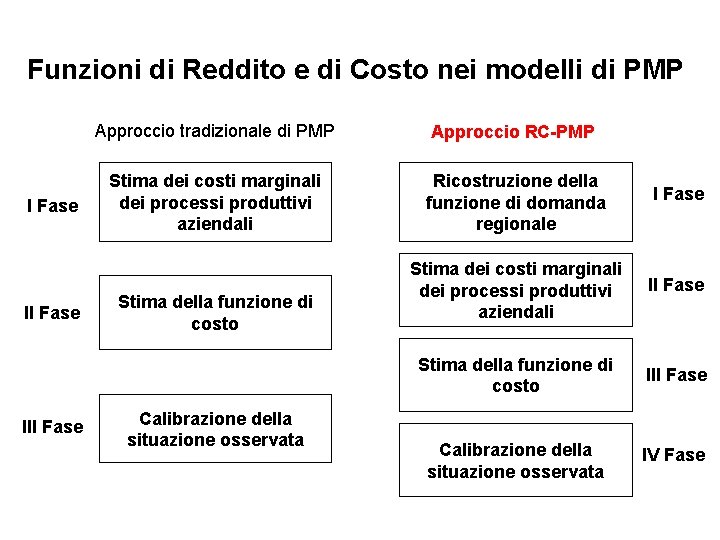
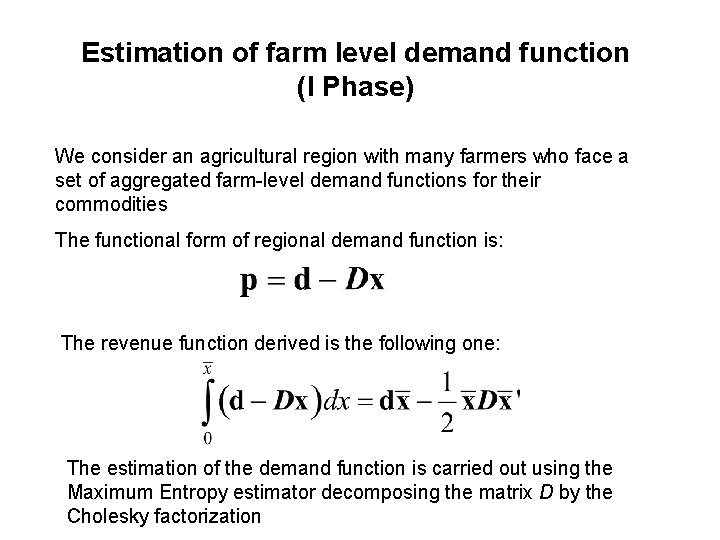
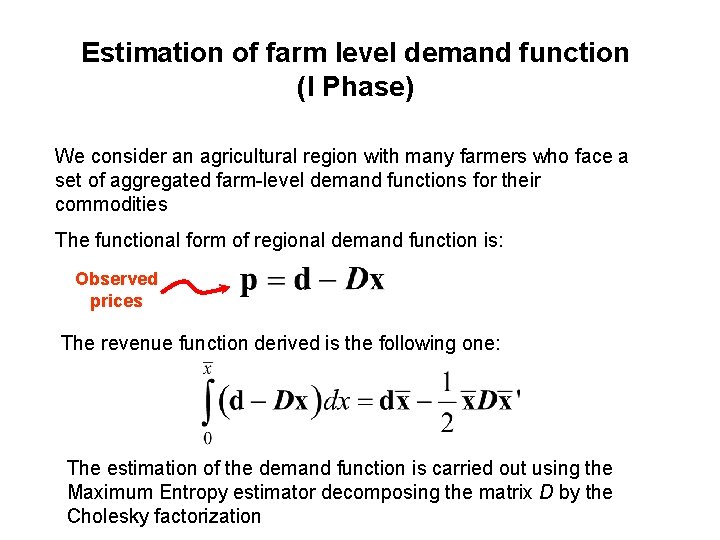
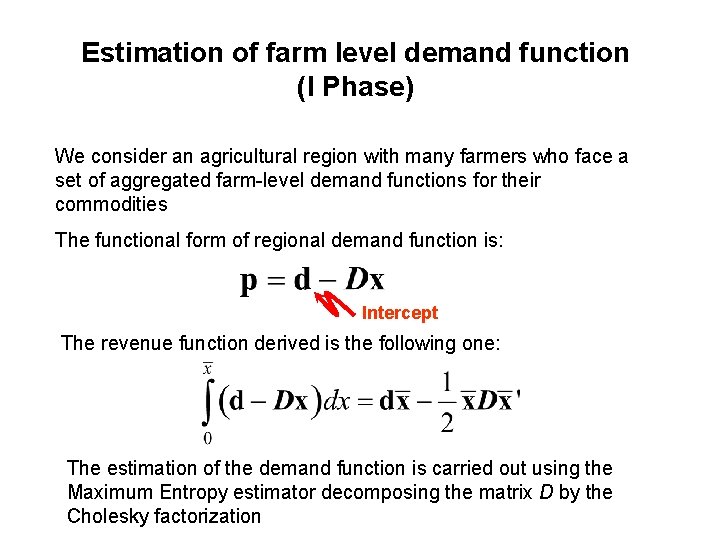
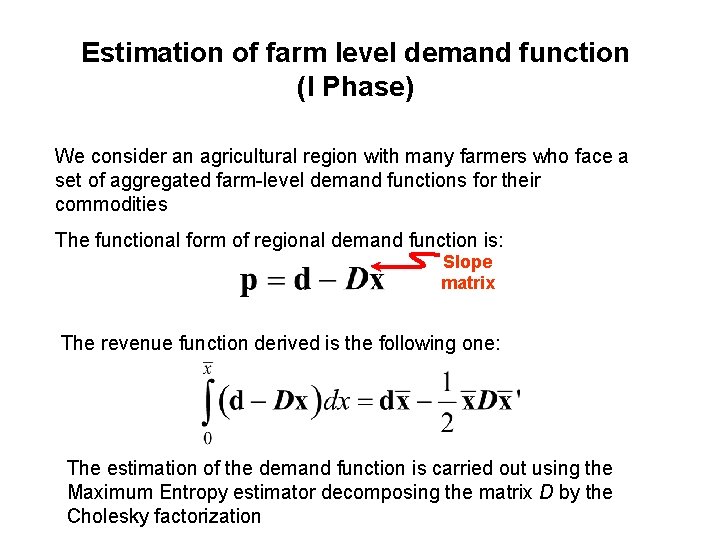
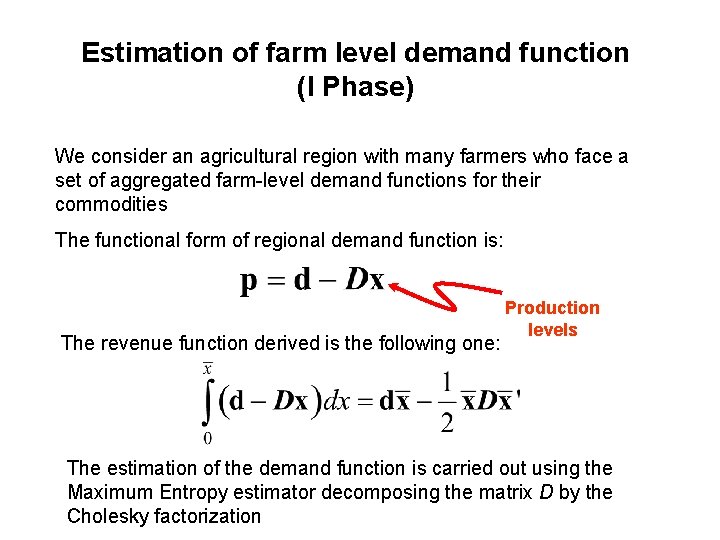
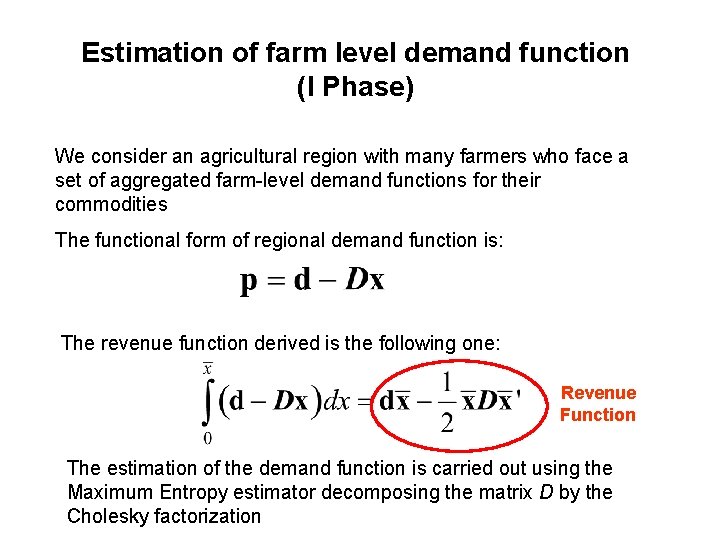
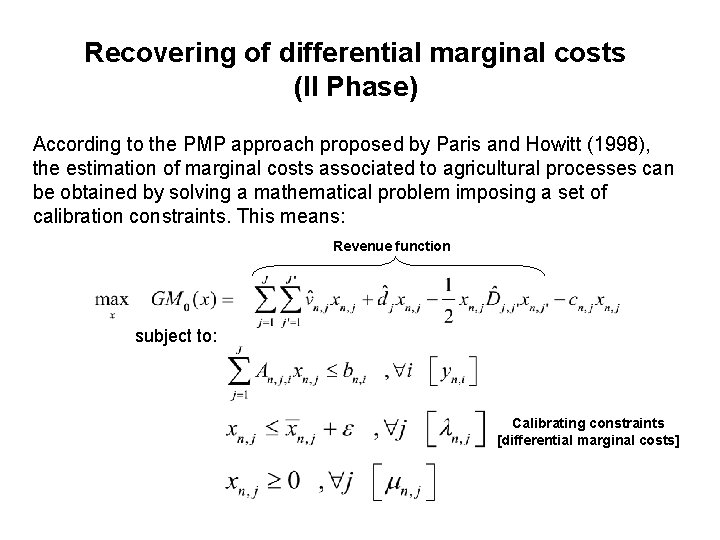
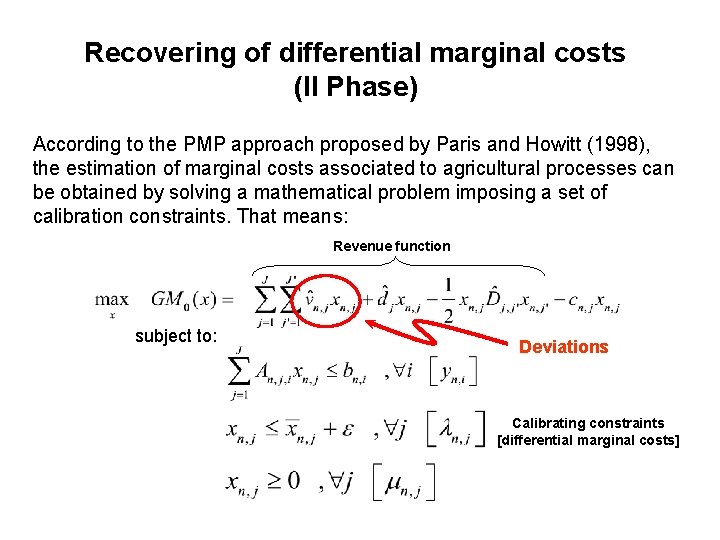
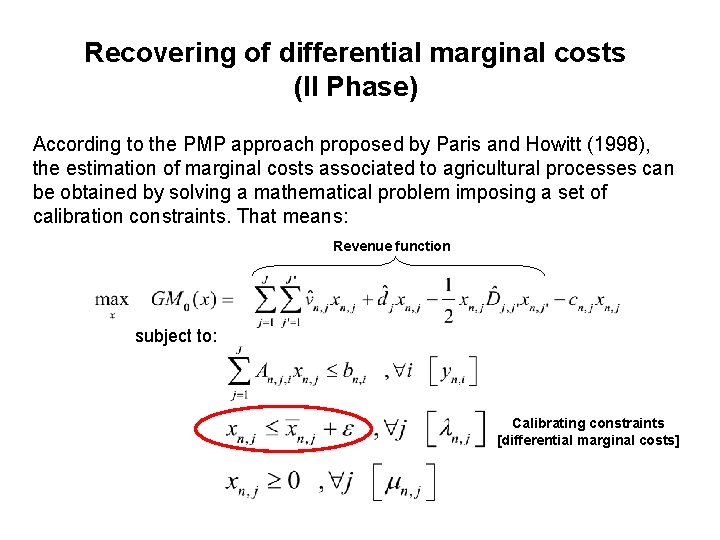
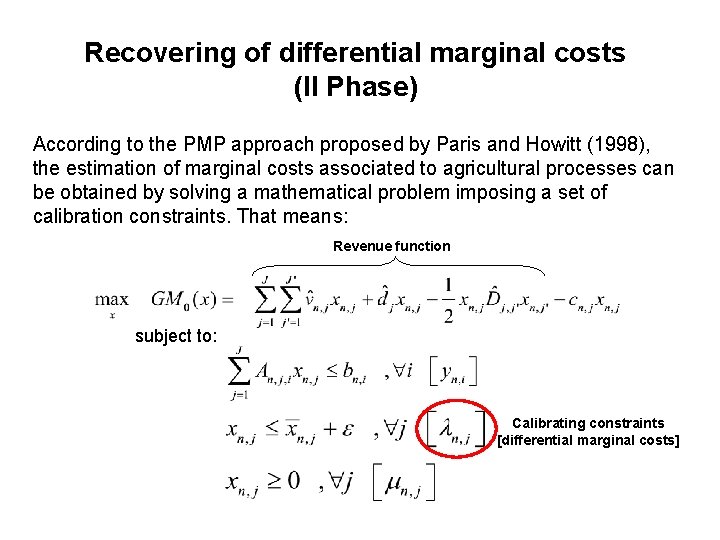
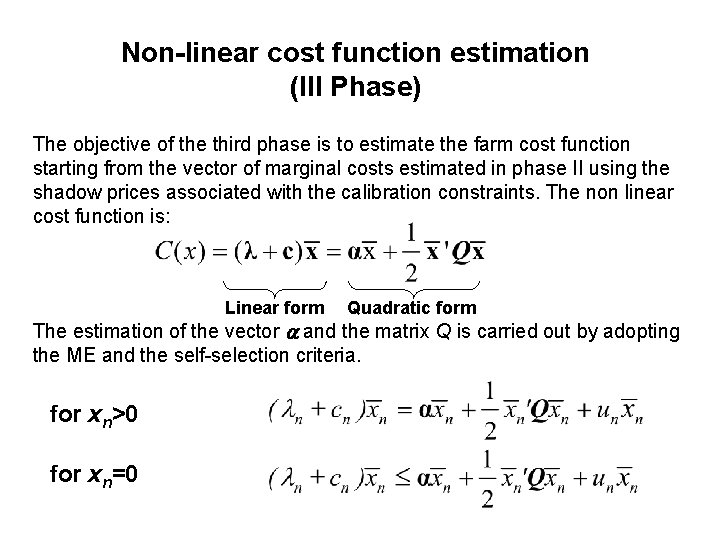
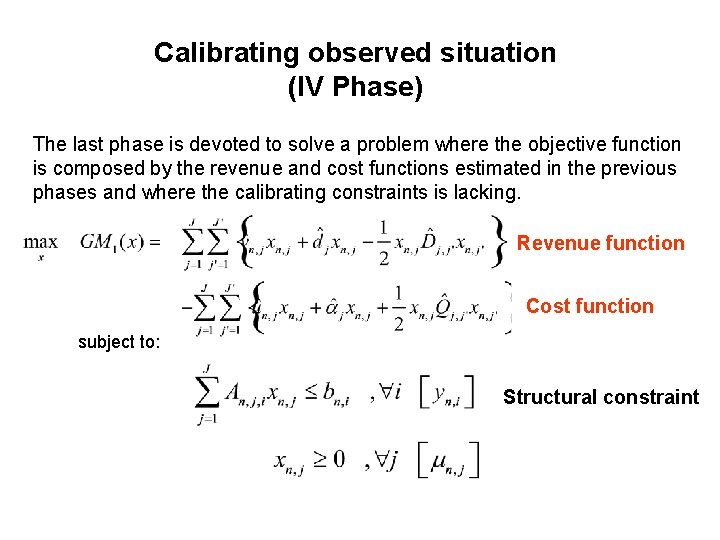
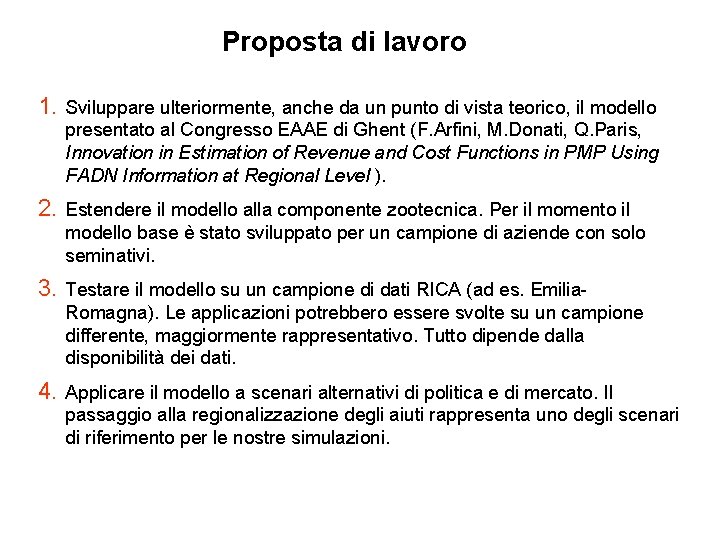
- Slides: 16
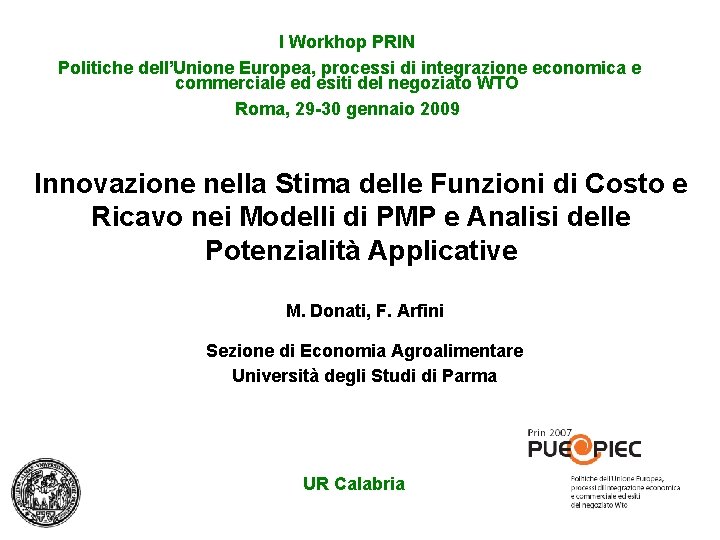
I Workhop PRIN Politiche dell’Unione Europea, processi di integrazione economica e commerciale ed esiti del negoziato WTO Roma, 29 -30 gennaio 2009 Innovazione nella Stima delle Funzioni di Costo e Ricavo nei Modelli di PMP e Analisi delle Potenzialità Applicative M. Donati, F. Arfini Sezione di Economia Agroalimentare Università degli Studi di Parma UR Calabria
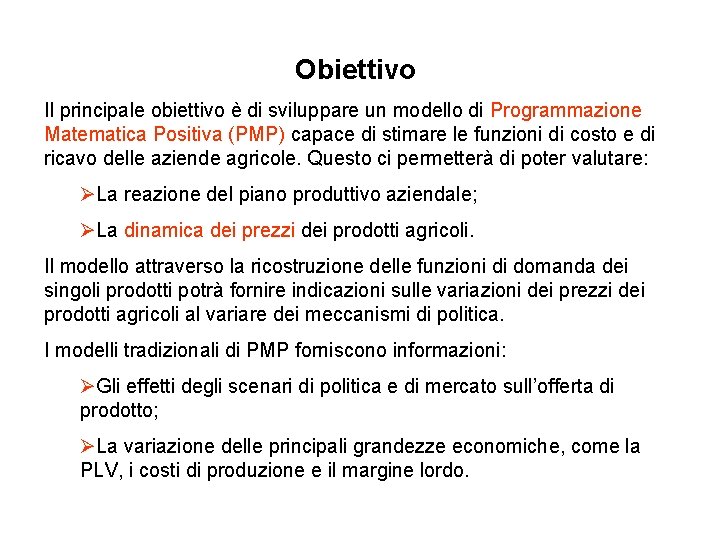
Obiettivo Il principale obiettivo è di sviluppare un modello di Programmazione Matematica Positiva (PMP) capace di stimare le funzioni di costo e di ricavo delle aziende agricole. Questo ci permetterà di poter valutare: ØLa reazione del piano produttivo aziendale; ØLa dinamica dei prezzi dei prodotti agricoli. Il modello attraverso la ricostruzione delle funzioni di domanda dei singoli prodotti potrà fornire indicazioni sulle variazioni dei prezzi dei prodotti agricoli al variare dei meccanismi di politica. I modelli tradizionali di PMP forniscono informazioni: ØGli effetti degli scenari di politica e di mercato sull’offerta di prodotto; ØLa variazione delle principali grandezze economiche, come la PLV, i costi di produzione e il margine lordo.
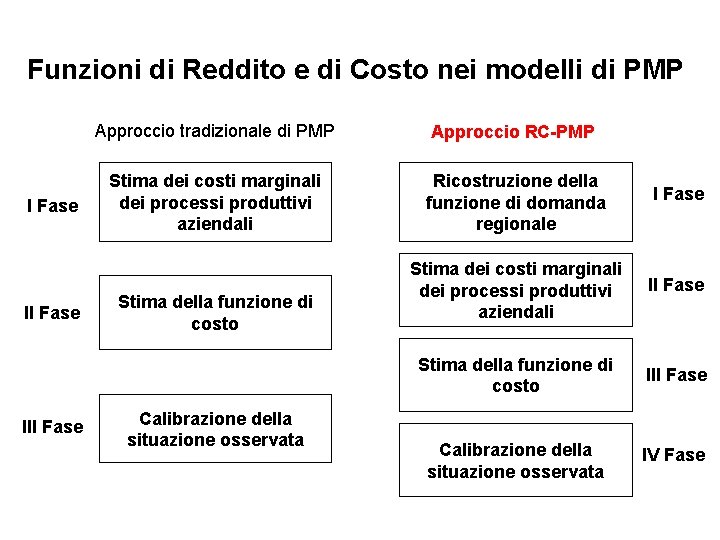
Funzioni di Reddito e di Costo nei modelli di PMP I Fase III Fase Approccio tradizionale di PMP Approccio RC-PMP Stima dei costi marginali dei processi produttivi aziendali Ricostruzione della funzione di domanda regionale I Fase Stima dei costi marginali dei processi produttivi aziendali II Fase Stima della funzione di costo III Fase Calibrazione della situazione osservata IV Fase Stima della funzione di costo Calibrazione della situazione osservata
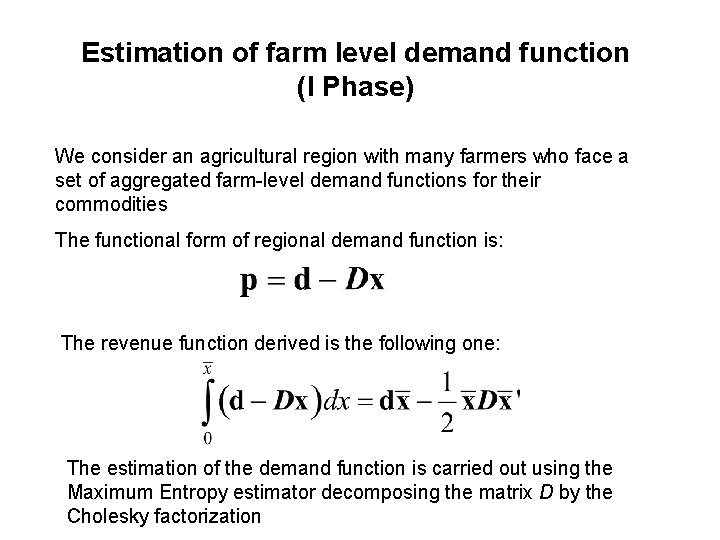
Estimation of farm level demand function (I Phase) We consider an agricultural region with many farmers who face a set of aggregated farm-level demand functions for their commodities The functional form of regional demand function is: The revenue function derived is the following one: The estimation of the demand function is carried out using the Maximum Entropy estimator decomposing the matrix D by the Cholesky factorization
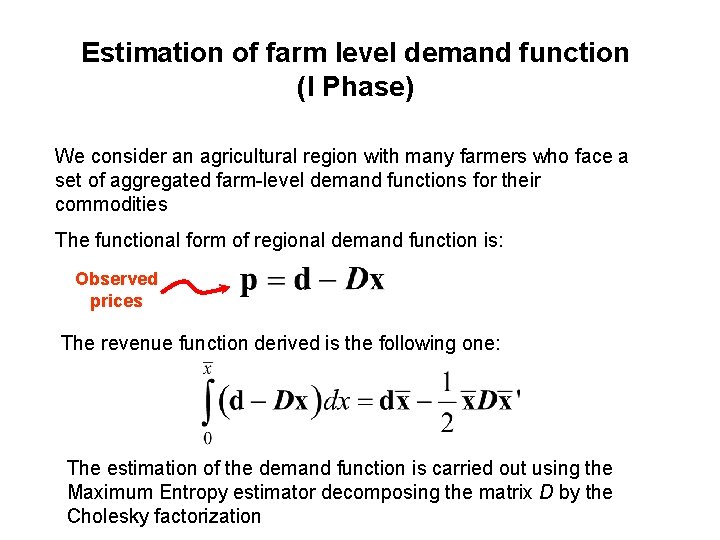
Estimation of farm level demand function (I Phase) We consider an agricultural region with many farmers who face a set of aggregated farm-level demand functions for their commodities The functional form of regional demand function is: Observed prices The revenue function derived is the following one: The estimation of the demand function is carried out using the Maximum Entropy estimator decomposing the matrix D by the Cholesky factorization
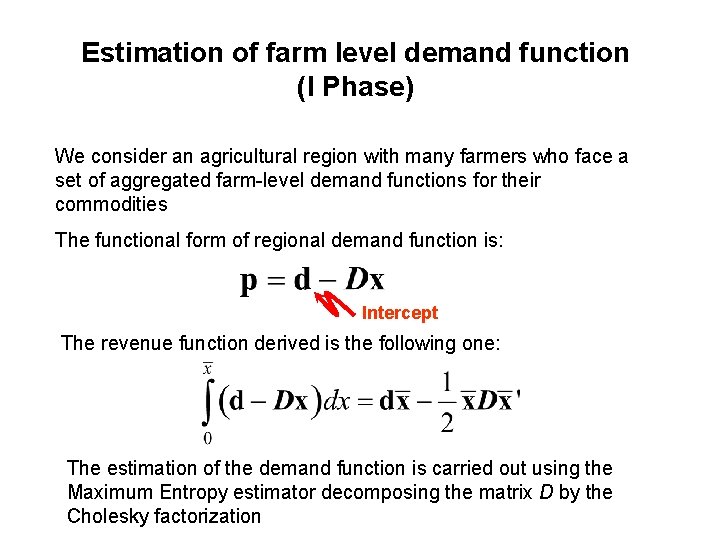
Estimation of farm level demand function (I Phase) We consider an agricultural region with many farmers who face a set of aggregated farm-level demand functions for their commodities The functional form of regional demand function is: Intercept The revenue function derived is the following one: The estimation of the demand function is carried out using the Maximum Entropy estimator decomposing the matrix D by the Cholesky factorization
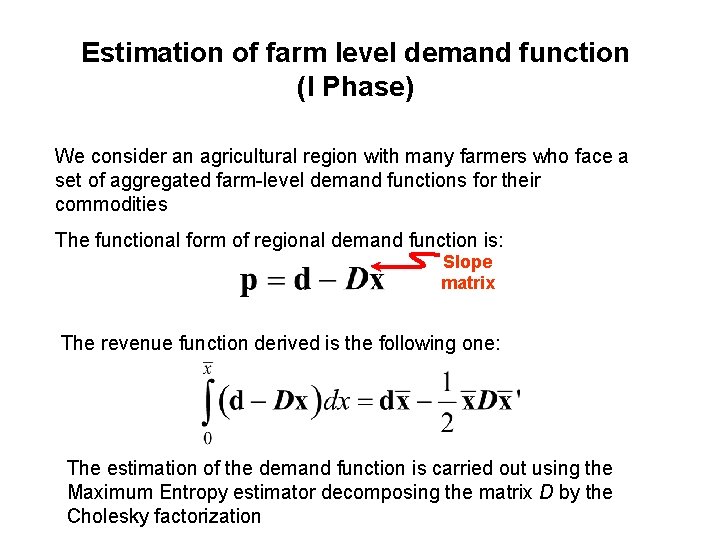
Estimation of farm level demand function (I Phase) We consider an agricultural region with many farmers who face a set of aggregated farm-level demand functions for their commodities The functional form of regional demand function is: Slope matrix The revenue function derived is the following one: The estimation of the demand function is carried out using the Maximum Entropy estimator decomposing the matrix D by the Cholesky factorization
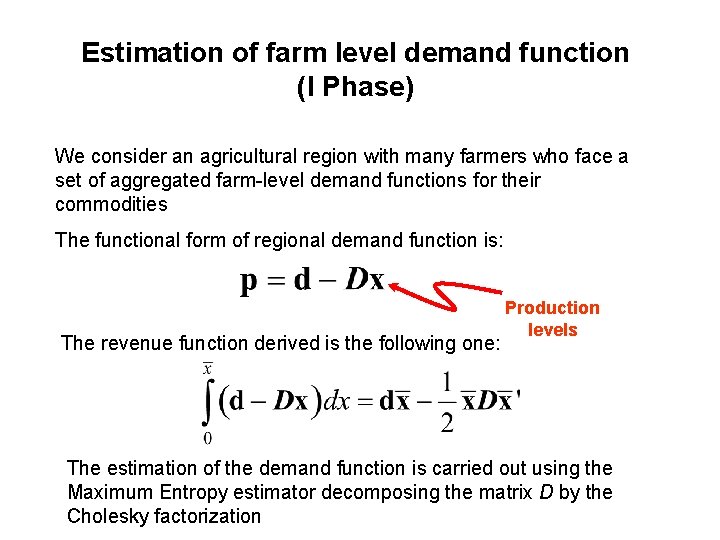
Estimation of farm level demand function (I Phase) We consider an agricultural region with many farmers who face a set of aggregated farm-level demand functions for their commodities The functional form of regional demand function is: The revenue function derived is the following one: Production levels The estimation of the demand function is carried out using the Maximum Entropy estimator decomposing the matrix D by the Cholesky factorization
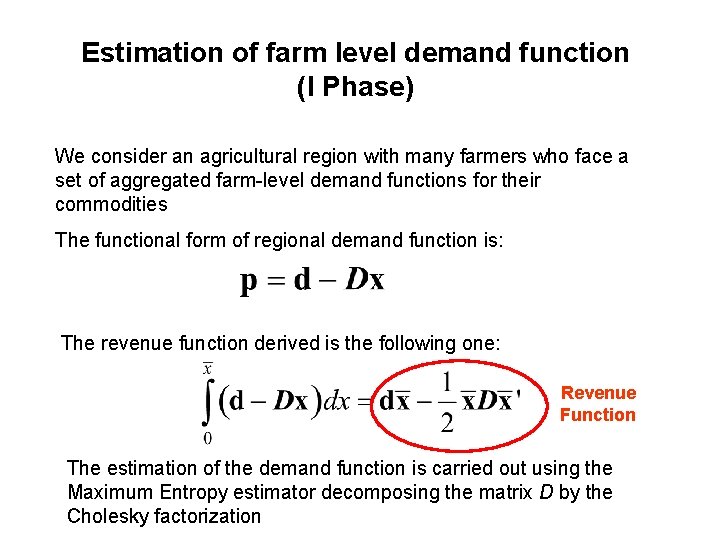
Estimation of farm level demand function (I Phase) We consider an agricultural region with many farmers who face a set of aggregated farm-level demand functions for their commodities The functional form of regional demand function is: The revenue function derived is the following one: Revenue Function The estimation of the demand function is carried out using the Maximum Entropy estimator decomposing the matrix D by the Cholesky factorization
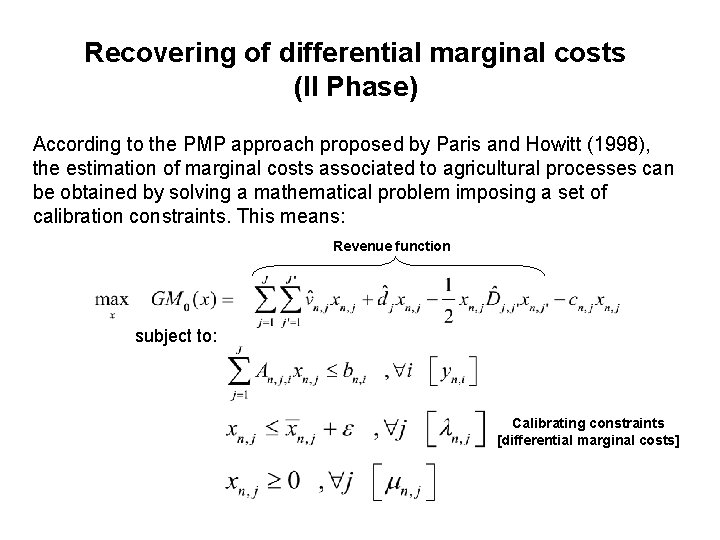
Recovering of differential marginal costs (II Phase) According to the PMP approach proposed by Paris and Howitt (1998), the estimation of marginal costs associated to agricultural processes can be obtained by solving a mathematical problem imposing a set of calibration constraints. This means: Revenue function subject to: Calibrating constraints [differential marginal costs]
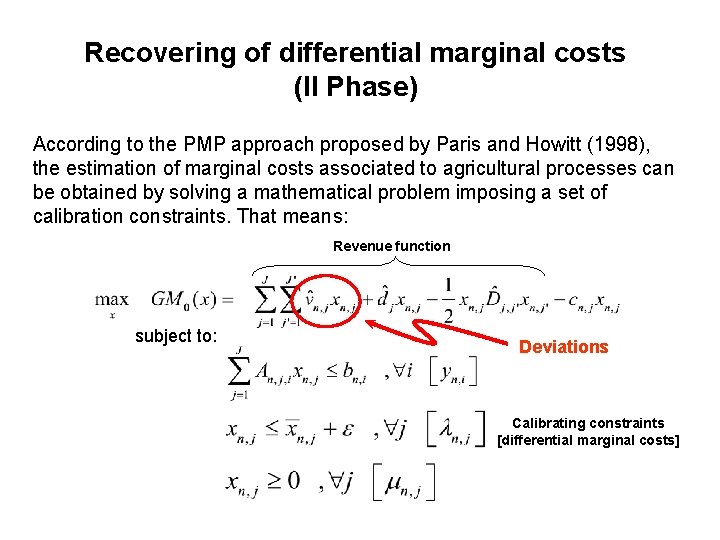
Recovering of differential marginal costs (II Phase) According to the PMP approach proposed by Paris and Howitt (1998), the estimation of marginal costs associated to agricultural processes can be obtained by solving a mathematical problem imposing a set of calibration constraints. That means: Revenue function subject to: Deviations Calibrating constraints [differential marginal costs]
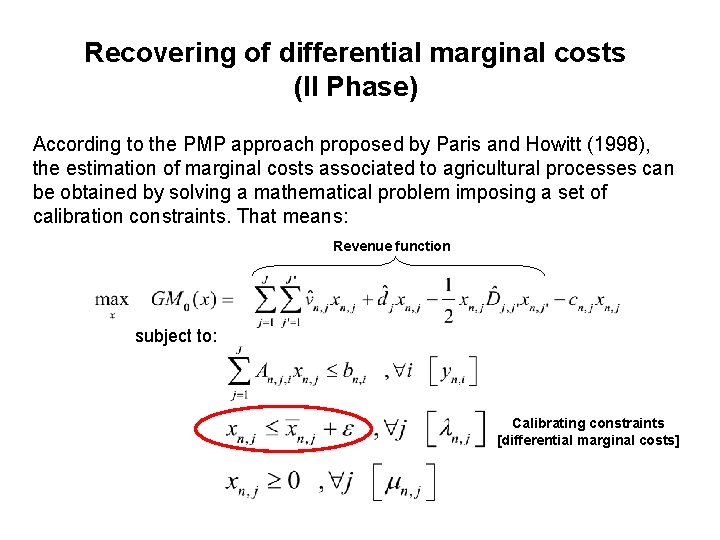
Recovering of differential marginal costs (II Phase) According to the PMP approach proposed by Paris and Howitt (1998), the estimation of marginal costs associated to agricultural processes can be obtained by solving a mathematical problem imposing a set of calibration constraints. That means: Revenue function subject to: Calibrating constraints [differential marginal costs]
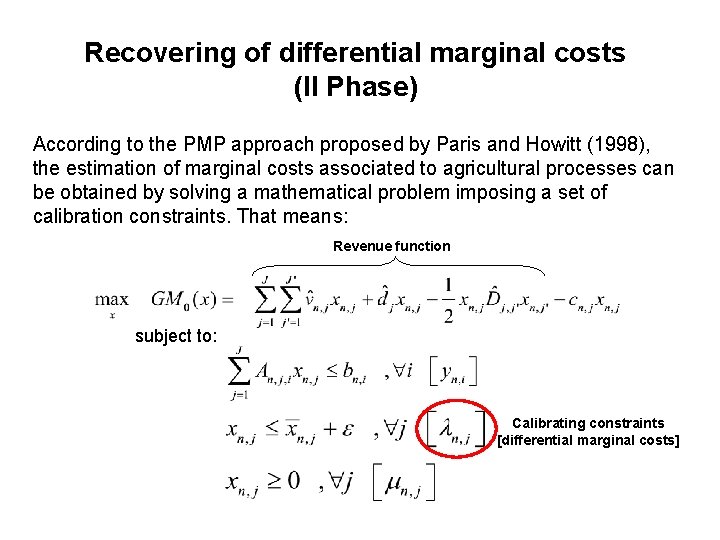
Recovering of differential marginal costs (II Phase) According to the PMP approach proposed by Paris and Howitt (1998), the estimation of marginal costs associated to agricultural processes can be obtained by solving a mathematical problem imposing a set of calibration constraints. That means: Revenue function subject to: Calibrating constraints [differential marginal costs]
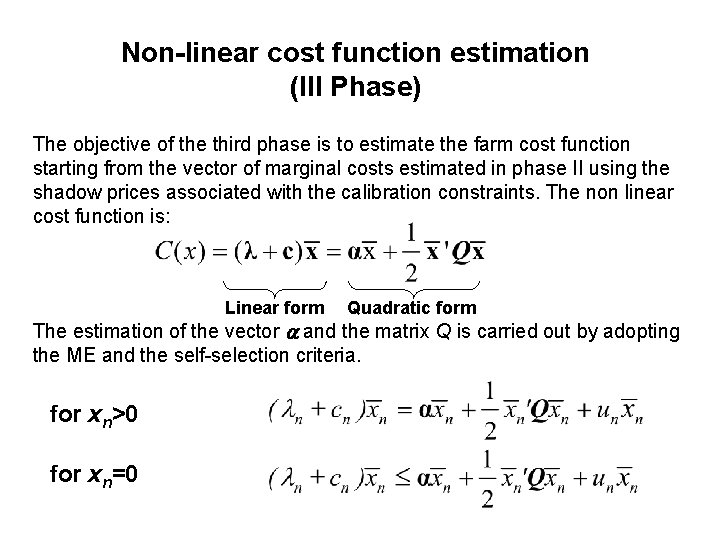
Non-linear cost function estimation (III Phase) The objective of the third phase is to estimate the farm cost function starting from the vector of marginal costs estimated in phase II using the shadow prices associated with the calibration constraints. The non linear cost function is: Linear form Quadratic form The estimation of the vector and the matrix Q is carried out by adopting the ME and the self-selection criteria. for xn>0 for xn=0
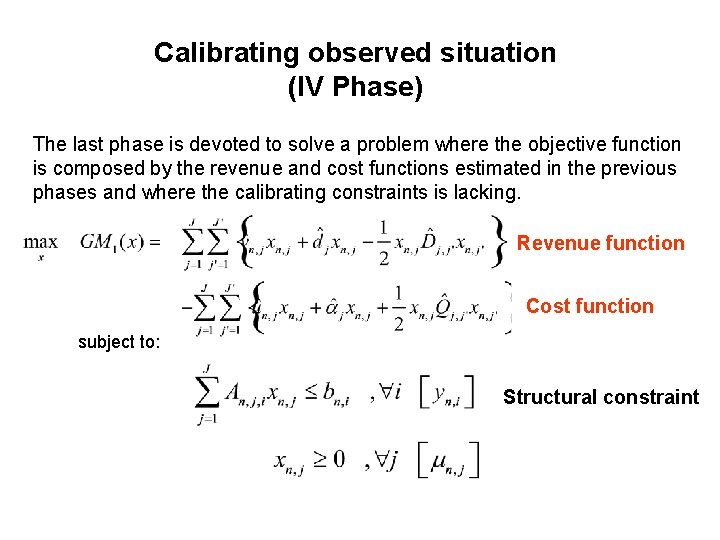
Calibrating observed situation (IV Phase) The last phase is devoted to solve a problem where the objective function is composed by the revenue and cost functions estimated in the previous phases and where the calibrating constraints is lacking. Revenue function Cost function subject to: Structural constraint
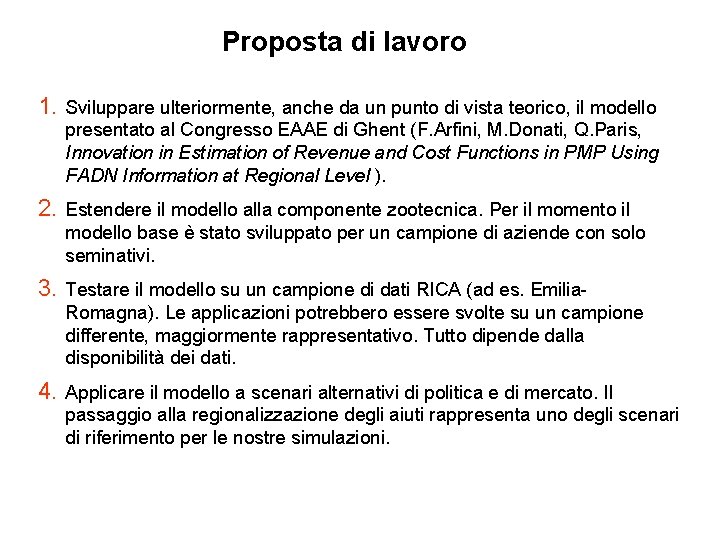
Proposta di lavoro 1. Sviluppare ulteriormente, anche da un punto di vista teorico, il modello presentato al Congresso EAAE di Ghent (F. Arfini, M. Donati, Q. Paris, Innovation in Estimation of Revenue and Cost Functions in PMP Using FADN Information at Regional Level ). 2. Estendere il modello alla componente zootecnica. Per il momento il modello base è stato sviluppato per un campione di aziende con solo seminativi. 3. Testare il modello su un campione di dati RICA (ad es. Emilia. Romagna). Le applicazioni potrebbero essere svolte su un campione differente, maggiormente rappresentativo. Tutto dipende dalla disponibilità dei dati. 4. Applicare il modello a scenari alternativi di politica e di mercato. Il passaggio alla regionalizzazione degli aiuti rappresenta uno degli scenari di riferimento per le nostre simulazioni.