Empirical Learning Methods in Natural Language Processing Ido
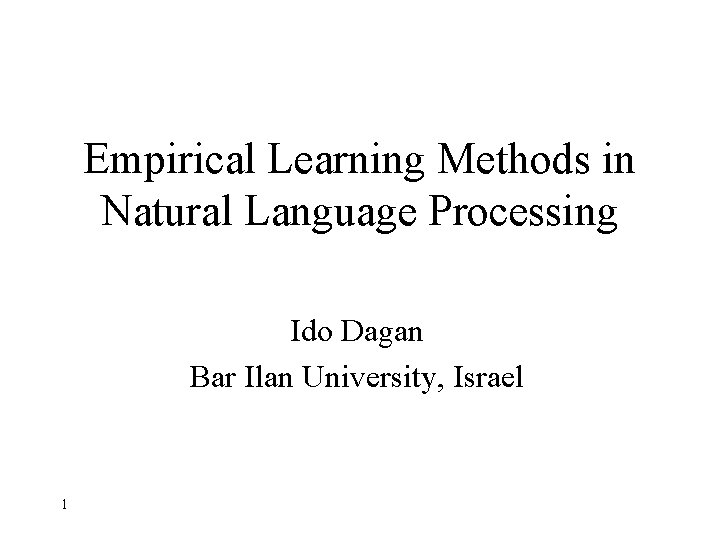
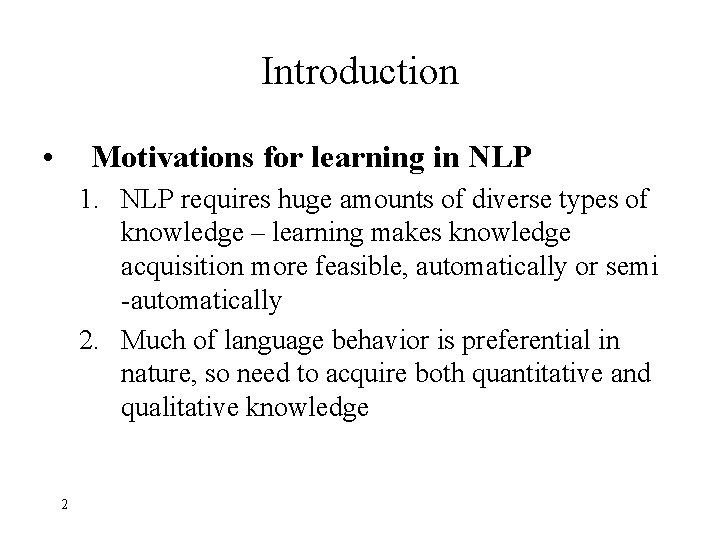
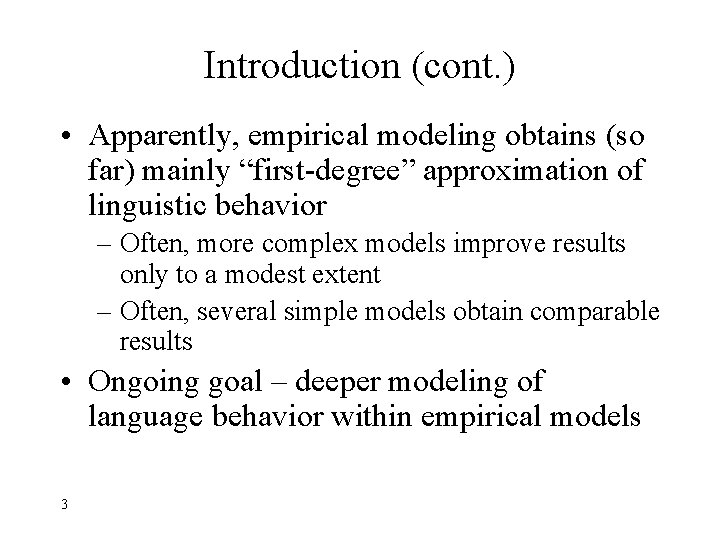
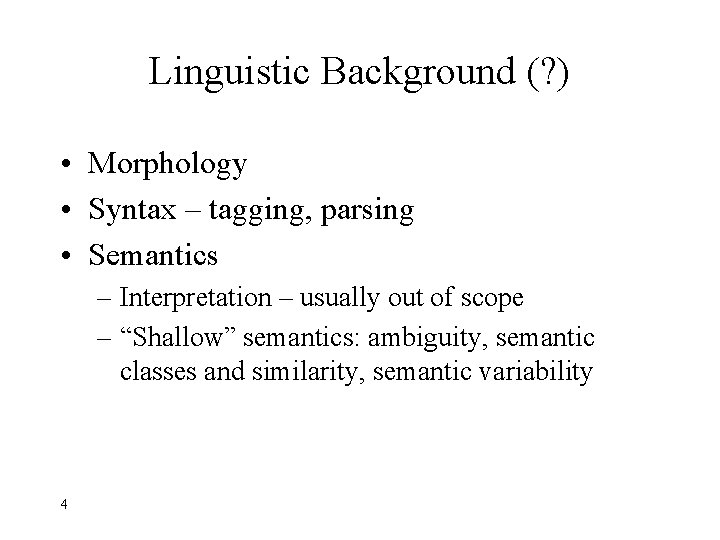
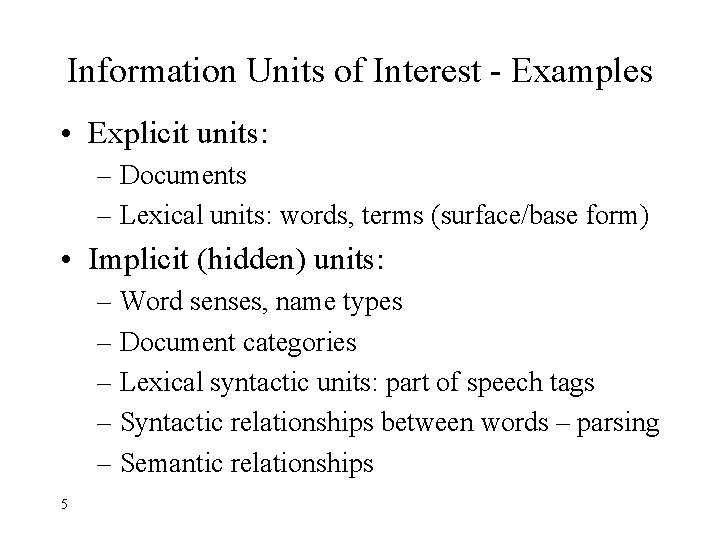
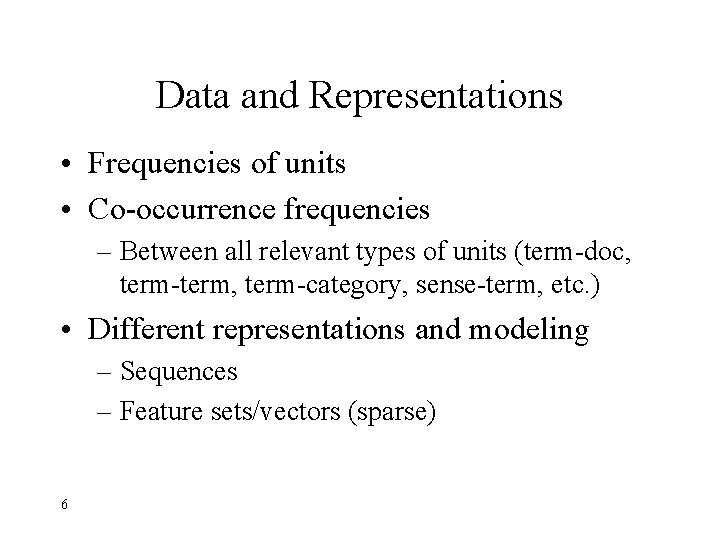
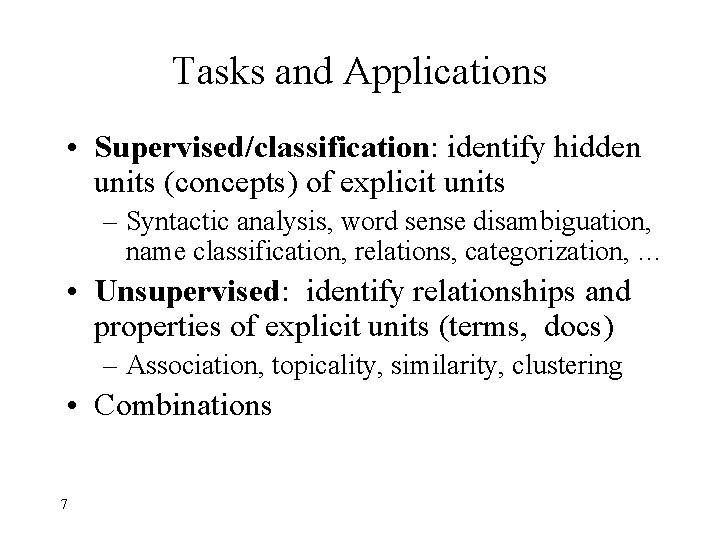
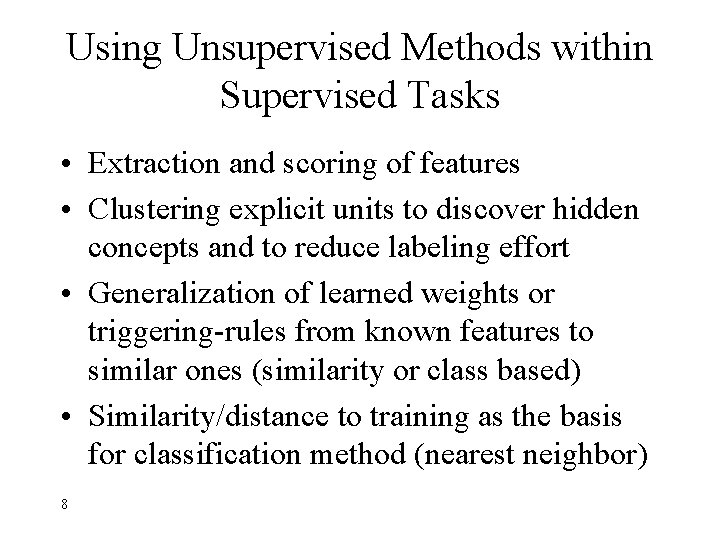
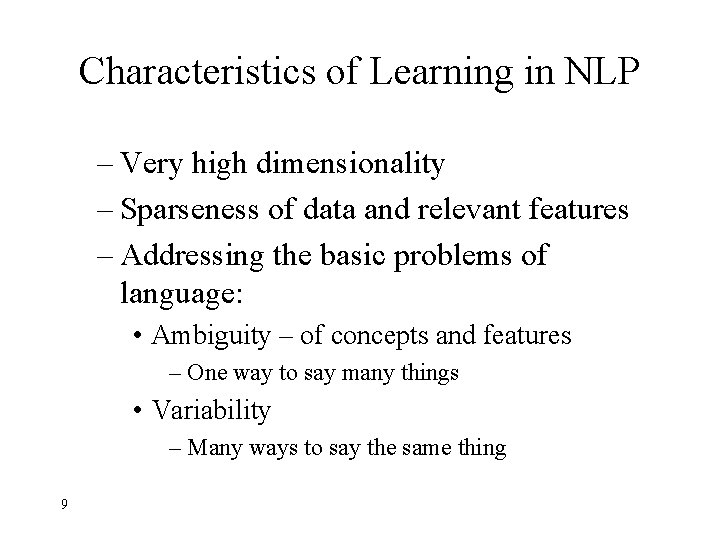
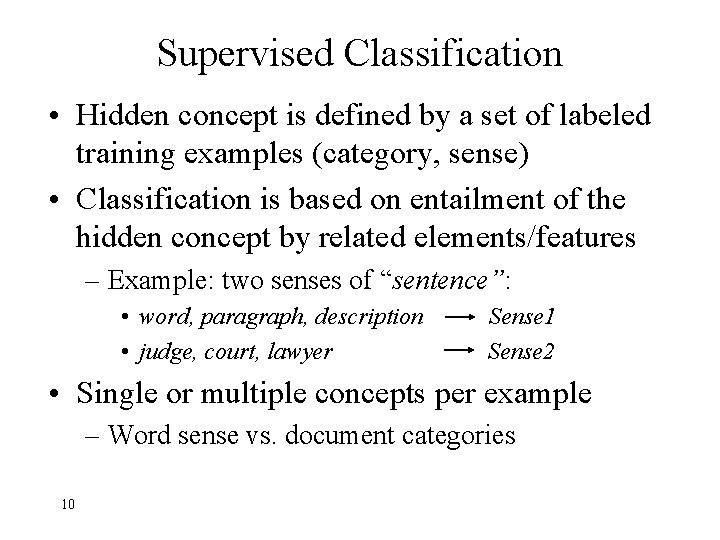
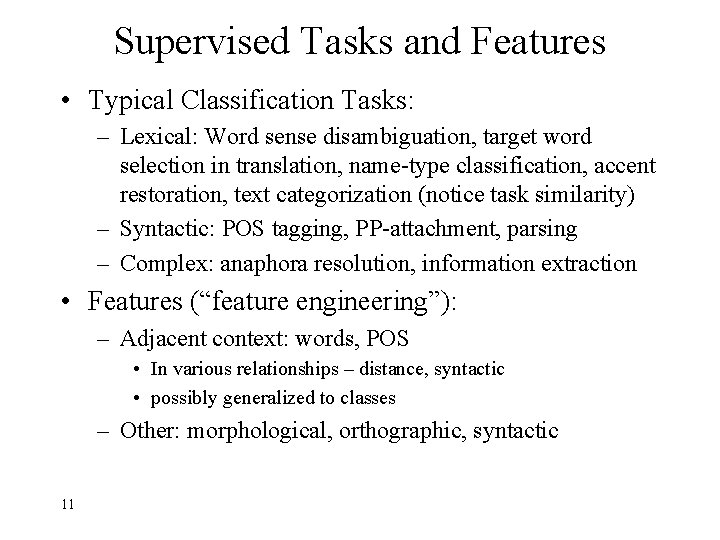
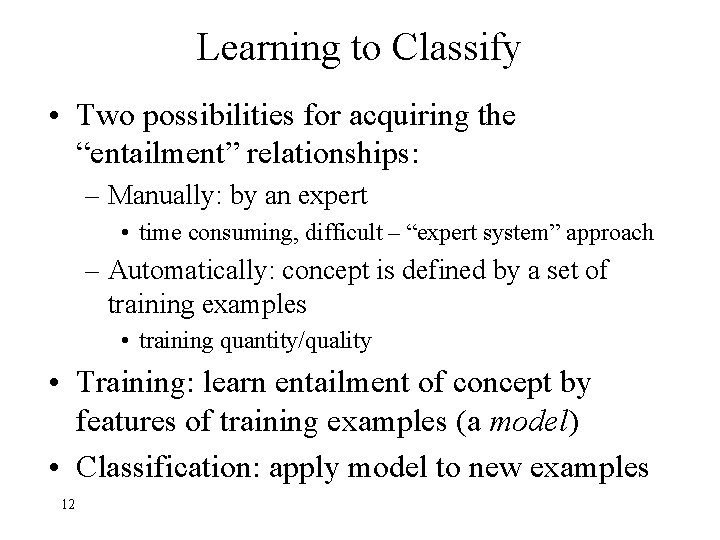
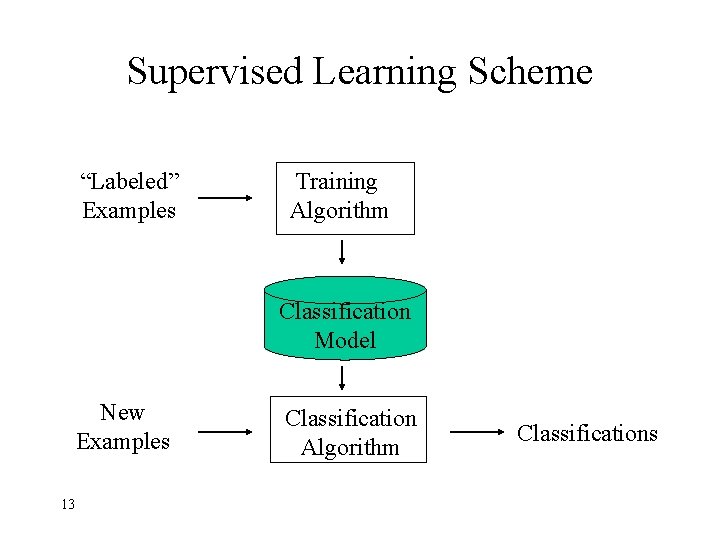
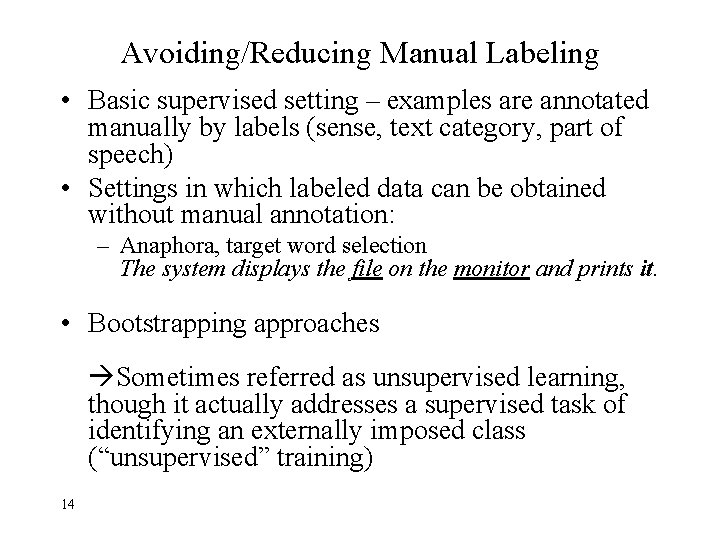
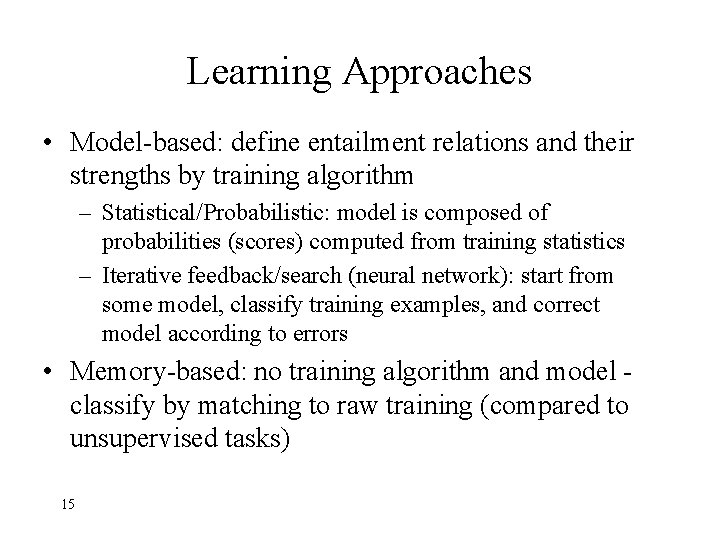
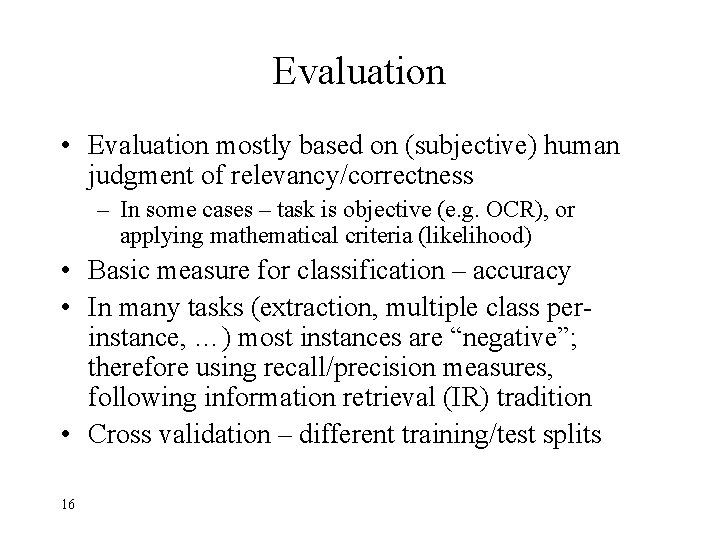
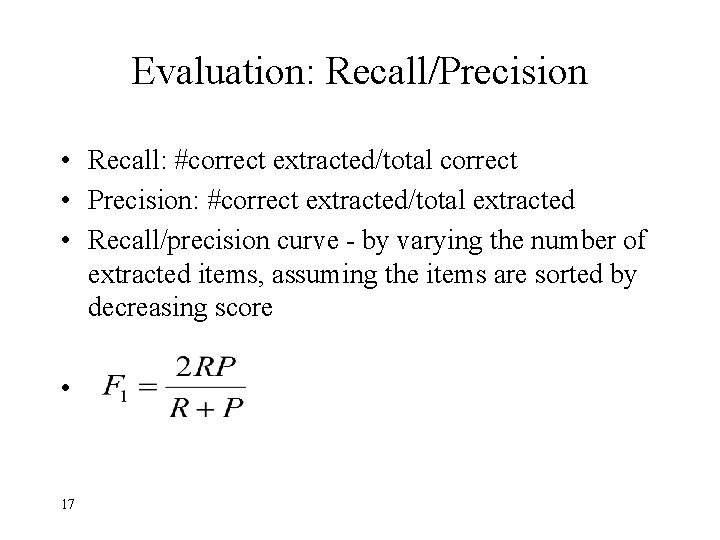
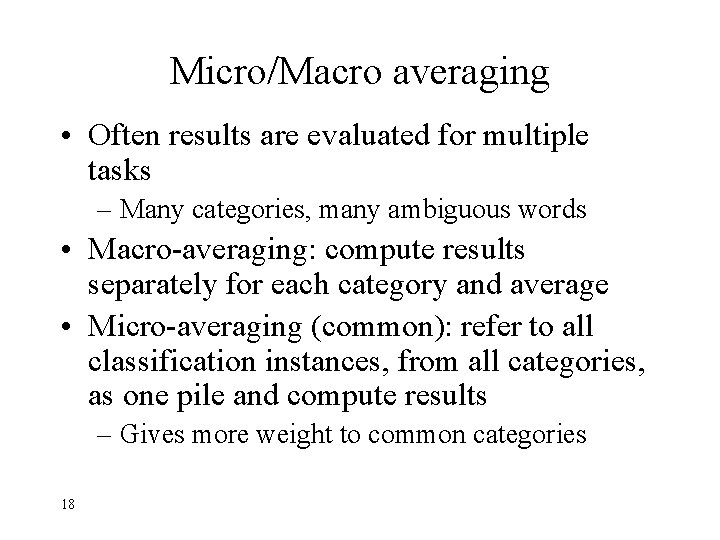
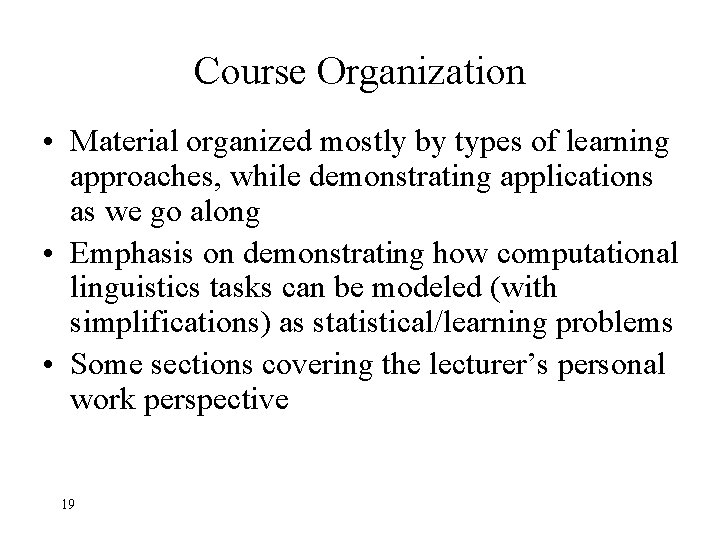
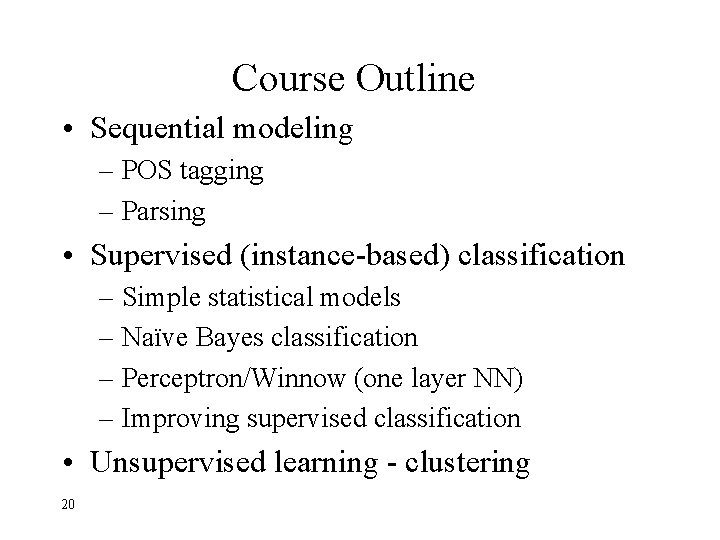
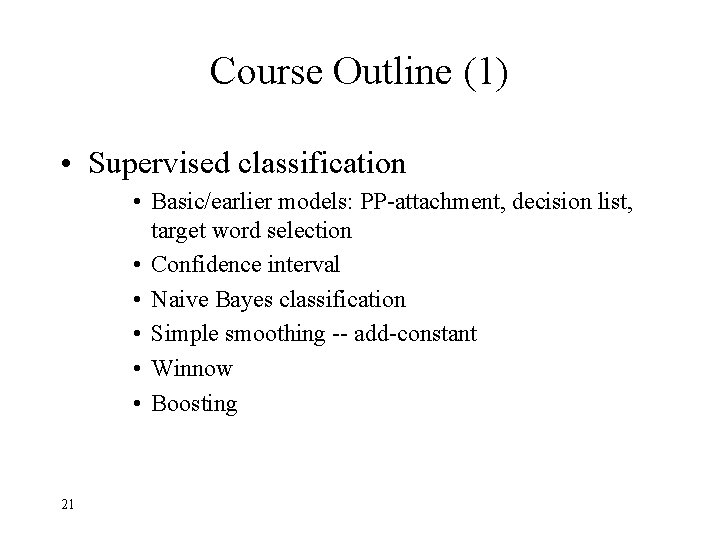
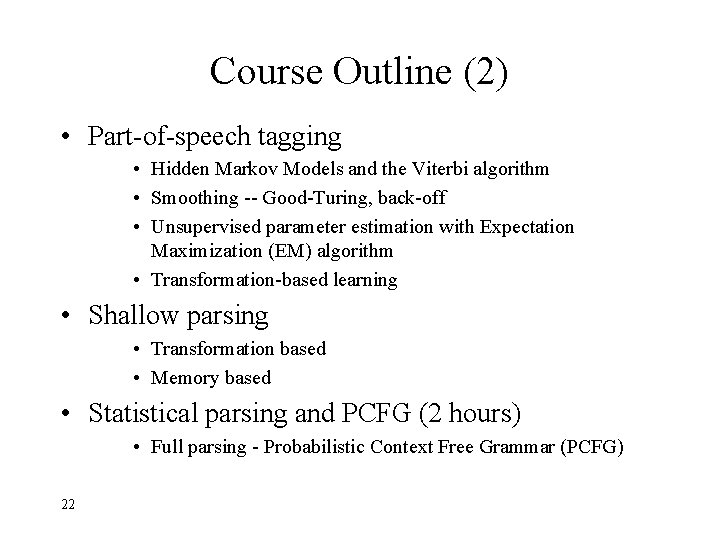
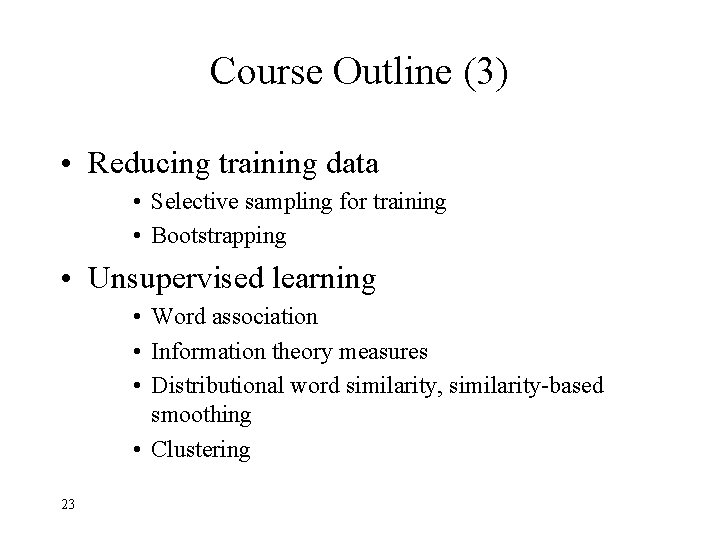
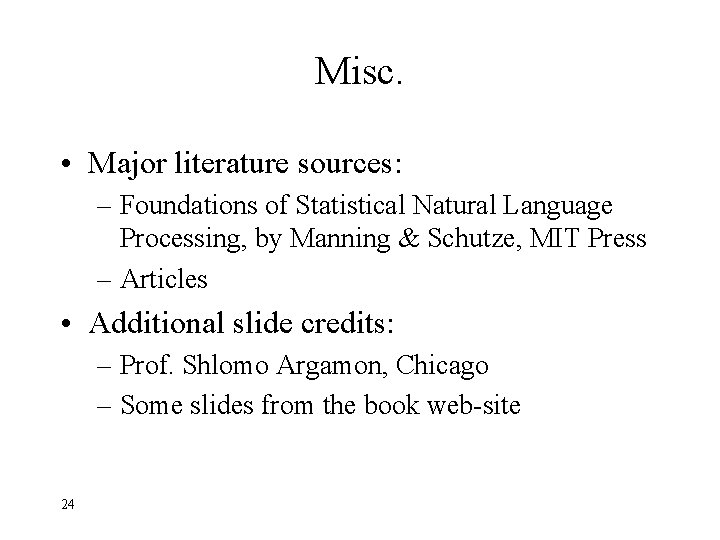
- Slides: 24
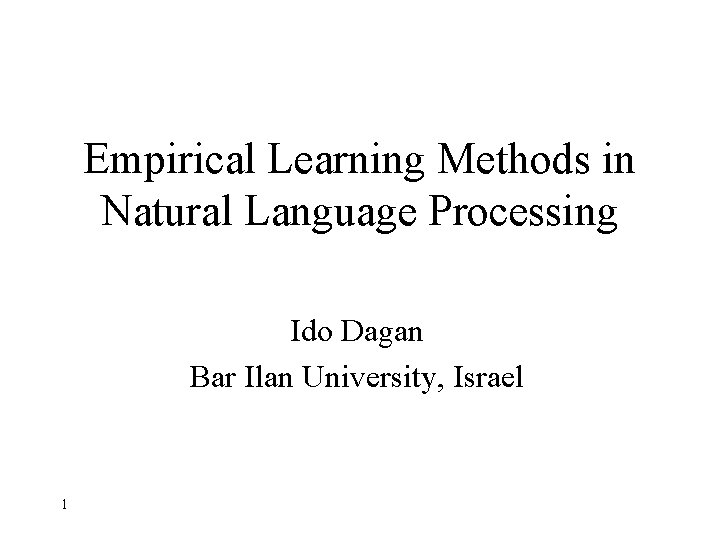
Empirical Learning Methods in Natural Language Processing Ido Dagan Bar Ilan University, Israel 1
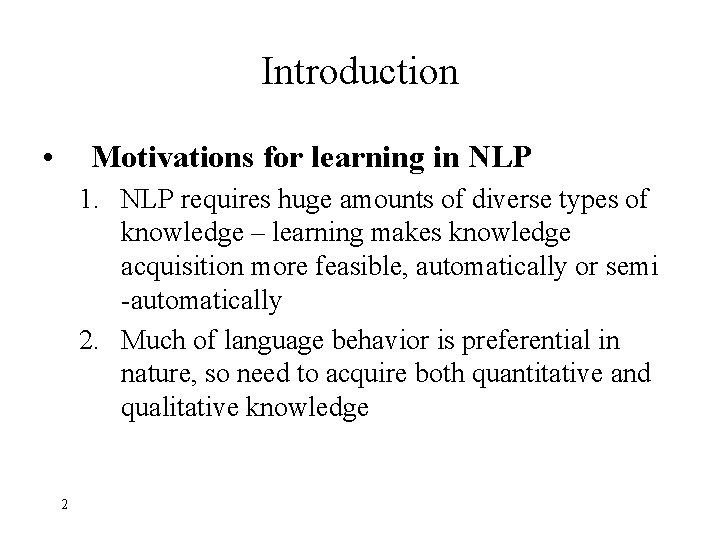
Introduction • Motivations for learning in NLP 1. NLP requires huge amounts of diverse types of knowledge – learning makes knowledge acquisition more feasible, automatically or semi -automatically 2. Much of language behavior is preferential in nature, so need to acquire both quantitative and qualitative knowledge 2
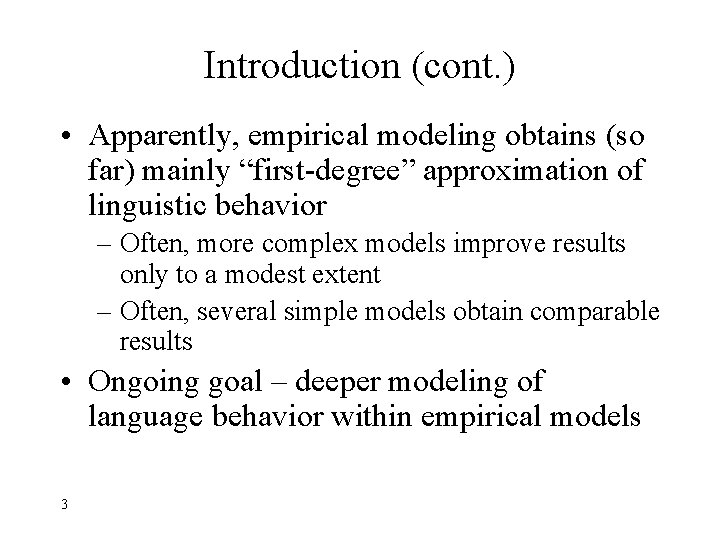
Introduction (cont. ) • Apparently, empirical modeling obtains (so far) mainly “first-degree” approximation of linguistic behavior – Often, more complex models improve results only to a modest extent – Often, several simple models obtain comparable results • Ongoing goal – deeper modeling of language behavior within empirical models 3
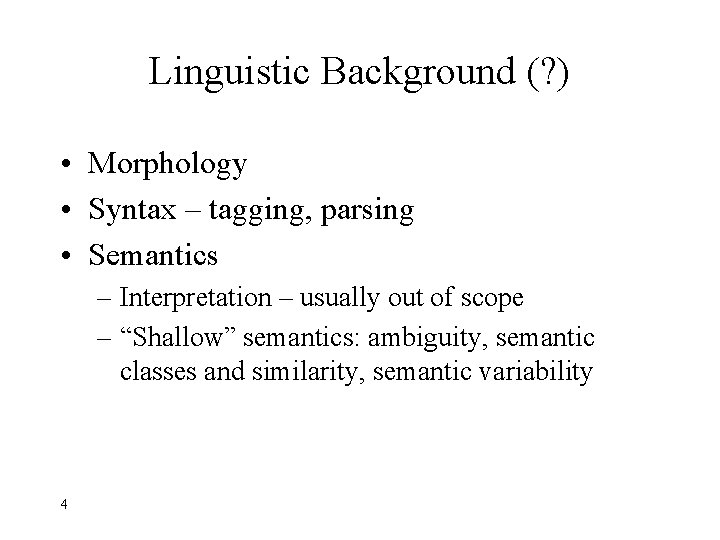
Linguistic Background (? ) • Morphology • Syntax – tagging, parsing • Semantics – Interpretation – usually out of scope – “Shallow” semantics: ambiguity, semantic classes and similarity, semantic variability 4
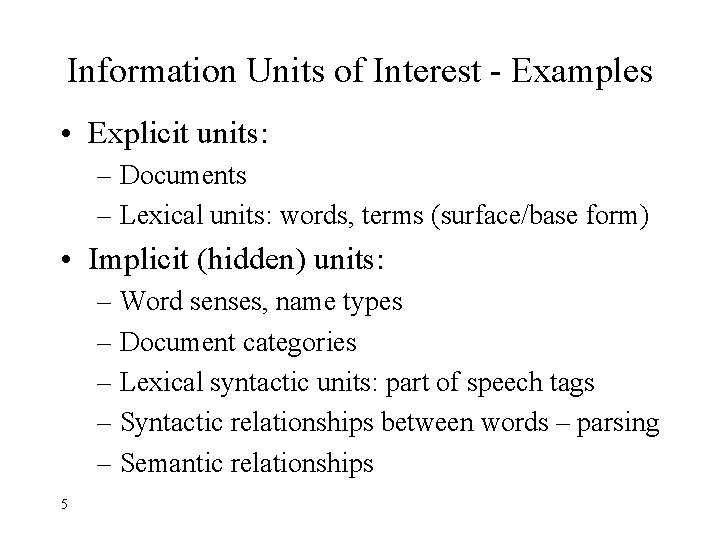
Information Units of Interest - Examples • Explicit units: – Documents – Lexical units: words, terms (surface/base form) • Implicit (hidden) units: – Word senses, name types – Document categories – Lexical syntactic units: part of speech tags – Syntactic relationships between words – parsing – Semantic relationships 5
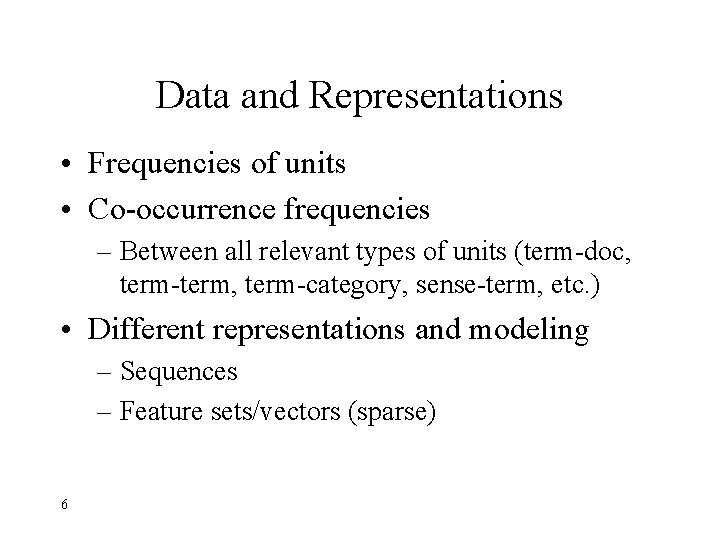
Data and Representations • Frequencies of units • Co-occurrence frequencies – Between all relevant types of units (term-doc, term-term, term-category, sense-term, etc. ) • Different representations and modeling – Sequences – Feature sets/vectors (sparse) 6
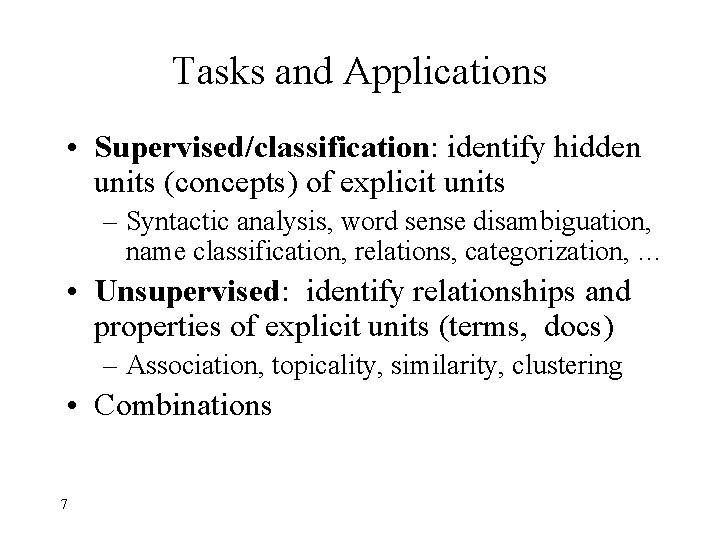
Tasks and Applications • Supervised/classification: identify hidden units (concepts) of explicit units – Syntactic analysis, word sense disambiguation, name classification, relations, categorization, … • Unsupervised: identify relationships and properties of explicit units (terms, docs) – Association, topicality, similarity, clustering • Combinations 7
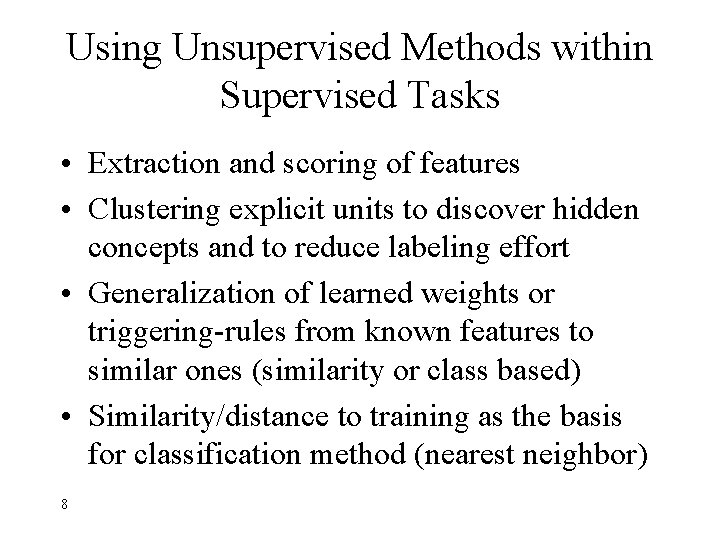
Using Unsupervised Methods within Supervised Tasks • Extraction and scoring of features • Clustering explicit units to discover hidden concepts and to reduce labeling effort • Generalization of learned weights or triggering-rules from known features to similar ones (similarity or class based) • Similarity/distance to training as the basis for classification method (nearest neighbor) 8
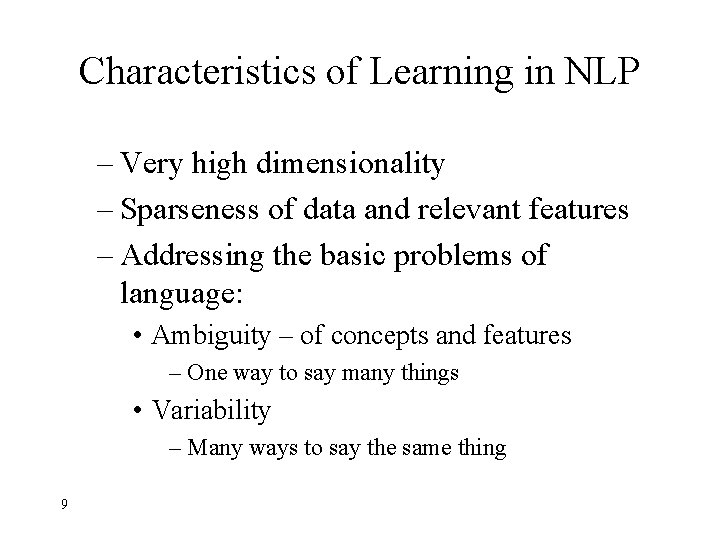
Characteristics of Learning in NLP – Very high dimensionality – Sparseness of data and relevant features – Addressing the basic problems of language: • Ambiguity – of concepts and features – One way to say many things • Variability – Many ways to say the same thing 9
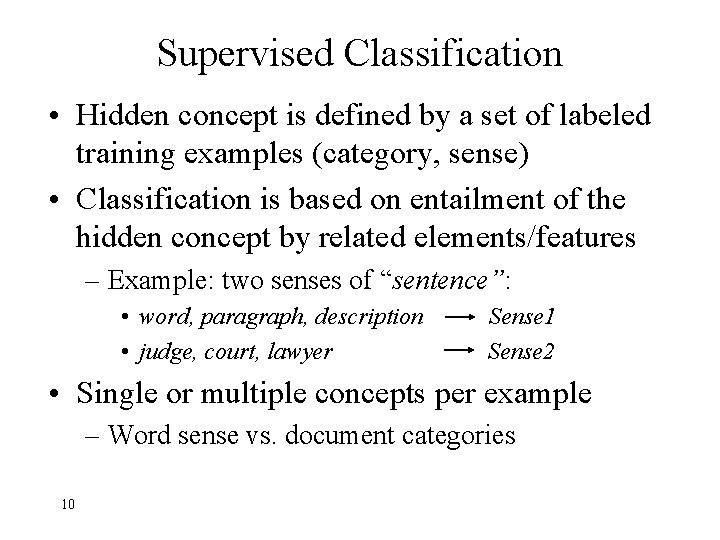
Supervised Classification • Hidden concept is defined by a set of labeled training examples (category, sense) • Classification is based on entailment of the hidden concept by related elements/features – Example: two senses of “sentence”: • word, paragraph, description • judge, court, lawyer Sense 1 Sense 2 • Single or multiple concepts per example – Word sense vs. document categories 10
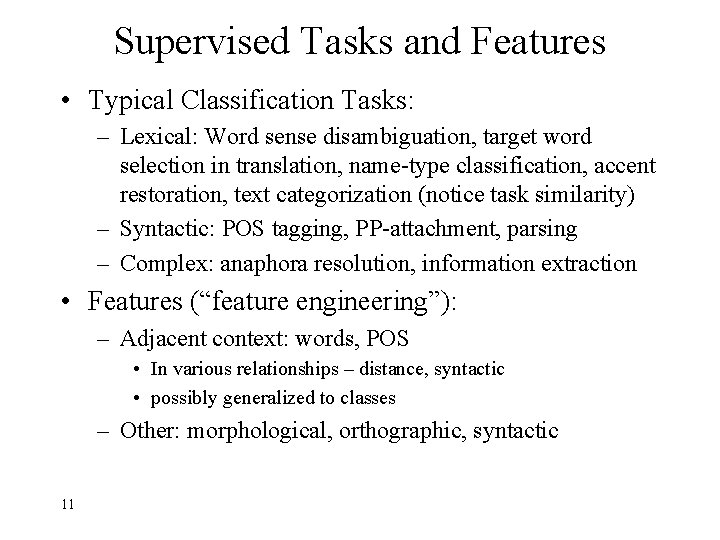
Supervised Tasks and Features • Typical Classification Tasks: – Lexical: Word sense disambiguation, target word selection in translation, name-type classification, accent restoration, text categorization (notice task similarity) – Syntactic: POS tagging, PP-attachment, parsing – Complex: anaphora resolution, information extraction • Features (“feature engineering”): – Adjacent context: words, POS • In various relationships – distance, syntactic • possibly generalized to classes – Other: morphological, orthographic, syntactic 11
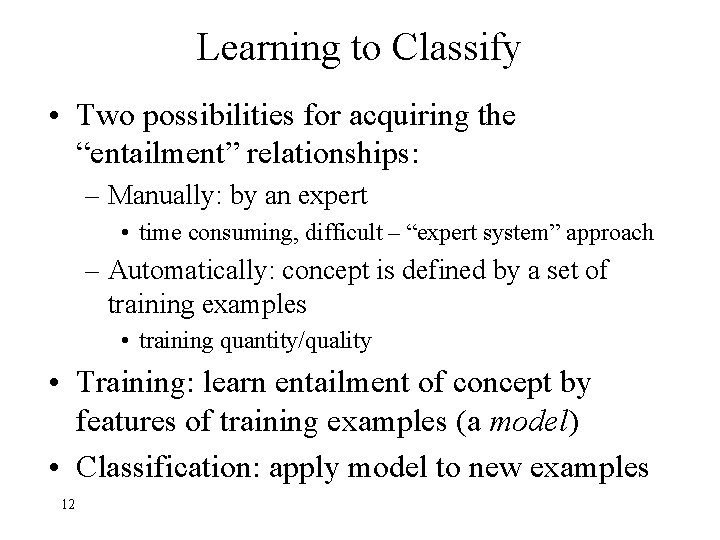
Learning to Classify • Two possibilities for acquiring the “entailment” relationships: – Manually: by an expert • time consuming, difficult – “expert system” approach – Automatically: concept is defined by a set of training examples • training quantity/quality • Training: learn entailment of concept by features of training examples (a model) • Classification: apply model to new examples 12
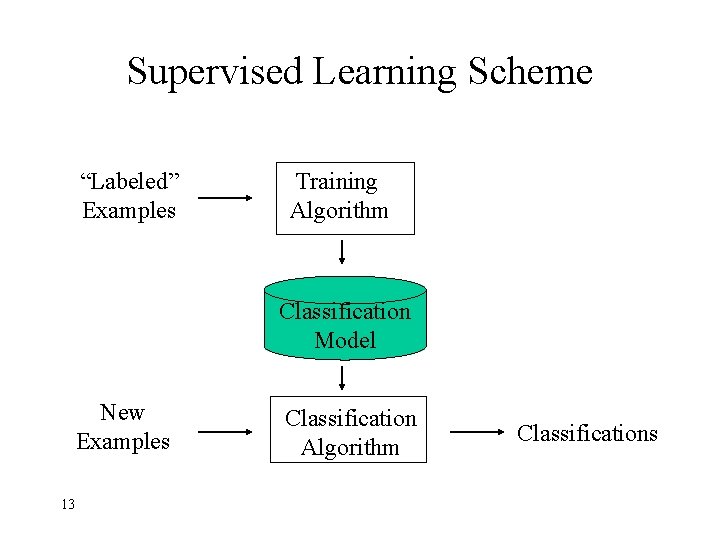
Supervised Learning Scheme “Labeled” Examples Training Algorithm Classification Model New Examples 13 Classification Algorithm Classifications
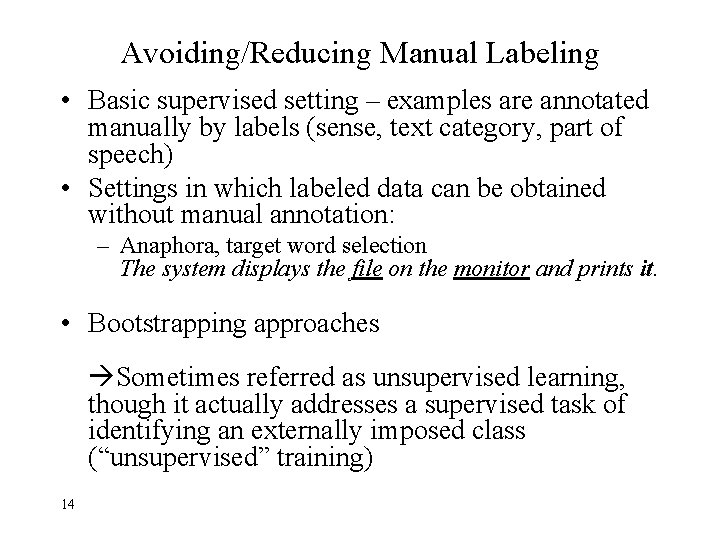
Avoiding/Reducing Manual Labeling • Basic supervised setting – examples are annotated manually by labels (sense, text category, part of speech) • Settings in which labeled data can be obtained without manual annotation: – Anaphora, target word selection The system displays the file on the monitor and prints it. • Bootstrapping approaches Sometimes referred as unsupervised learning, though it actually addresses a supervised task of identifying an externally imposed class (“unsupervised” training) 14
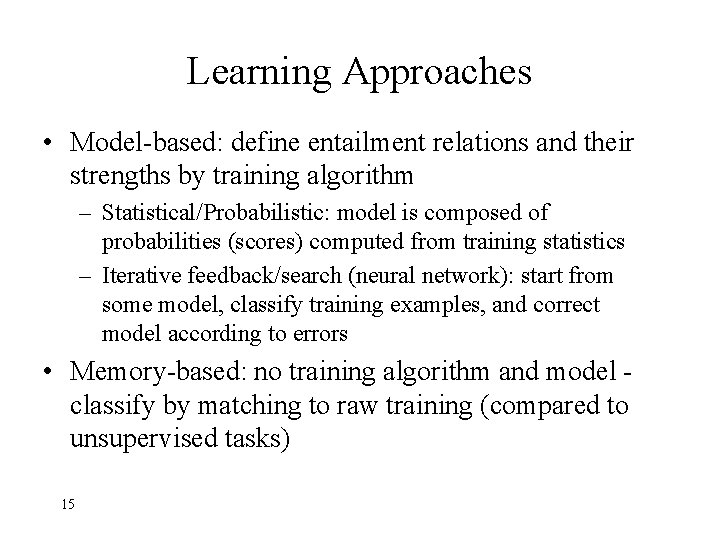
Learning Approaches • Model-based: define entailment relations and their strengths by training algorithm – Statistical/Probabilistic: model is composed of probabilities (scores) computed from training statistics – Iterative feedback/search (neural network): start from some model, classify training examples, and correct model according to errors • Memory-based: no training algorithm and model - classify by matching to raw training (compared to unsupervised tasks) 15
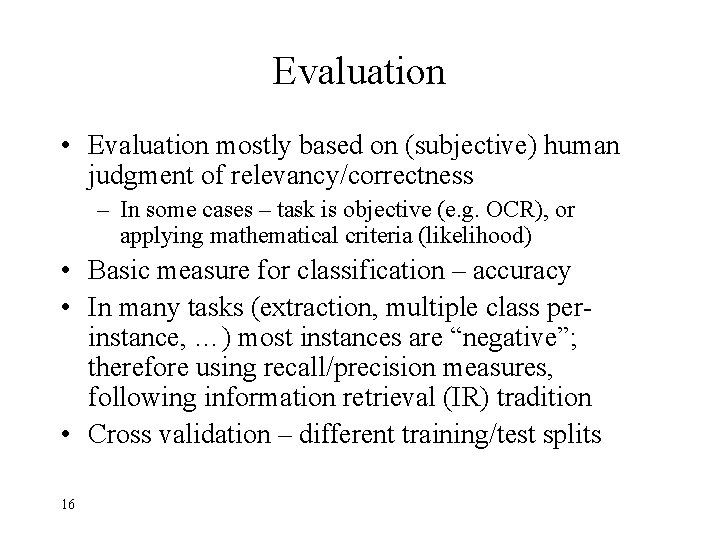
Evaluation • Evaluation mostly based on (subjective) human judgment of relevancy/correctness – In some cases – task is objective (e. g. OCR), or applying mathematical criteria (likelihood) • Basic measure for classification – accuracy • In many tasks (extraction, multiple class perinstance, …) most instances are “negative”; therefore using recall/precision measures, following information retrieval (IR) tradition • Cross validation – different training/test splits 16
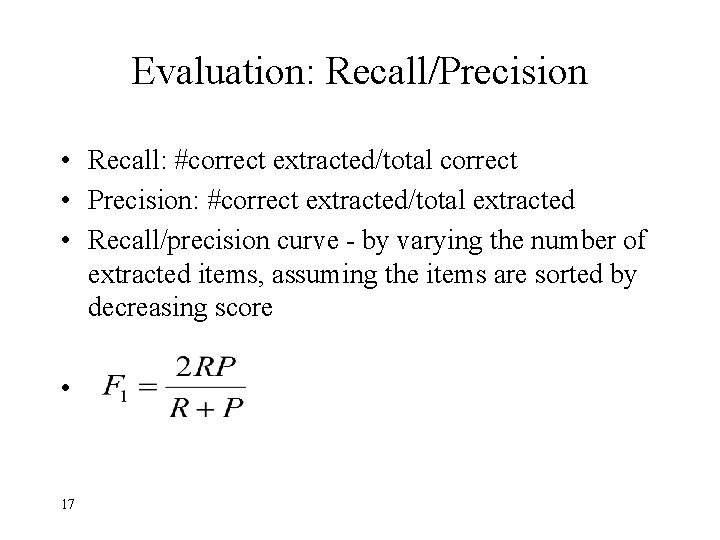
Evaluation: Recall/Precision • Recall: #correct extracted/total correct • Precision: #correct extracted/total extracted • Recall/precision curve - by varying the number of extracted items, assuming the items are sorted by decreasing score • 17
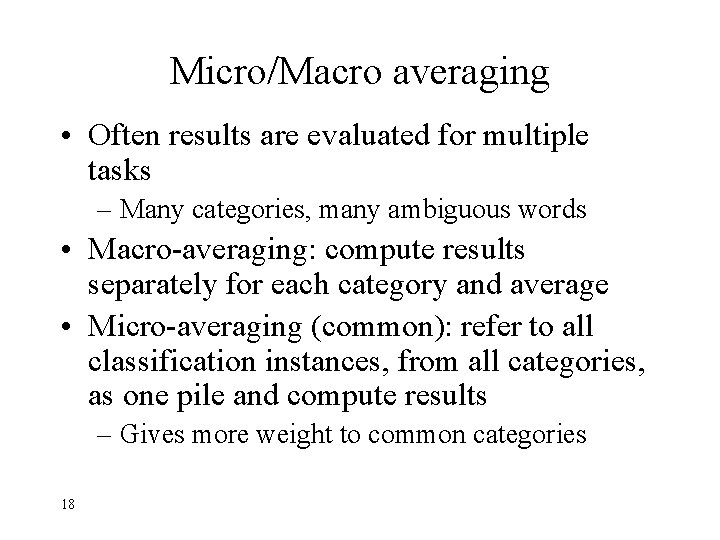
Micro/Macro averaging • Often results are evaluated for multiple tasks – Many categories, many ambiguous words • Macro-averaging: compute results separately for each category and average • Micro-averaging (common): refer to all classification instances, from all categories, as one pile and compute results – Gives more weight to common categories 18
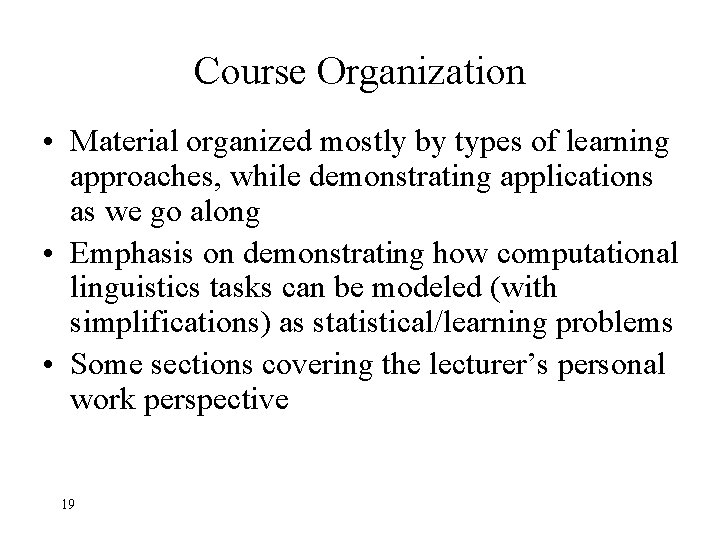
Course Organization • Material organized mostly by types of learning approaches, while demonstrating applications as we go along • Emphasis on demonstrating how computational linguistics tasks can be modeled (with simplifications) as statistical/learning problems • Some sections covering the lecturer’s personal work perspective 19
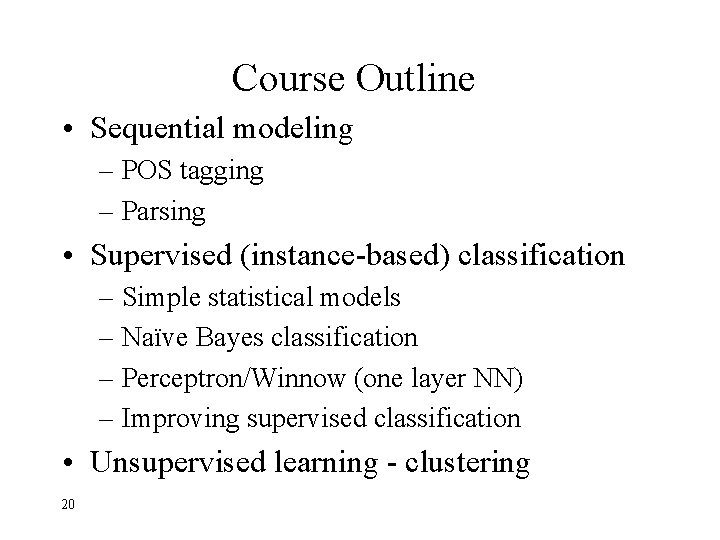
Course Outline • Sequential modeling – POS tagging – Parsing • Supervised (instance-based) classification – Simple statistical models – Naïve Bayes classification – Perceptron/Winnow (one layer NN) – Improving supervised classification • Unsupervised learning - clustering 20
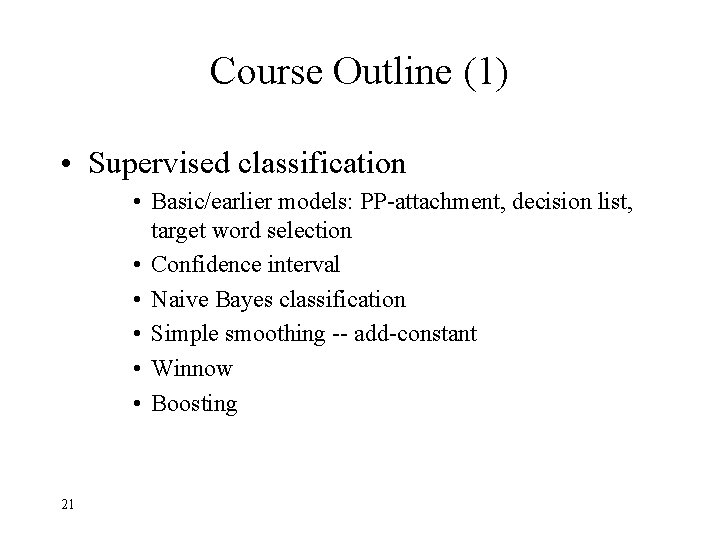
Course Outline (1) • Supervised classification • Basic/earlier models: PP-attachment, decision list, target word selection • Confidence interval • Naive Bayes classification • Simple smoothing -- add-constant • Winnow • Boosting 21
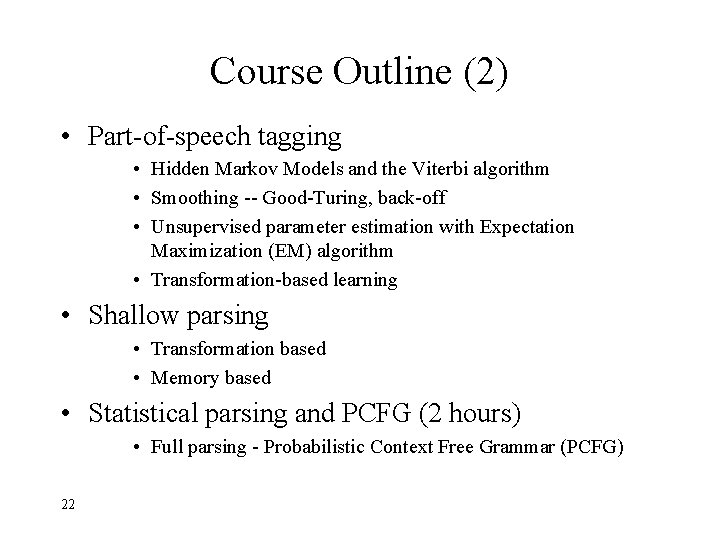
Course Outline (2) • Part-of-speech tagging • Hidden Markov Models and the Viterbi algorithm • Smoothing -- Good-Turing, back-off • Unsupervised parameter estimation with Expectation Maximization (EM) algorithm • Transformation-based learning • Shallow parsing • Transformation based • Memory based • Statistical parsing and PCFG (2 hours) • Full parsing - Probabilistic Context Free Grammar (PCFG) 22
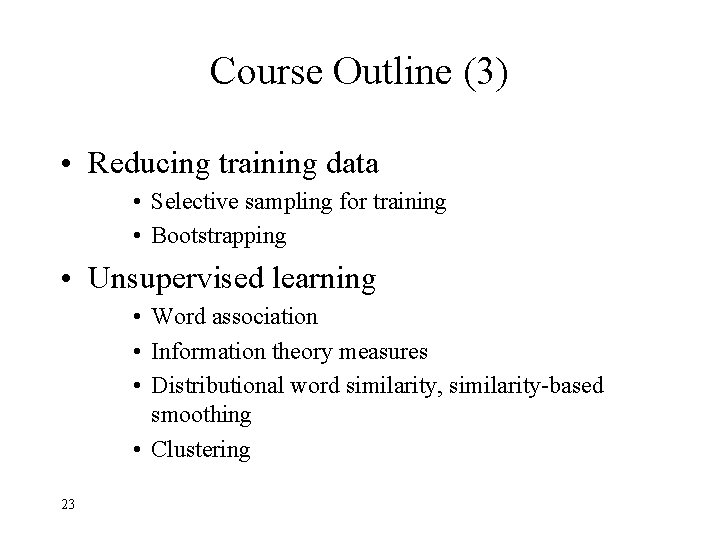
Course Outline (3) • Reducing training data • Selective sampling for training • Bootstrapping • Unsupervised learning • Word association • Information theory measures • Distributional word similarity, similarity-based smoothing • Clustering 23
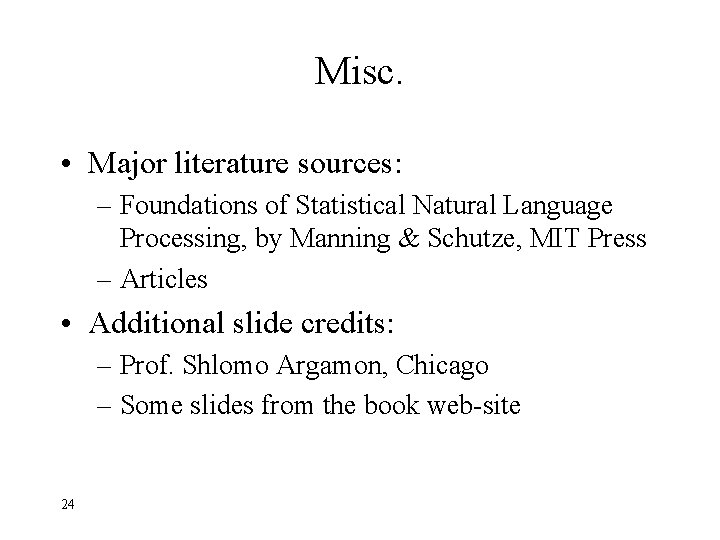
Misc. • Major literature sources: – Foundations of Statistical Natural Language Processing, by Manning & Schutze, MIT Press – Articles • Additional slide credits: – Prof. Shlomo Argamon, Chicago – Some slides from the book web-site 24