Eidgenssisches Departement des Innern EDI Bundesamt fr Meteorologie
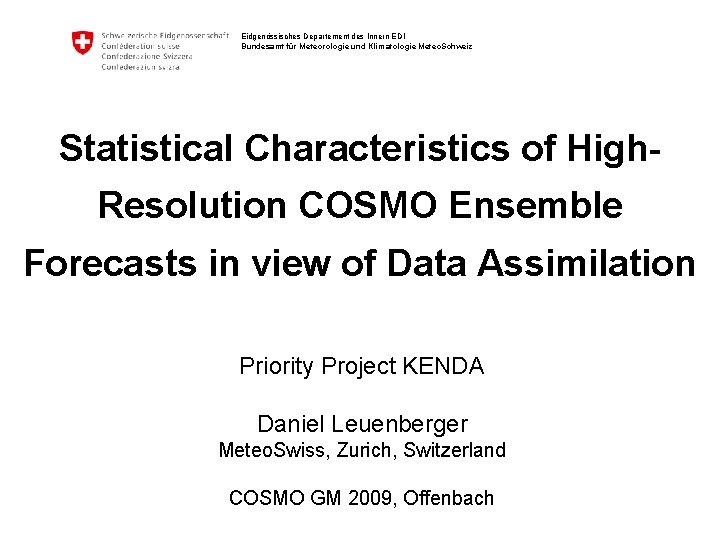
Eidgenössisches Departement des Innern EDI Bundesamt für Meteorologie und Klimatologie Meteo. Schweiz Statistical Characteristics of High. Resolution COSMO Ensemble Forecasts in view of Data Assimilation Priority Project KENDA Daniel Leuenberger Meteo. Swiss, Zurich, Switzerland COSMO GM 2009, Offenbach
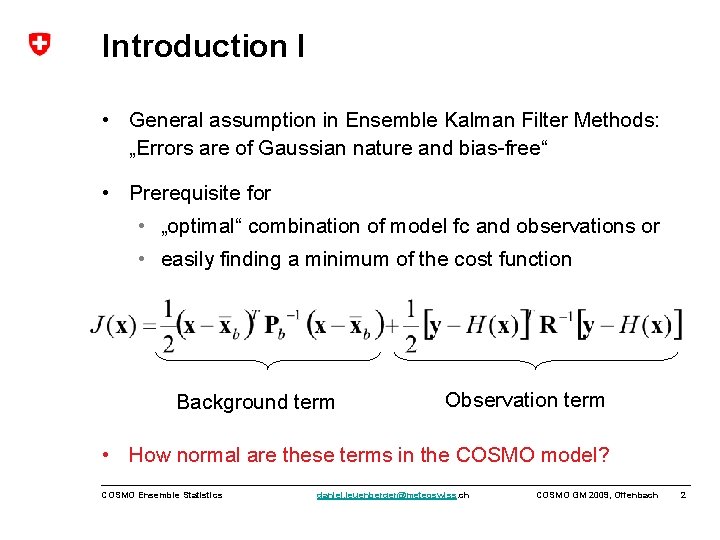
Introduction I • General assumption in Ensemble Kalman Filter Methods: „Errors are of Gaussian nature and bias-free“ • Prerequisite for • „optimal“ combination of model fc and observations or • easily finding a minimum of the cost function Background term Observation term • How normal are these terms in the COSMO model? COSMO Ensemble Statistics daniel. leuenberger@meteoswiss. ch COSMO GM 2009, Offenbach 2
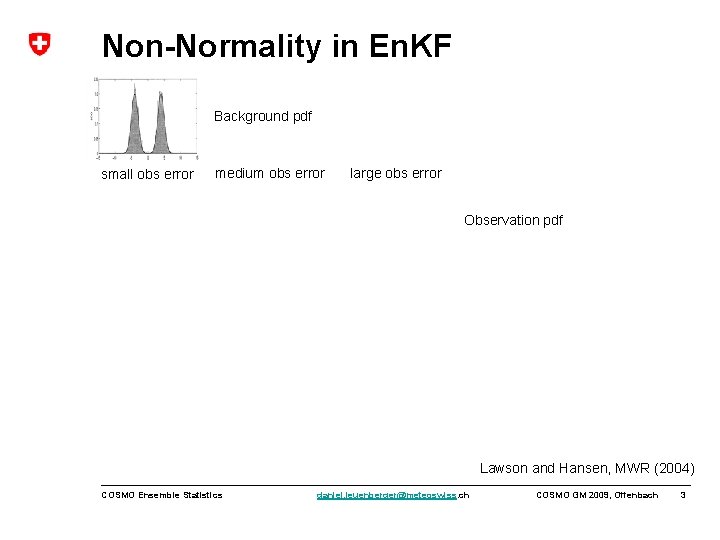
Non-Normality in En. KF Background pdf small obs error medium obs error large obs error Observation pdf Pdf after update with stochastic En. KF Pdf after update with deterministic En. KF Lawson and Hansen, MWR (2004) COSMO Ensemble Statistics daniel. leuenberger@meteoswiss. ch COSMO GM 2009, Offenbach 3
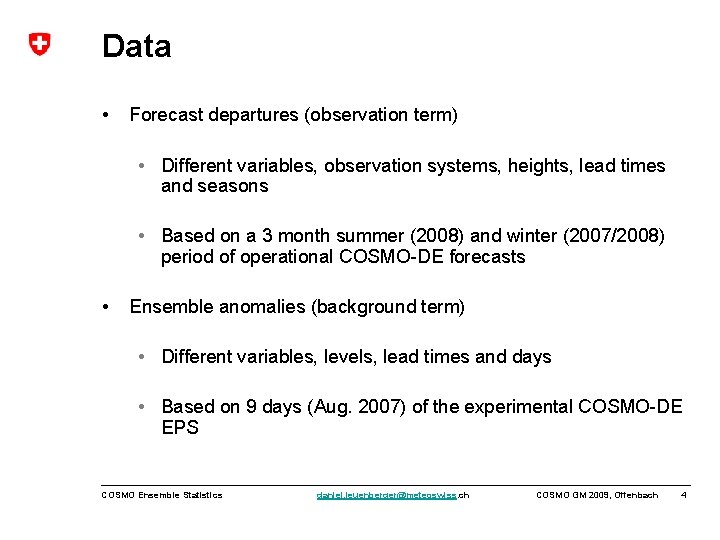
Data • Forecast departures (observation term) • Different variables, observation systems, heights, lead times and seasons • Based on a 3 month summer (2008) and winter (2007/2008) period of operational COSMO-DE forecasts • Ensemble anomalies (background term) • Different variables, levels, lead times and days • Based on 9 days (Aug. 2007) of the experimental COSMO-DE EPS COSMO Ensemble Statistics daniel. leuenberger@meteoswiss. ch COSMO GM 2009, Offenbach 4
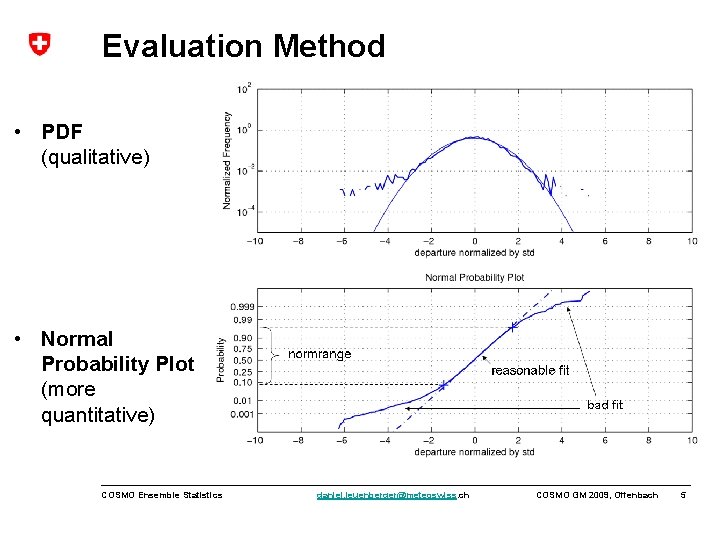
Evaluation Method • PDF (qualitative) • Normal Probability Plot (more quantitative) COSMO Ensemble Statistics daniel. leuenberger@meteoswiss. ch COSMO GM 2009, Offenbach 5
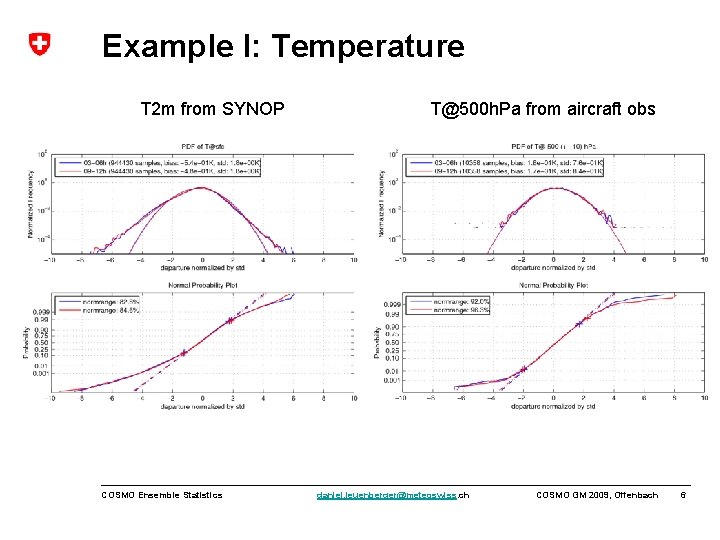
Example I: Temperature T 2 m from SYNOP COSMO Ensemble Statistics T@500 h. Pa from aircraft obs daniel. leuenberger@meteoswiss. ch COSMO GM 2009, Offenbach 6
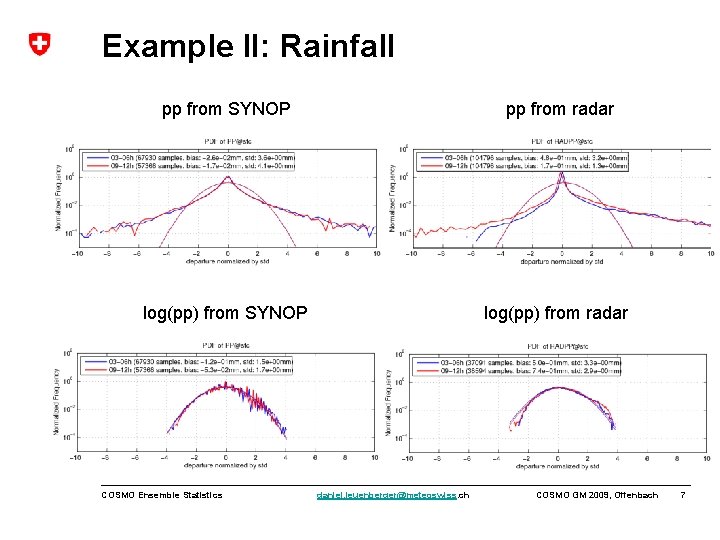
Example II: Rainfall pp from SYNOP pp from radar log(pp) from SYNOP log(pp) from radar COSMO Ensemble Statistics daniel. leuenberger@meteoswiss. ch COSMO GM 2009, Offenbach 7
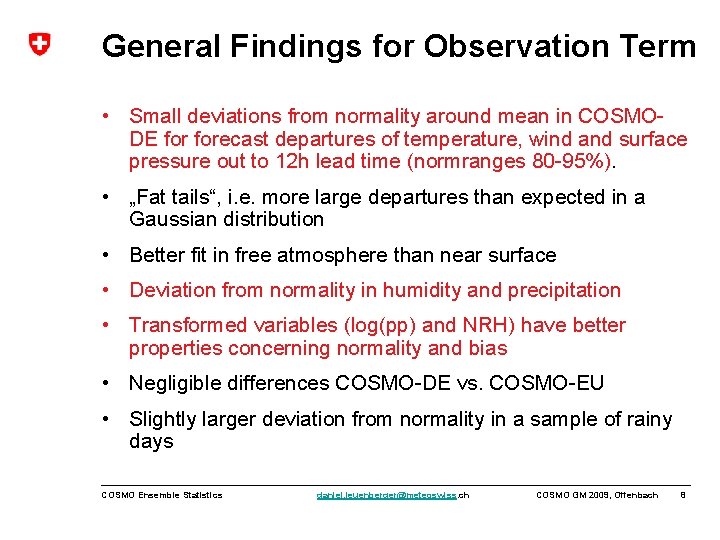
General Findings for Observation Term • Small deviations from normality around mean in COSMODE forecast departures of temperature, wind and surface pressure out to 12 h lead time (normranges 80 -95%). • „Fat tails“, i. e. more large departures than expected in a Gaussian distribution • Better fit in free atmosphere than near surface • Deviation from normality in humidity and precipitation • Transformed variables (log(pp) and NRH) have better properties concerning normality and bias • Negligible differences COSMO-DE vs. COSMO-EU • Slightly larger deviation from normality in a sample of rainy days COSMO Ensemble Statistics daniel. leuenberger@meteoswiss. ch COSMO GM 2009, Offenbach 8
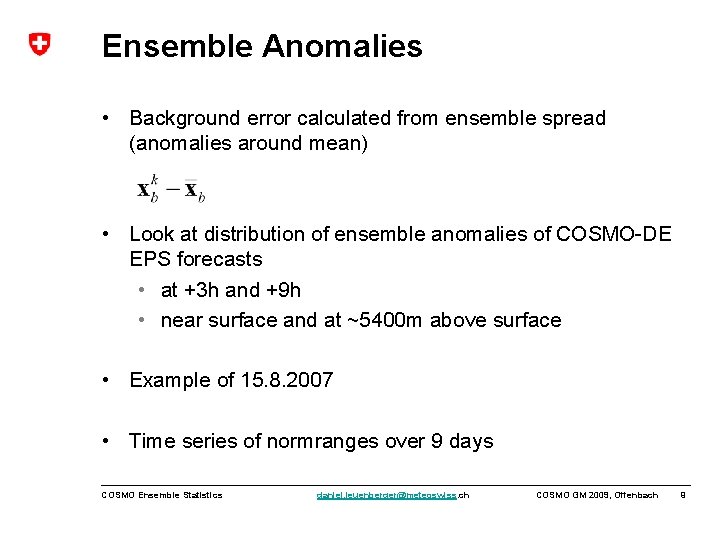
Ensemble Anomalies • Background error calculated from ensemble spread (anomalies around mean) • Look at distribution of ensemble anomalies of COSMO-DE EPS forecasts • at +3 h and +9 h • near surface and at ~5400 m above surface • Example of 15. 8. 2007 • Time series of normranges over 9 days COSMO Ensemble Statistics daniel. leuenberger@meteoswiss. ch COSMO GM 2009, Offenbach 9
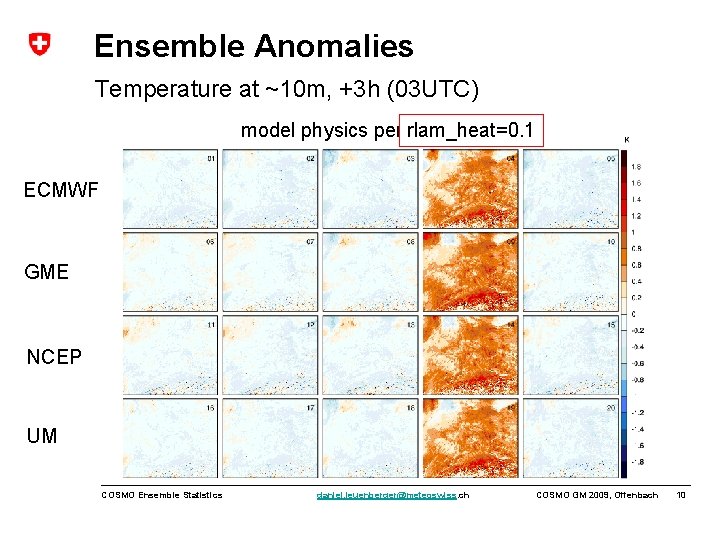
Ensemble Anomalies Temperature at ~10 m, +3 h (03 UTC) model physics perturbations rlam_heat=0. 1 ECMWF GME NCEP UM COSMO Ensemble Statistics daniel. leuenberger@meteoswiss. ch COSMO GM 2009, Offenbach 10
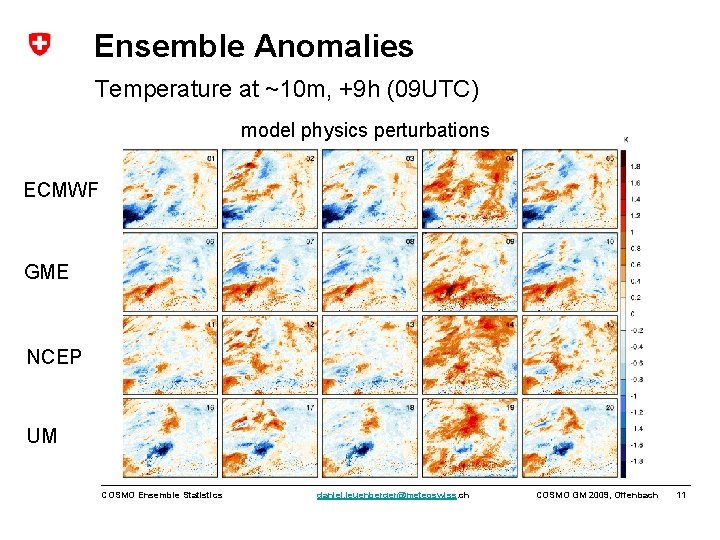
Ensemble Anomalies Temperature at ~10 m, +9 h (09 UTC) model physics perturbations ECMWF GME NCEP UM COSMO Ensemble Statistics daniel. leuenberger@meteoswiss. ch COSMO GM 2009, Offenbach 11
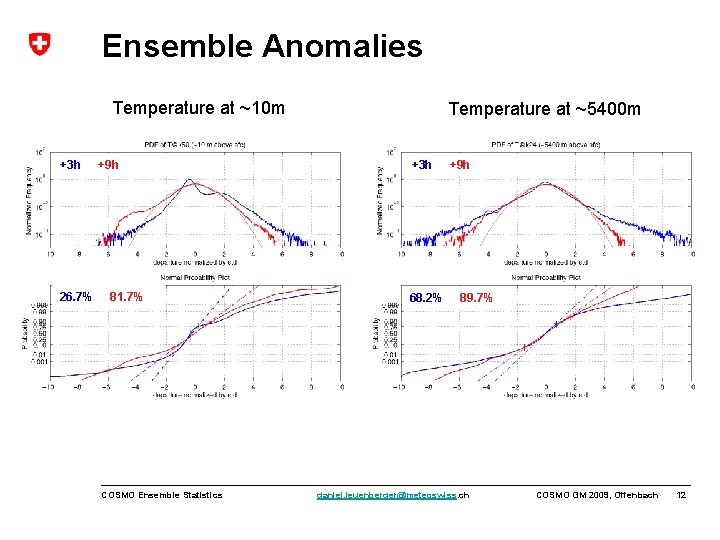
Ensemble Anomalies Temperature at ~10 m +3 h 26. 7% +9 h 81. 7% COSMO Ensemble Statistics Temperature at ~5400 m +3 h 68. 2% +9 h 89. 7% daniel. leuenberger@meteoswiss. ch COSMO GM 2009, Offenbach 12
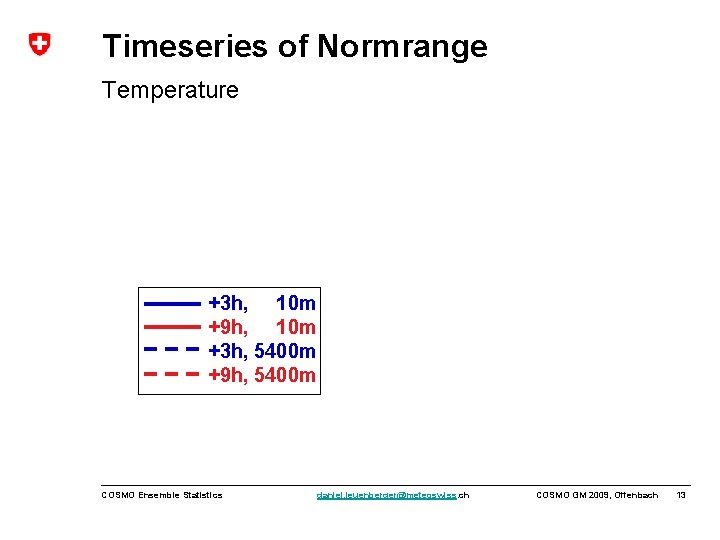
Timeseries of Normrange Temperature +3 h, 10 m +9 h, 10 m +3 h, 5400 m +9 h, 5400 m COSMO Ensemble Statistics daniel. leuenberger@meteoswiss. ch COSMO GM 2009, Offenbach 13
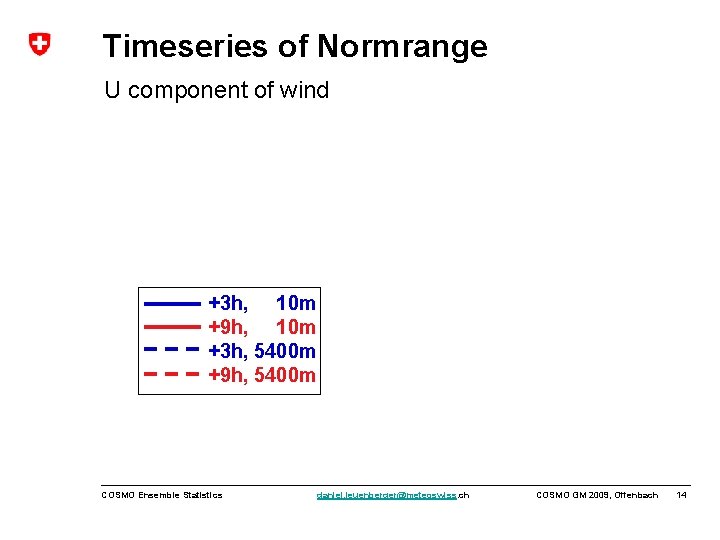
Timeseries of Normrange U component of wind +3 h, 10 m +9 h, 10 m +3 h, 5400 m +9 h, 5400 m COSMO Ensemble Statistics daniel. leuenberger@meteoswiss. ch COSMO GM 2009, Offenbach 14
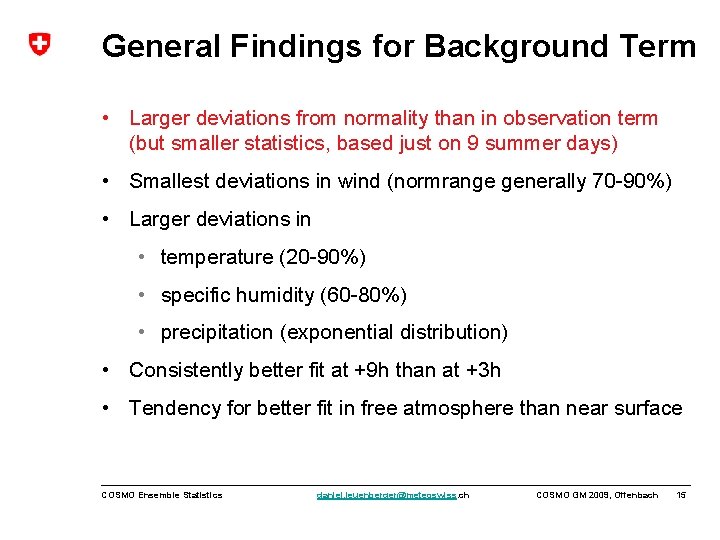
General Findings for Background Term • Larger deviations from normality than in observation term (but smaller statistics, based just on 9 summer days) • Smallest deviations in wind (normrange generally 70 -90%) • Larger deviations in • temperature (20 -90%) • specific humidity (60 -80%) • precipitation (exponential distribution) • Consistently better fit at +9 h than at +3 h • Tendency for better fit in free atmosphere than near surface COSMO Ensemble Statistics daniel. leuenberger@meteoswiss. ch COSMO GM 2009, Offenbach 15
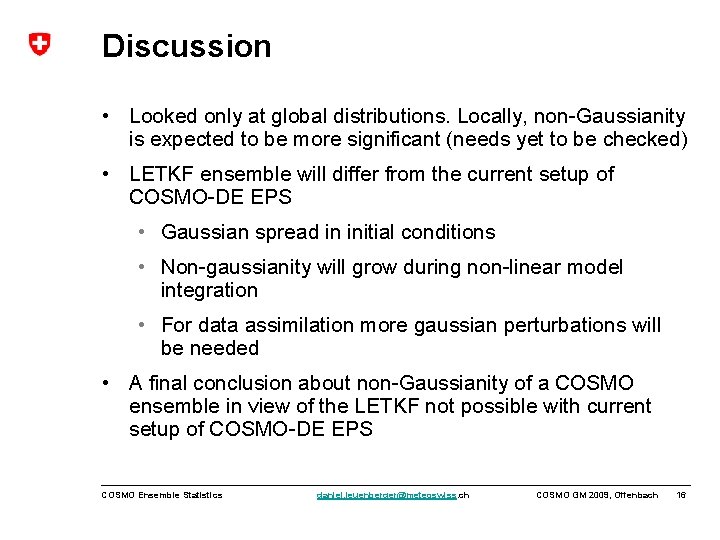
Discussion • Looked only at global distributions. Locally, non-Gaussianity is expected to be more significant (needs yet to be checked) • LETKF ensemble will differ from the current setup of COSMO-DE EPS • Gaussian spread in initial conditions • Non-gaussianity will grow during non-linear model integration • For data assimilation more gaussian perturbations will be needed • A final conclusion about non-Gaussianity of a COSMO ensemble in view of the LETKF not possible with current setup of COSMO-DE EPS COSMO Ensemble Statistics daniel. leuenberger@meteoswiss. ch COSMO GM 2009, Offenbach 16
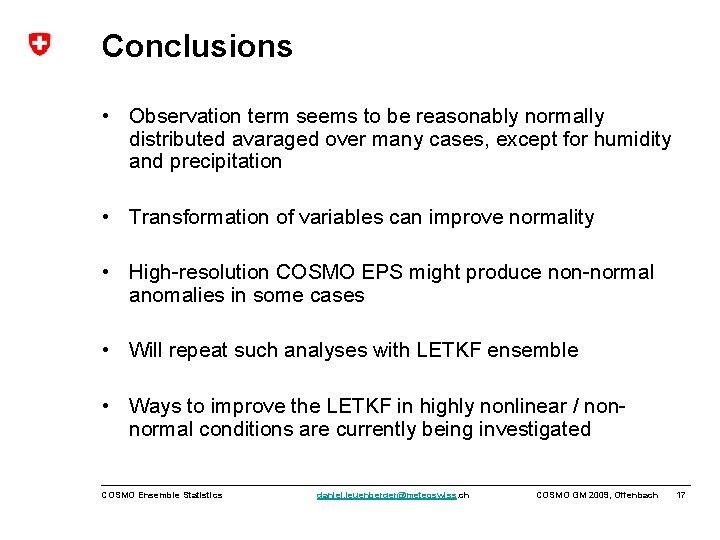
Conclusions • Observation term seems to be reasonably normally distributed avaraged over many cases, except for humidity and precipitation • Transformation of variables can improve normality • High-resolution COSMO EPS might produce non-normal anomalies in some cases • Will repeat such analyses with LETKF ensemble • Ways to improve the LETKF in highly nonlinear / nonnormal conditions are currently being investigated COSMO Ensemble Statistics daniel. leuenberger@meteoswiss. ch COSMO GM 2009, Offenbach 17
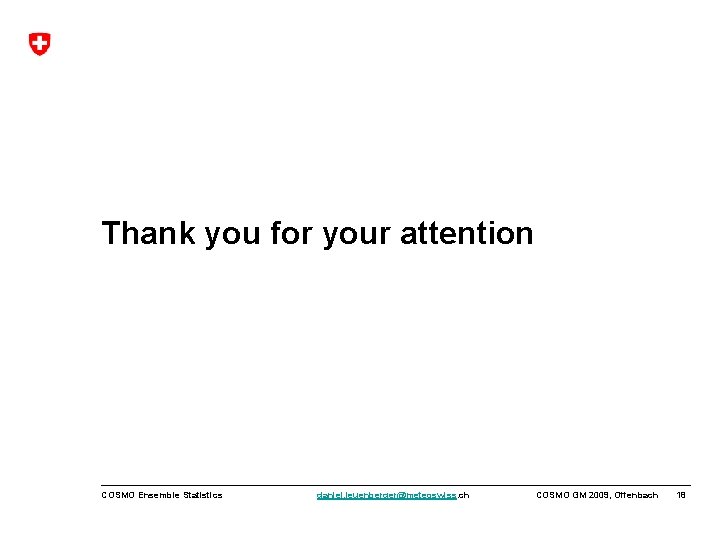
Thank you for your attention COSMO Ensemble Statistics daniel. leuenberger@meteoswiss. ch COSMO GM 2009, Offenbach 18
- Slides: 18