HYPOTHESIS TESTING Definition The Hypothesis Testing is a
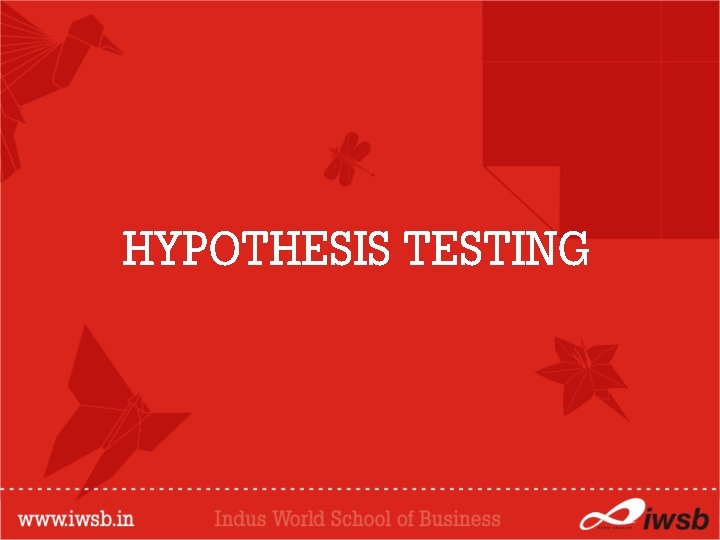
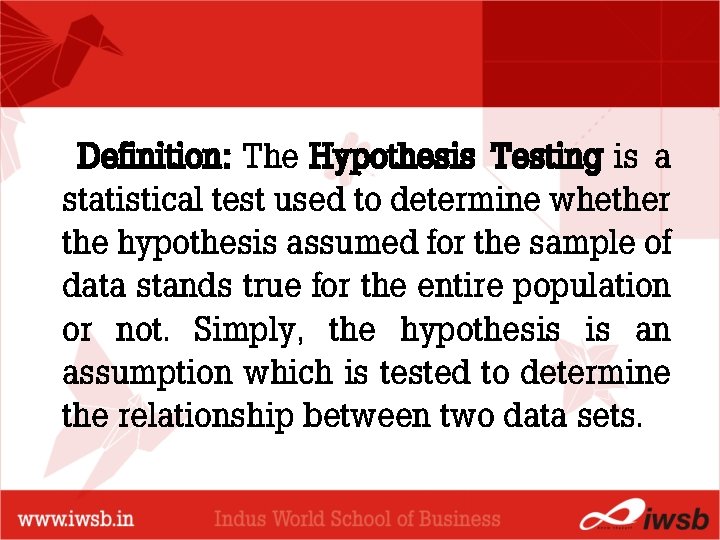
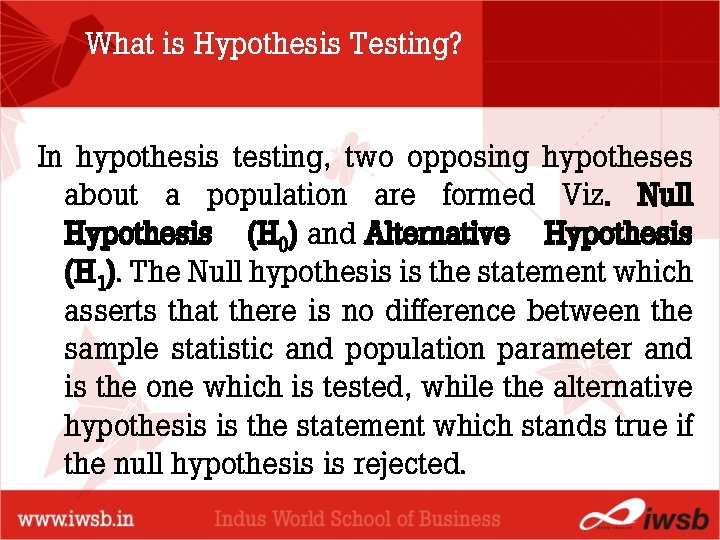
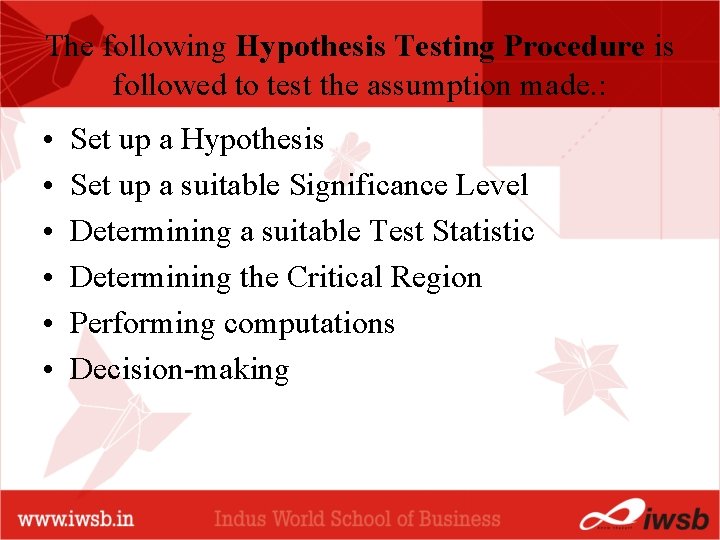
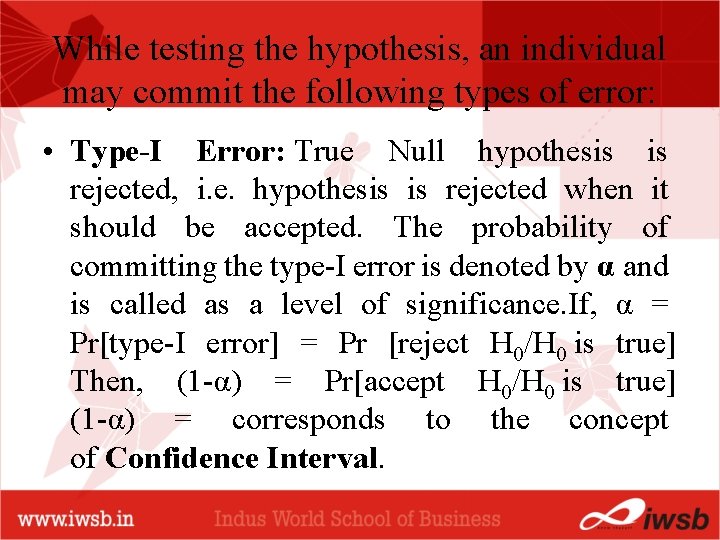
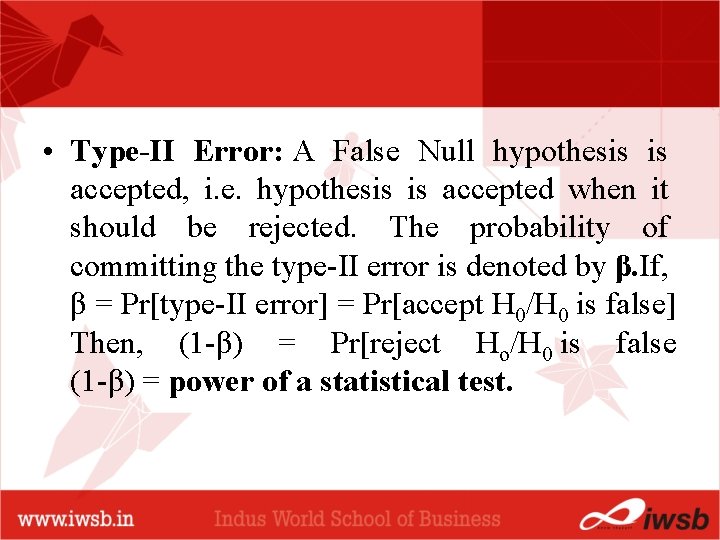
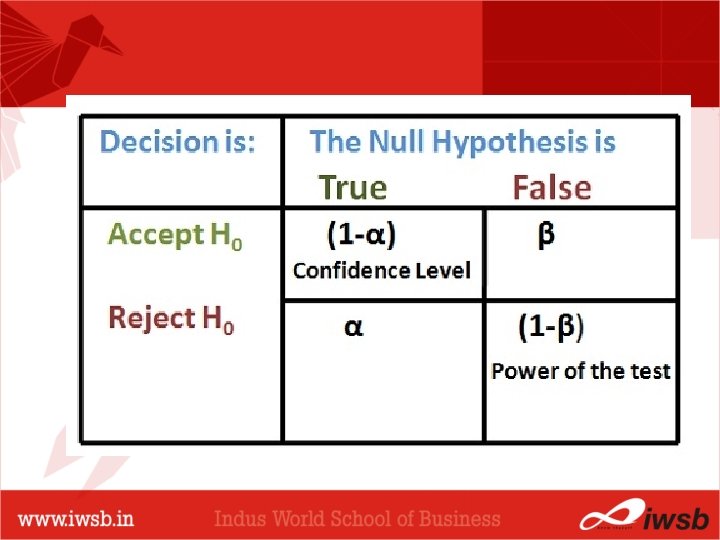
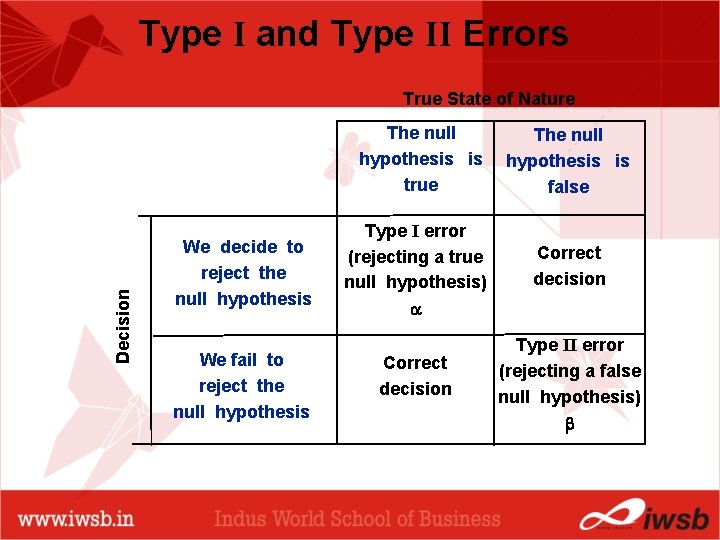
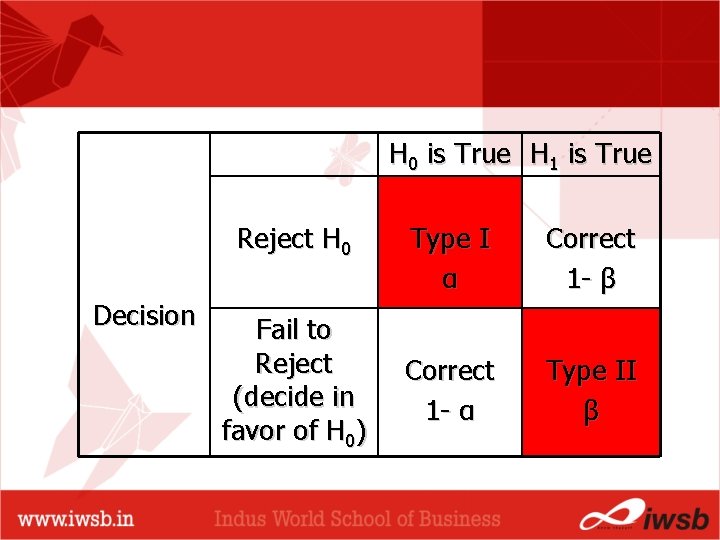
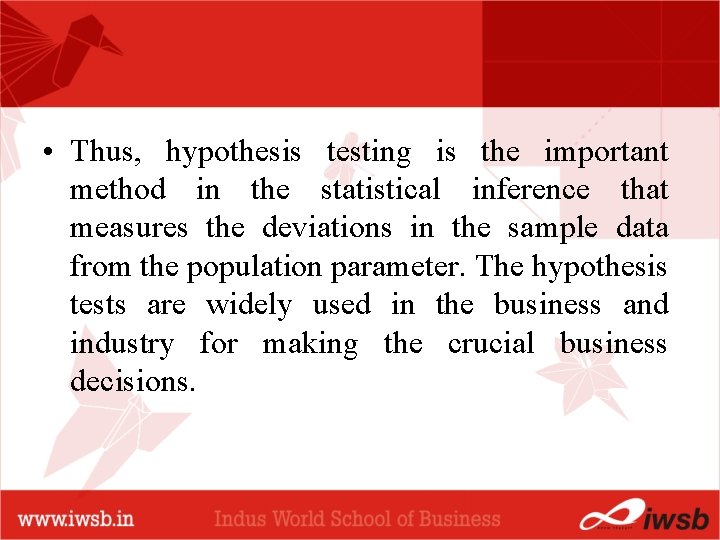
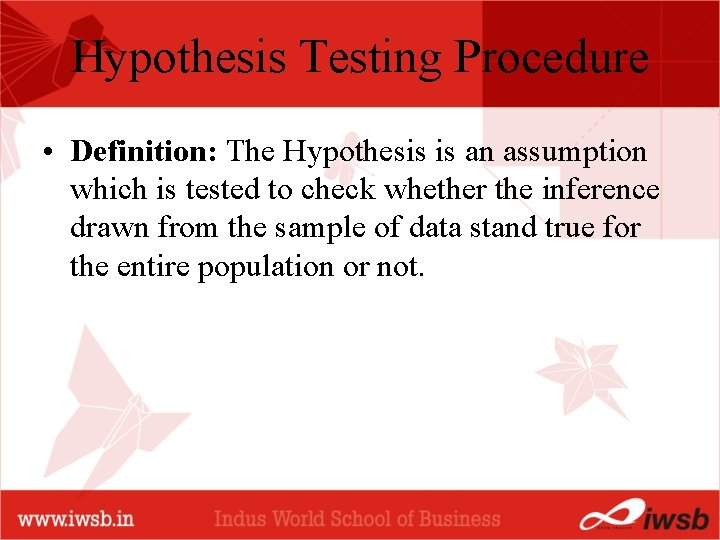
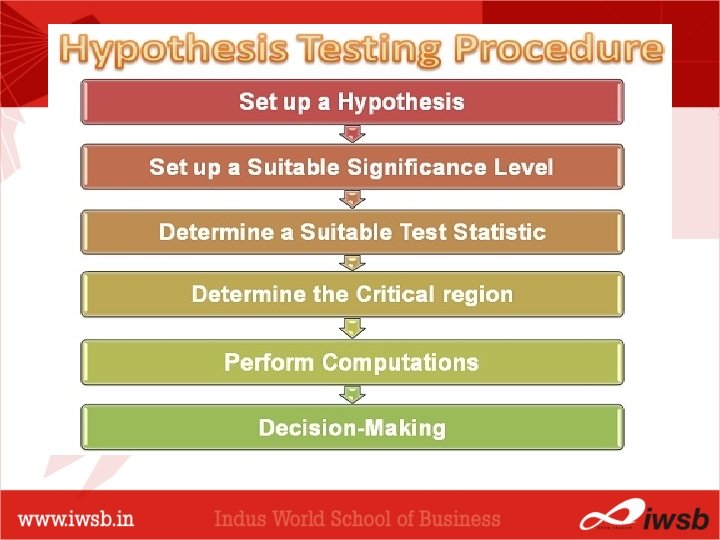
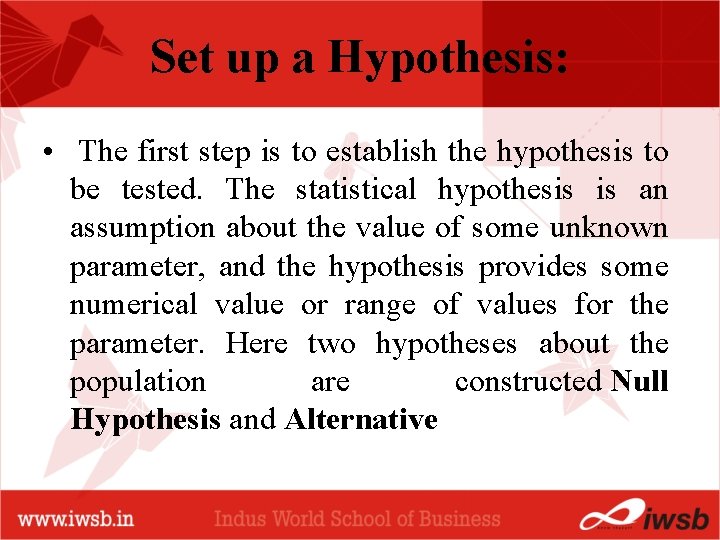
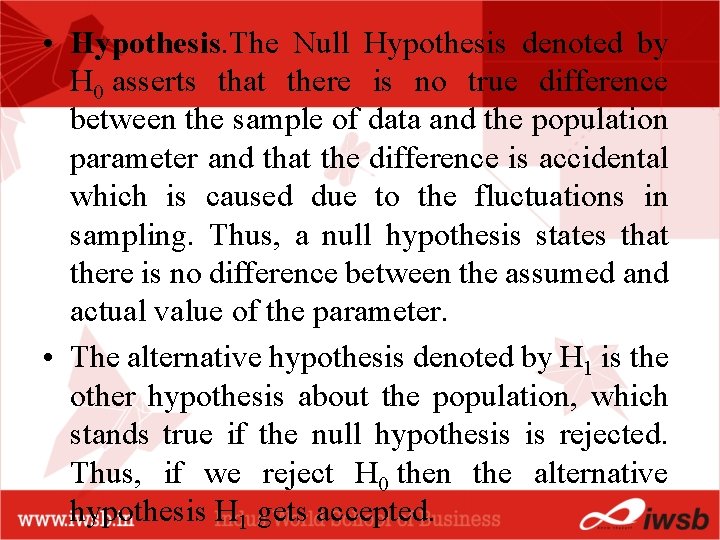
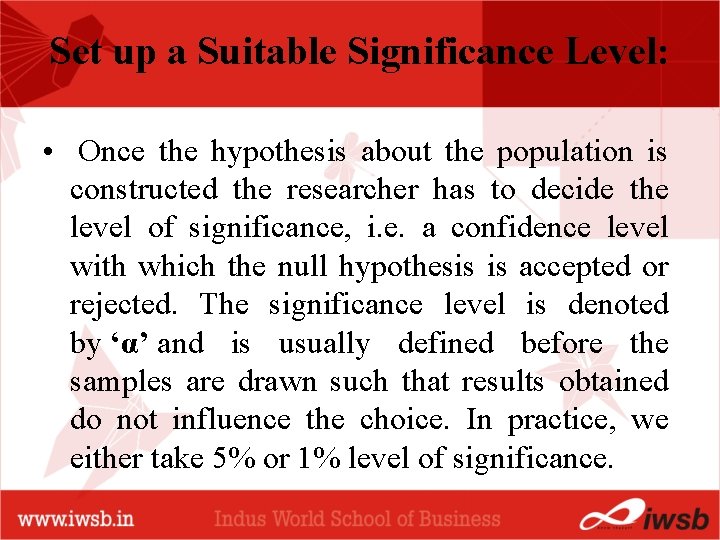
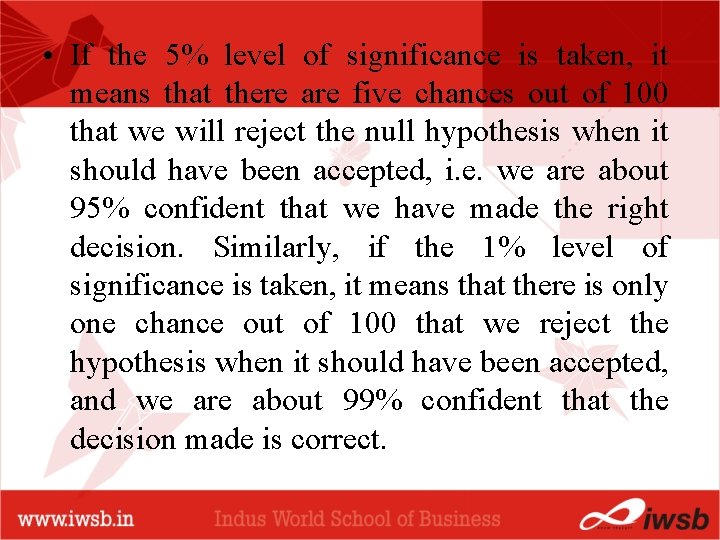
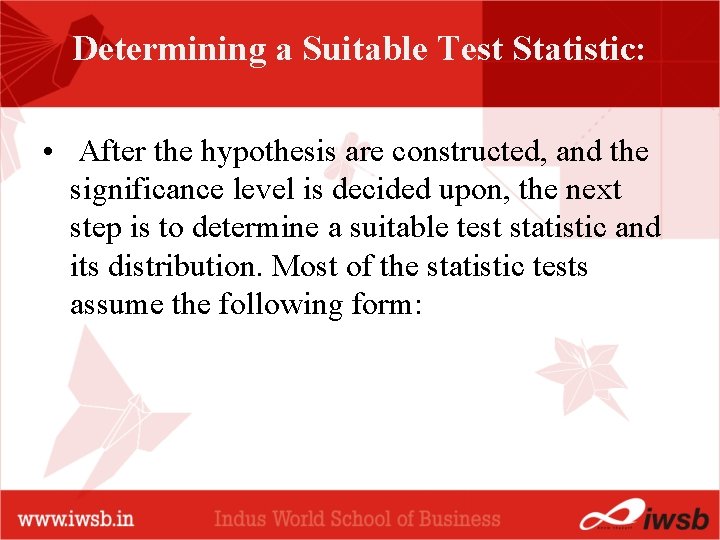
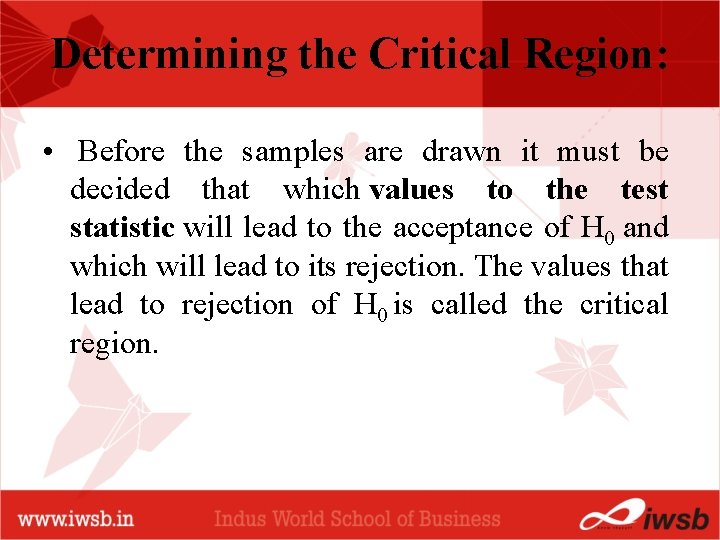
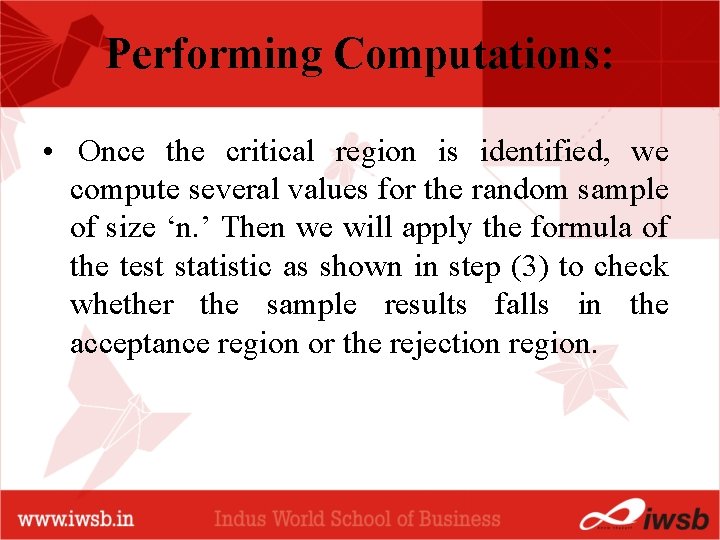
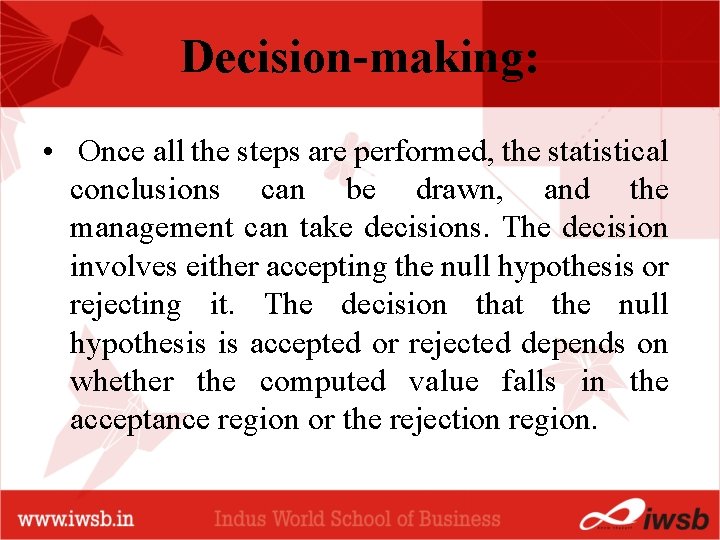
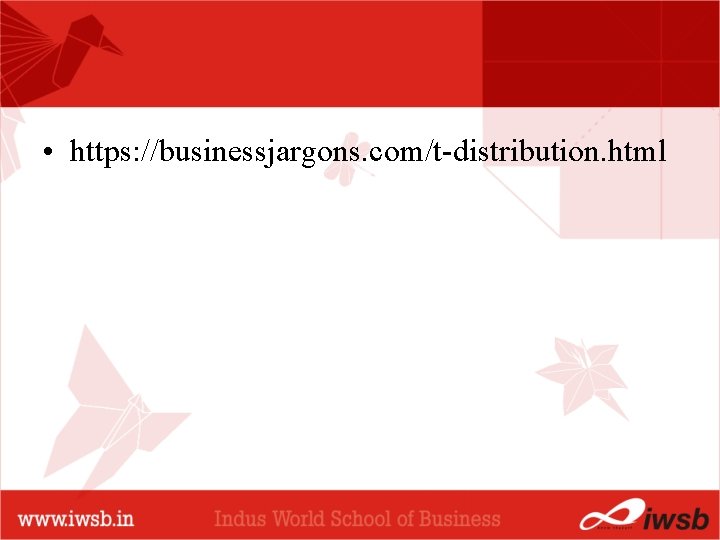
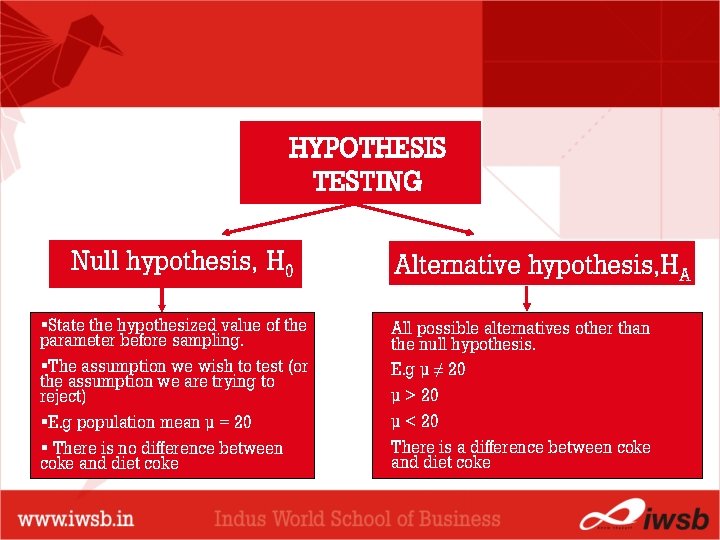
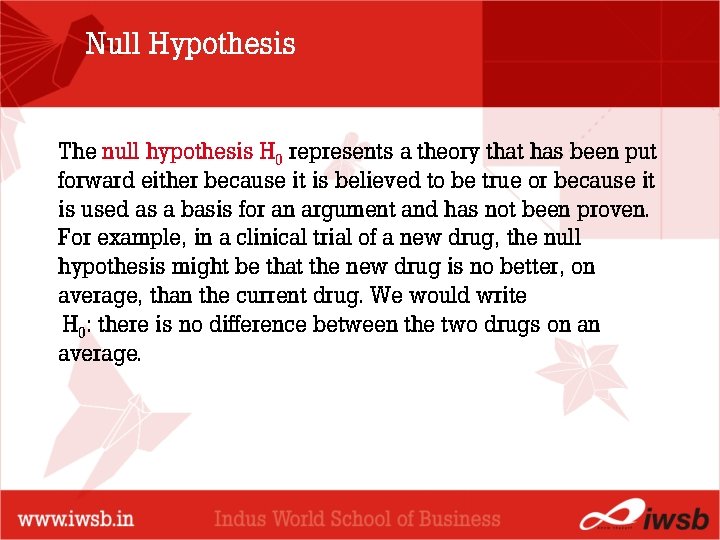
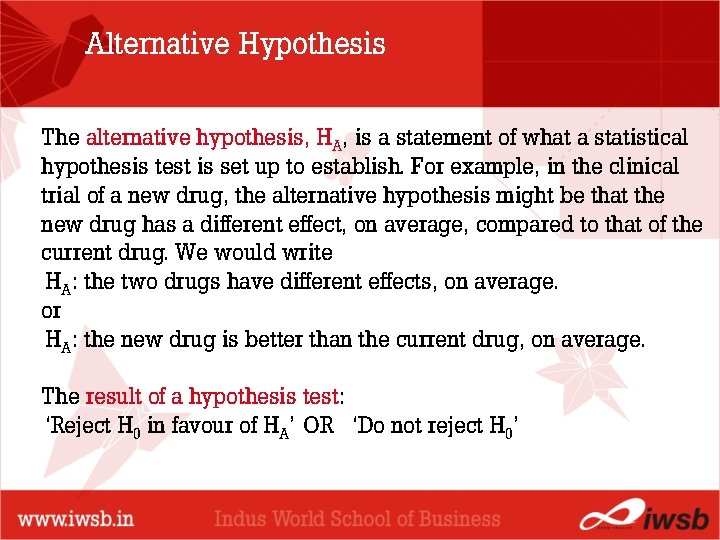
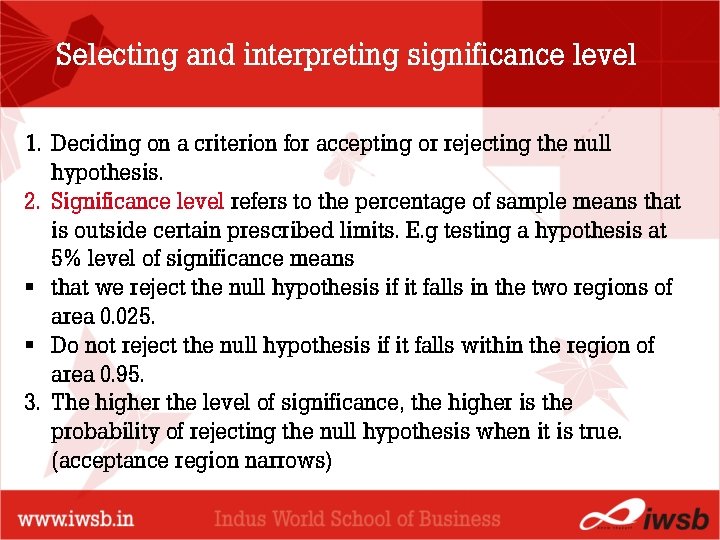
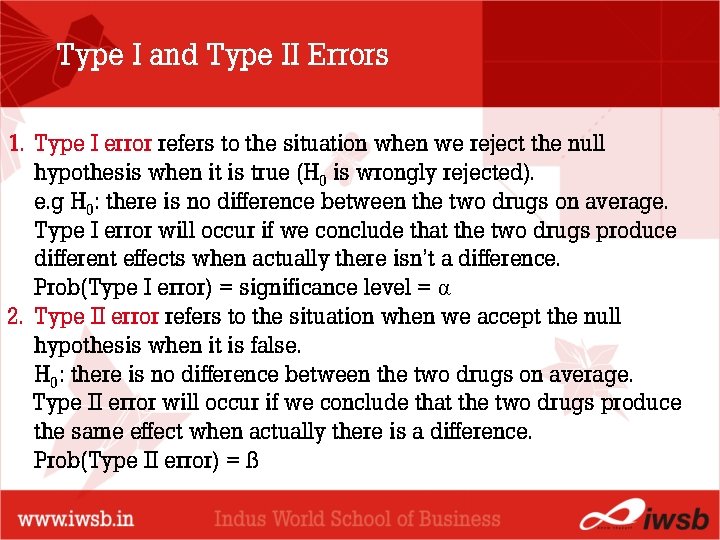
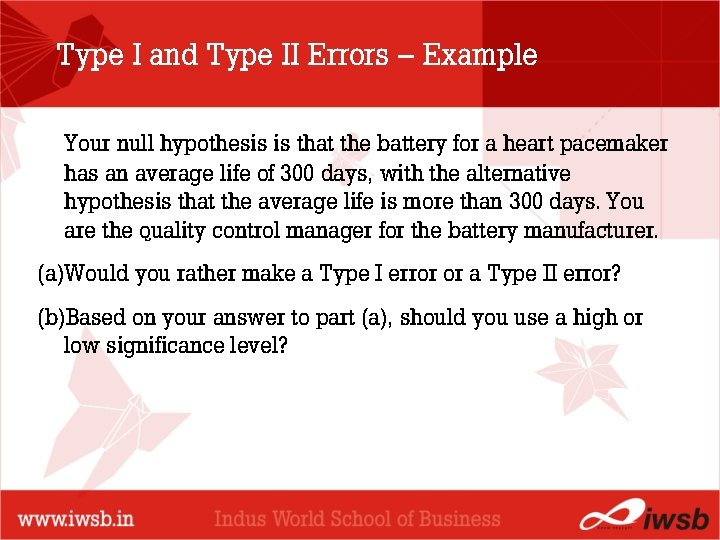
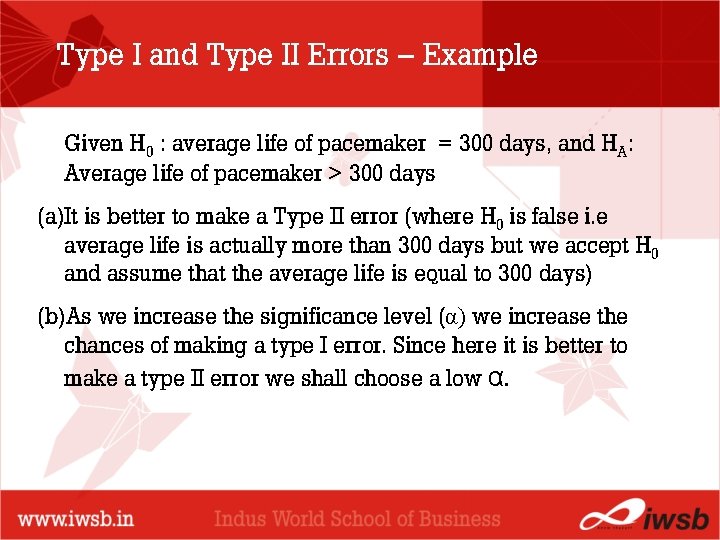
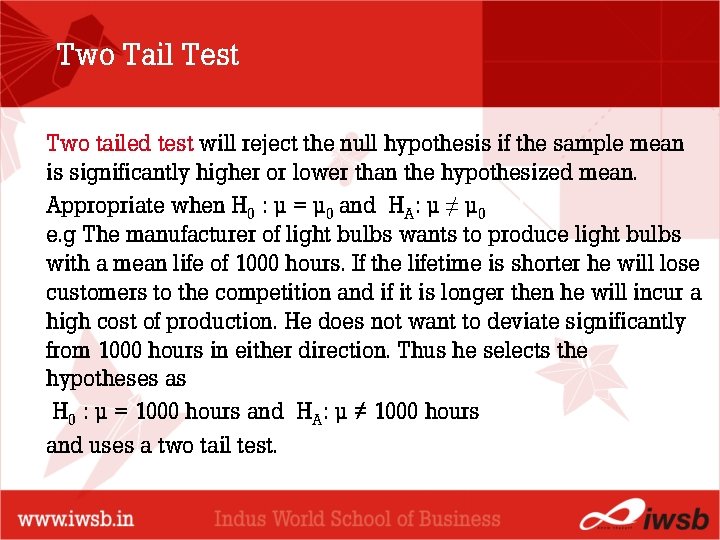
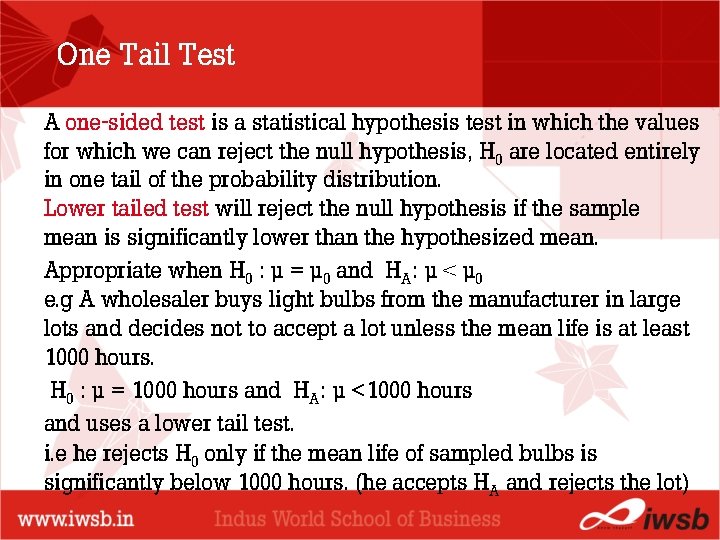
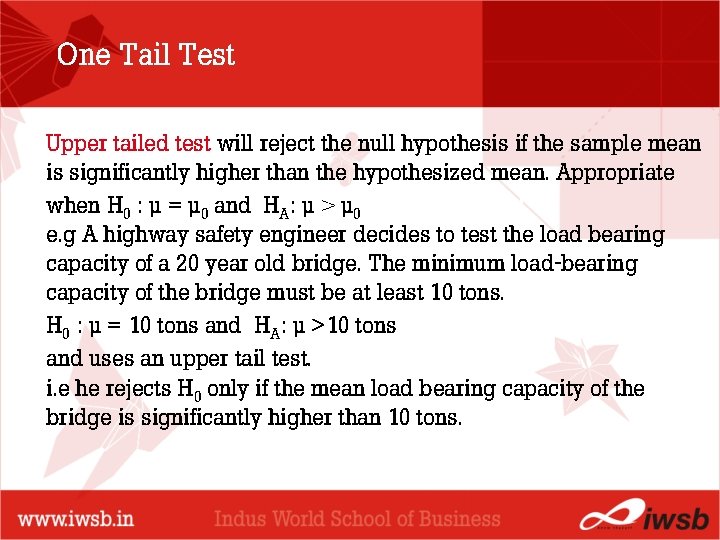
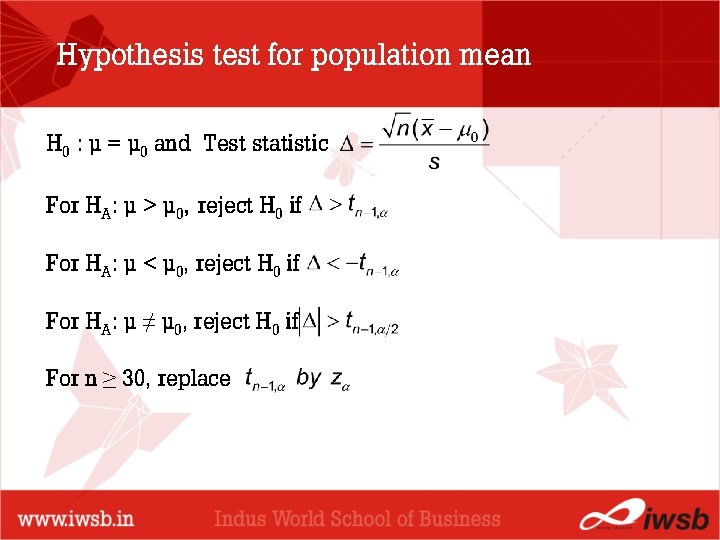
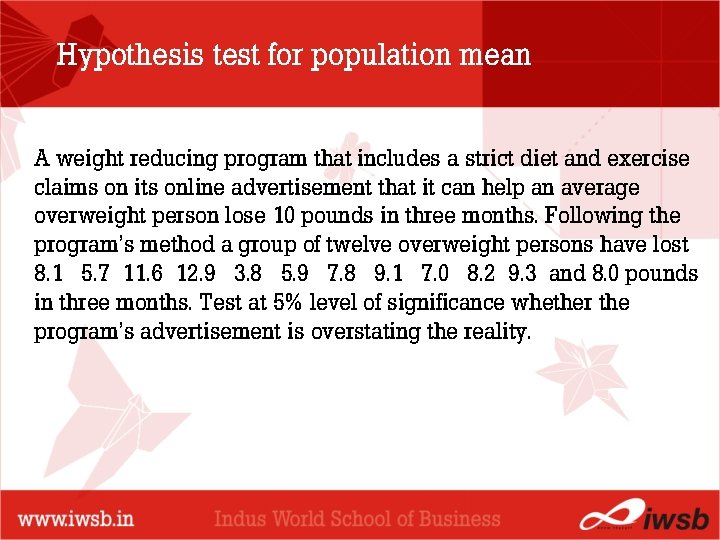
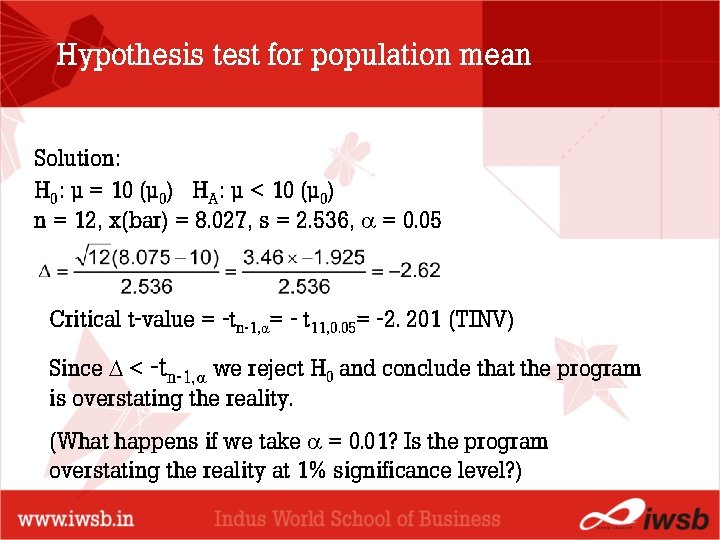
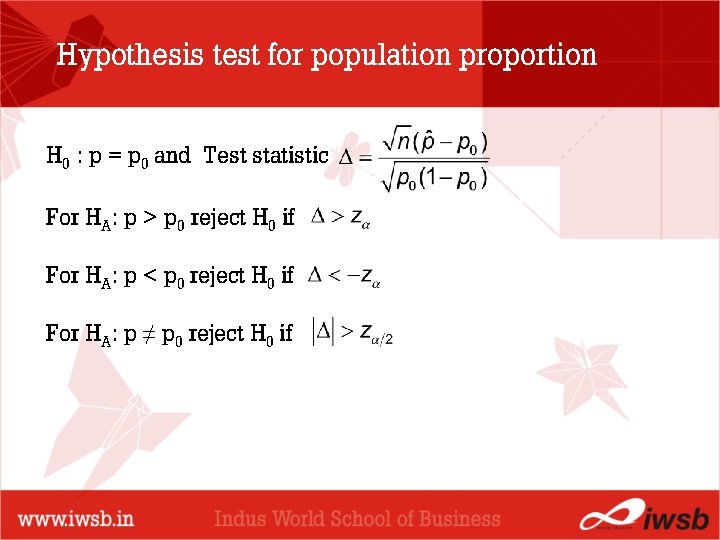
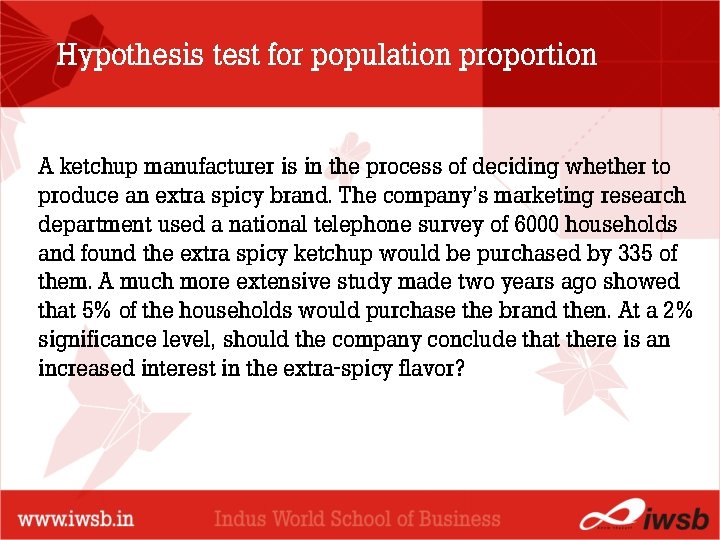
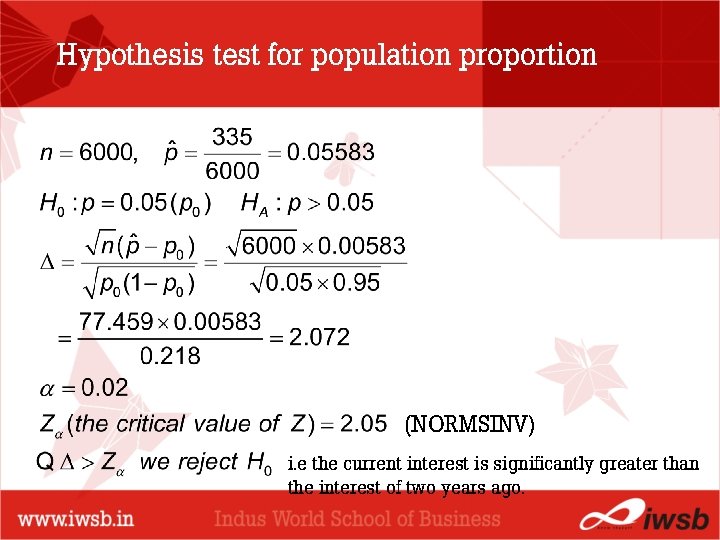
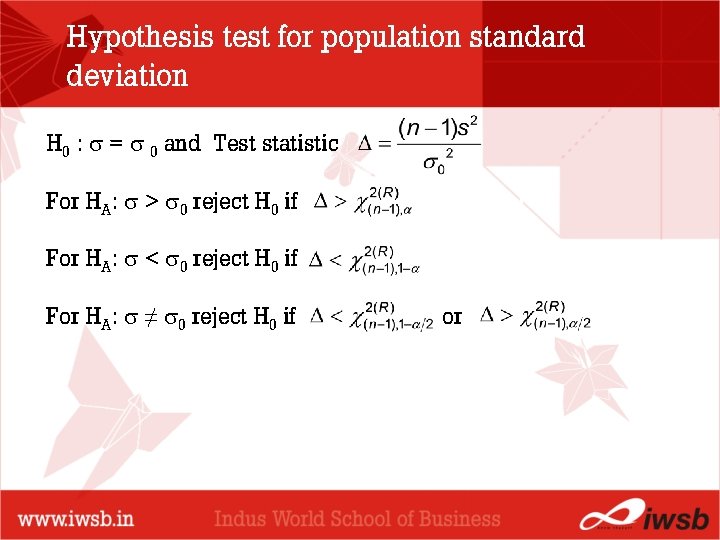
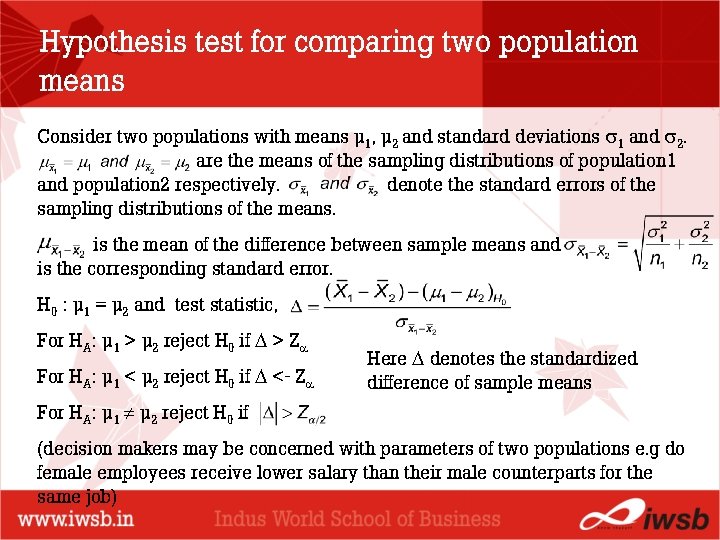
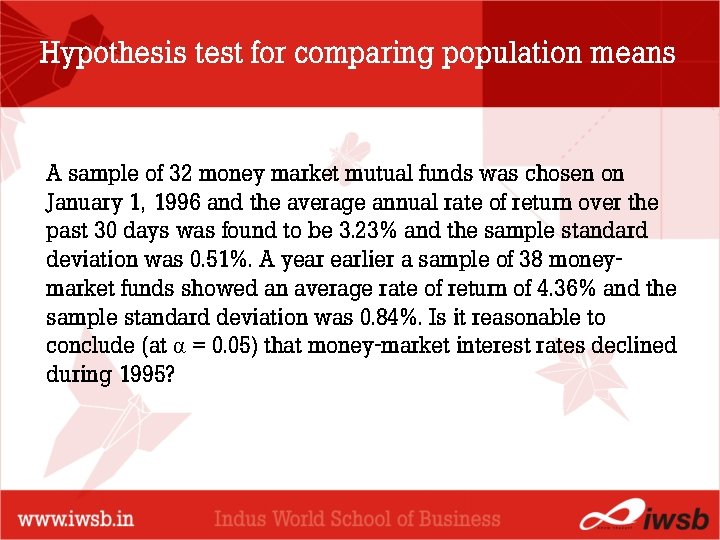
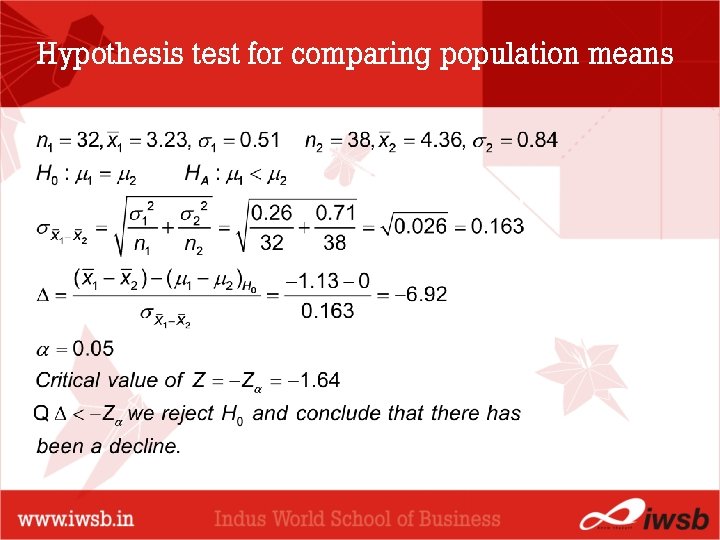
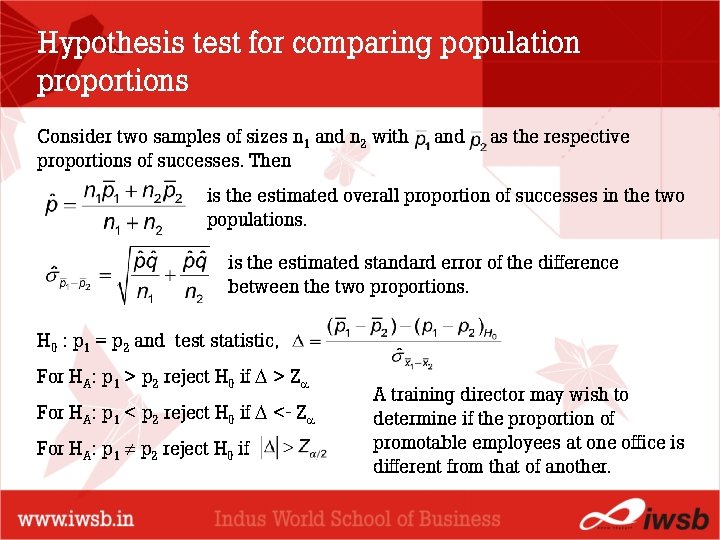
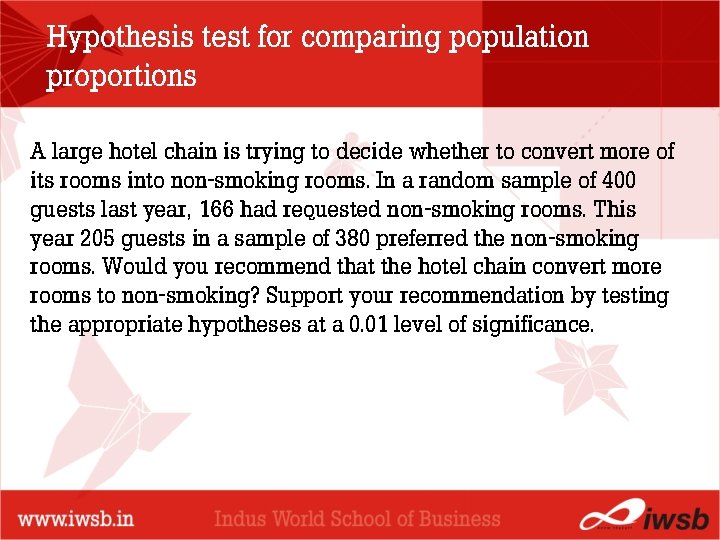
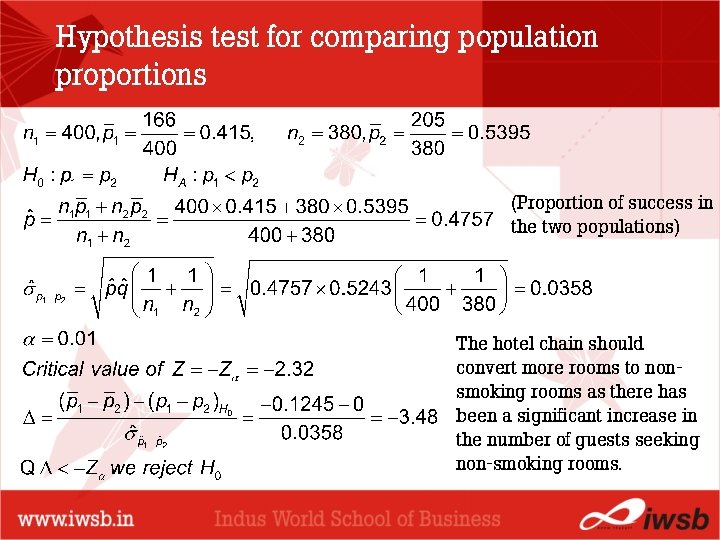
- Slides: 44
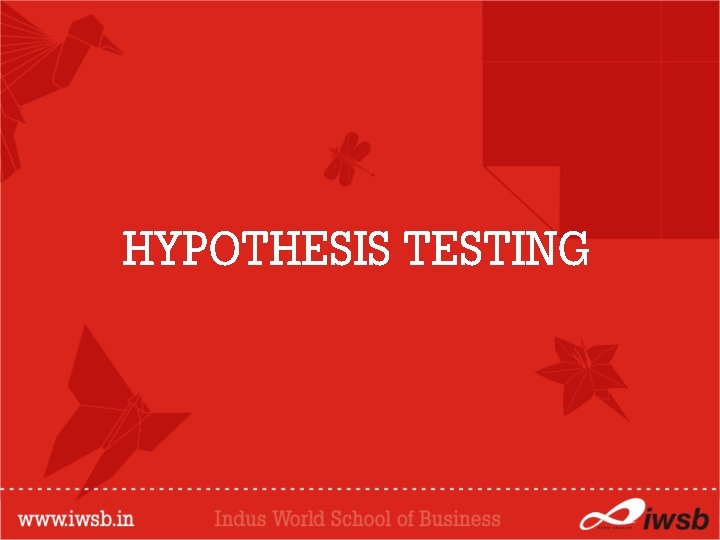
HYPOTHESIS TESTING
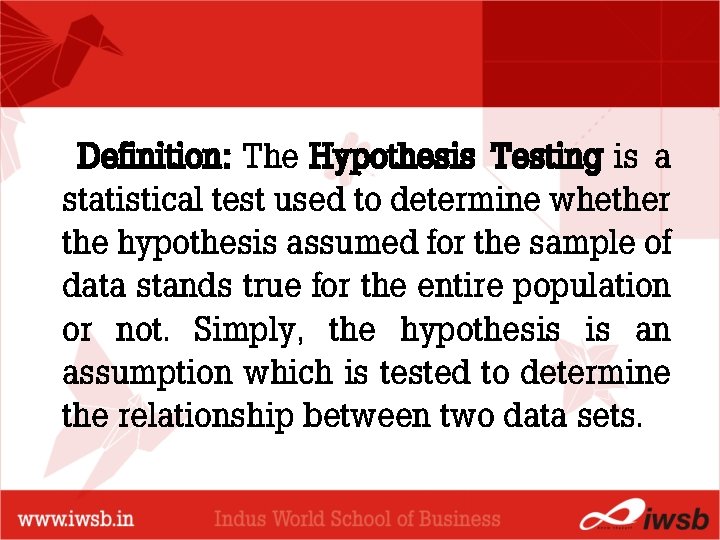
Definition: The Hypothesis Testing is a statistical test used to determine whether the hypothesis assumed for the sample of data stands true for the entire population or not. Simply, the hypothesis is an assumption which is tested to determine the relationship between two data sets.
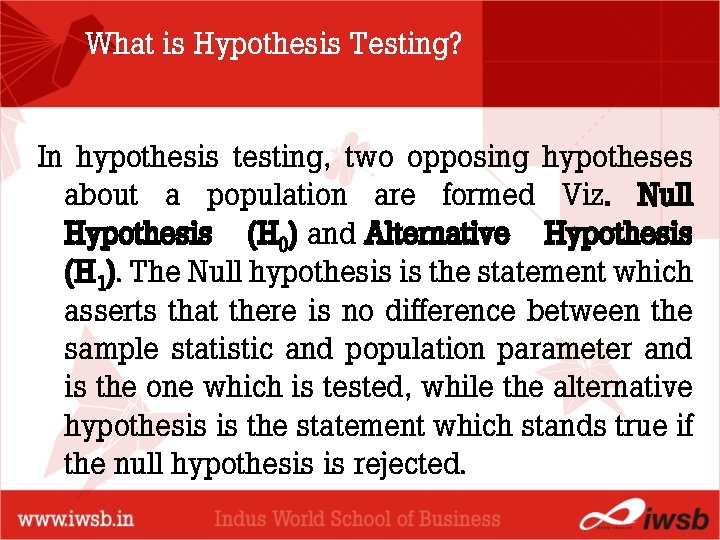
What is Hypothesis Testing? In hypothesis testing, two opposing hypotheses about a population formed Viz. Null - the are B-school Hypothesis (H 0) and Alternative Hypothesis (H 1). The Null hypothesis is the statement which asserts that there is no difference between the sample statistic and population parameter and is the one which is tested, while the alternative hypothesis is the statement which stands true if the null hypothesis is rejected.
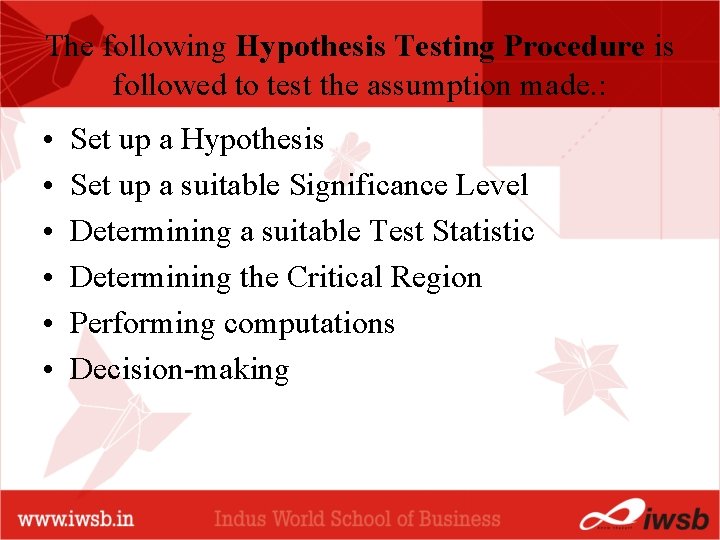
The following Hypothesis Testing Procedure is followed to test the assumption made. : • • • Set up a Hypothesis Set up a suitable Significance Level Determining a suitable Test Statistic Determining the Critical Region Performing computations Decision-making
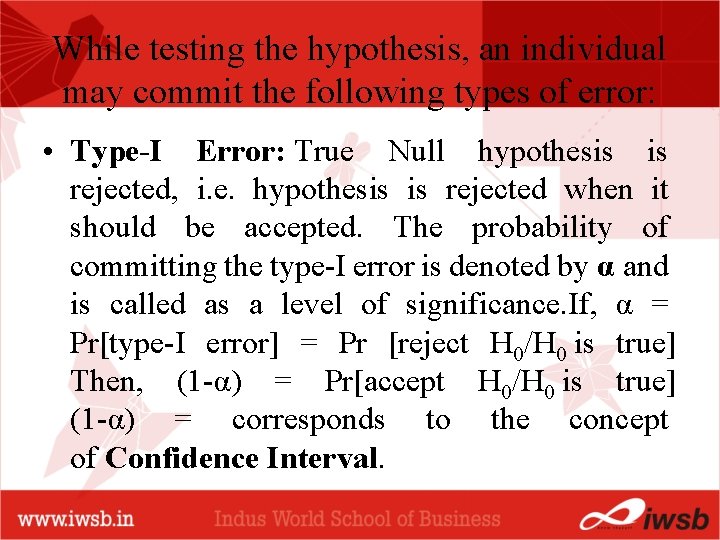
While testing the hypothesis, an individual may commit the following types of error: • Type-I Error: True Null hypothesis is rejected, i. e. hypothesis is rejected when it should be accepted. The probability of committing the type-I error is denoted by α and is called as a level of significance. If, α = Pr[type-I error] = Pr [reject H 0/H 0 is true] Then, (1 -α) = Pr[accept H 0/H 0 is true] (1 -α) = corresponds to the concept of Confidence Interval.
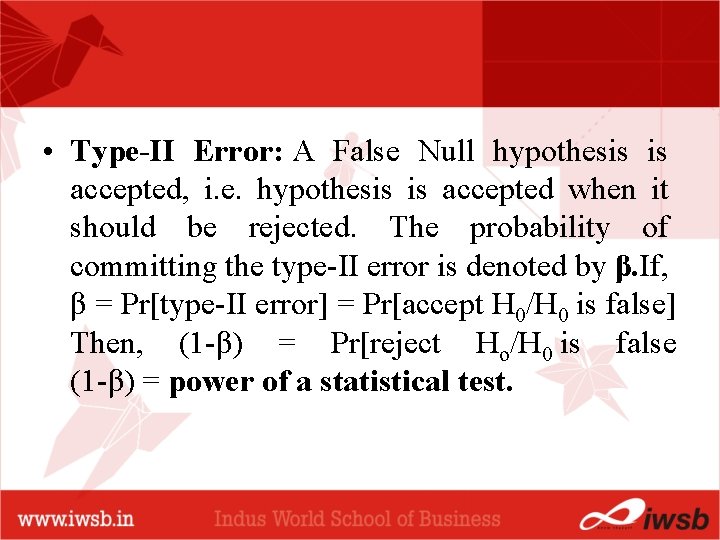
• Type-II Error: A False Null hypothesis is accepted, i. e. hypothesis is accepted when it should be rejected. The probability of committing the type-II error is denoted by β. If, β = Pr[type-II error] = Pr[accept H 0/H 0 is false] Then, (1 -β) = Pr[reject Ho/H 0 is false (1 -β) = power of a statistical test.
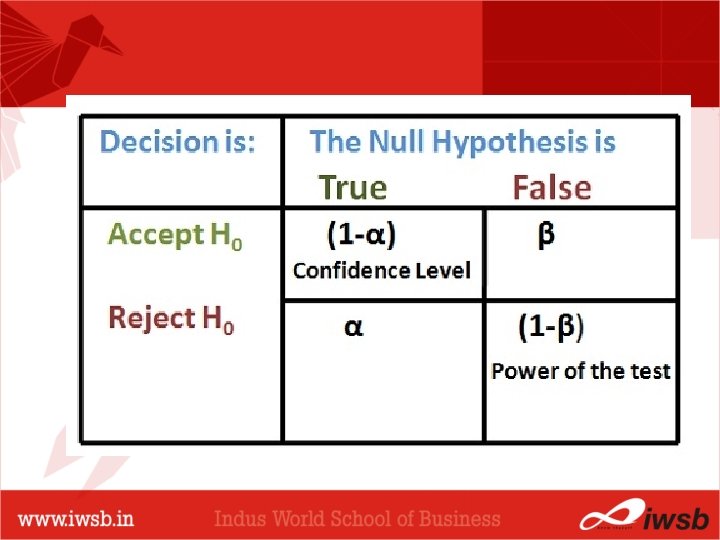
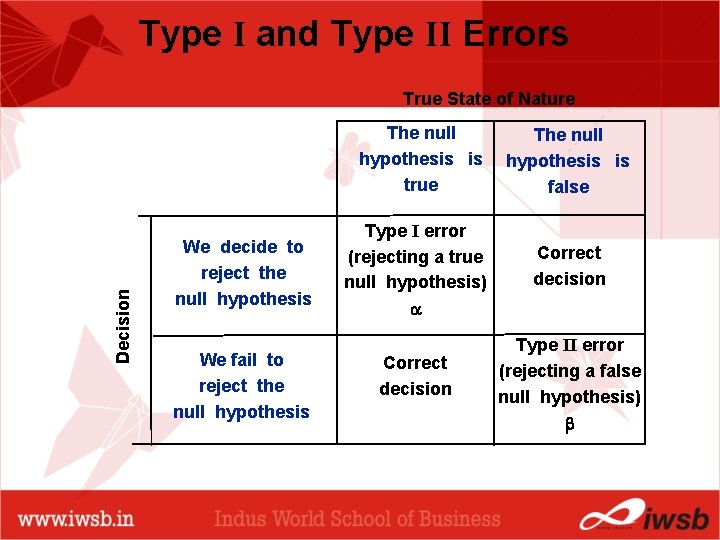
Type I and Type II Errors Decision True State of Nature We decide to reject the null hypothesis We fail to reject the null hypothesis The null hypothesis is true The null hypothesis is false Type I error (rejecting a true null hypothesis) Correct decision Type II error (rejecting a false null hypothesis)
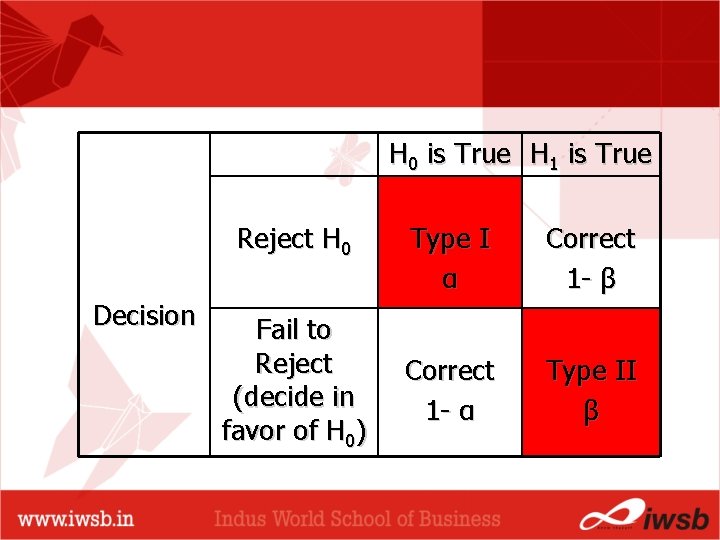
H 0 is True H 1 is True Reject H 0 Decision Fail to Reject (decide in favor of H 0) Type I α Correct 1 - β Correct 1 - α Type II β
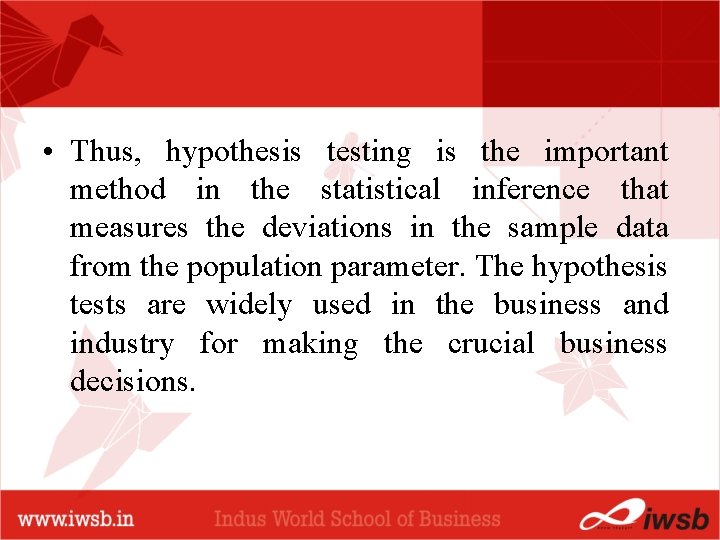
• Thus, hypothesis testing is the important method in the statistical inference that measures the deviations in the sample data from the population parameter. The hypothesis tests are widely used in the business and industry for making the crucial business decisions.
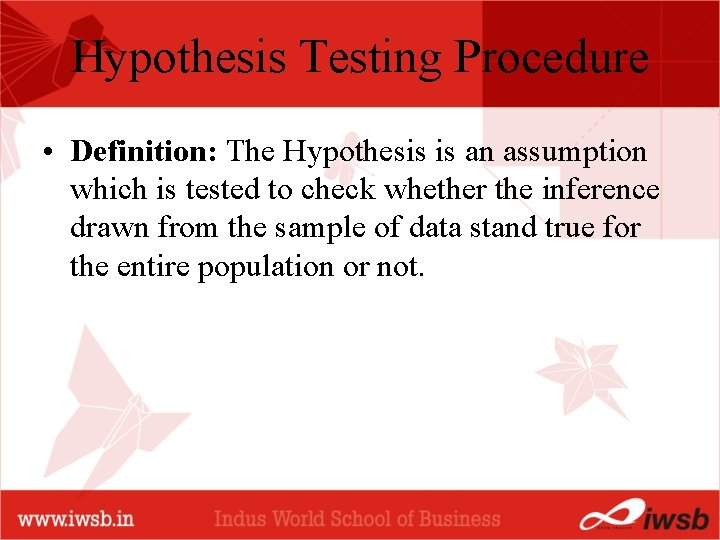
Hypothesis Testing Procedure • Definition: The Hypothesis is an assumption which is tested to check whether the inference drawn from the sample of data stand true for the entire population or not.
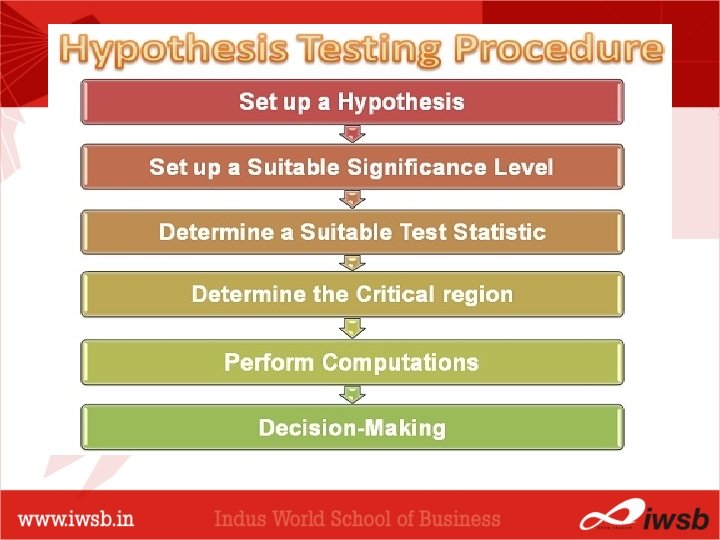
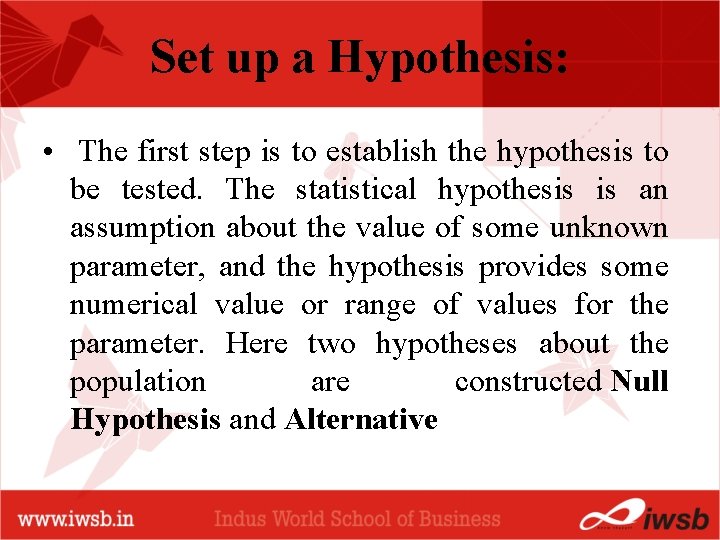
Set up a Hypothesis: • The first step is to establish the hypothesis to be tested. The statistical hypothesis is an assumption about the value of some unknown parameter, and the hypothesis provides some numerical value or range of values for the parameter. Here two hypotheses about the population are constructed Null Hypothesis and Alternative
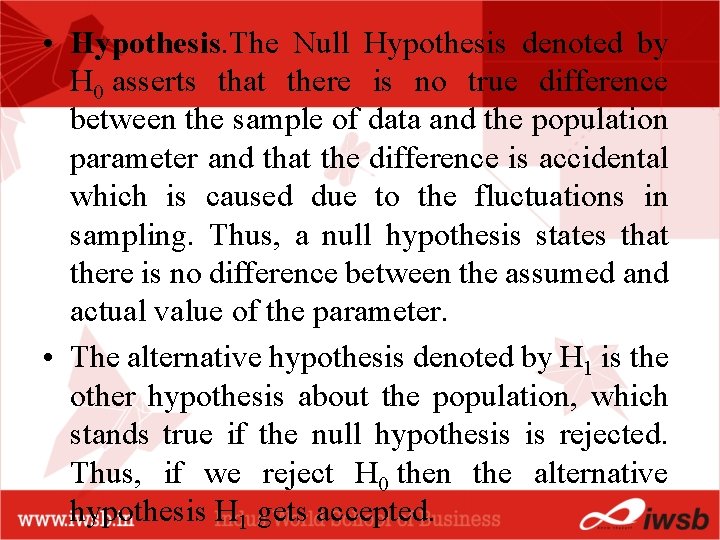
• Hypothesis. The Null Hypothesis denoted by H 0 asserts that there is no true difference between the sample of data and the population parameter and that the difference is accidental which is caused due to the fluctuations in sampling. Thus, a null hypothesis states that there is no difference between the assumed and actual value of the parameter. • The alternative hypothesis denoted by H 1 is the other hypothesis about the population, which stands true if the null hypothesis is rejected. Thus, if we reject H 0 then the alternative hypothesis H 1 gets accepted.
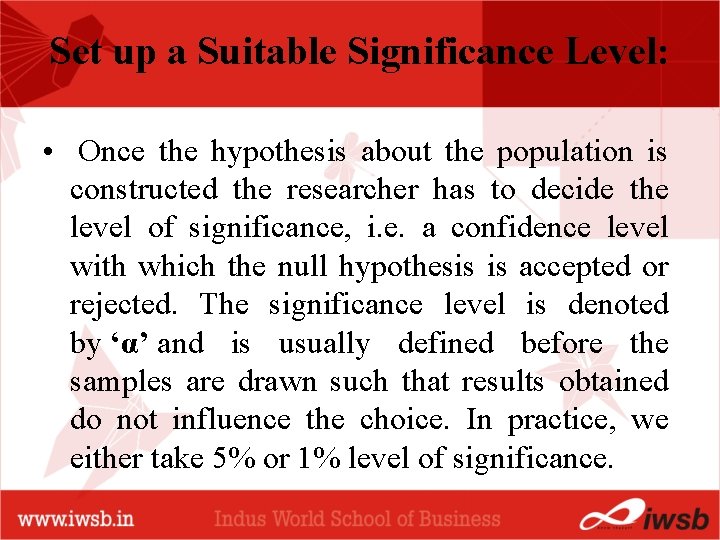
Set up a Suitable Significance Level: • Once the hypothesis about the population is constructed the researcher has to decide the level of significance, i. e. a confidence level with which the null hypothesis is accepted or rejected. The significance level is denoted by ‘α’ and is usually defined before the samples are drawn such that results obtained do not influence the choice. In practice, we either take 5% or 1% level of significance.
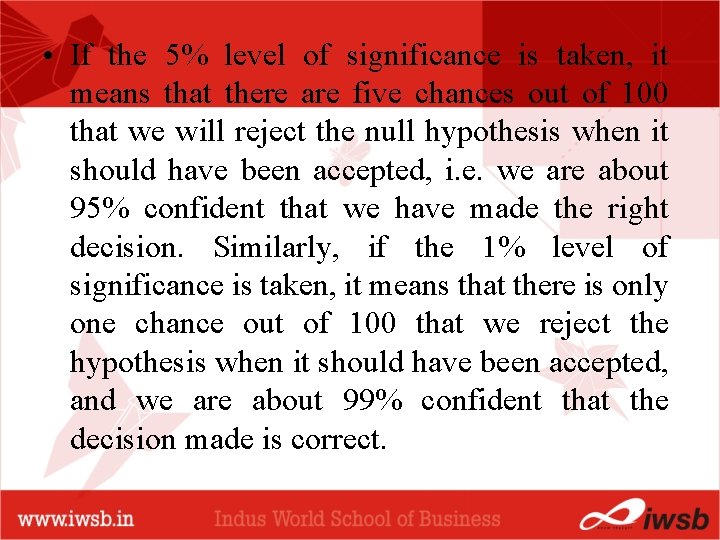
• If the 5% level of significance is taken, it means that there are five chances out of 100 that we will reject the null hypothesis when it should have been accepted, i. e. we are about 95% confident that we have made the right decision. Similarly, if the 1% level of significance is taken, it means that there is only one chance out of 100 that we reject the hypothesis when it should have been accepted, and we are about 99% confident that the decision made is correct.
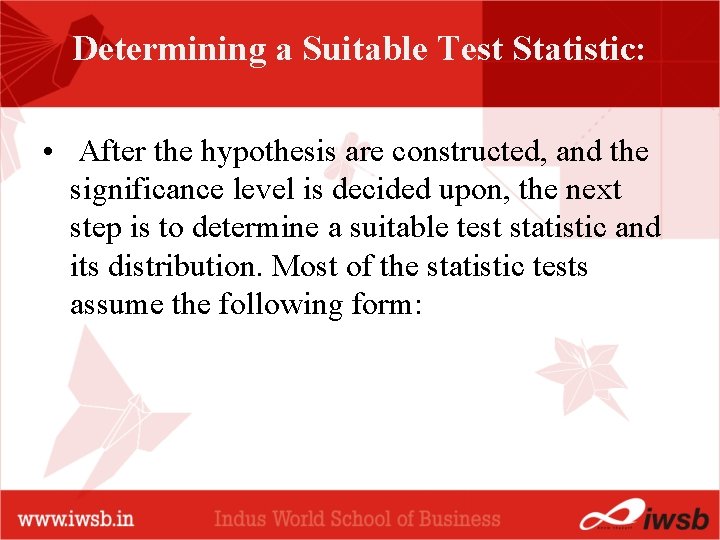
Determining a Suitable Test Statistic: • After the hypothesis are constructed, and the significance level is decided upon, the next step is to determine a suitable test statistic and its distribution. Most of the statistic tests assume the following form:
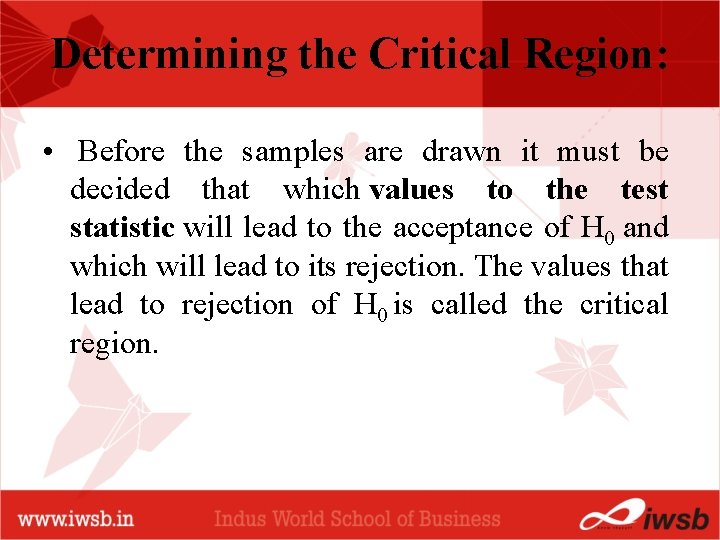
Determining the Critical Region: • Before the samples are drawn it must be decided that which values to the test statistic will lead to the acceptance of H 0 and which will lead to its rejection. The values that lead to rejection of H 0 is called the critical region.
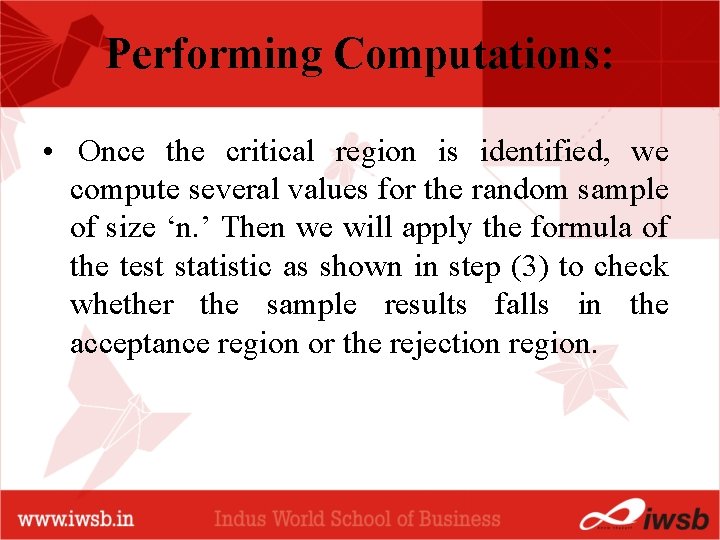
Performing Computations: • Once the critical region is identified, we compute several values for the random sample of size ‘n. ’ Then we will apply the formula of the test statistic as shown in step (3) to check whether the sample results falls in the acceptance region or the rejection region.
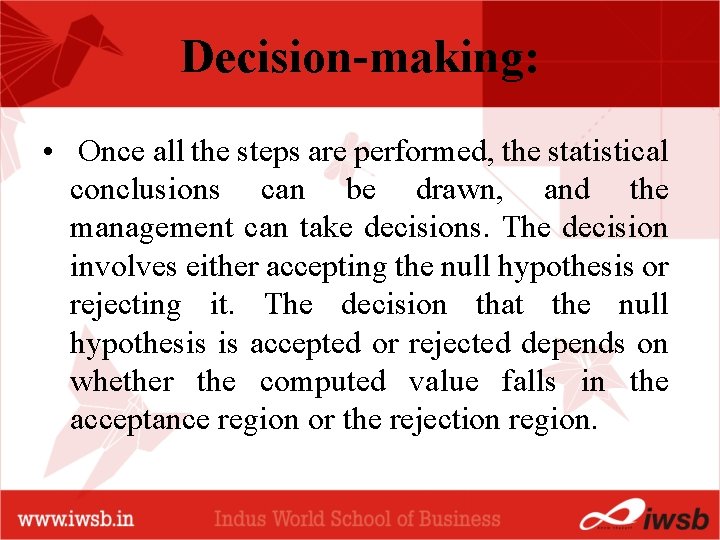
Decision-making: • Once all the steps are performed, the statistical conclusions can be drawn, and the management can take decisions. The decision involves either accepting the null hypothesis or rejecting it. The decision that the null hypothesis is accepted or rejected depends on whether the computed value falls in the acceptance region or the rejection region.
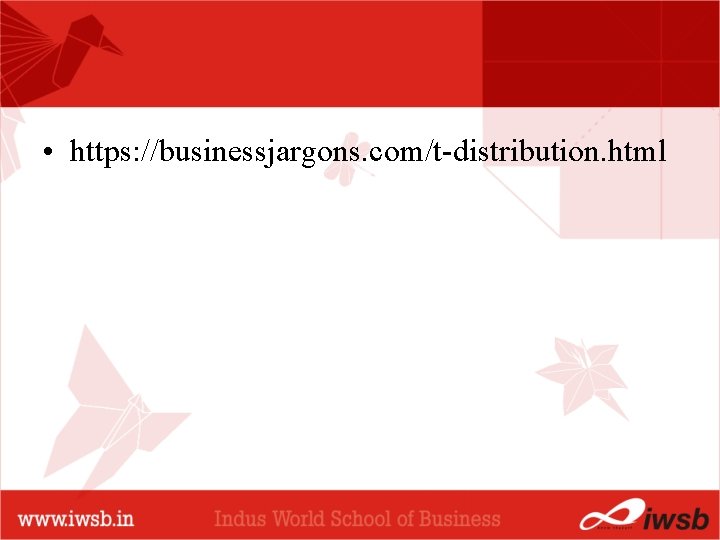
• https: //businessjargons. com/t-distribution. html
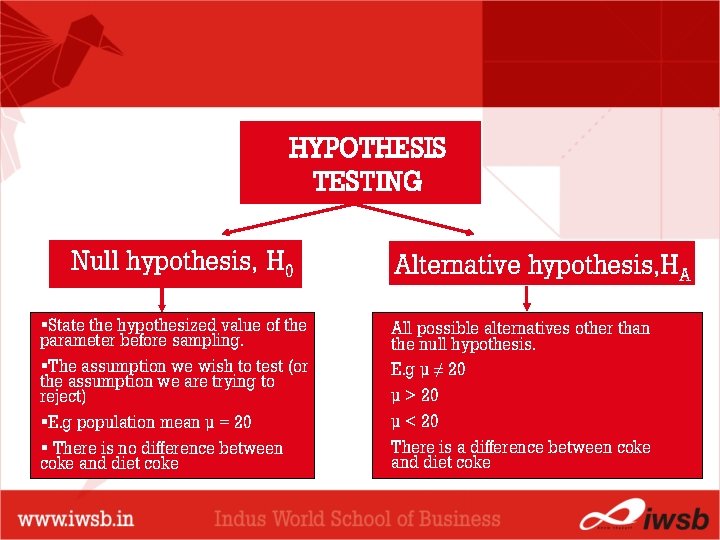
HYPOTHESIS TESTING Null hypothesis, H 0 §State the hypothesized value of the parameter before sampling. §The assumption we wish to test (or the assumption we are trying to reject) §E. g population mean µ = 20 § There is no difference between coke and diet coke Alternative hypothesis, HA All possible alternatives other than the null hypothesis. E. g µ ≠ 20 µ > 20 µ < 20 There is a difference between coke and diet coke
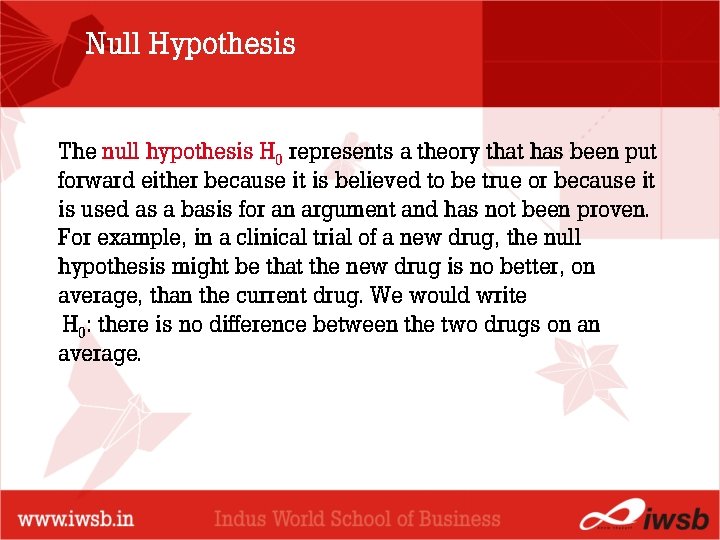
Null Hypothesis The null hypothesis H 0 represents a theory that has been put forward either because it is believed to be true or because it is used as a basis for an argument and has not been proven. For example, in a clinical trial of a new drug, the null hypothesis might be that the new drug is no better, on average, than the current drug. We would write H 0: there is no difference between the two drugs on an average. - the B-school
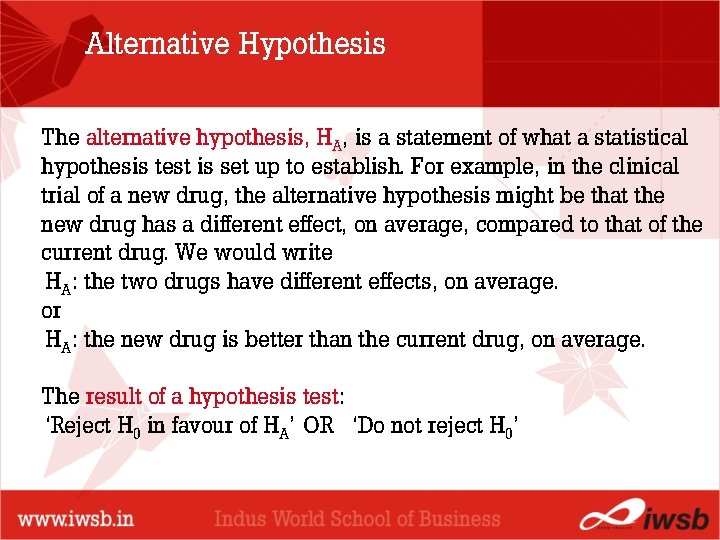
Alternative Hypothesis The alternative hypothesis, HA, is a statement of what a statistical hypothesis test is set up to establish. For example, in the clinical trial of a new drug, the alternative hypothesis might be that the new drug has a different effect, on average, compared to that of the current drug. We would write HA: the two drugs have different effects, on average. or HA: the new drug is better than the current drug, on average. - the B-school The result of a hypothesis test: ‘Reject H 0 in favour of HA’ OR ‘Do not reject H 0’
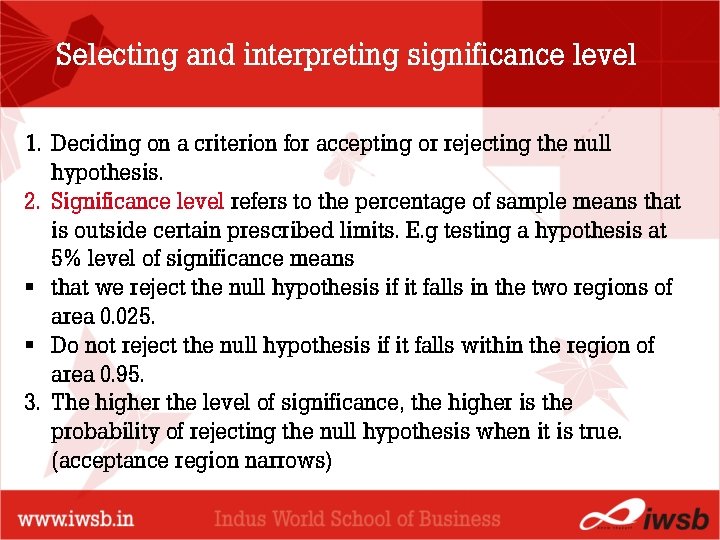
Selecting and interpreting significance level 1. Deciding on a criterion for accepting or rejecting the null hypothesis. 2. Significance level refers to the percentage of sample means that is outside certain prescribed limits. E. g testing a hypothesis at 5% level of significance means § that we reject the null hypothesis if it falls in the two regions of area 0. 025. § Do not reject the null hypothesis if it falls within the region of area 0. 95. 3. The higher the level of significance, the higher is the probability of rejecting the null hypothesis when it is true. (acceptance region narrows) - the B-school
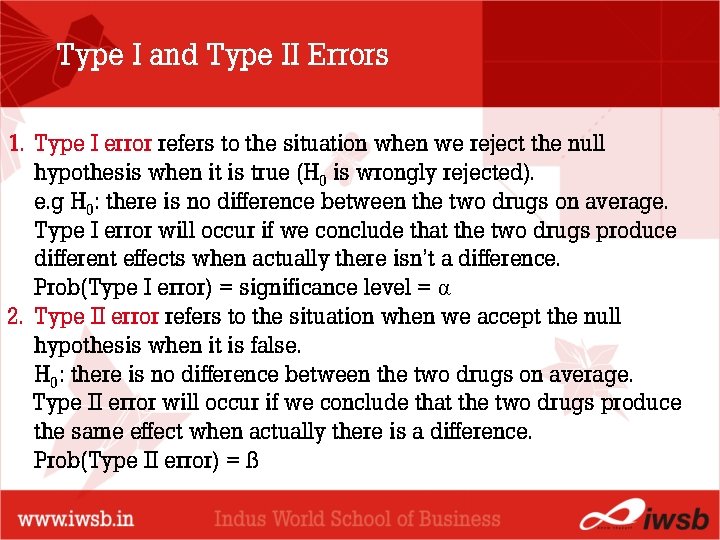
Type I and Type II Errors 1. Type I error refers to the situation when we reject the null hypothesis when it is true (H 0 is wrongly rejected). e. g H 0: there is no difference between the two drugs on average. Type I error will occur if we conclude that the two drugs produce different effects when actually there isn’t a difference. Prob(Type I error) = significance level = α 2. Type II error refers to the situation when we accept the null hypothesis when it is false. H 0: there is no difference between the two drugs on average. Type II error will occur if we conclude that the two drugs produce the same effect when actually there is a difference. Prob(Type II error) = ß - the B-school
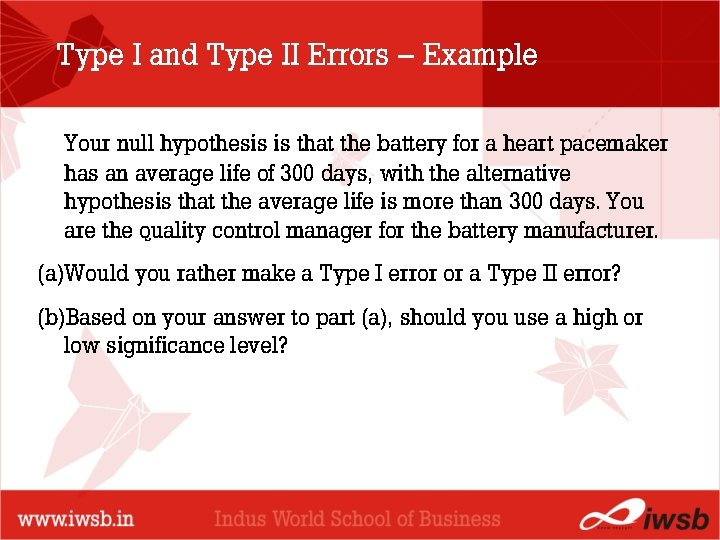
Type I and Type II Errors – Example Your null hypothesis is that the battery for a heart pacemaker has an average life of 300 days, with the alternative hypothesis that the average life is more than 300 days. You are the quality control manager for the battery manufacturer. - the B-school (a)Would you rather make a Type I error or a Type II error? (b)Based on your answer to part (a), should you use a high or low significance level?
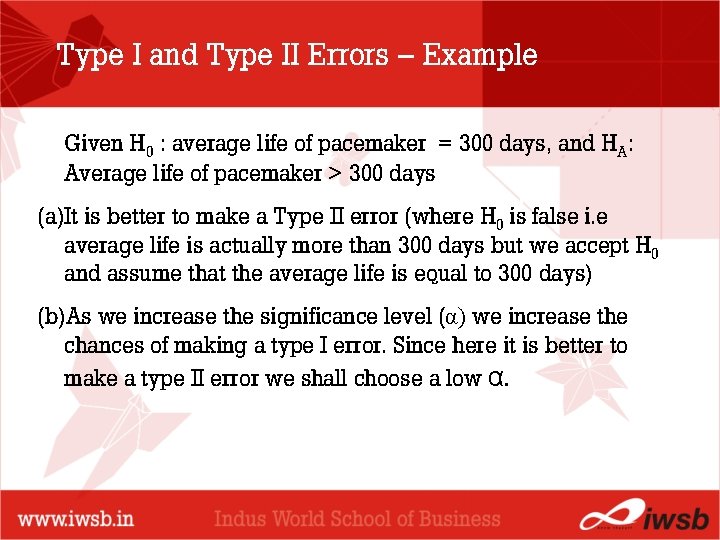
Type I and Type II Errors – Example Given H 0 : average life of pacemaker = 300 days, and HA: Average life of pacemaker > 300 days - the B-school (a)It is better to make a Type II error (where H 0 is false i. e average life is actually more than 300 days but we accept H 0 and assume that the average life is equal to 300 days) (b)As we increase the significance level (α) we increase the chances of making a type I error. Since here it is better to make a type II error we shall choose a low α.
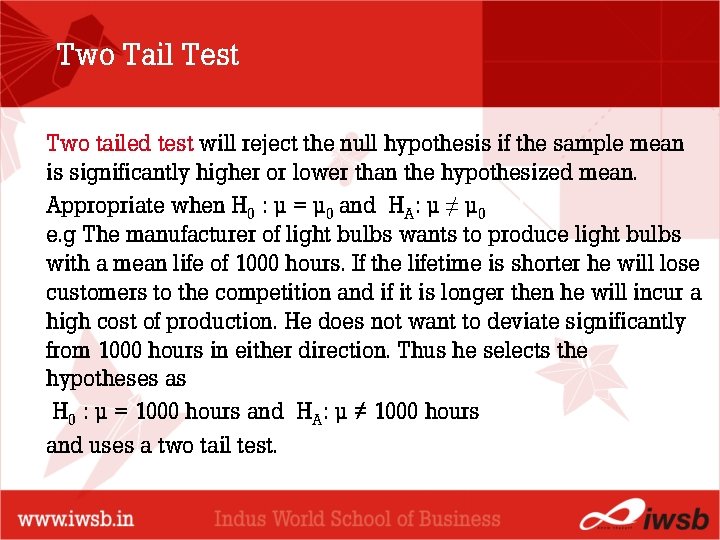
Two Tail Test Two tailed test will reject the null hypothesis if the sample mean is significantly higher or lower than the hypothesized mean. Appropriate when H 0 : µ = µ 0 and HA: µ ≠ µ 0 e. g The manufacturer of light bulbs wants to produce light bulbs with a mean life of 1000 hours. If the lifetime is shorter he will lose customers to the competition and if it is longer then he will incur a high cost of production. He does not want to deviate significantly from 1000 hours in either direction. Thus he selects the hypotheses as H 0 : µ = 1000 hours and HA: µ ≠ 1000 hours and uses a two tail test. - the B-school
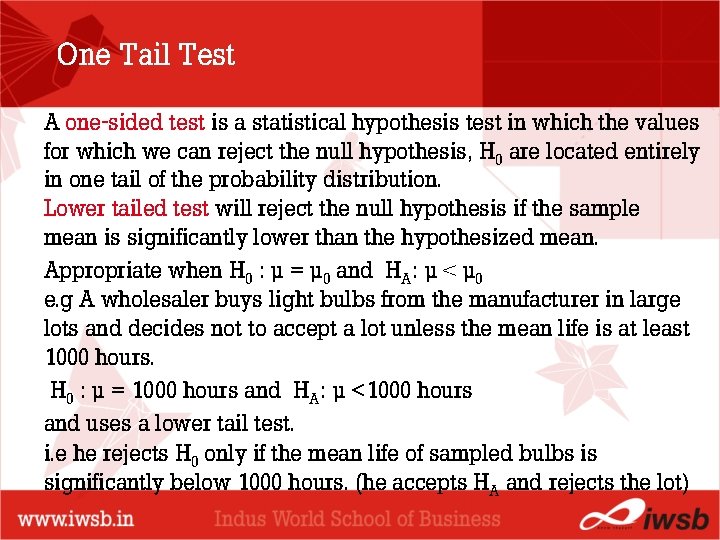
One Tail Test A one-sided test is a statistical hypothesis test in which the values for which we can reject the null hypothesis, H 0 are located entirely in one tail of the probability distribution. Lower tailed test will reject the null hypothesis if the sample mean is significantly lower than the hypothesized mean. Appropriate when H 0 : µ = µ 0 and HA: µ < µ 0 e. g A wholesaler buys light bulbs from the manufacturer in large lots and decides not to accept a lot unless the mean life is at least 1000 hours. H 0 : µ = 1000 hours and HA: µ <1000 hours and uses a lower tail test. i. e he rejects H 0 only if the mean life of sampled bulbs is significantly below 1000 hours. (he accepts HA and rejects the lot) - the B-school
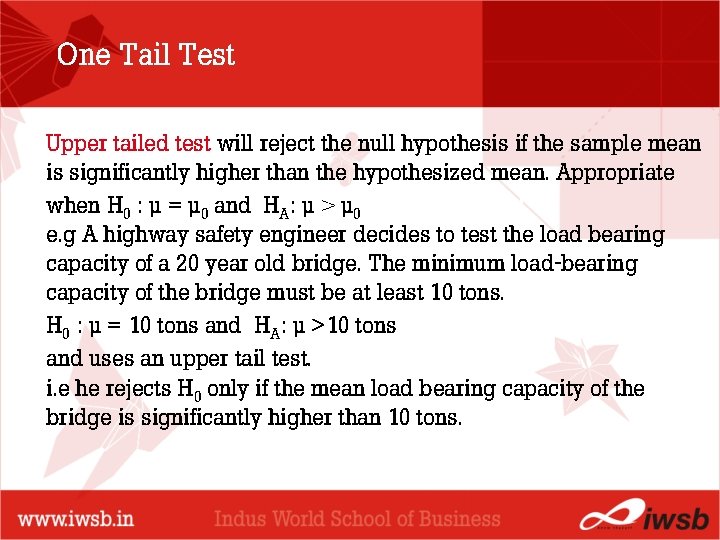
One Tail Test Upper tailed test will reject the null hypothesis if the sample mean is significantly higher than the hypothesized mean. Appropriate when H 0 : µ = µ 0 and HA: µ > µ 0 e. g A highway safety engineer decides to test the load bearing capacity of a 20 year old bridge. The minimum load-bearing capacity of the bridge must be at least 10 tons. H 0 : µ = 10 tons and HA: µ >10 tons and uses an upper tail test. i. e he rejects H 0 only if the mean load bearing capacity of the bridge is significantly higher than 10 tons. - the B-school
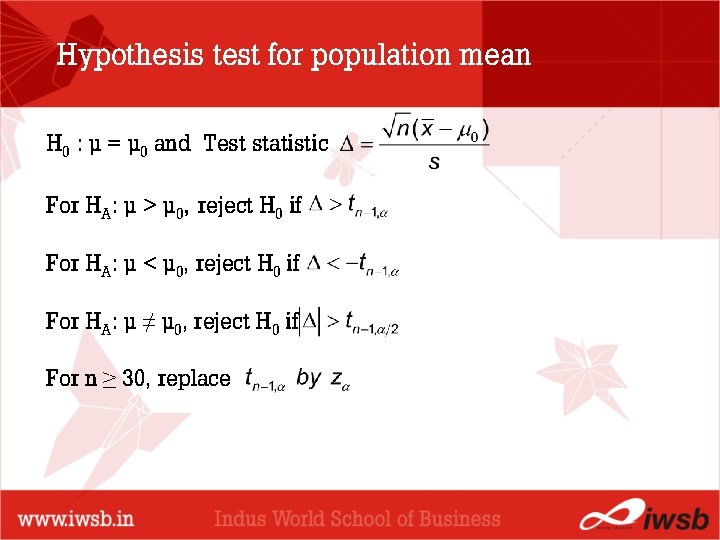
Hypothesis test for population mean H 0 : µ = µ 0 and Test statistic - the B-school For HA: µ > µ 0, reject H 0 if For HA: µ < µ 0, reject H 0 if For HA: µ ≠ µ 0, reject H 0 if For n ≥ 30, replace
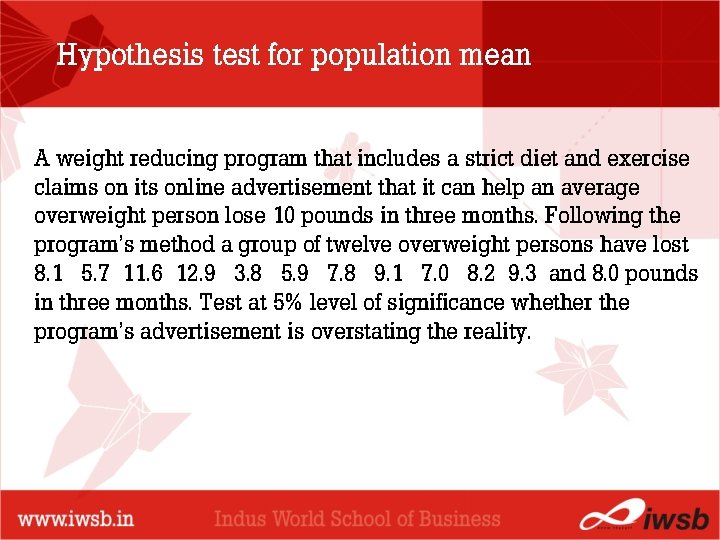
Hypothesis test for population mean A weight reducing program that includes a strict diet and exercise claims on its online advertisement that it can help an average overweight person lose 10 pounds in three months. Following the program’s method a group of twelve overweight persons have lost 8. 1 5. 7 11. 6 12. 9 3. 8 5. 9 7. 8 9. 1 7. 0 8. 2 9. 3 and 8. 0 pounds in three months. Test at 5% level of significance whether the program’s advertisement is overstating the reality. - the B-school
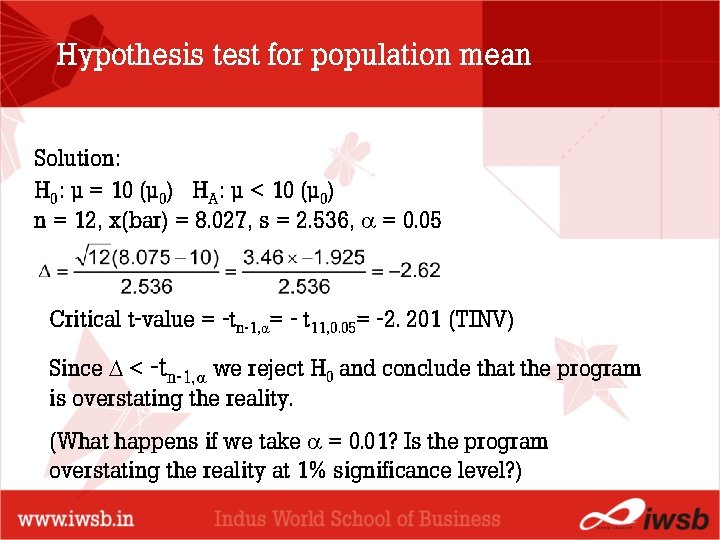
Hypothesis test for population mean Solution: H 0: µ = 10 (µ 0) HA: µ < 10 (µ 0) n = 12, x(bar) = 8. 027, s = 2. 536, = 0. 05 Critical t-value = -tn-1, α= - t 11, 0. 05= -2. 201 (TINV) Since < -tn-1, α we reject H 0 and conclude that the program is overstating the reality. (What happens if we take = 0. 01? Is the program overstating the reality at 1% significance level? )
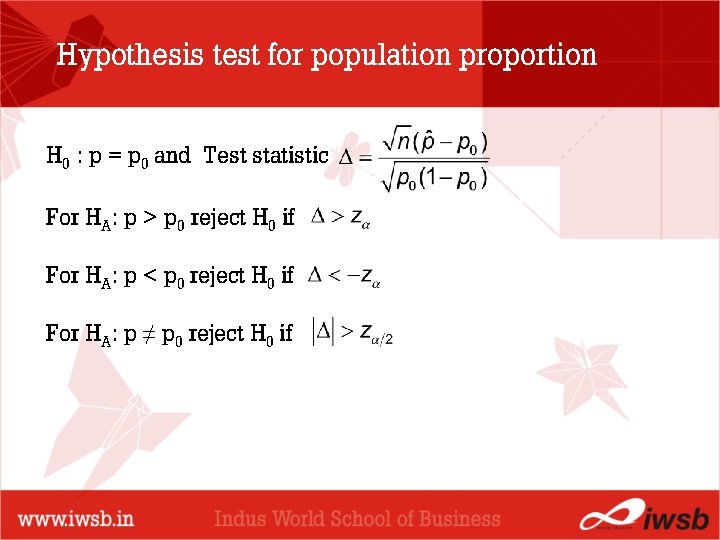
Hypothesis test for population proportion H 0 : p = p 0 and Test statistic For HA: p > p 0 reject H 0 if For HA: p < p 0 reject H 0 if For HA: p ≠ p 0 reject H 0 if - the B-school
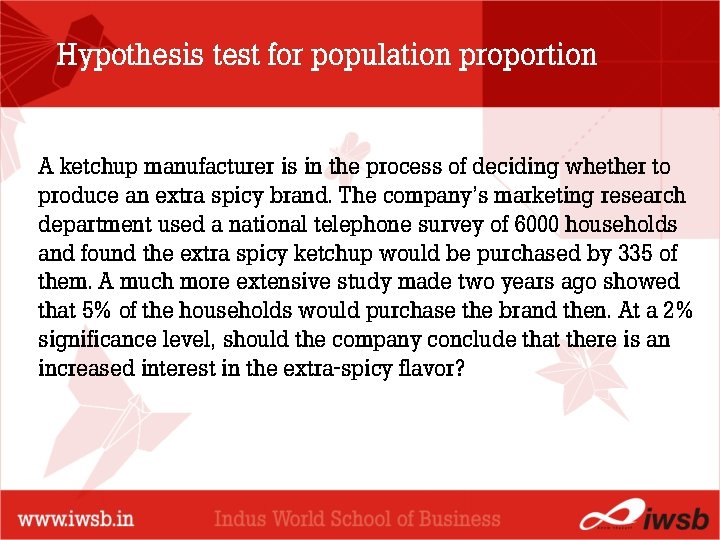
Hypothesis test for population proportion A ketchup manufacturer is in the process of deciding whether to produce an extra spicy brand. The company’s marketing research department used a national telephone survey of 6000 households and found the extra spicy ketchup would be purchased by 335 of them. A much more extensive study made two years ago showed that 5% of the households would purchase the brand then. At a 2% significance level, should the company conclude that there is an increased interest in the extra-spicy flavor? - the B-school
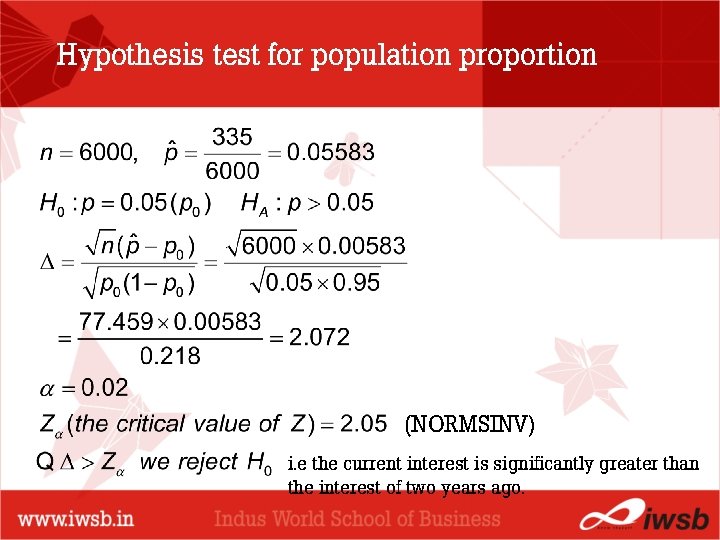
Hypothesis test for population proportion - the B-school (NORMSINV) i. e the current interest is significantly greater than the interest of two years ago.
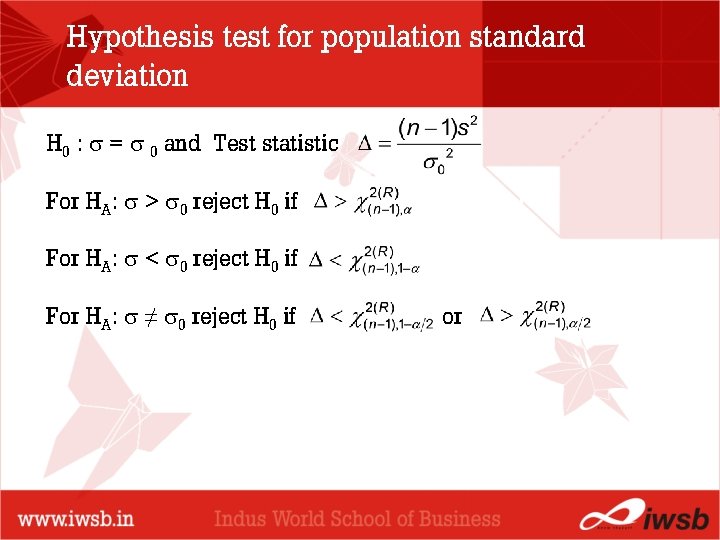
Hypothesis test for population standard deviation H 0 : = 0 and Test statistic - the B-school For HA: > 0 reject H 0 if For HA: < 0 reject H 0 if For HA: ≠ 0 reject H 0 if or
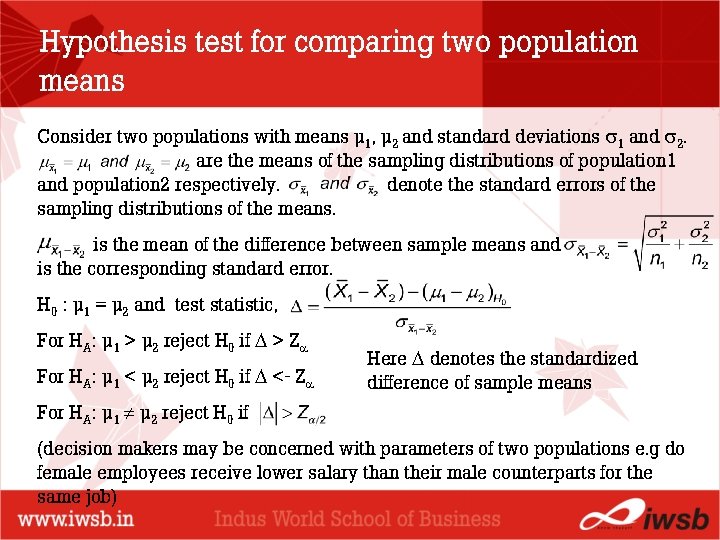
Hypothesis test for comparing two population means Consider two populations with means µ 1, µ 2 and standard deviations 1 and 2. are the means of the sampling distributions of population 1 and population 2 respectively. denote the standard errors of the sampling distributions of the means. - the B-school is the mean of the difference between sample means and is the corresponding standard error. H 0 : µ 1 = µ 2 and test statistic, For HA: µ 1 > µ 2 reject H 0 if > Z For HA: µ 1 < µ 2 reject H 0 if <- Z Here denotes the standardized difference of sample means For HA: µ 1 µ 2 reject H 0 if (decision makers may be concerned with parameters of two populations e. g do female employees receive lower salary than their male counterparts for the same job)
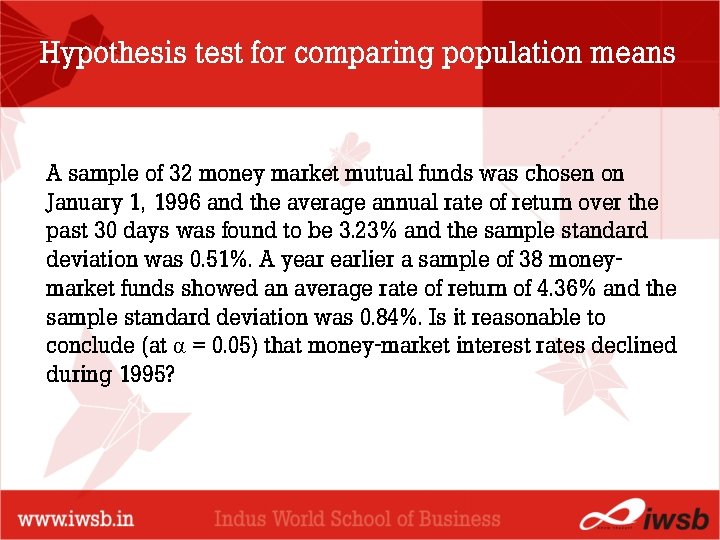
Hypothesis test for comparing population means A sample of 32 money market mutual funds was chosen on January 1, 1996 and the average annual rate of return over the past 30 days was found to be 3. 23% and the sample standard deviation was 0. 51%. A year earlier a sample of 38 moneymarket funds showed an average rate of return of 4. 36% and the sample standard deviation was 0. 84%. Is it reasonable to conclude (at α = 0. 05) that money-market interest rates declined during 1995? - the B-school
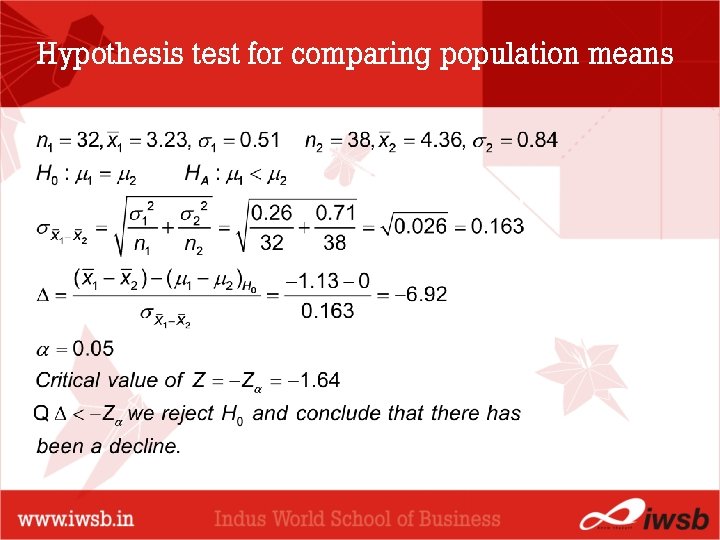
Hypothesis test for comparing population means - the B-school
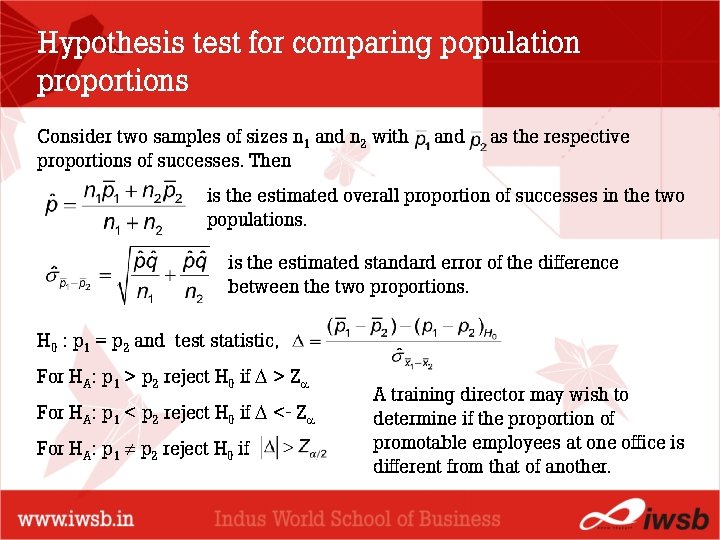
Hypothesis test for comparing population proportions Consider two samples of sizes n 1 and n 2 with proportions of successes. Then and as the respective - the B-school is the estimated overall proportion of successes in the two populations. is the estimated standard error of the difference between the two proportions. H 0 : p 1 = p 2 and test statistic, For HA: p 1 > p 2 reject H 0 if > Z For HA: p 1 < p 2 reject H 0 if <- Z For HA: p 1 p 2 reject H 0 if A training director may wish to determine if the proportion of promotable employees at one office is different from that of another.
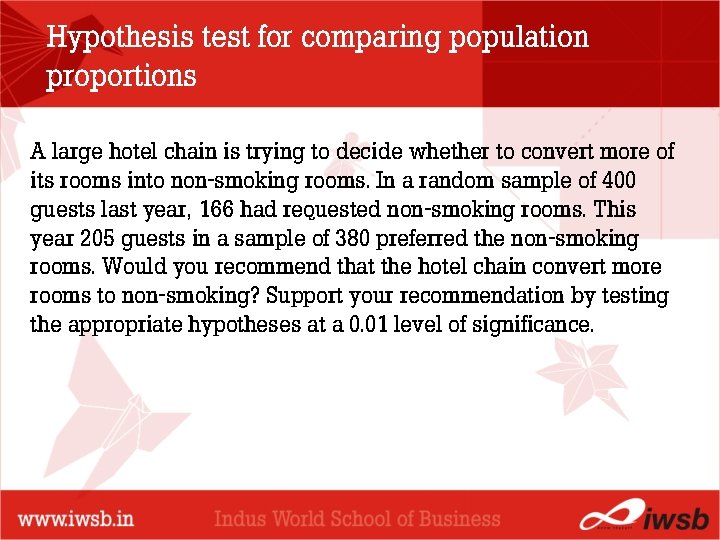
Hypothesis test for comparing population proportions A large hotel chain is trying to decide whether to convert more of its rooms into non-smoking rooms. In a random sample of 400 guests last year, 166 had requested non-smoking rooms. This year 205 guests in a sample of 380 preferred the non-smoking rooms. Would you recommend that the hotel chain convert more rooms to non-smoking? Support your recommendation by testing the appropriate hypotheses at a 0. 01 level of significance. - the B-school
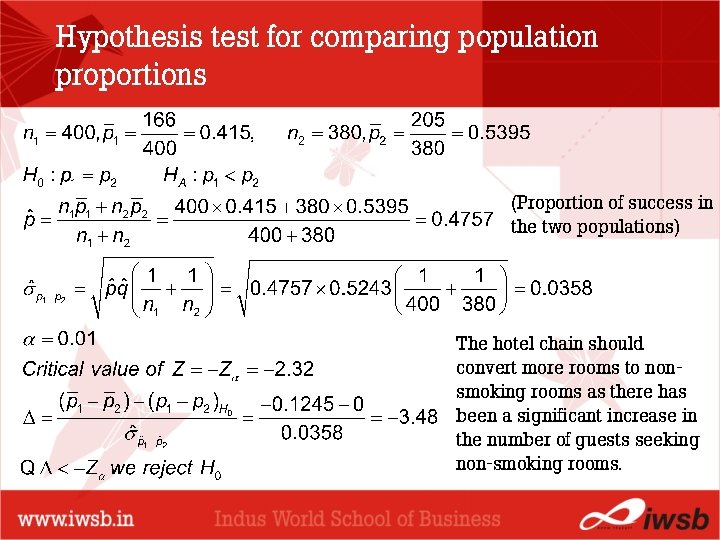
Hypothesis test for comparing population proportions of success in - the B-school(Proportion the two populations) The hotel chain should convert more rooms to nonsmoking rooms as there has been a significant increase in the number of guests seeking non-smoking rooms.