Hedera Dynamic Flow Scheduling for Data Center Networks
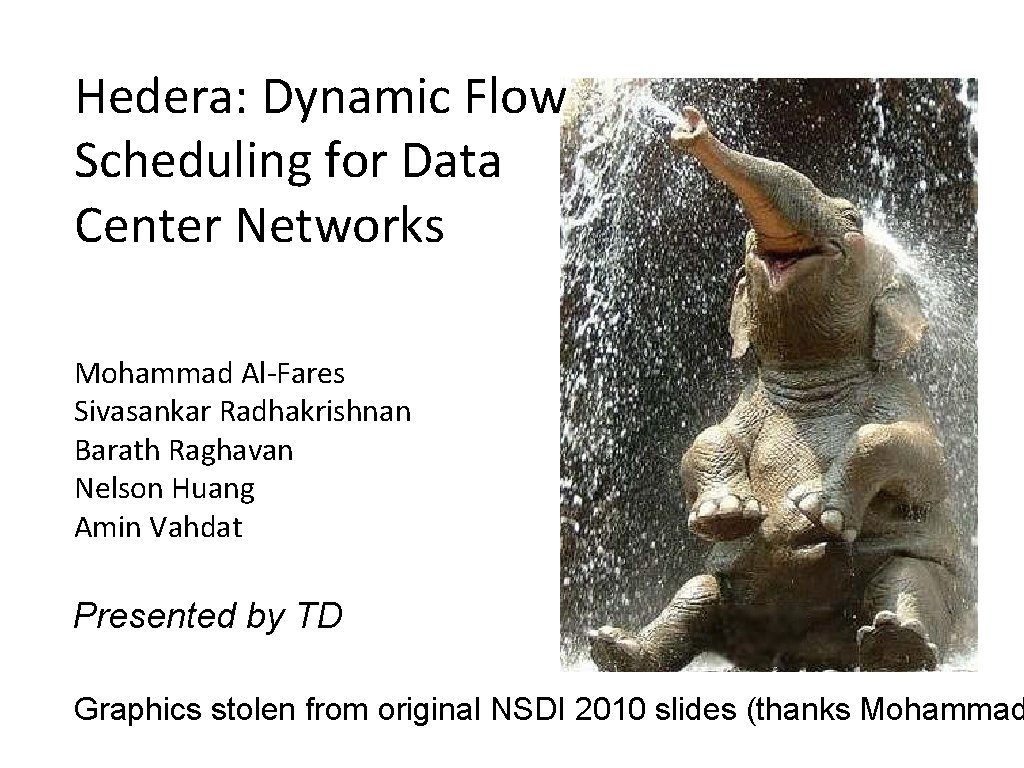
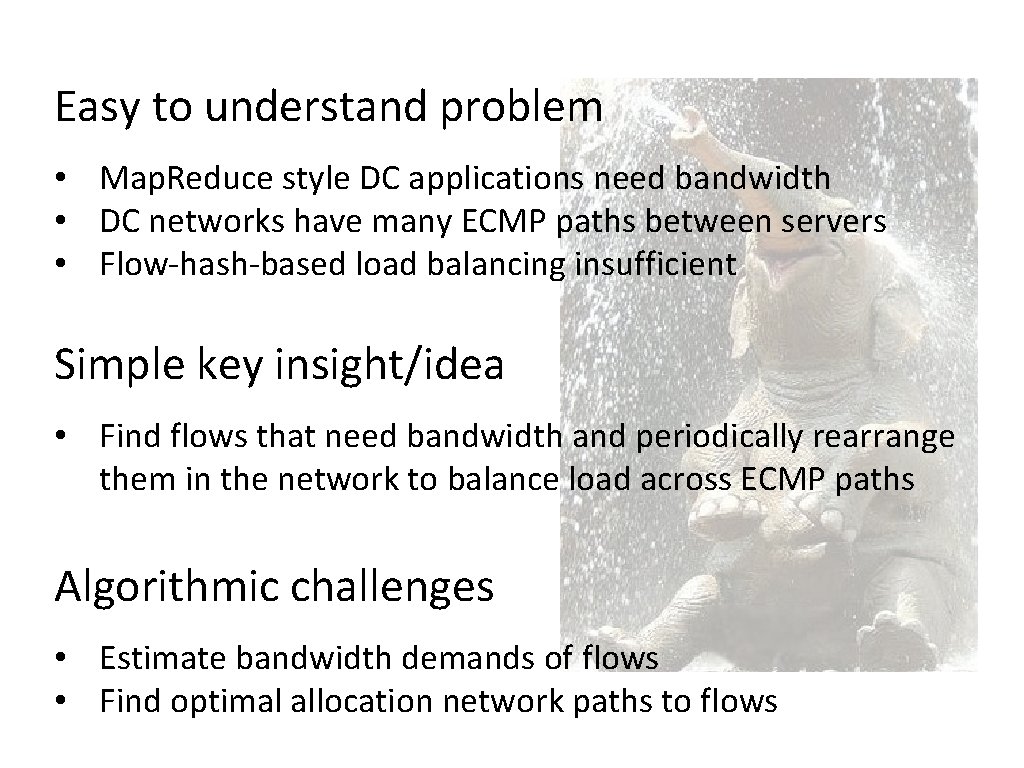
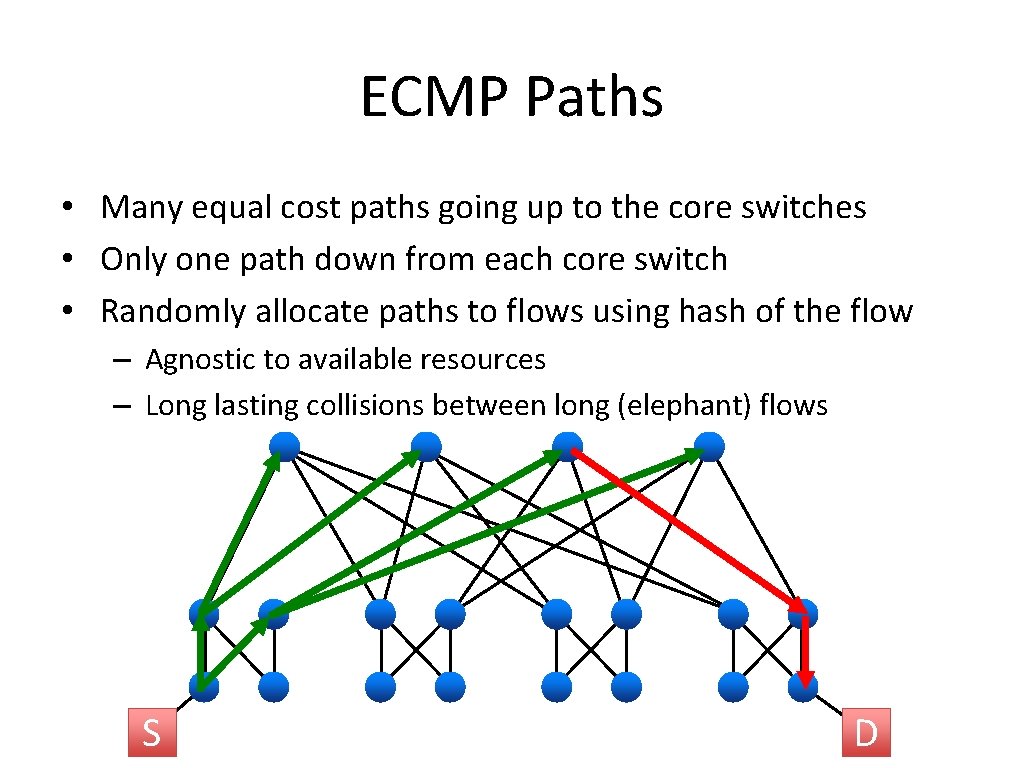
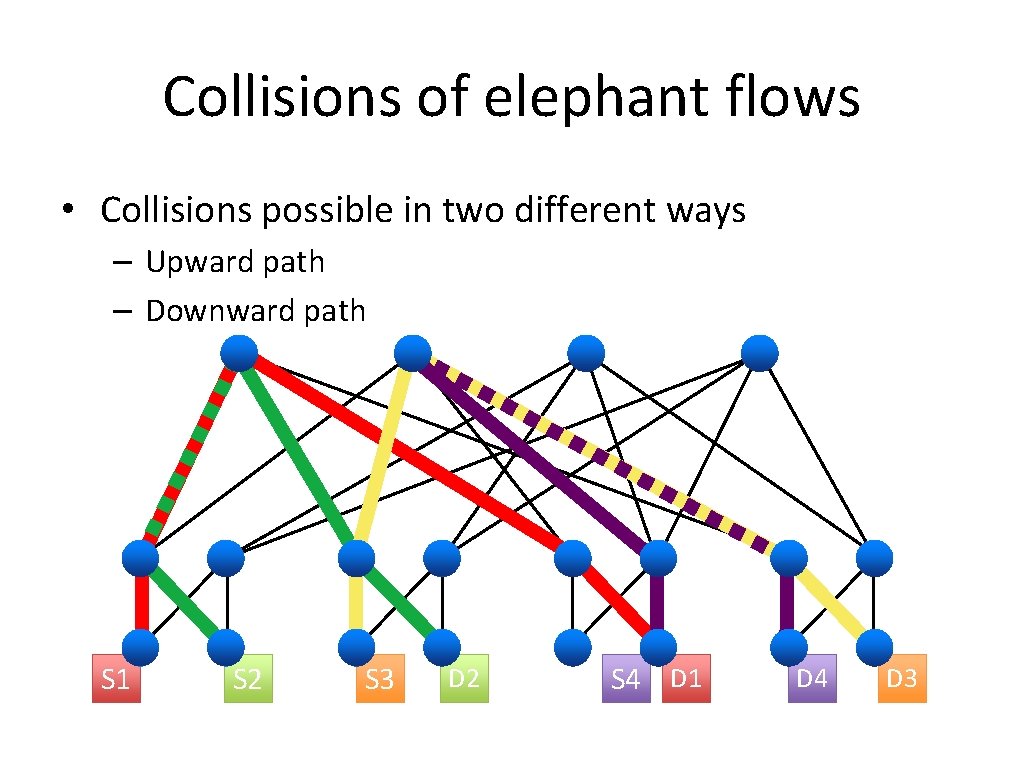
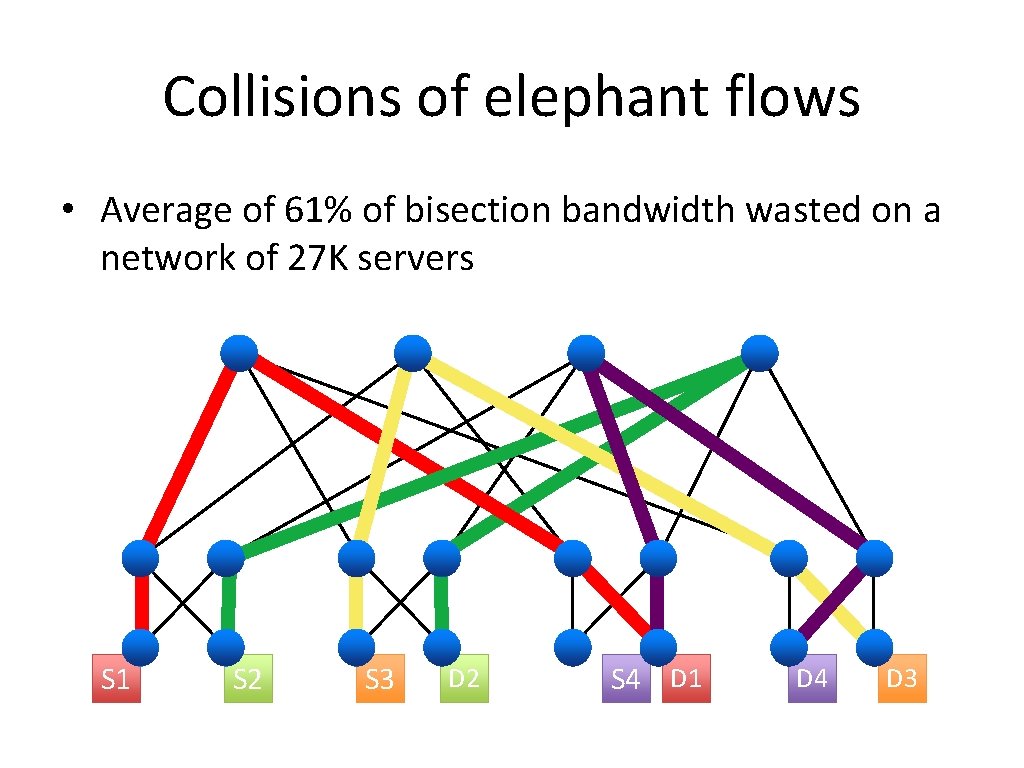
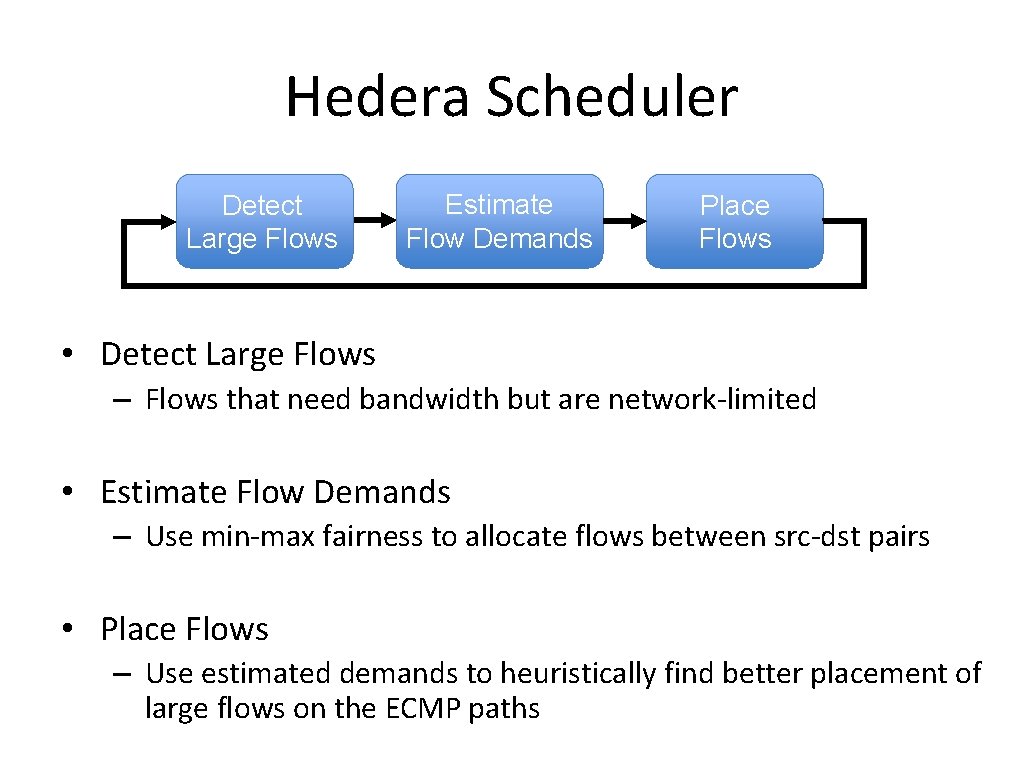
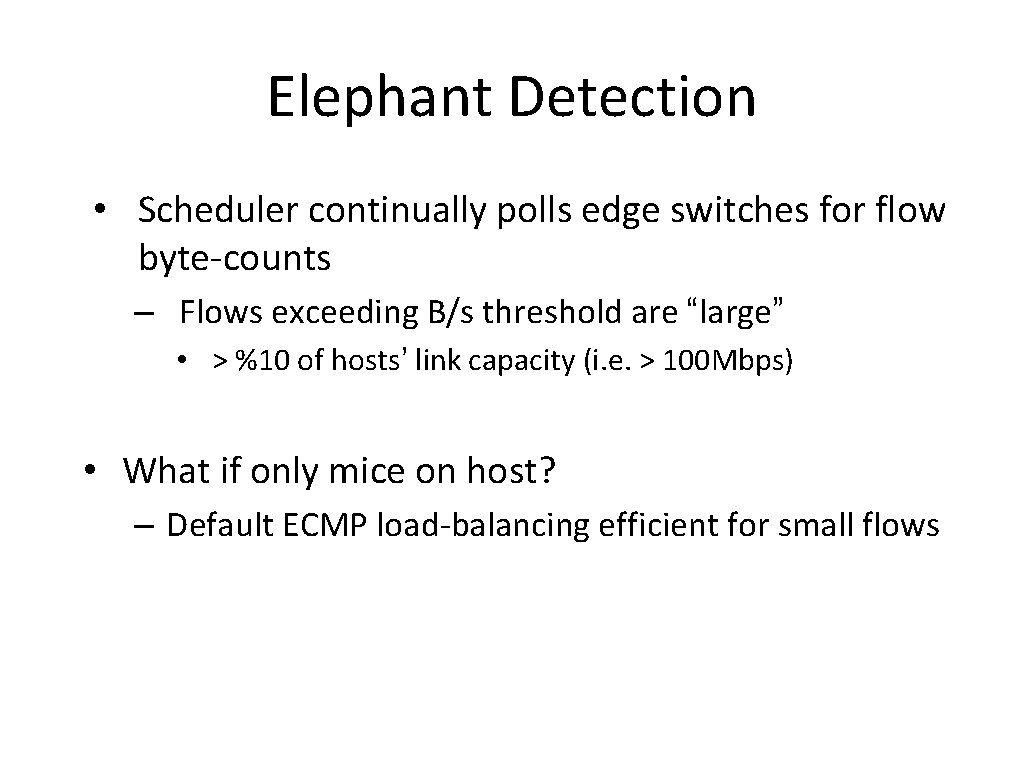
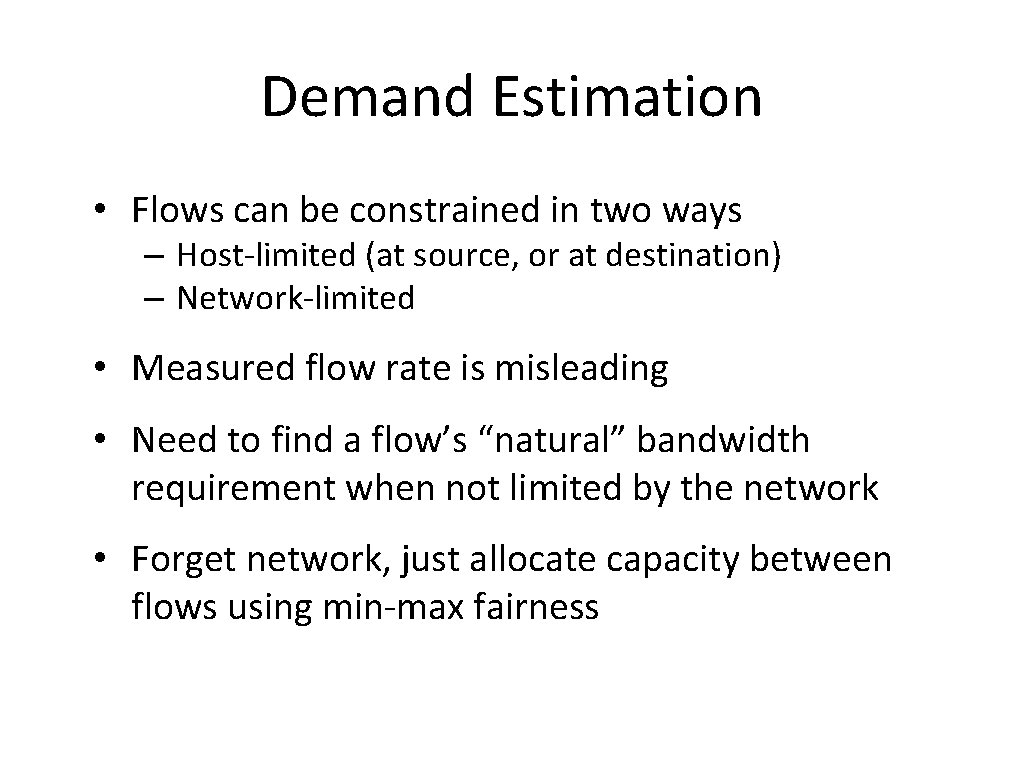
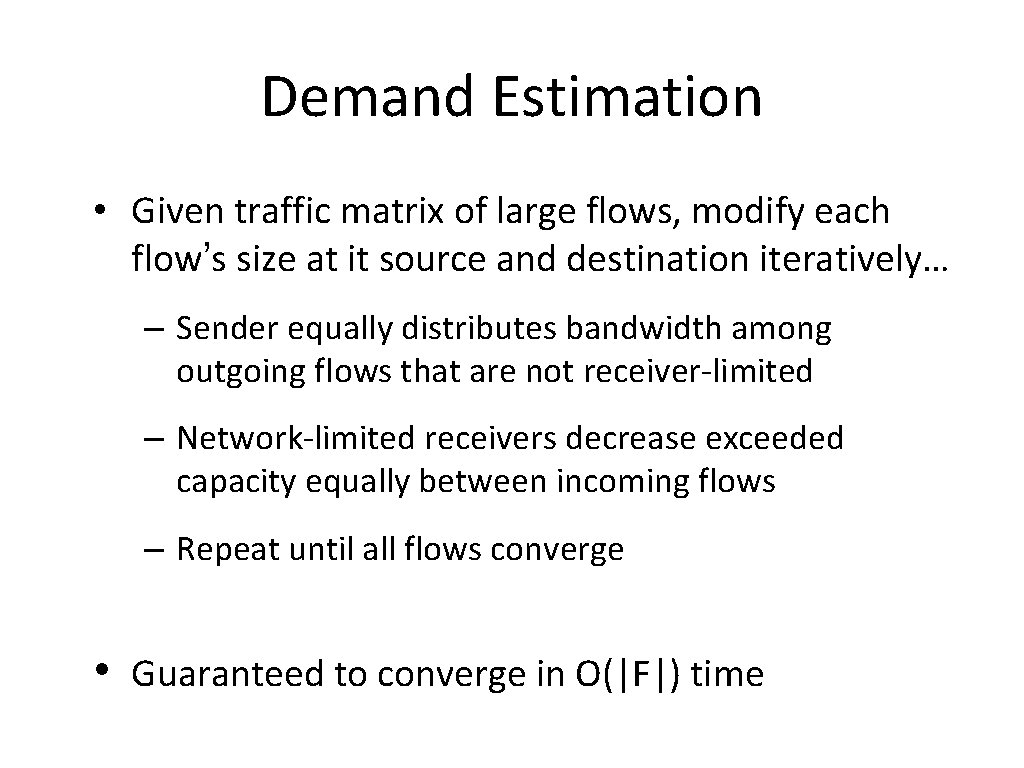
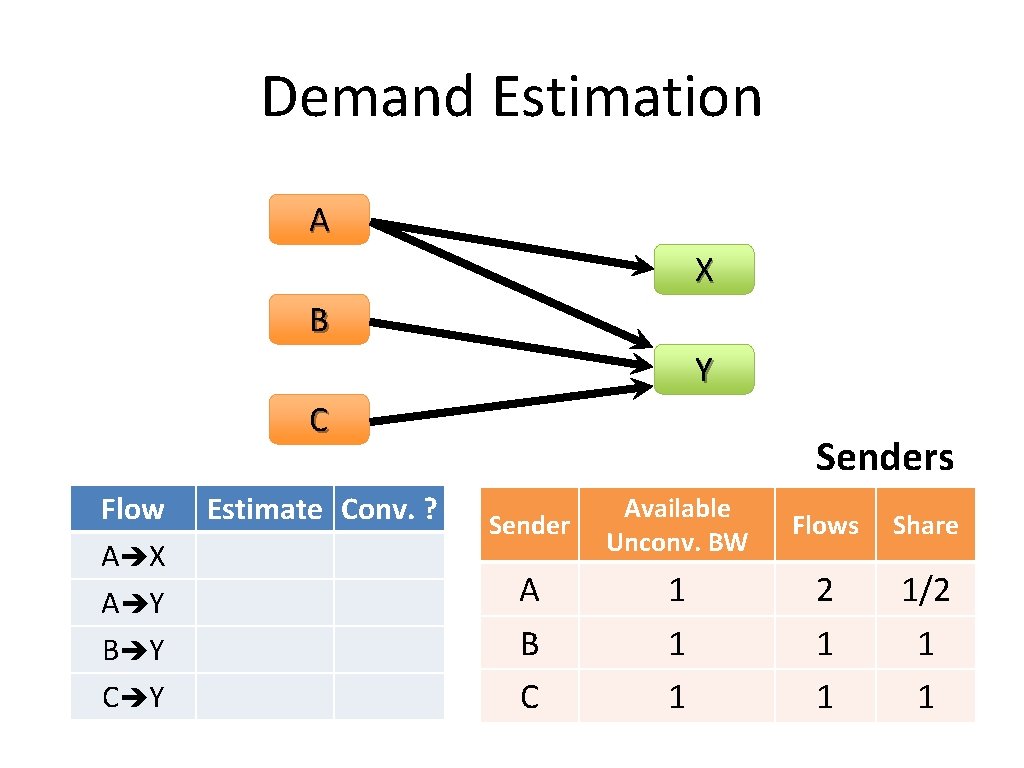
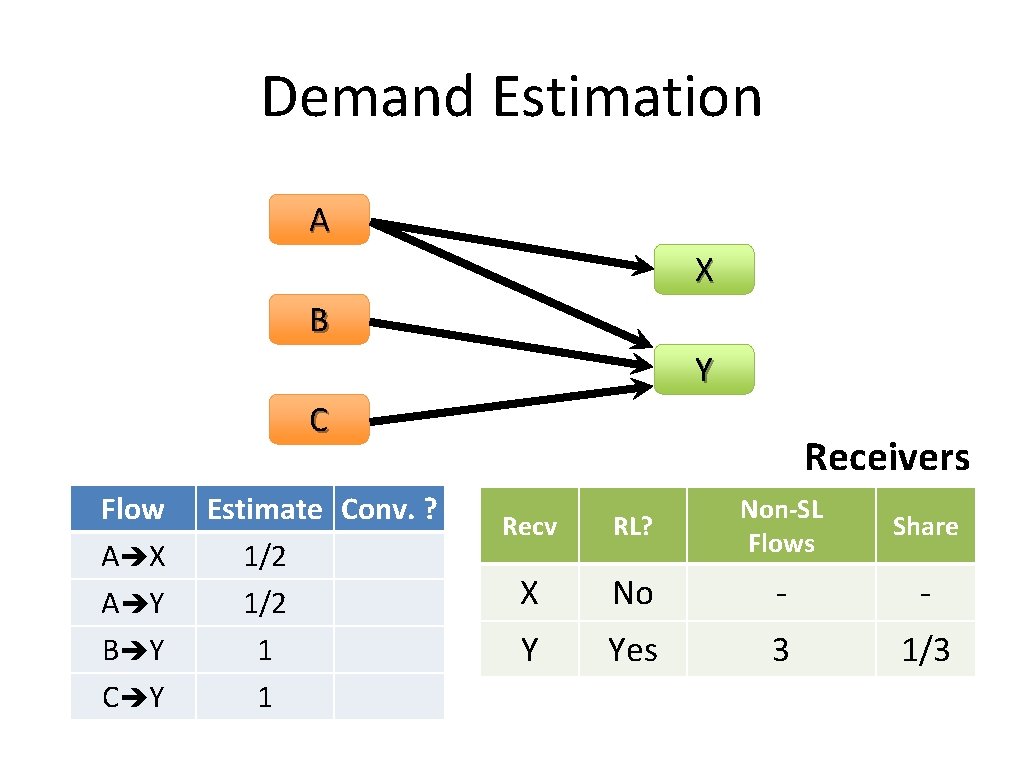
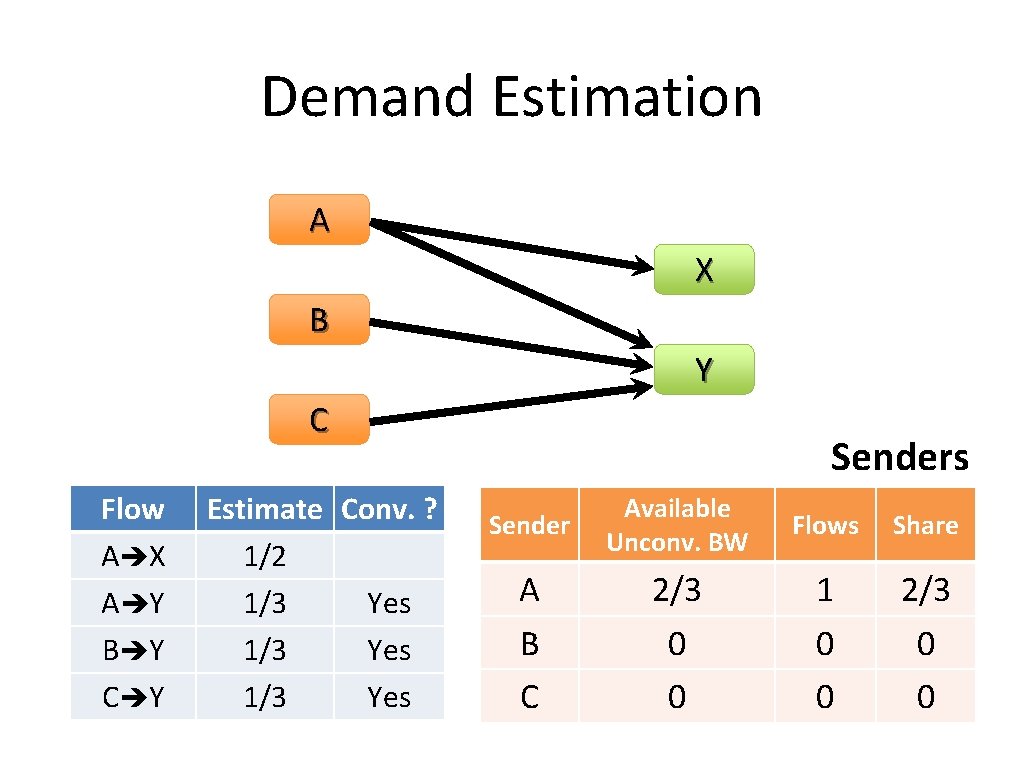
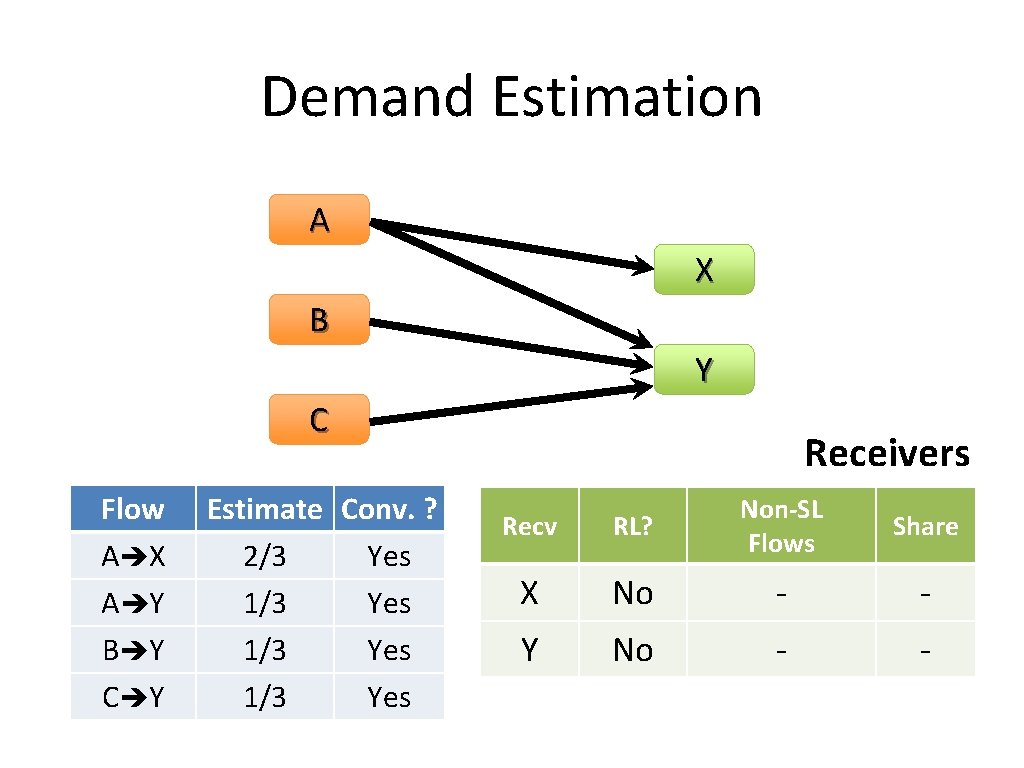
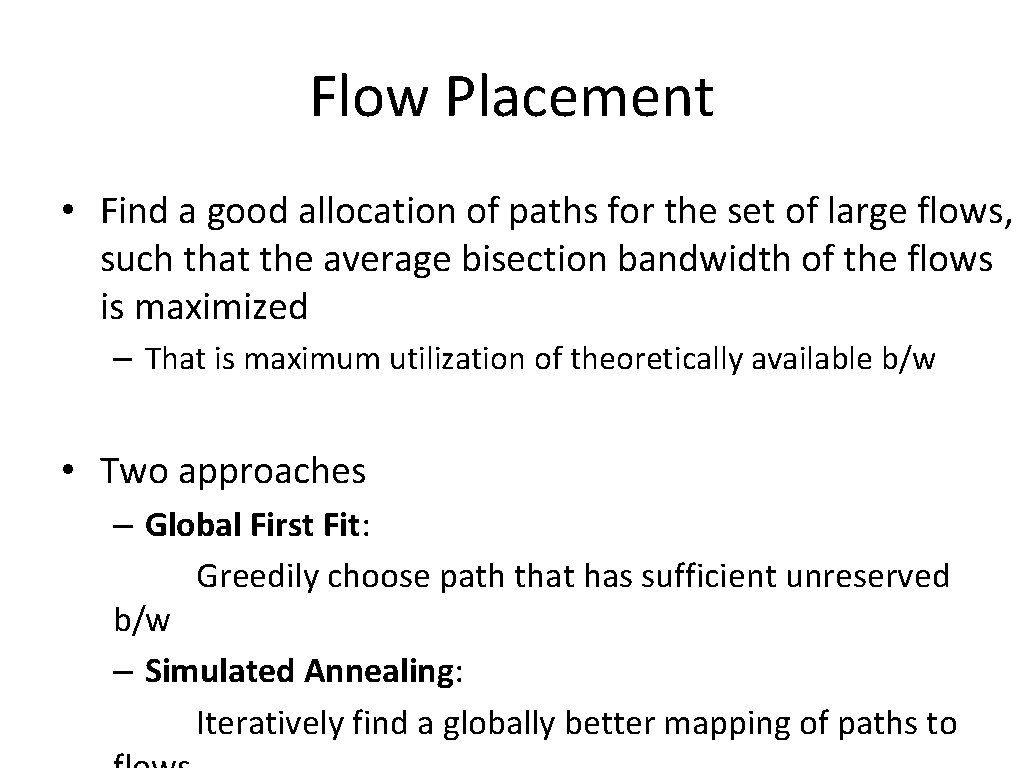
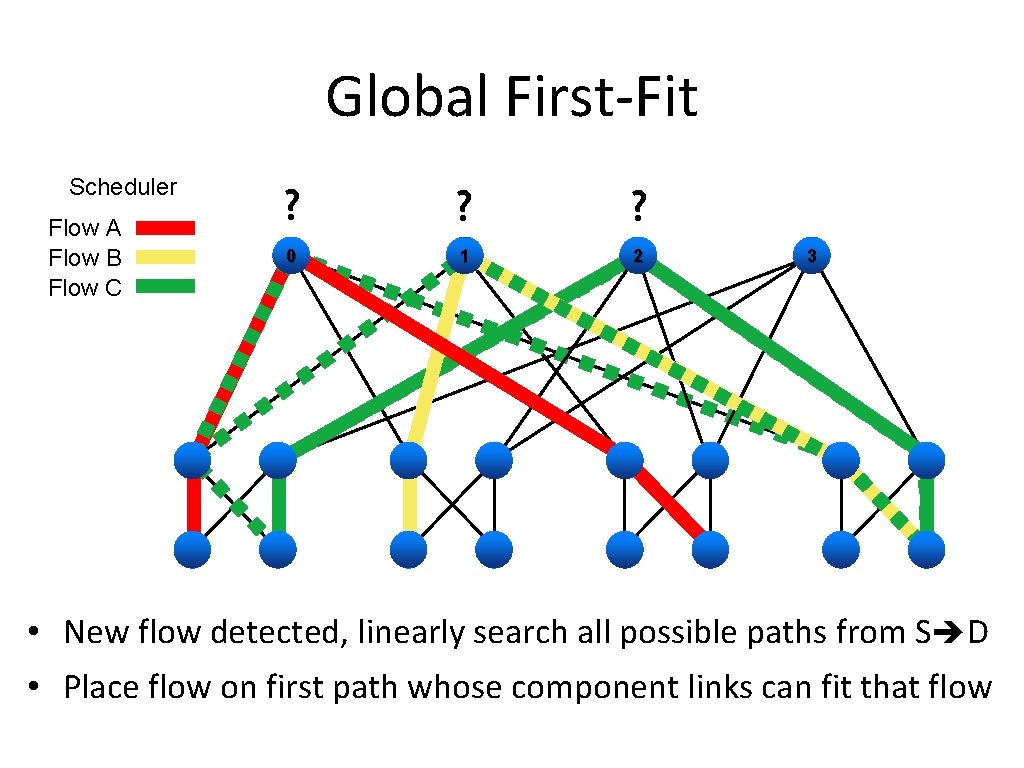
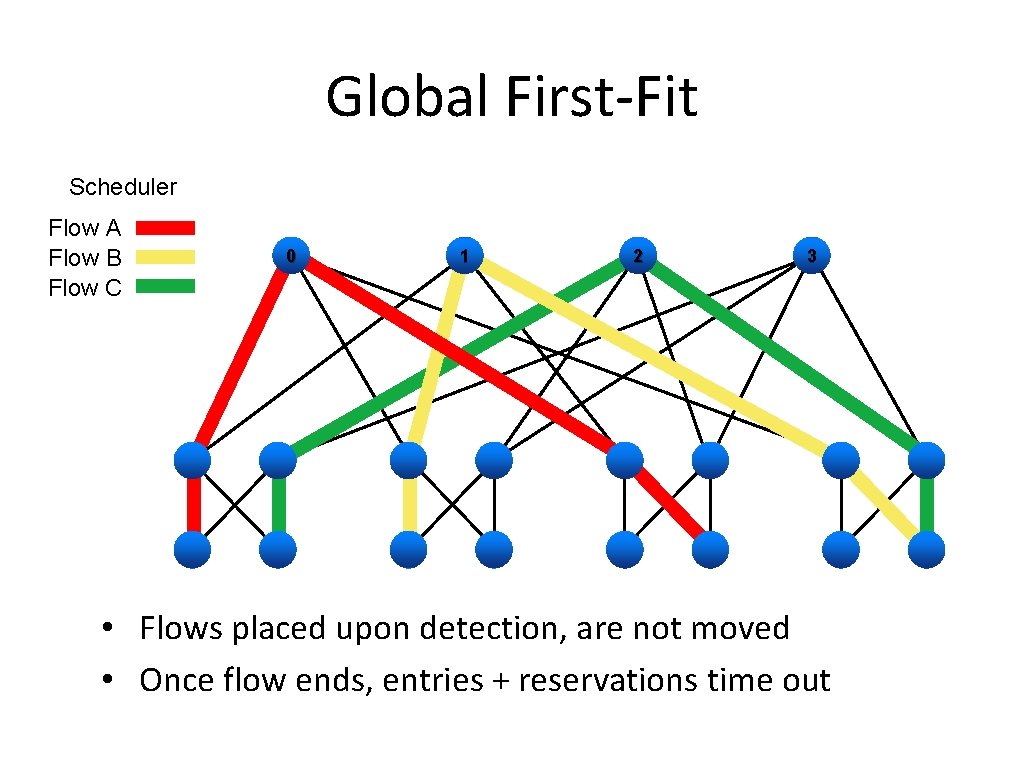
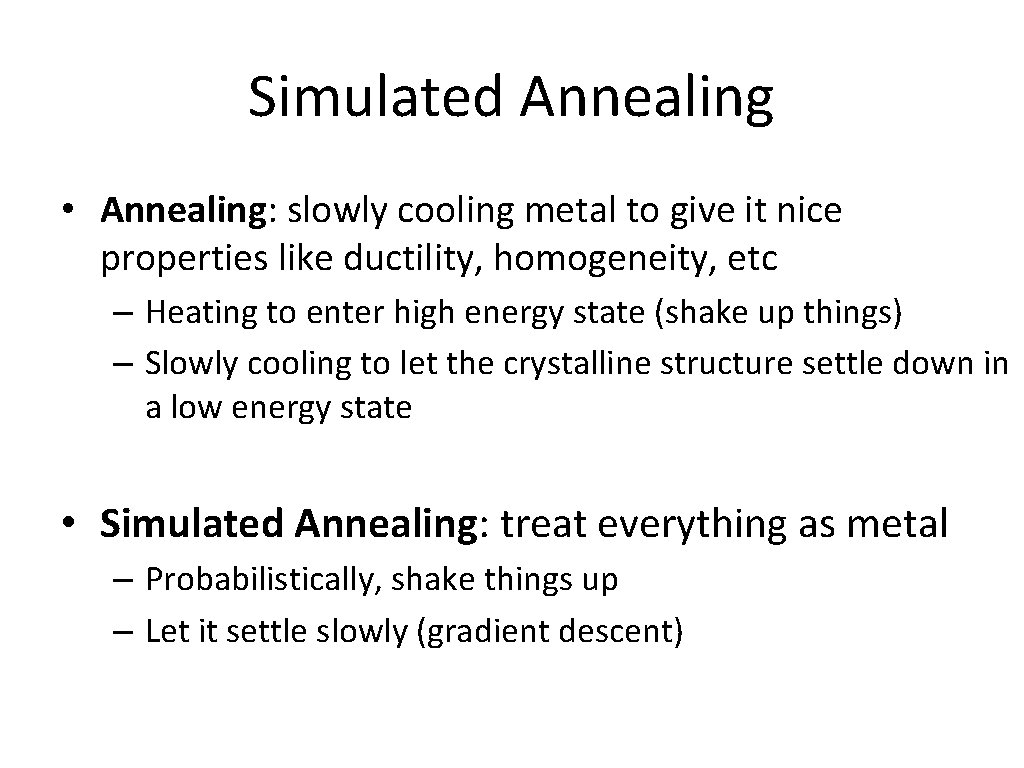
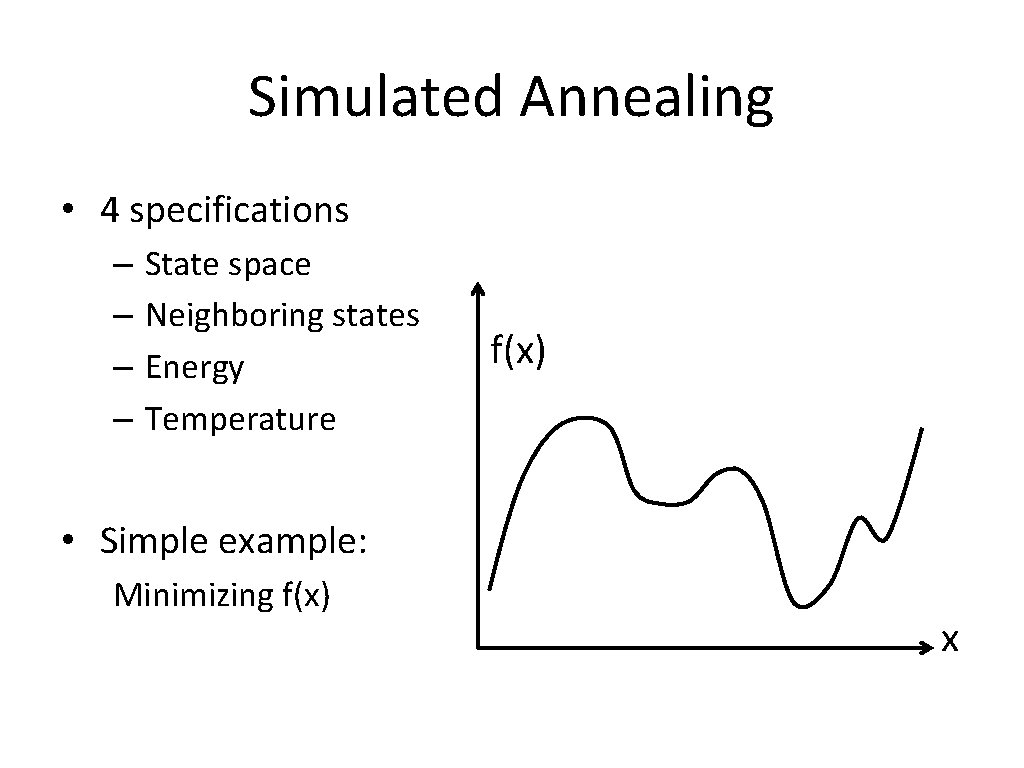
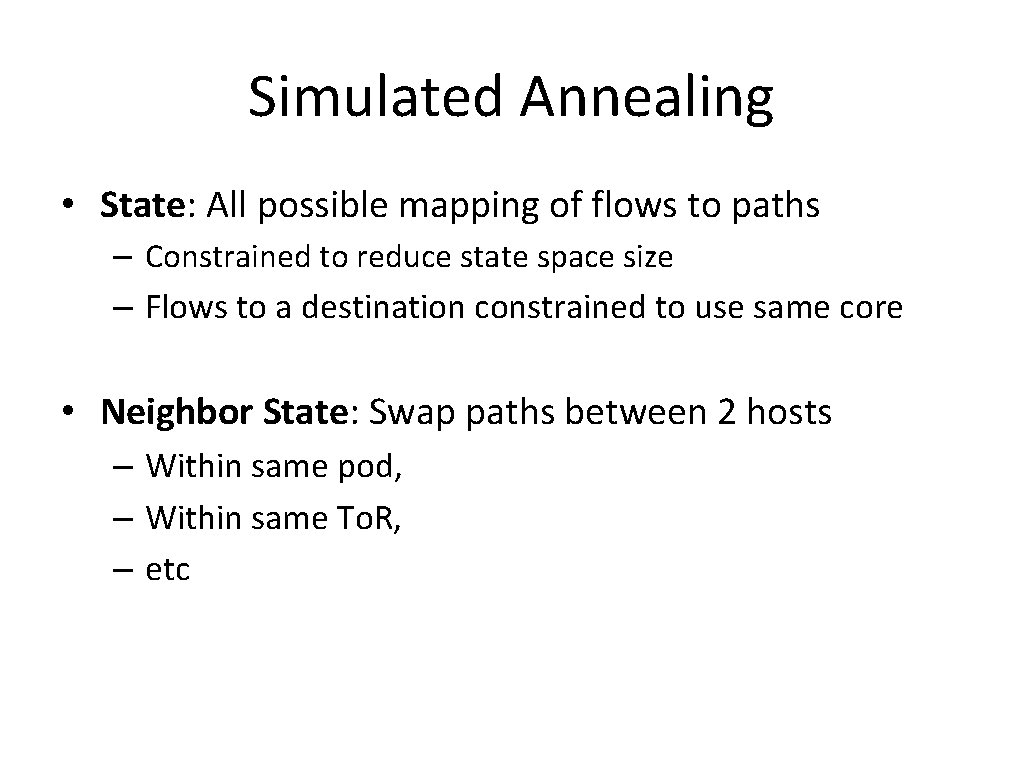
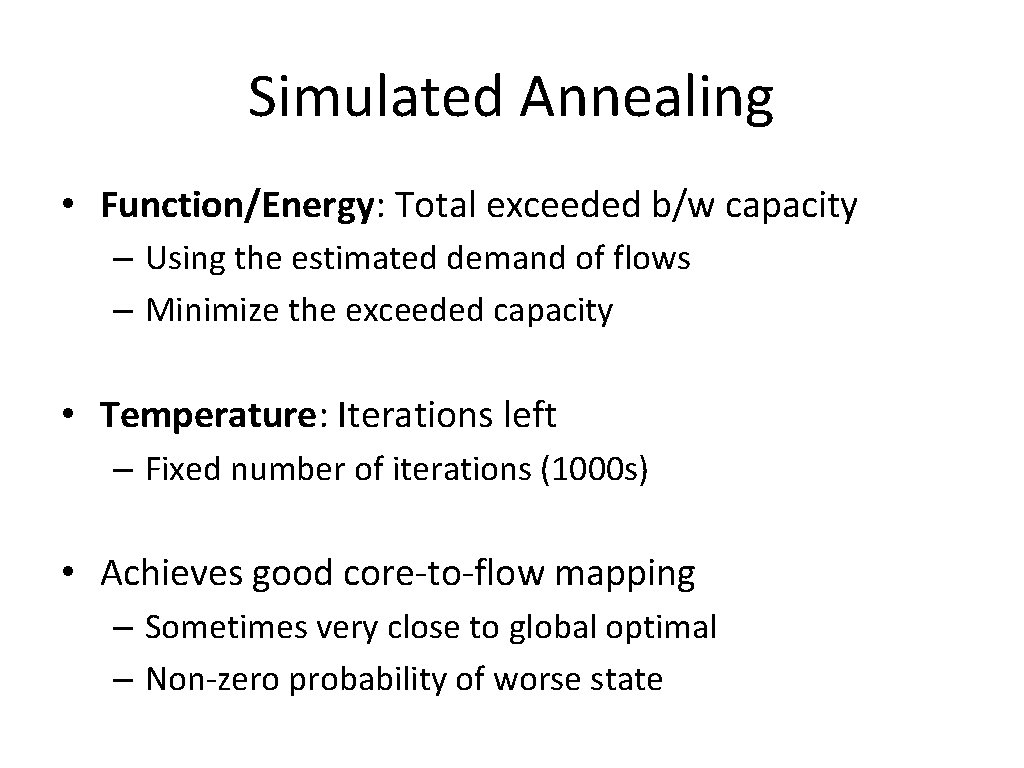
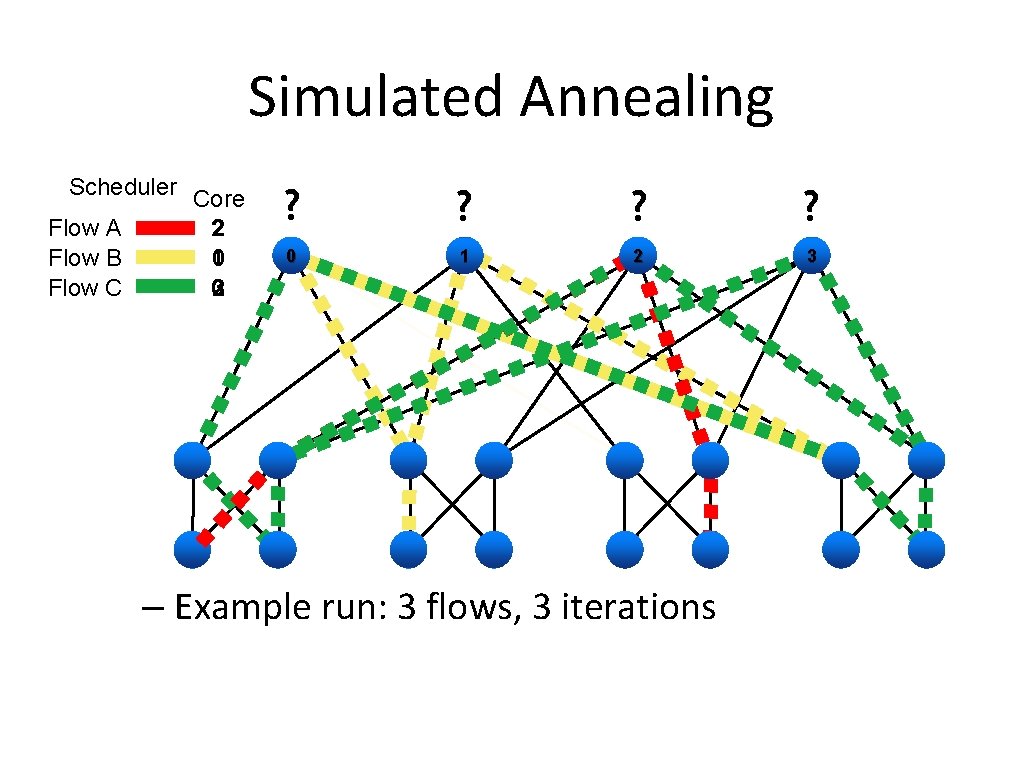
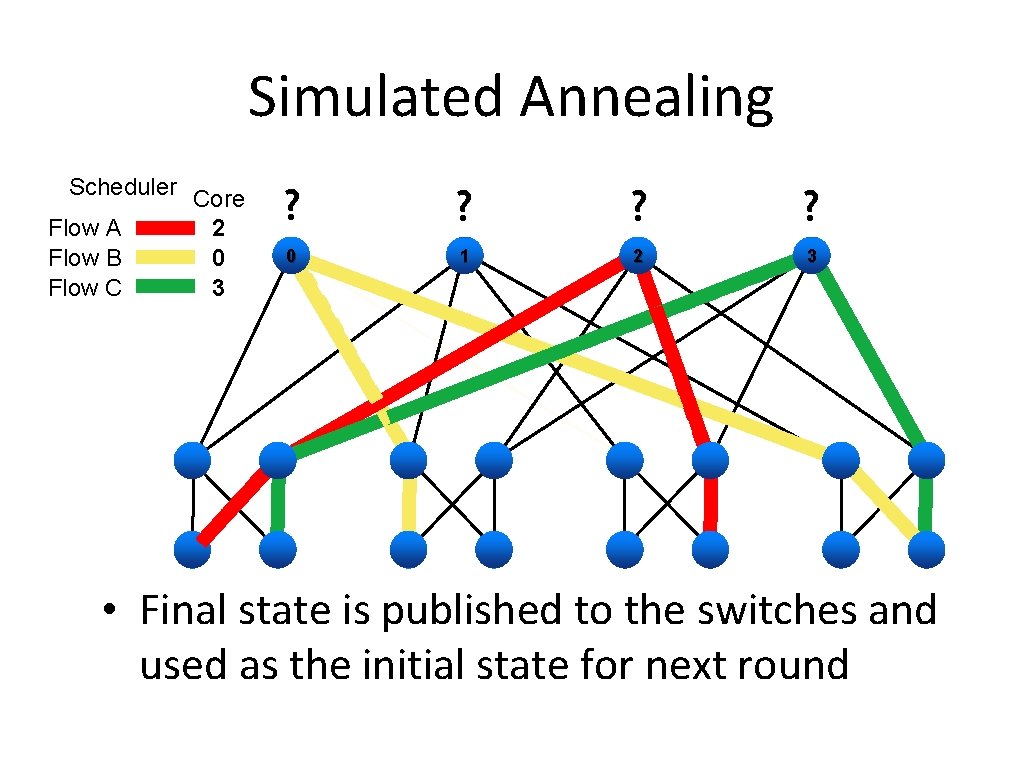
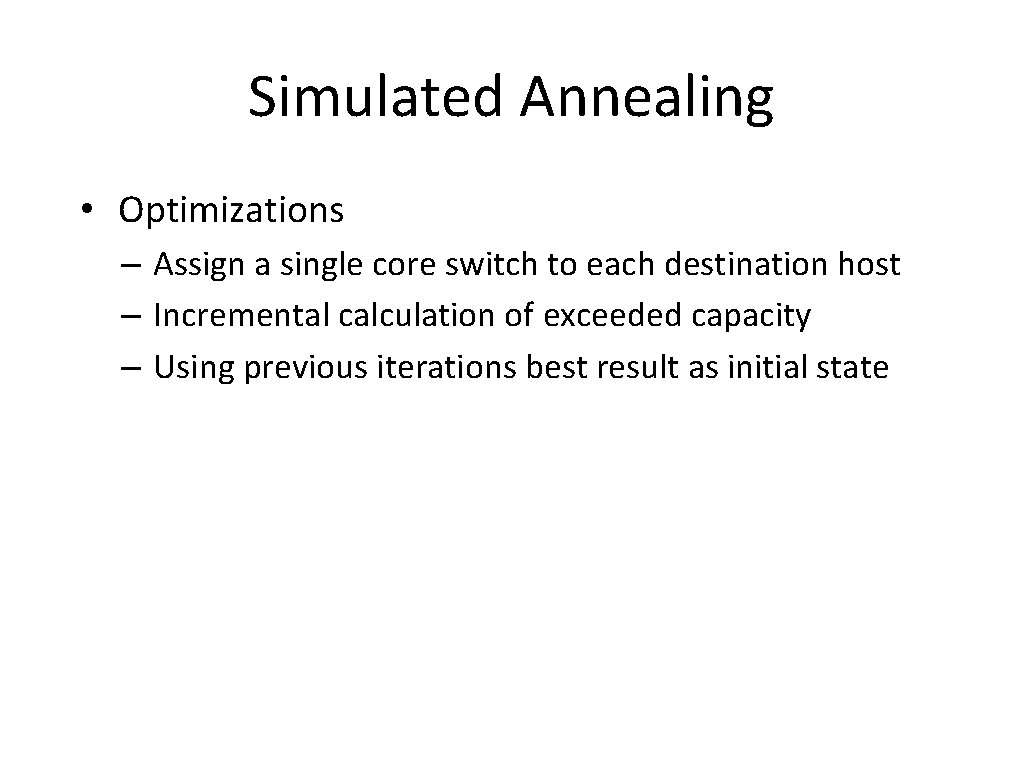
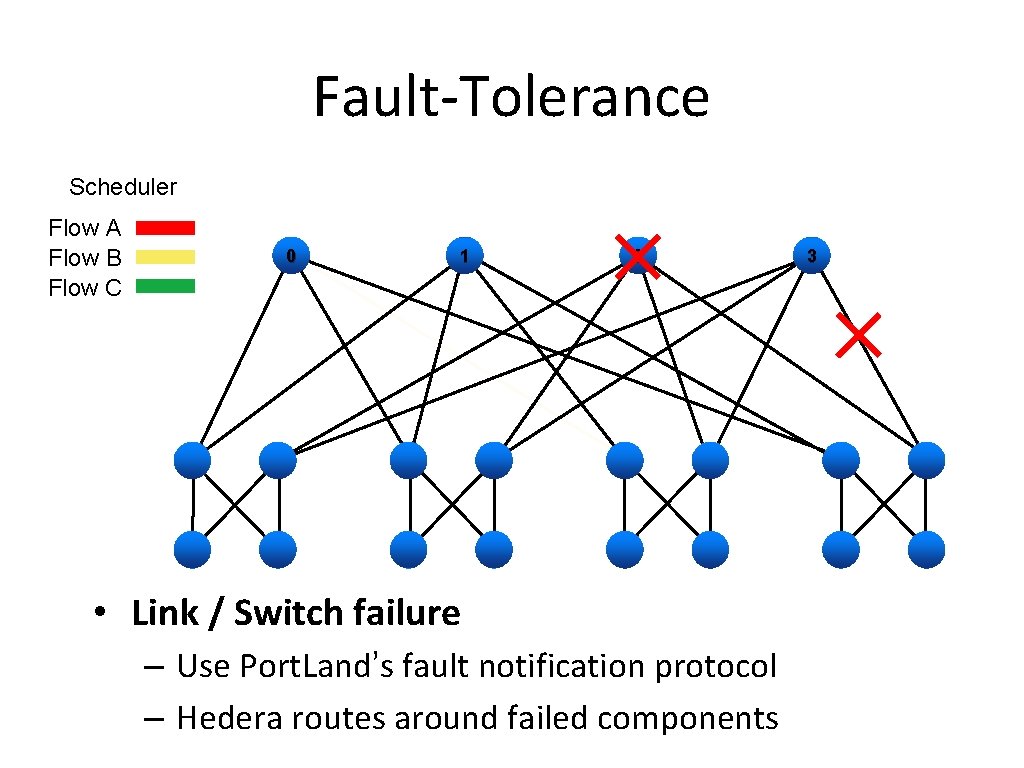
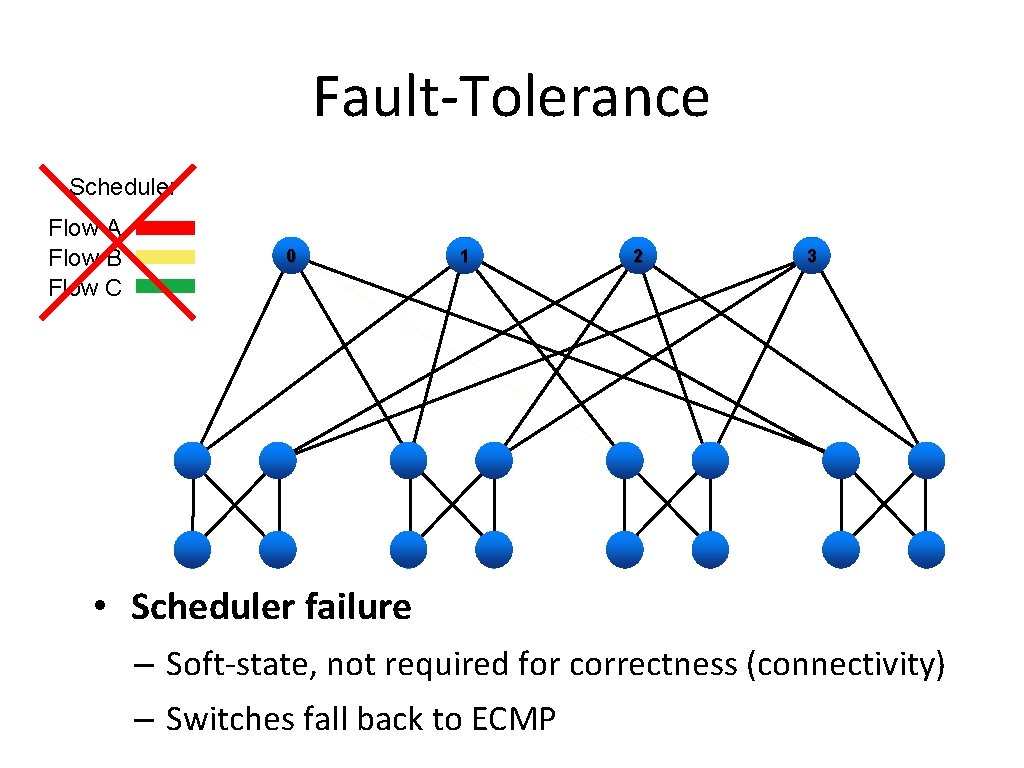
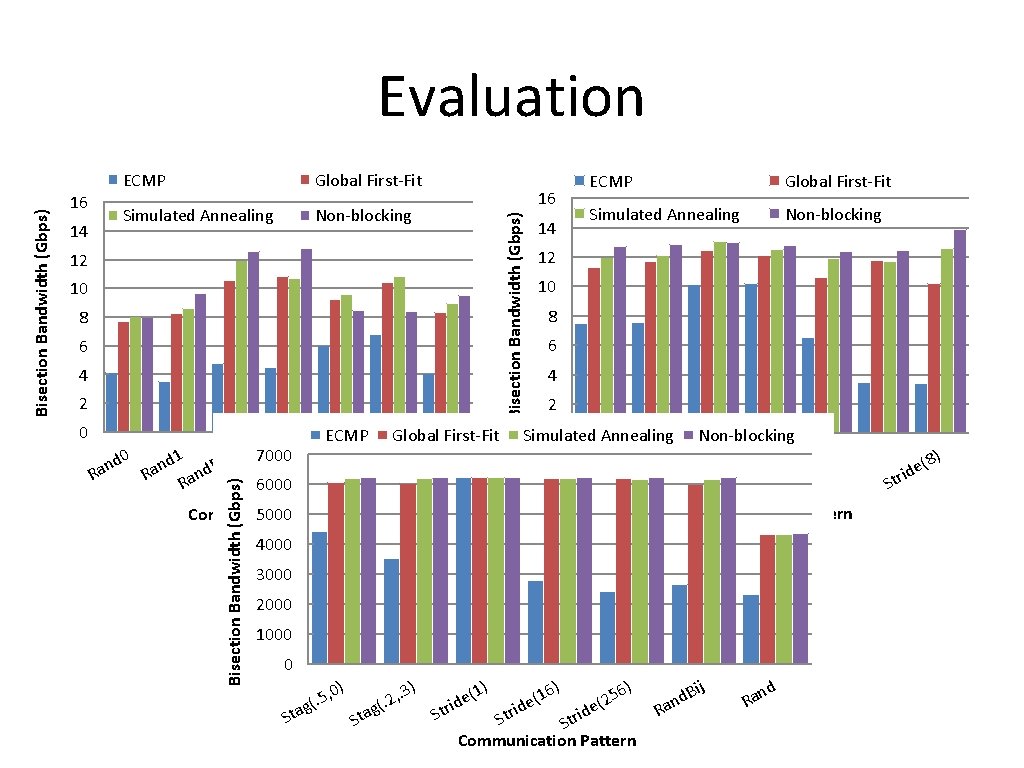
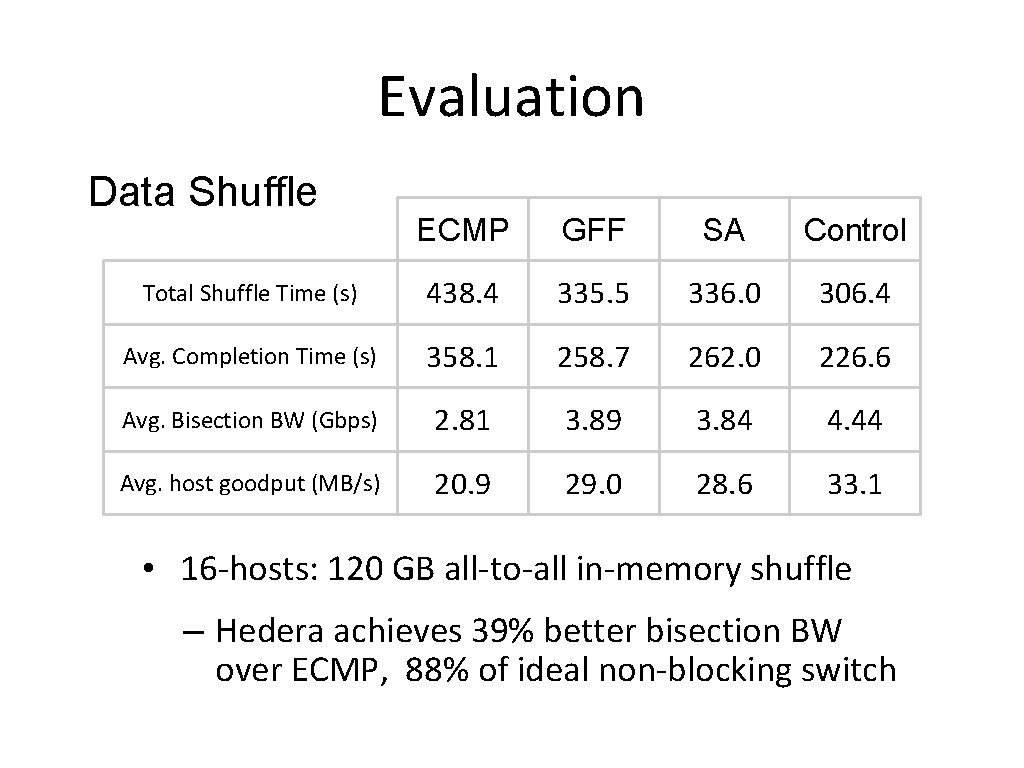
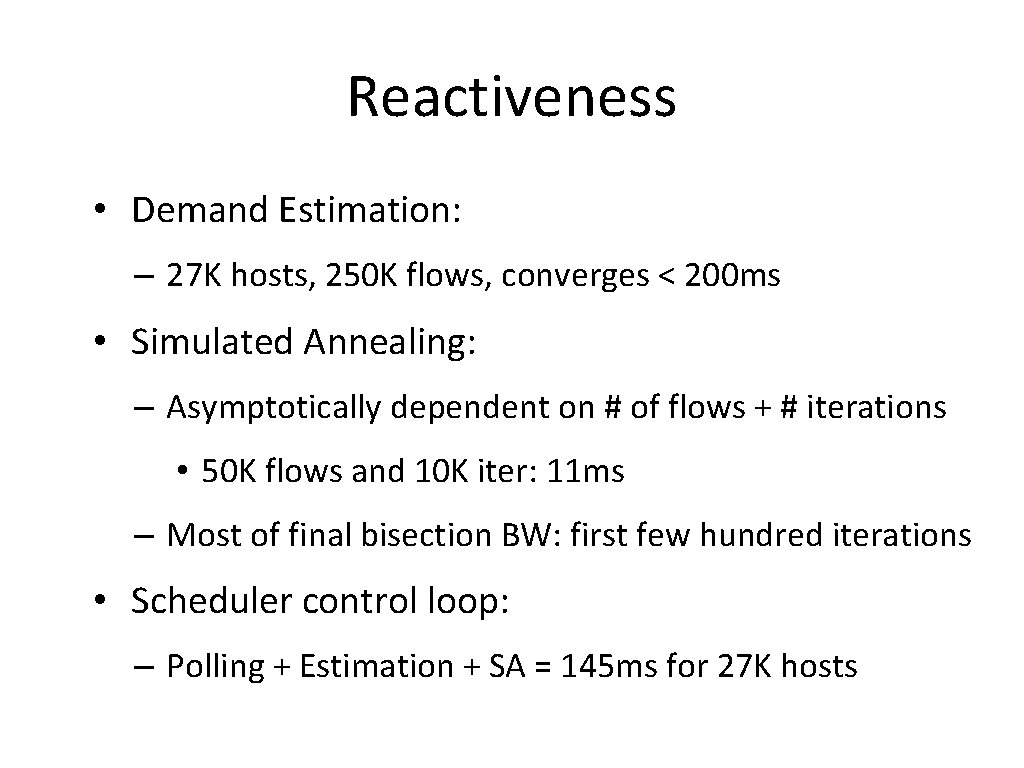
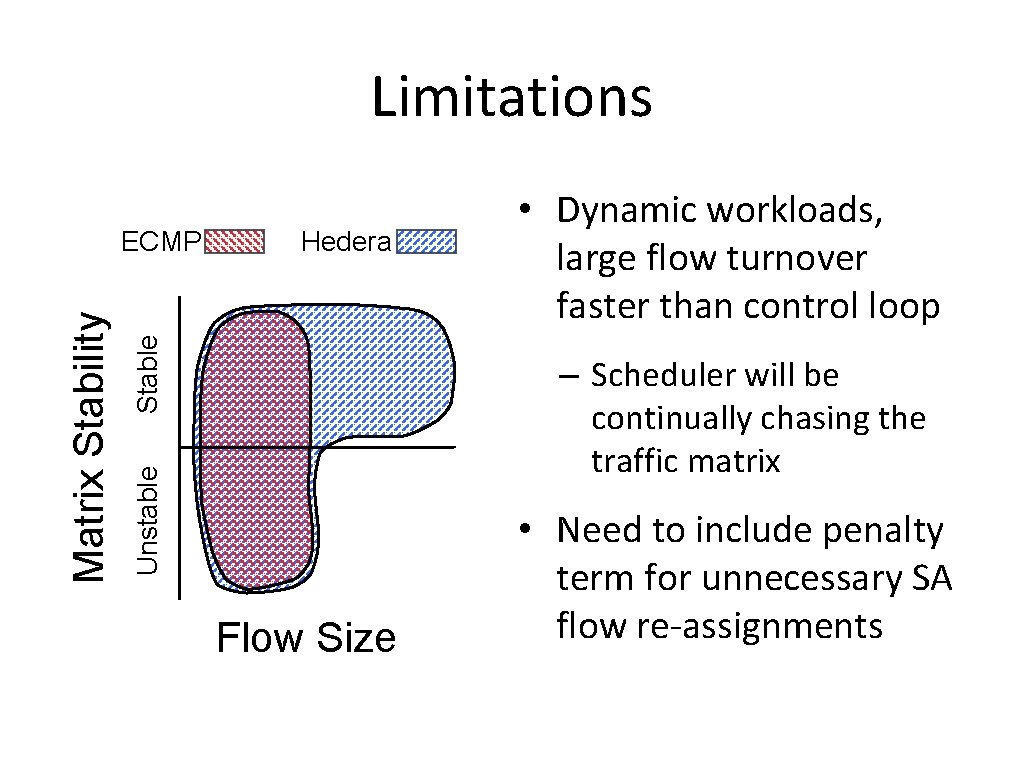
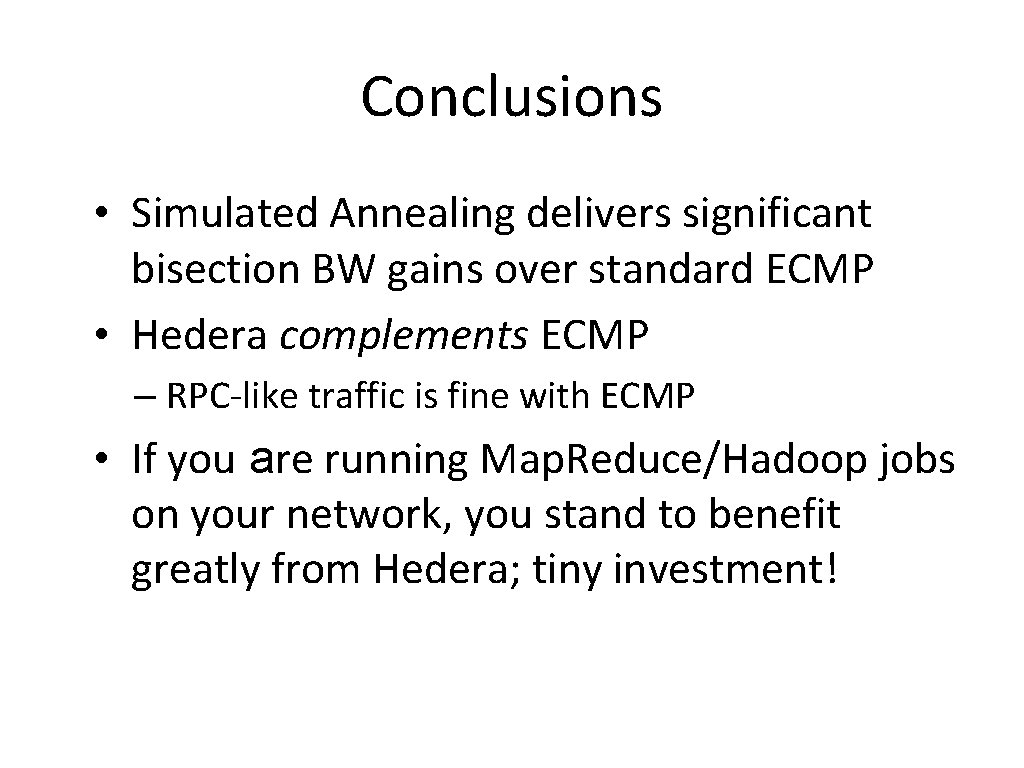
![Thoughts [1] Inconsistencies • Motivation is Map. Reduce, but Demand Estimation assumes sparse traffic Thoughts [1] Inconsistencies • Motivation is Map. Reduce, but Demand Estimation assumes sparse traffic](https://slidetodoc.com/presentation_image_h/defe7a62270bb8e3b7cec43167763eb8/image-31.jpg)
![Thoughts [2] Inconsistencies • Evaluation Results (already discussed) • Simulation using their own flow-level Thoughts [2] Inconsistencies • Evaluation Results (already discussed) • Simulation using their own flow-level](https://slidetodoc.com/presentation_image_h/defe7a62270bb8e3b7cec43167763eb8/image-32.jpg)
![Thoughts [3] • Periodicity of 5 seconds – Sufficient? – Limit? – Scalable to Thoughts [3] • Periodicity of 5 seconds – Sufficient? – Limit? – Scalable to](https://slidetodoc.com/presentation_image_h/defe7a62270bb8e3b7cec43167763eb8/image-33.jpg)
- Slides: 33
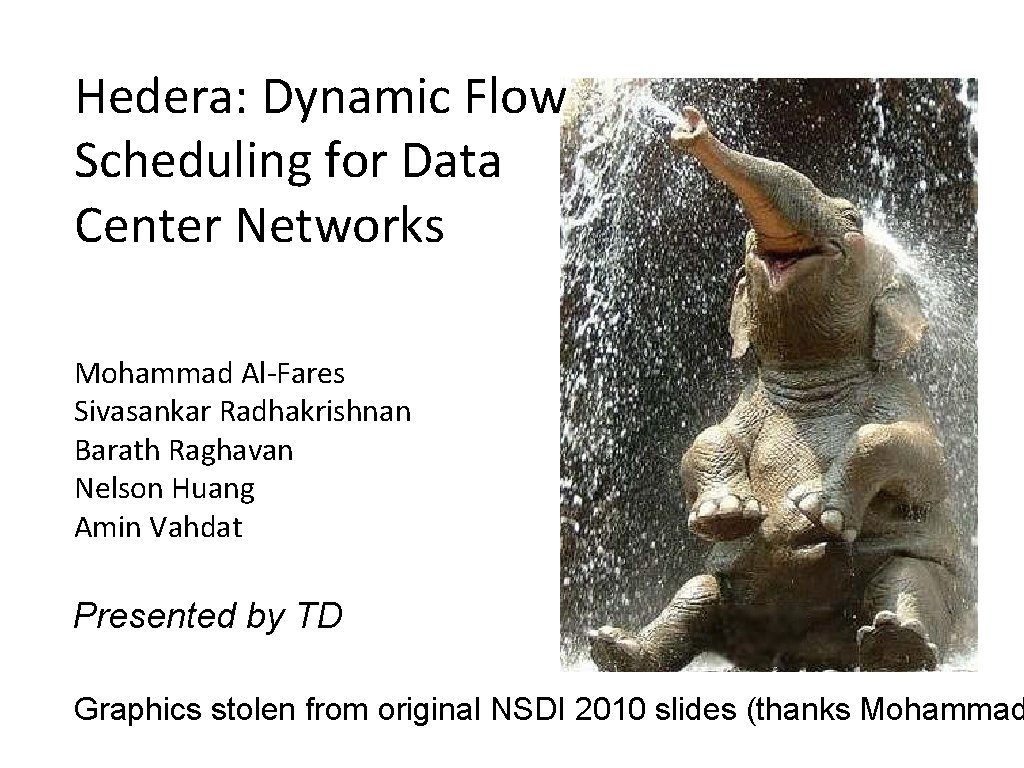
Hedera: Dynamic Flow Scheduling for Data Center Networks Mohammad Al-Fares Sivasankar Radhakrishnan Barath Raghavan Nelson Huang Amin Vahdat Presented by TD Graphics stolen from original NSDI 2010 slides (thanks Mohammad
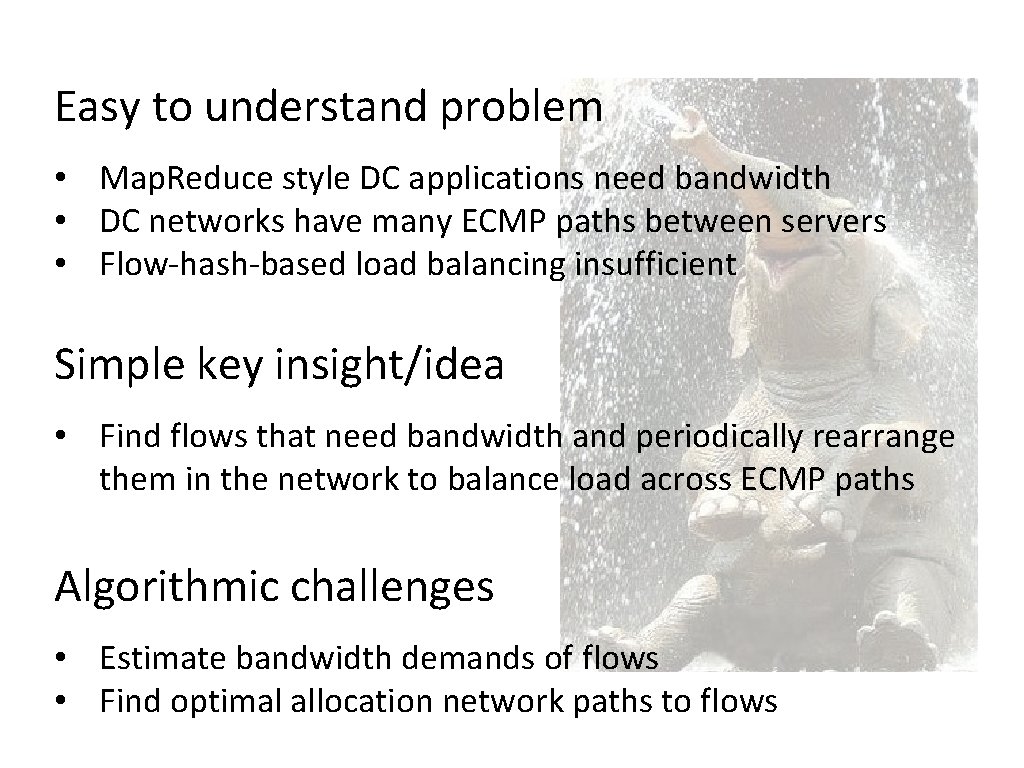
Easy to understand problem • Map. Reduce style DC applications need bandwidth • DC networks have many ECMP paths between servers • Flow-hash-based load balancing insufficient Simple key insight/idea • Find flows that need bandwidth and periodically rearrange them in the network to balance load across ECMP paths Algorithmic challenges • Estimate bandwidth demands of flows • Find optimal allocation network paths to flows
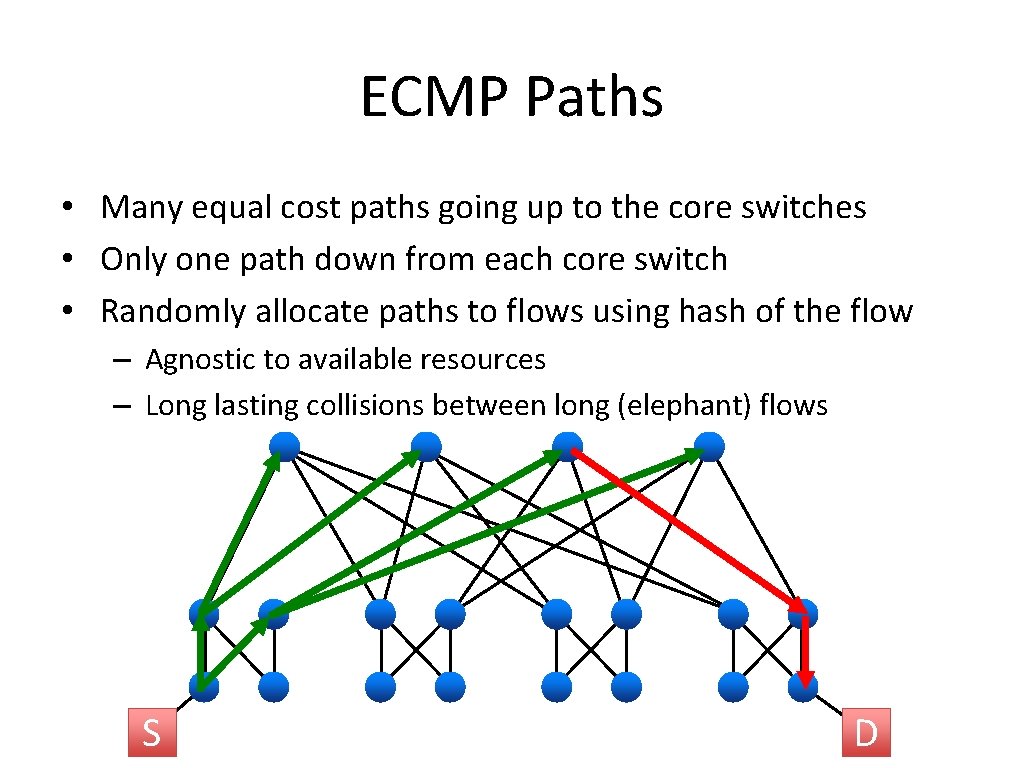
ECMP Paths • Many equal cost paths going up to the core switches • Only one path down from each core switch • Randomly allocate paths to flows using hash of the flow – Agnostic to available resources – Long lasting collisions between long (elephant) flows S D
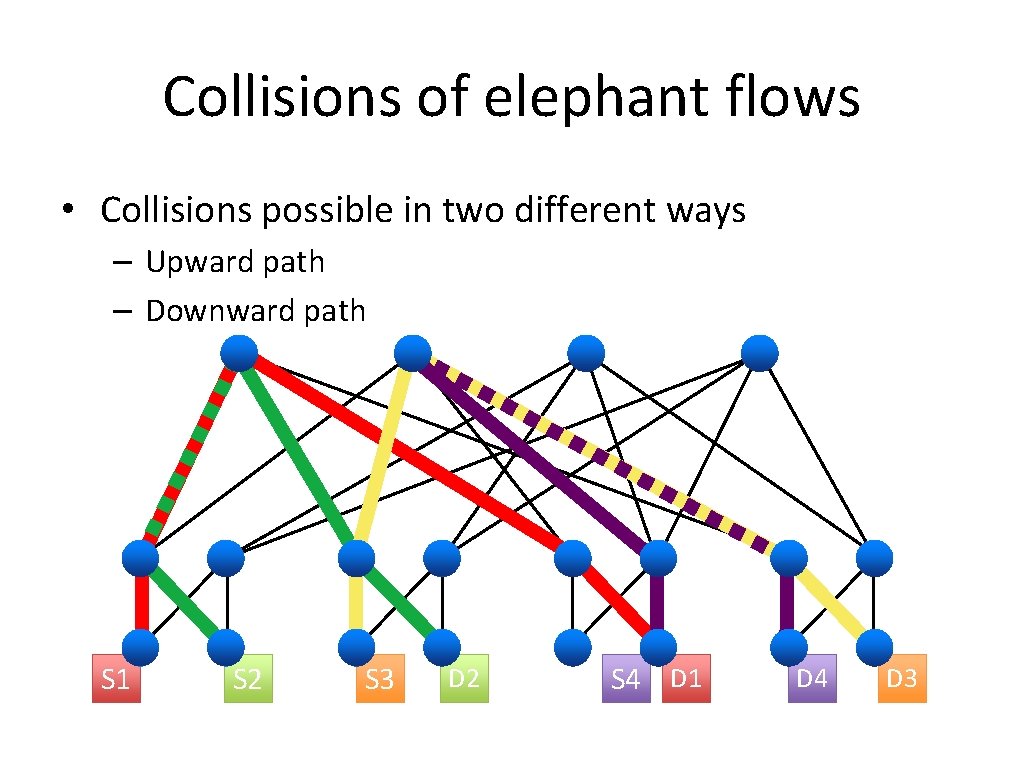
Collisions of elephant flows • Collisions possible in two different ways – Upward path – Downward path S 1 S 2 S 3 D 2 S 4 D 1 D 4 D 3
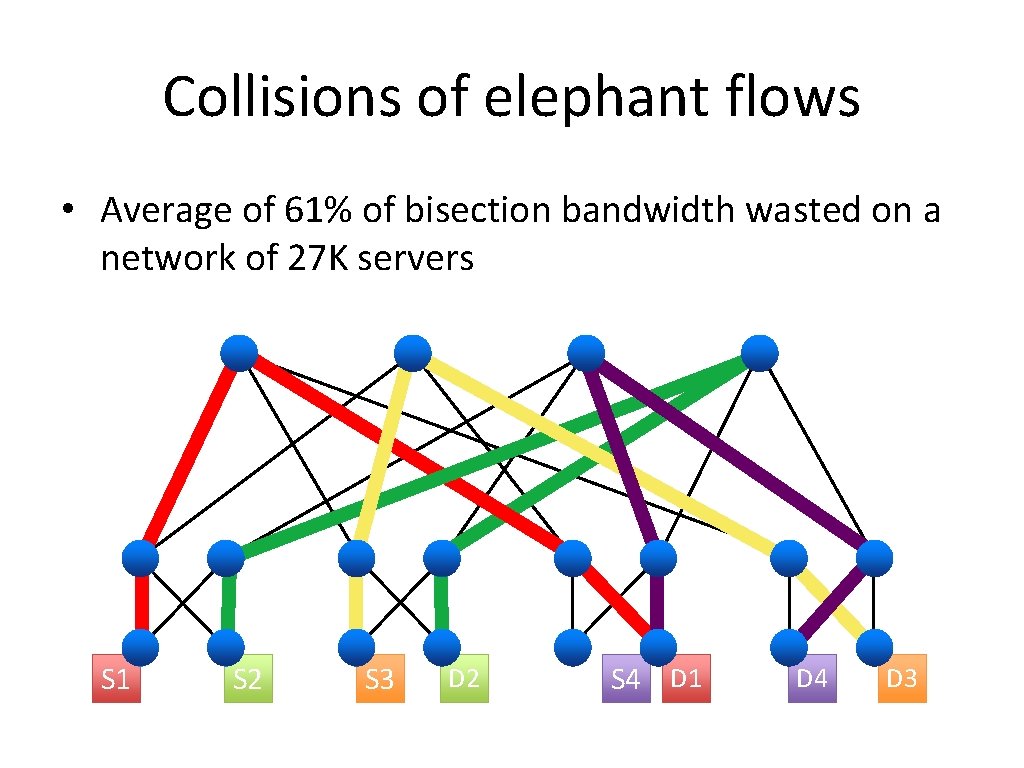
Collisions of elephant flows • Average of 61% of bisection bandwidth wasted on a network of 27 K servers S 1 S 2 S 3 D 2 S 4 D 1 D 4 D 3
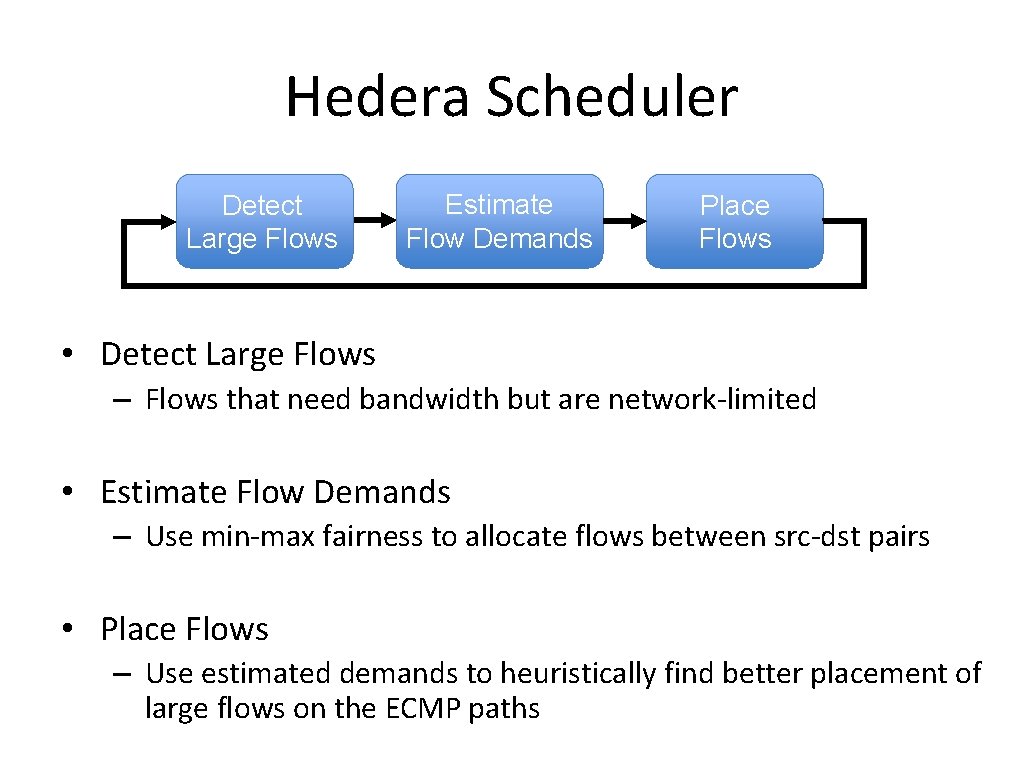
Hedera Scheduler Detect Large Flows Estimate Flow Demands Place Flows • Detect Large Flows – Flows that need bandwidth but are network-limited • Estimate Flow Demands – Use min-max fairness to allocate flows between src-dst pairs • Place Flows – Use estimated demands to heuristically find better placement of large flows on the ECMP paths
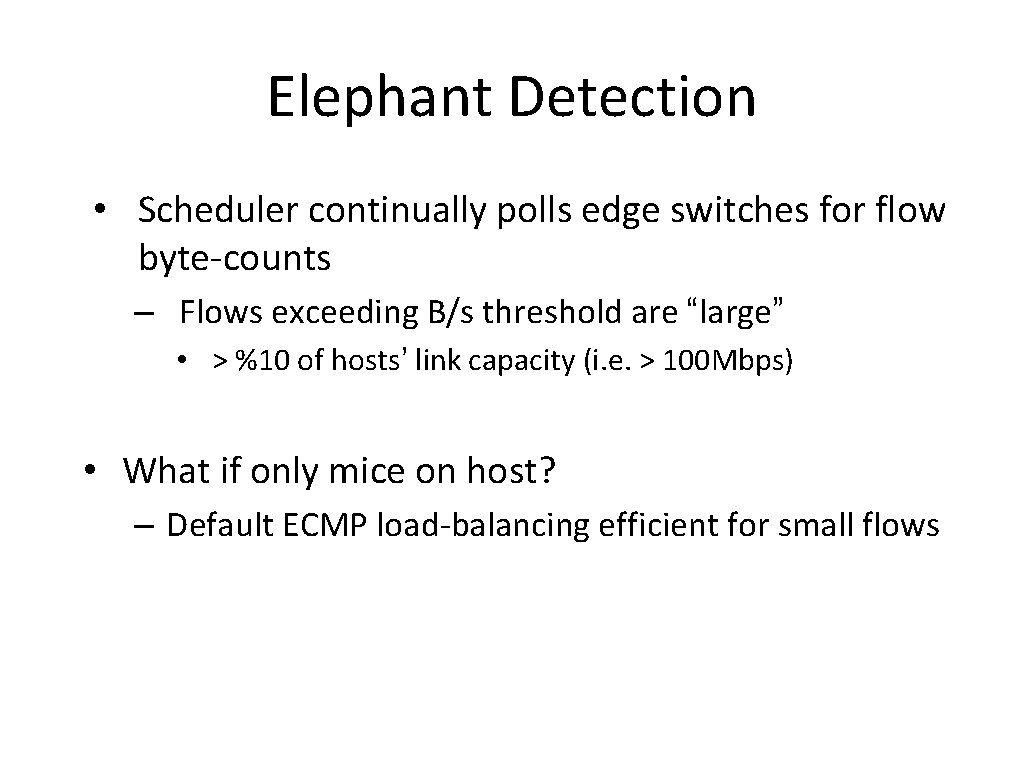
Elephant Detection • Scheduler continually polls edge switches for flow byte-counts – Flows exceeding B/s threshold are “large” • > %10 of hosts’ link capacity (i. e. > 100 Mbps) • What if only mice on host? – Default ECMP load-balancing efficient for small flows
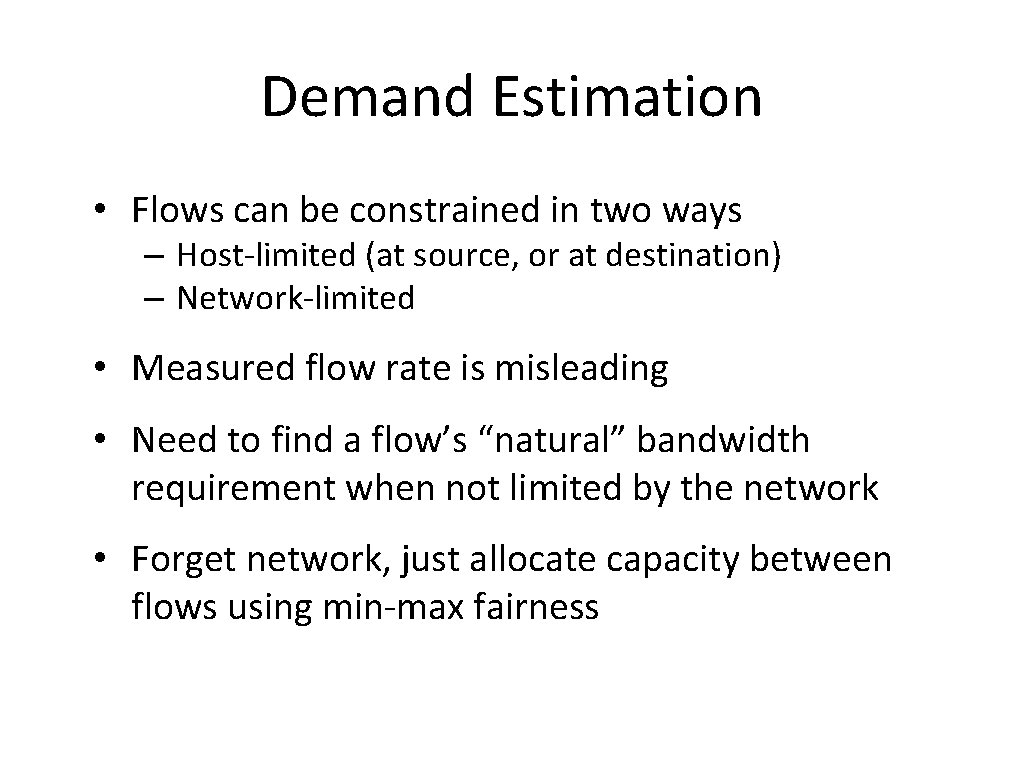
Demand Estimation • Flows can be constrained in two ways – Host-limited (at source, or at destination) – Network-limited • Measured flow rate is misleading • Need to find a flow’s “natural” bandwidth requirement when not limited by the network • Forget network, just allocate capacity between flows using min-max fairness
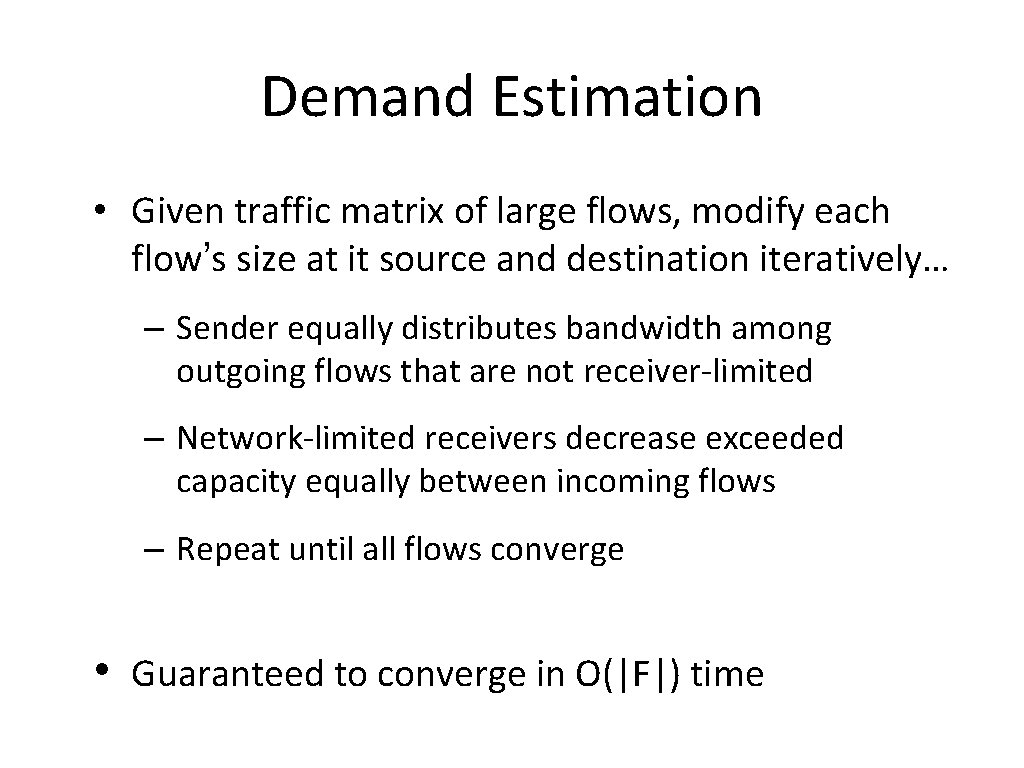
Demand Estimation • Given traffic matrix of large flows, modify each flow’s size at it source and destination iteratively… – Sender equally distributes bandwidth among outgoing flows that are not receiver-limited – Network-limited receivers decrease exceeded capacity equally between incoming flows – Repeat until all flows converge • Guaranteed to converge in O(|F|) time
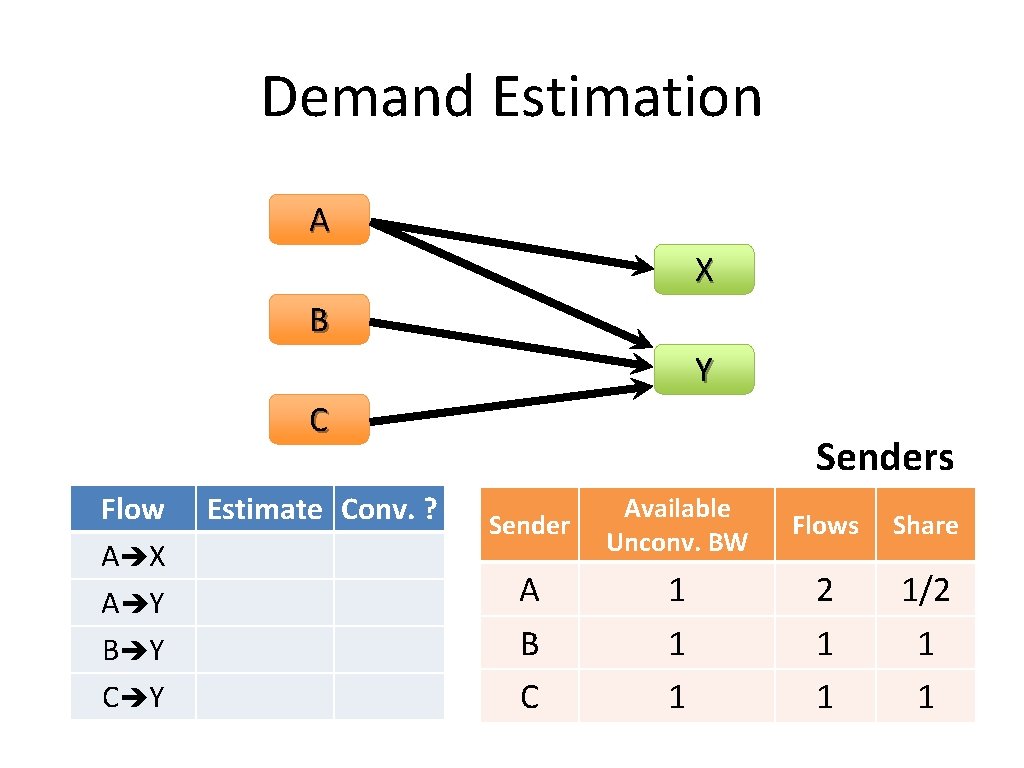
Demand Estimation A X B Y C Flow A X A Y B Y C Y Estimate Conv. ? Senders Sender Available Unconv. BW Flows Share A B C 1 1 1 2 1 1 1/2 1 1
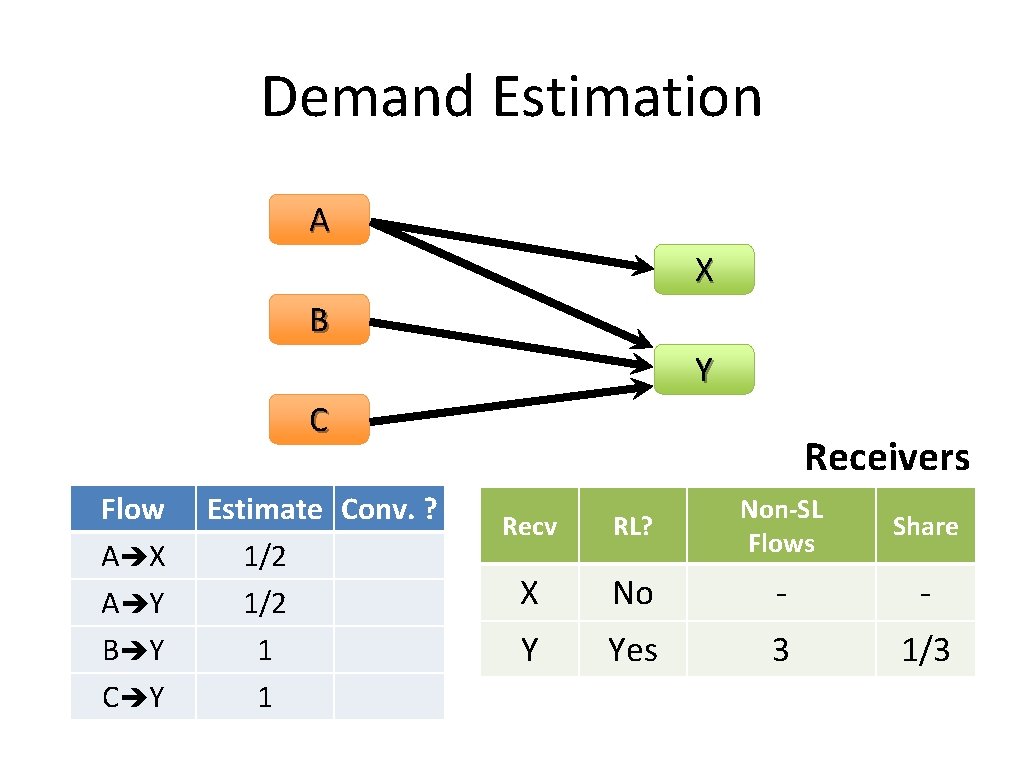
Demand Estimation A X B Y C Flow A X A Y B Y C Y Estimate Conv. ? 1/2 1 1 Receivers Recv RL? Non-SL Flows X No - - Y Yes 3 1/3 Share
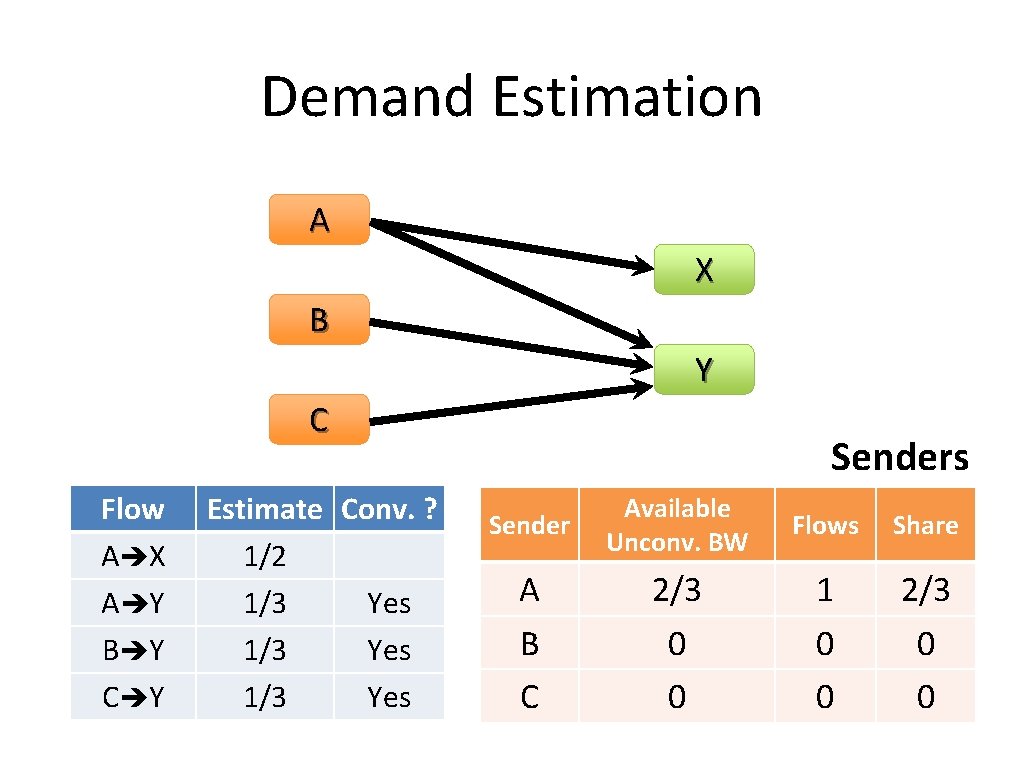
Demand Estimation A X B Y C Flow A X A Y B Y C Y Estimate Conv. ? 1/2 1/3 Yes Sender Available Unconv. BW Flows Share A B C 2/3 0 0 1 0 0 2/3 0 0
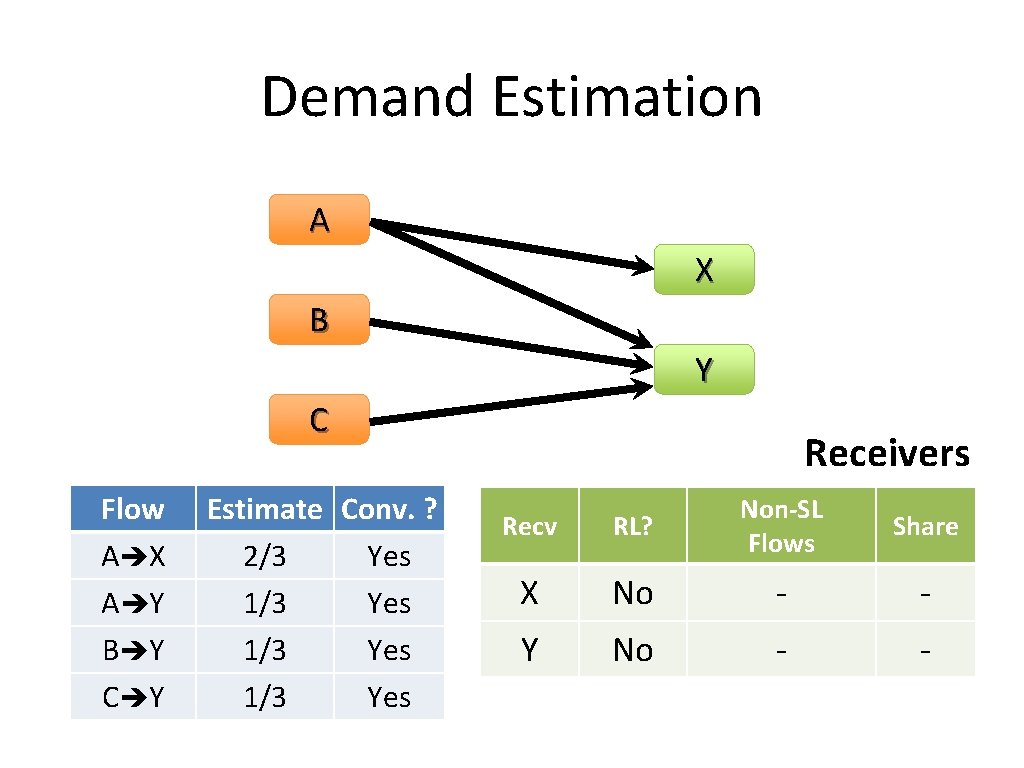
Demand Estimation A X B Y C Flow A X A Y B Y C Y Estimate Conv. ? 2/3 Yes 1/3 Yes Receivers Recv RL? Non-SL Flows X No - - Y No - - Share
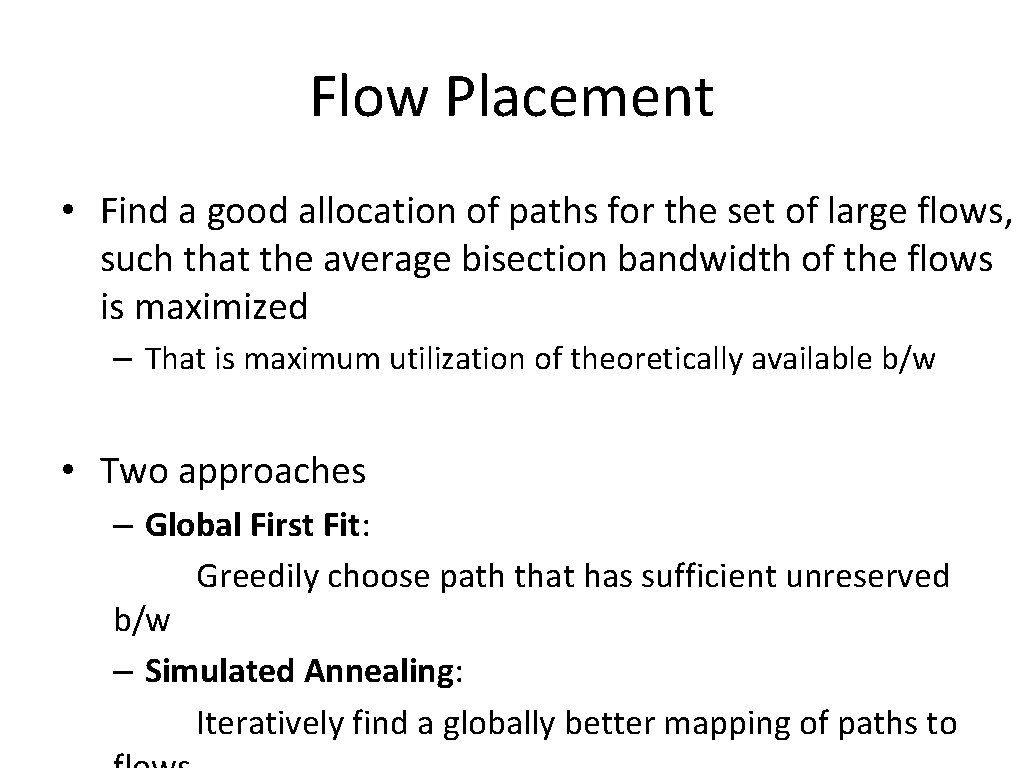
Flow Placement • Find a good allocation of paths for the set of large flows, such that the average bisection bandwidth of the flows is maximized – That is maximum utilization of theoretically available b/w • Two approaches – Global First Fit: Greedily choose path that has sufficient unreserved b/w – Simulated Annealing: Iteratively find a globally better mapping of paths to
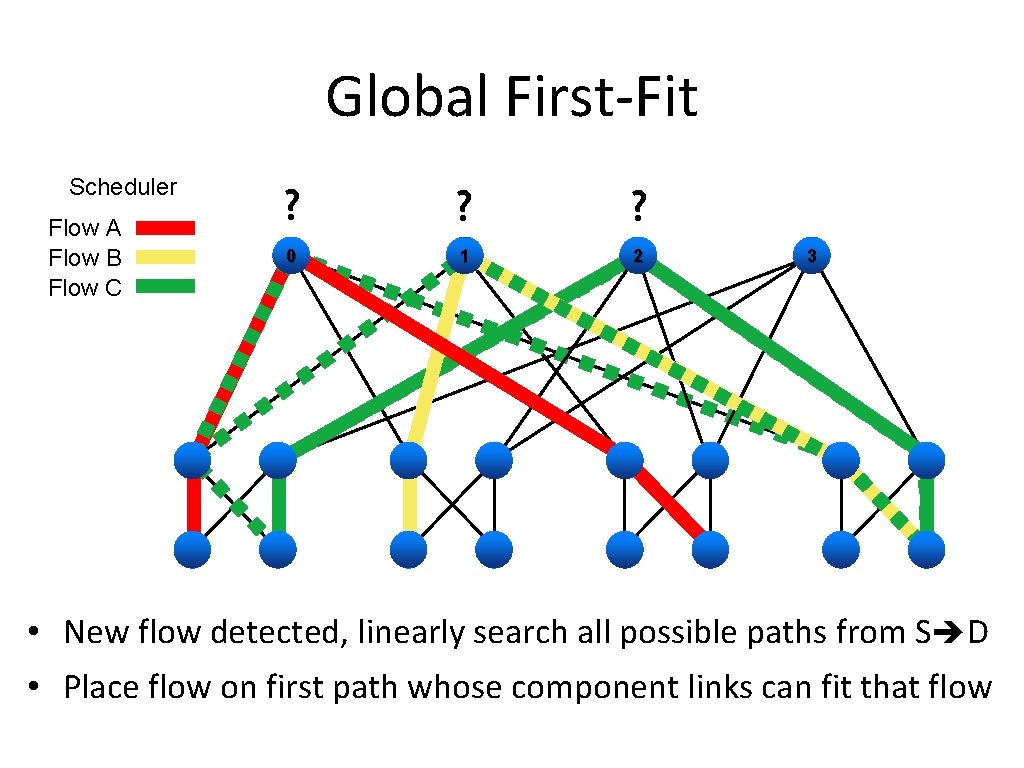
Global First-Fit Scheduler Flow A Flow B Flow C ? ? ? 0 1 2 3 • New flow detected, linearly search all possible paths from S D • Place flow on first path whose component links can fit that flow
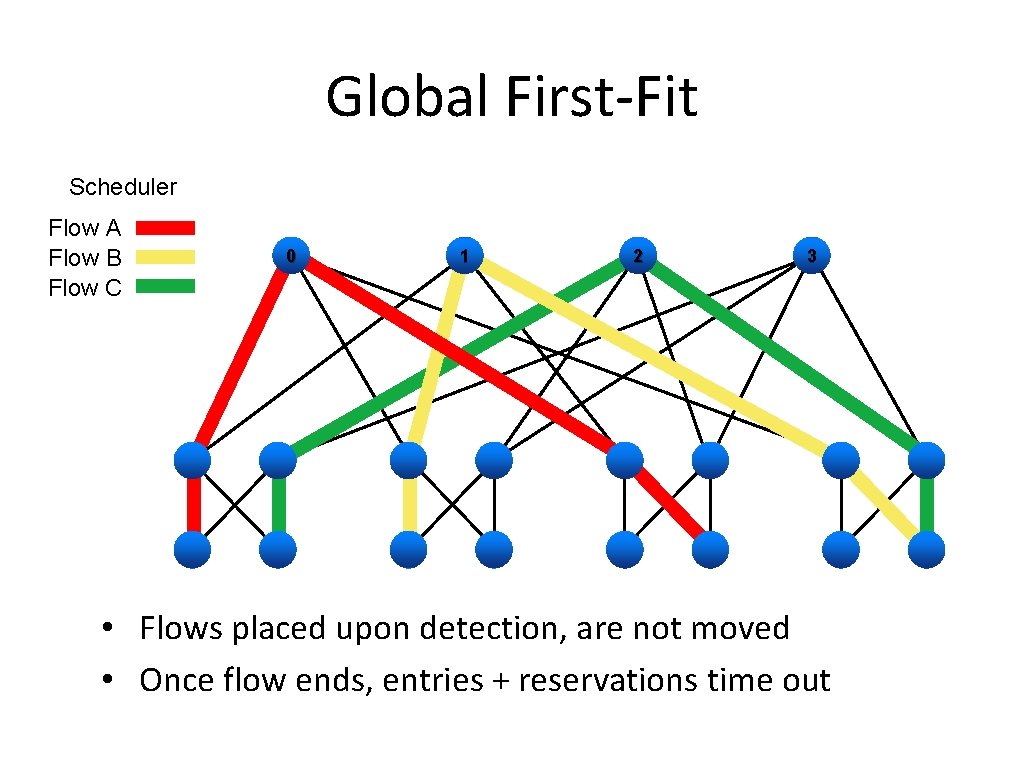
Global First-Fit Scheduler Flow A Flow B Flow C 0 1 2 3 • Flows placed upon detection, are not moved • Once flow ends, entries + reservations time out
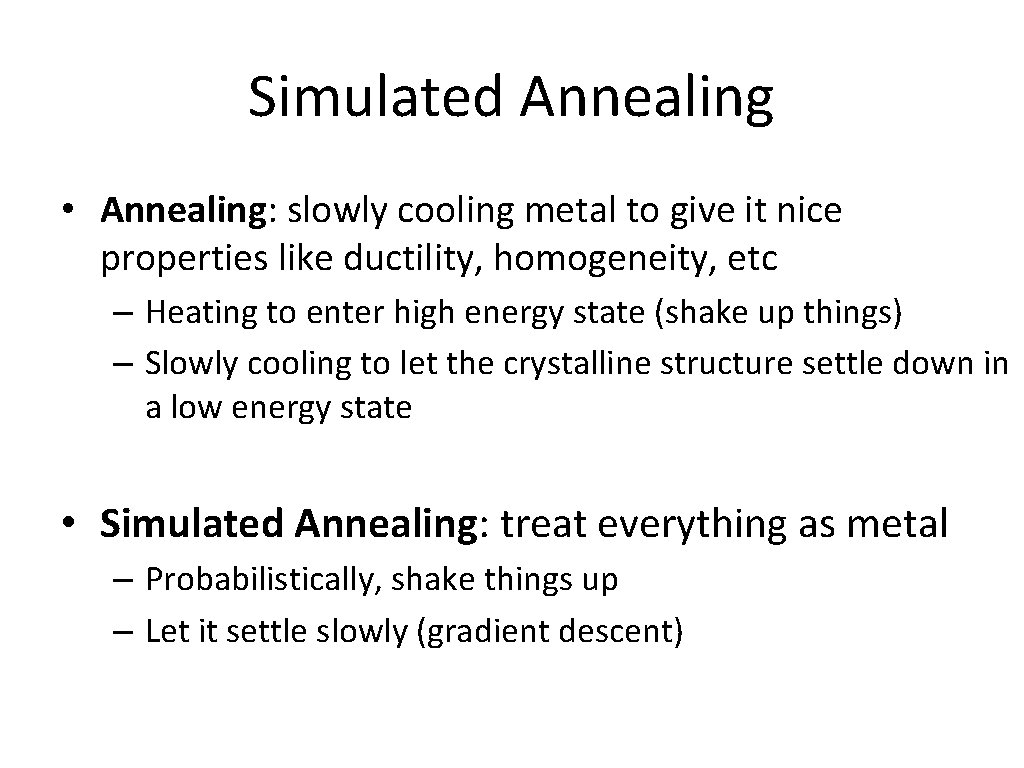
Simulated Annealing • Annealing: slowly cooling metal to give it nice properties like ductility, homogeneity, etc – Heating to enter high energy state (shake up things) – Slowly cooling to let the crystalline structure settle down in a low energy state • Simulated Annealing: treat everything as metal – Probabilistically, shake things up – Let it settle slowly (gradient descent)
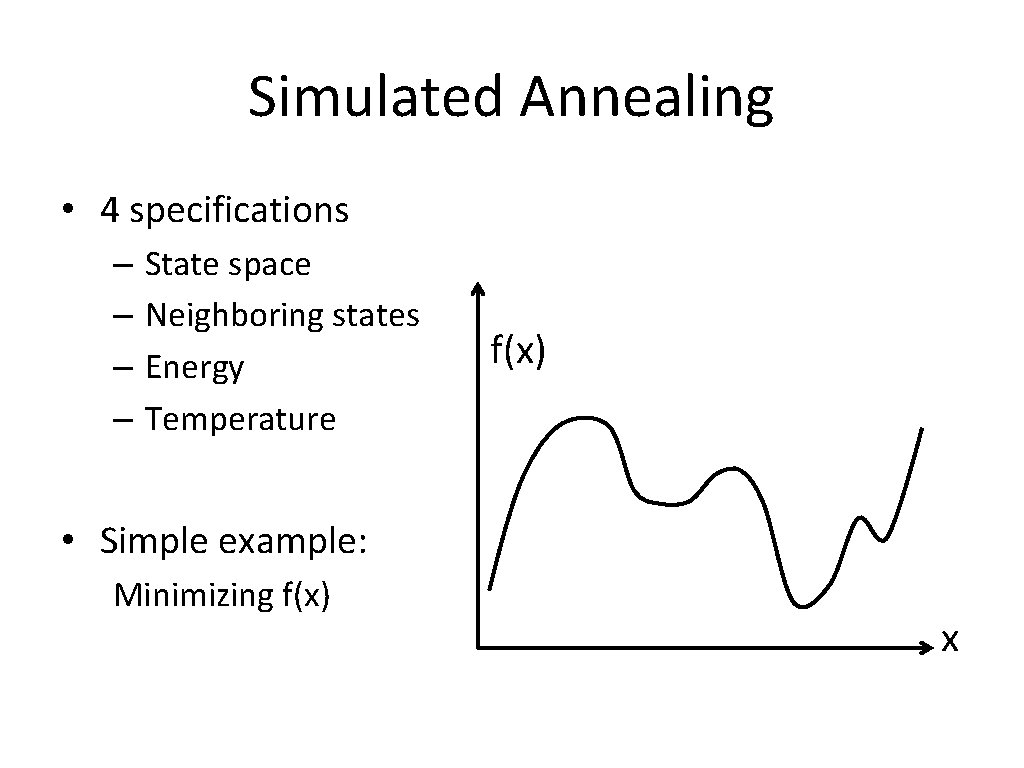
Simulated Annealing • 4 specifications – – State space Neighboring states Energy Temperature f(x) • Simple example: Minimizing f(x) x
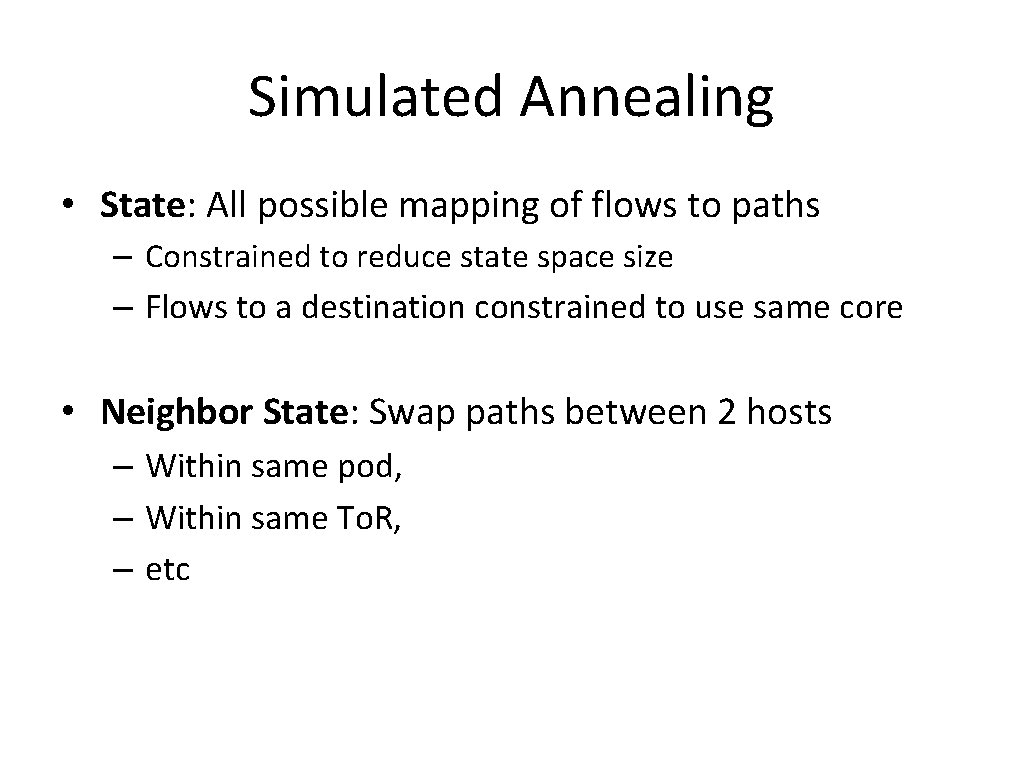
Simulated Annealing • State: All possible mapping of flows to paths – Constrained to reduce state space size – Flows to a destination constrained to use same core • Neighbor State: Swap paths between 2 hosts – Within same pod, – Within same To. R, – etc
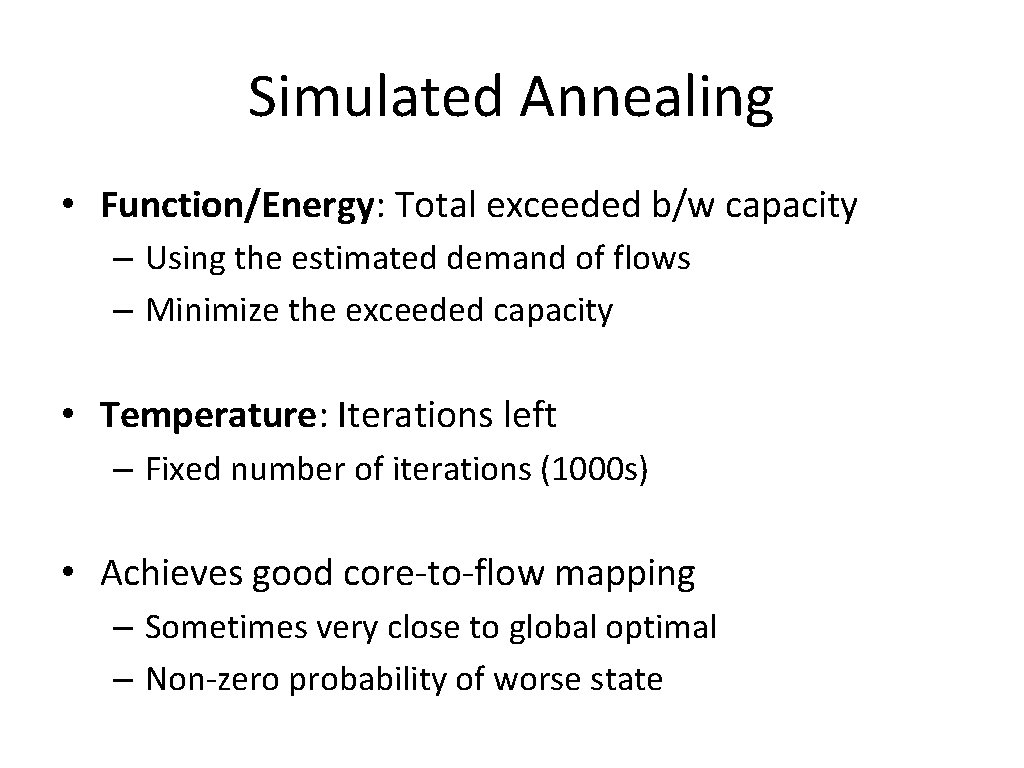
Simulated Annealing • Function/Energy: Total exceeded b/w capacity – Using the estimated demand of flows – Minimize the exceeded capacity • Temperature: Iterations left – Fixed number of iterations (1000 s) • Achieves good core-to-flow mapping – Sometimes very close to global optimal – Non-zero probability of worse state
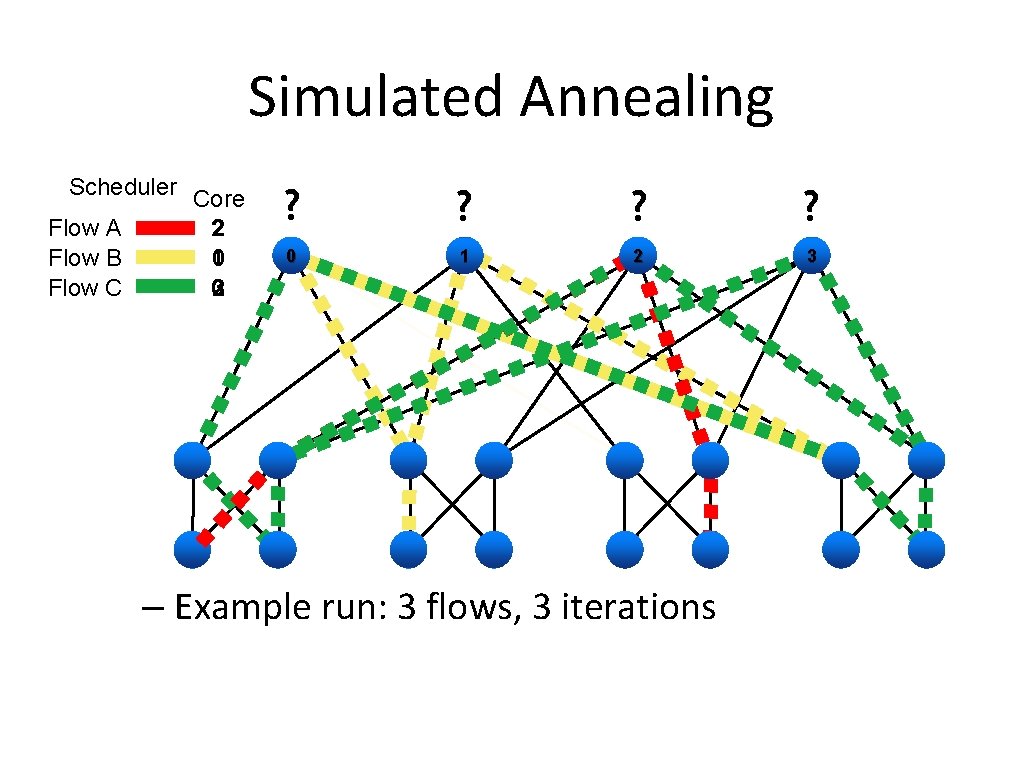
Simulated Annealing Scheduler Core Flow A 2 Flow B 1 0 Flow C 0 2 3 ? ? 0 1 2 3 – Example run: 3 flows, 3 iterations
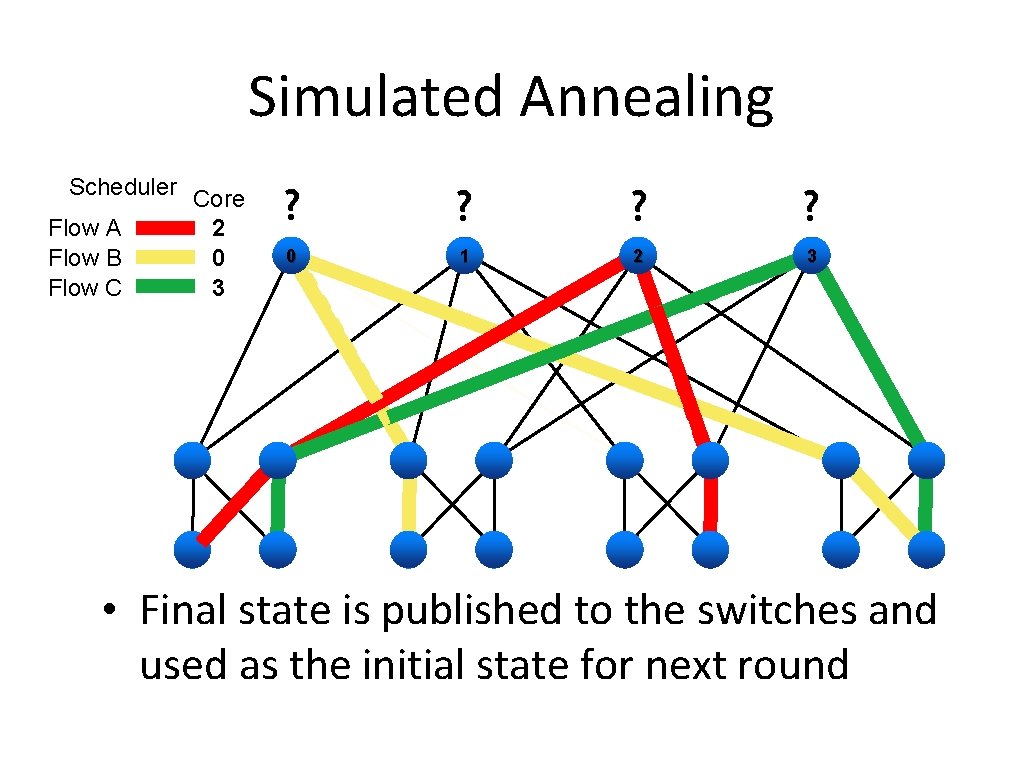
Simulated Annealing Scheduler Core Flow A 2 Flow B 0 Flow C 3 ? ? 0 1 2 3 • Final state is published to the switches and used as the initial state for next round
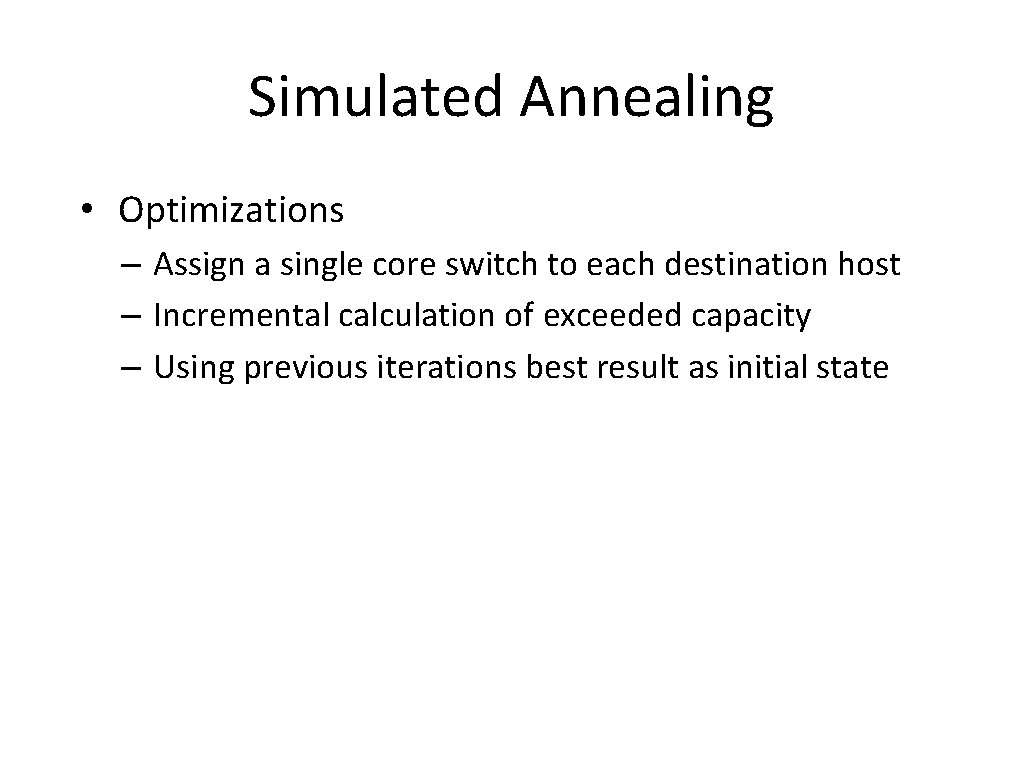
Simulated Annealing • Optimizations – Assign a single core switch to each destination host – Incremental calculation of exceeded capacity – Using previous iterations best result as initial state
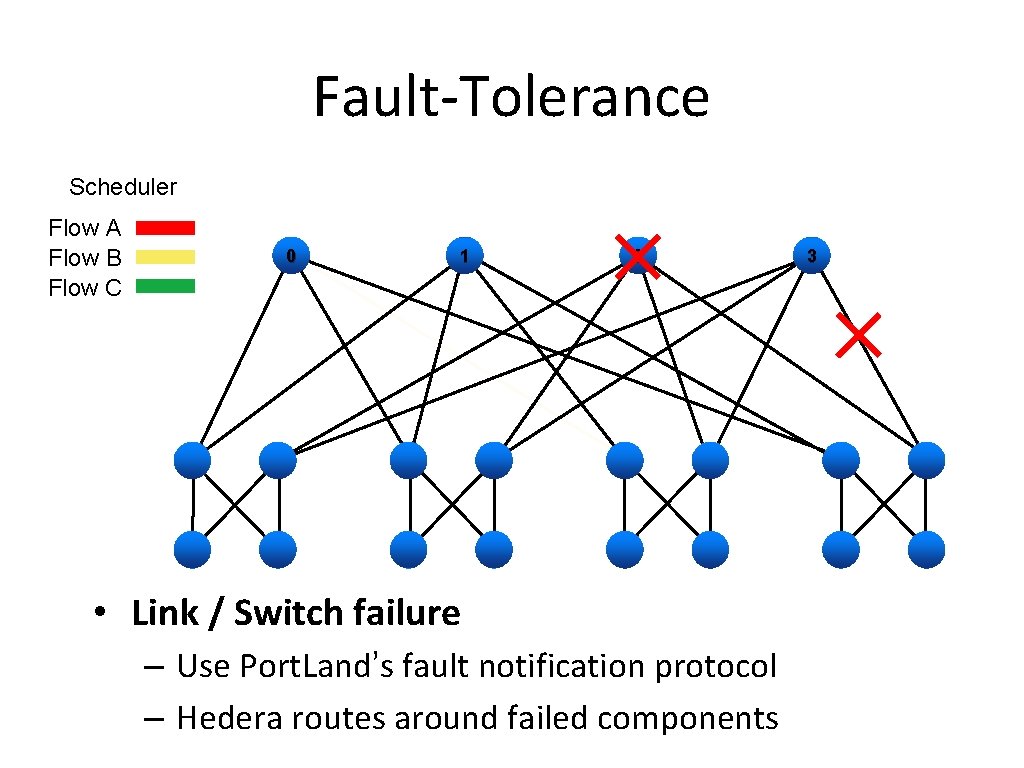
Fault-Tolerance Scheduler Flow A Flow B Flow C 0 1 2 • Link / Switch failure – Use Port. Land’s fault notification protocol – Hedera routes around failed components 3
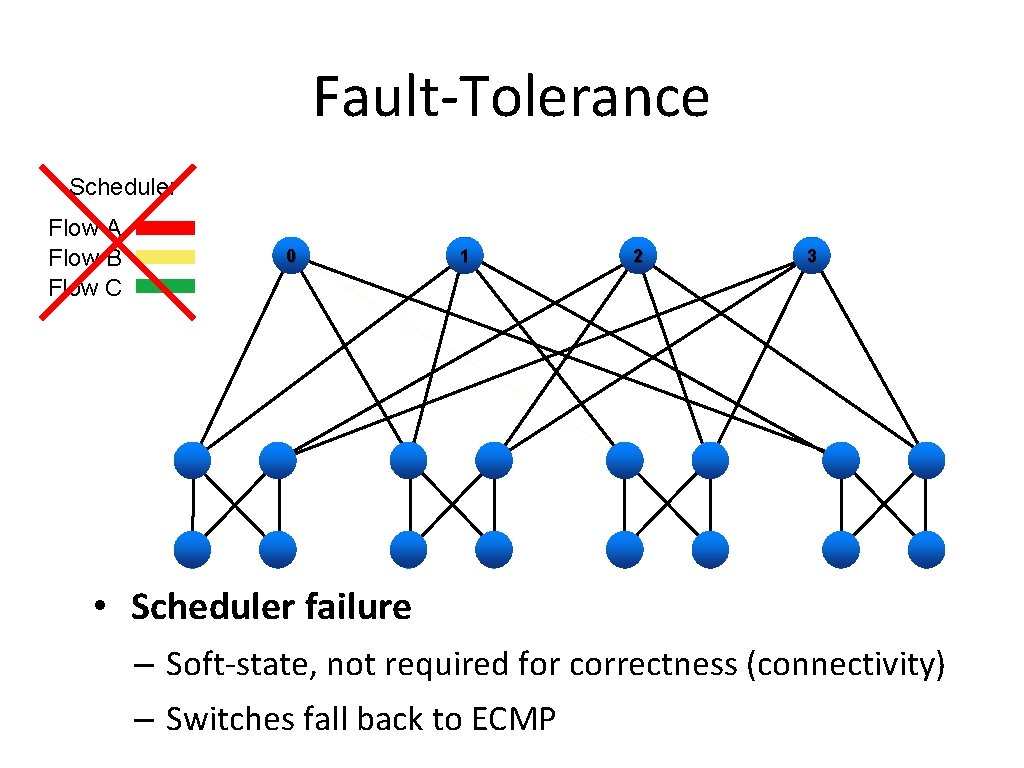
Fault-Tolerance Scheduler Flow A Flow B Flow C 0 1 2 3 • Scheduler failure – Soft-state, not required for correctness (connectivity) – Switches fall back to ECMP
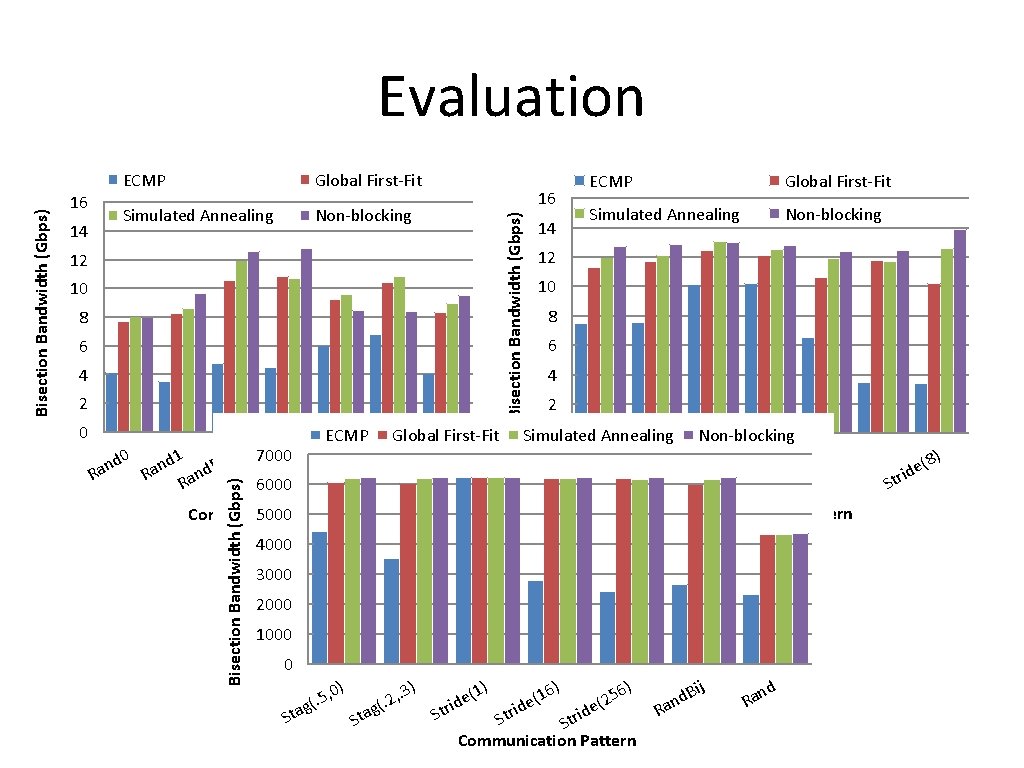
14 Global First-Fit Simulated Annealing Non-blocking 16 Bisection Bandwidth (Gbps) 16 ECMP 12 10 8 6 4 2 0 ECMP Global First-Fit 0 1 0 7000 1 X 2 X 3 spot Bij d d nd nd a a d d n t an n n R R Ra Ho Ra Ra 6000 R Bisection Bandwidth (Gbps) Evaluation Communication 5000 Pattern 14 ECMP Global First-Fit Simulated Annealing Non-blocking 12 10 8 6 4 2 0 Simulated Annealing Non-blocking 2). 3) ( , , e 2 5 (. (. rid 1 1 t g g S Sta Communication Pattern 4000 3000 2000 1000 0 ) S 5, 0. ( tag ) g Sta , . 3 (. 2 S (1) e trid ) 6 e(1 ) 6 (25 id ide r Str t S Communication Pattern Ra Bij d n nd a R ) Str (8 ide
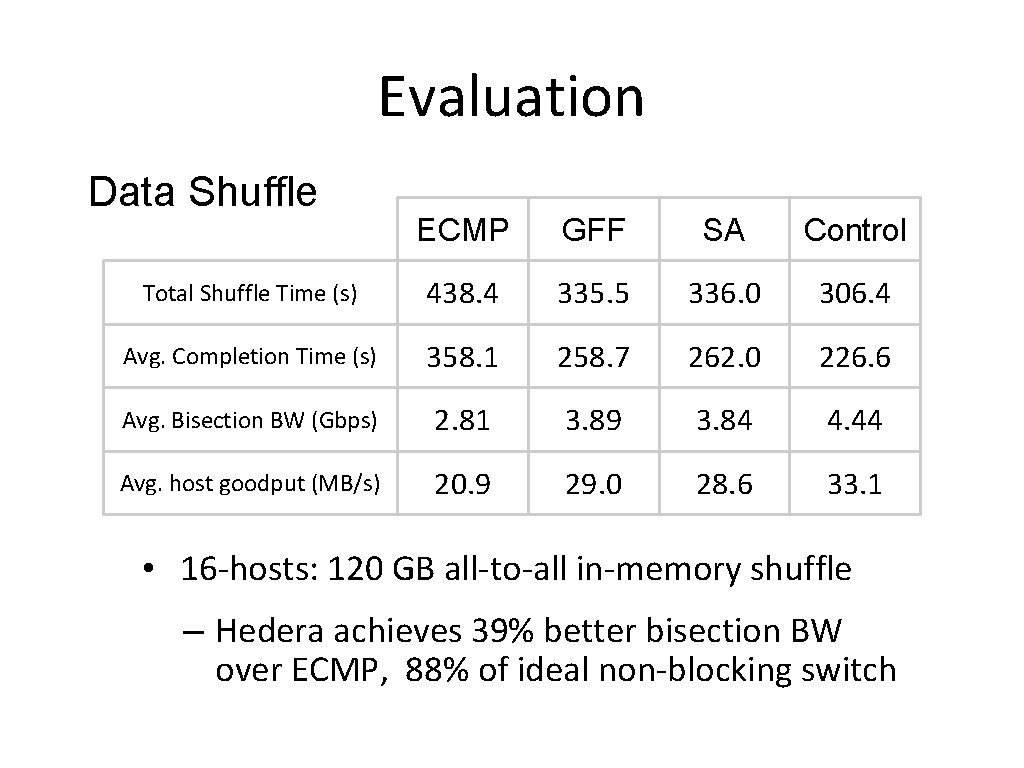
Evaluation Data Shuffle ECMP GFF SA Control Total Shuffle Time (s) 438. 4 335. 5 336. 0 306. 4 Avg. Completion Time (s) 358. 1 258. 7 262. 0 226. 6 Avg. Bisection BW (Gbps) 2. 81 3. 89 3. 84 4. 44 Avg. host goodput (MB/s) 20. 9 29. 0 28. 6 33. 1 • 16 -hosts: 120 GB all-to-all in-memory shuffle – Hedera achieves 39% better bisection BW over ECMP, 88% of ideal non-blocking switch
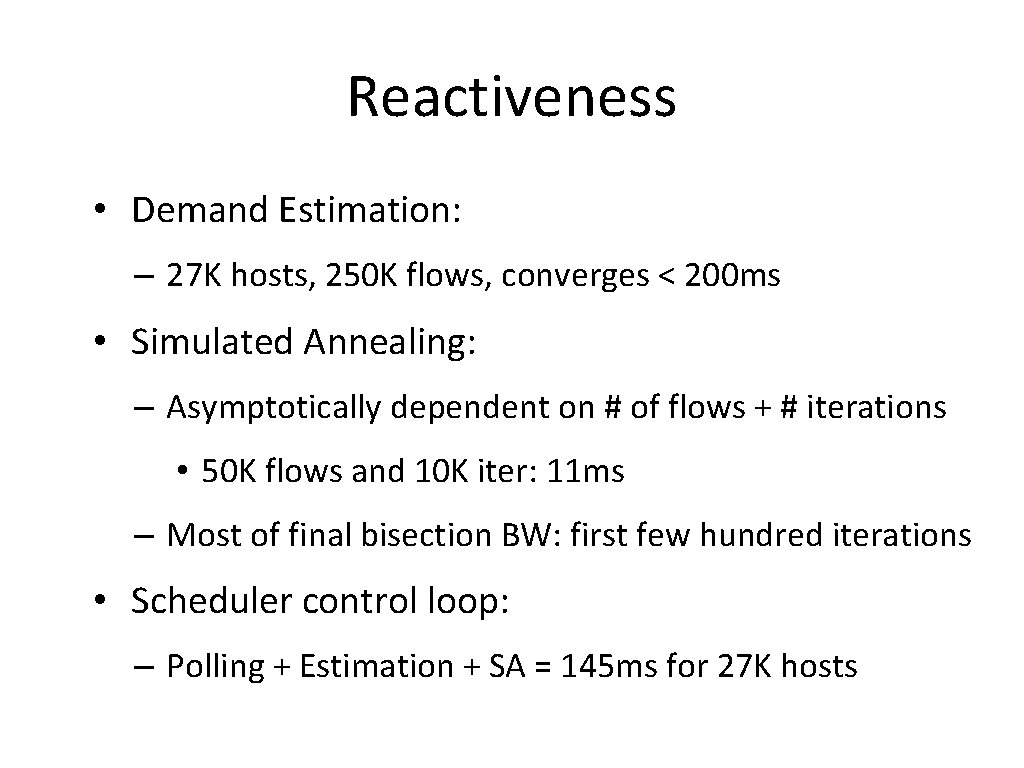
Reactiveness • Demand Estimation: – 27 K hosts, 250 K flows, converges < 200 ms • Simulated Annealing: – Asymptotically dependent on # of flows + # iterations • 50 K flows and 10 K iter: 11 ms – Most of final bisection BW: first few hundred iterations • Scheduler control loop: – Polling + Estimation + SA = 145 ms for 27 K hosts
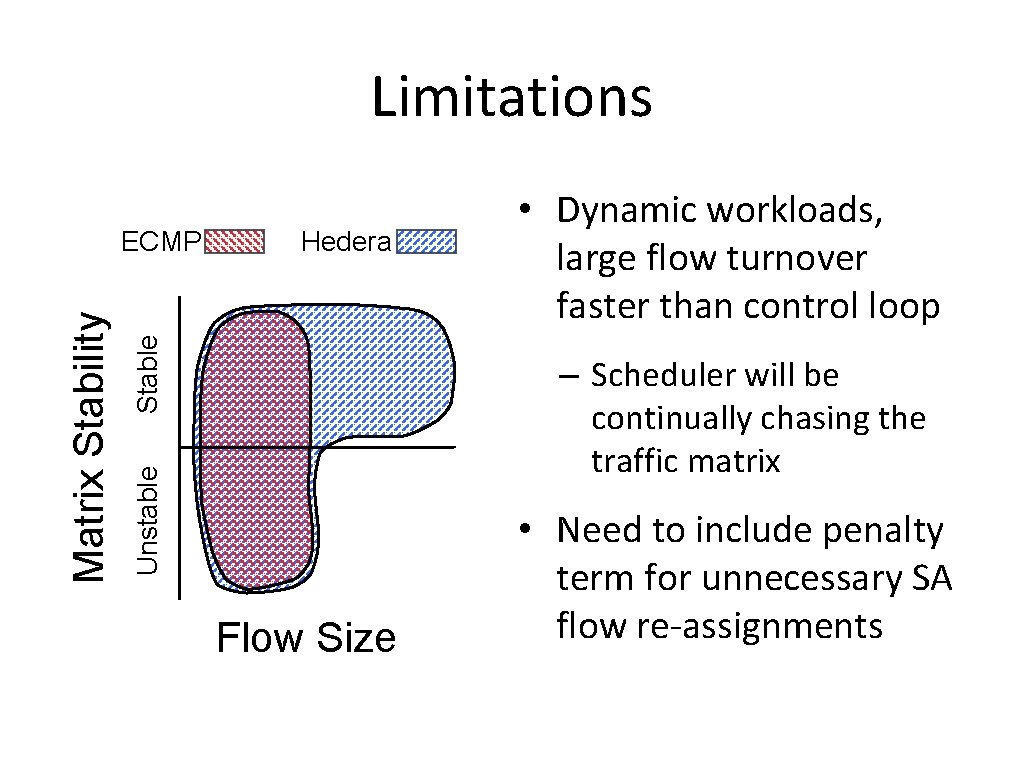
Limitations Stable Hedera – Scheduler will be continually chasing the traffic matrix Unstable Matrix Stability ECMP • Dynamic workloads, large flow turnover faster than control loop Flow Size • Need to include penalty term for unnecessary SA flow re-assignments
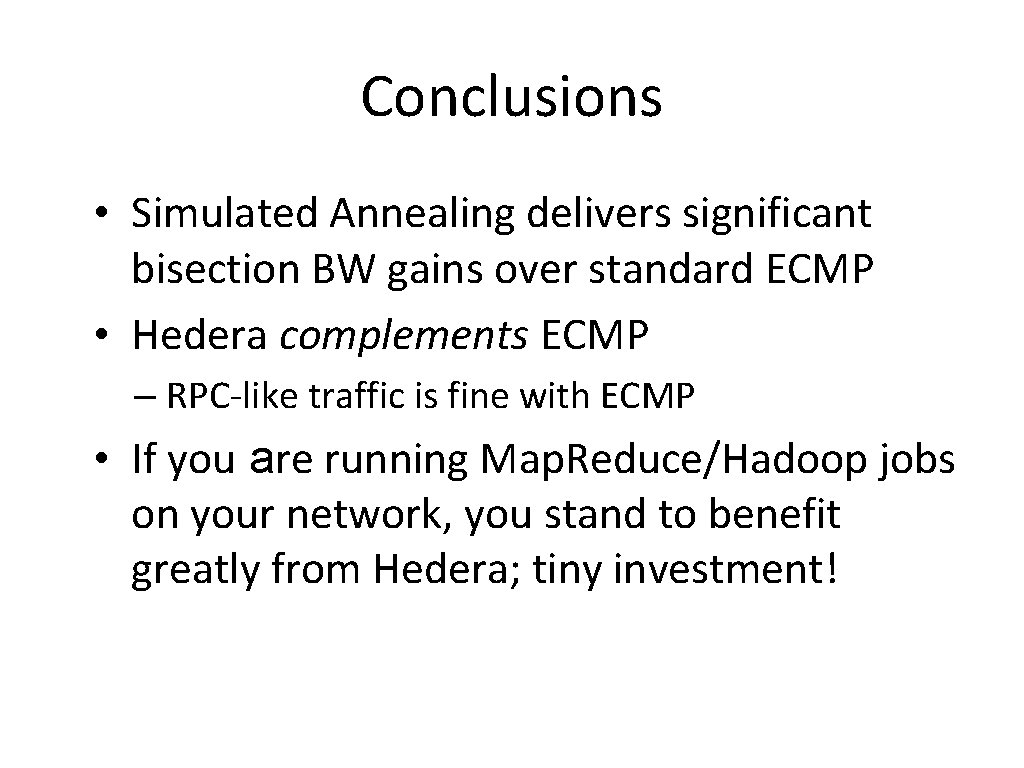
Conclusions • Simulated Annealing delivers significant bisection BW gains over standard ECMP • Hedera complements ECMP – RPC-like traffic is fine with ECMP • If you are running Map. Reduce/Hadoop jobs on your network, you stand to benefit greatly from Hedera; tiny investment!
![Thoughts 1 Inconsistencies Motivation is Map Reduce but Demand Estimation assumes sparse traffic Thoughts [1] Inconsistencies • Motivation is Map. Reduce, but Demand Estimation assumes sparse traffic](https://slidetodoc.com/presentation_image_h/defe7a62270bb8e3b7cec43167763eb8/image-31.jpg)
Thoughts [1] Inconsistencies • Motivation is Map. Reduce, but Demand Estimation assumes sparse traffic matrix – Something fuzzy • Demand Estimation step assumes all bandwidth available at host – Forgets the poor mice : ’(
![Thoughts 2 Inconsistencies Evaluation Results already discussed Simulation using their own flowlevel Thoughts [2] Inconsistencies • Evaluation Results (already discussed) • Simulation using their own flow-level](https://slidetodoc.com/presentation_image_h/defe7a62270bb8e3b7cec43167763eb8/image-32.jpg)
Thoughts [2] Inconsistencies • Evaluation Results (already discussed) • Simulation using their own flow-level simulator – Makes me question things • Some evaluation details fuzzy – No specification of flow sizes (other than shuffle), arrival rate, etc
![Thoughts 3 Periodicity of 5 seconds Sufficient Limit Scalable to Thoughts [3] • Periodicity of 5 seconds – Sufficient? – Limit? – Scalable to](https://slidetodoc.com/presentation_image_h/defe7a62270bb8e3b7cec43167763eb8/image-33.jpg)
Thoughts [3] • Periodicity of 5 seconds – Sufficient? – Limit? – Scalable to higher b/w?