Effects of Virtual Manipulatives with Two Instructional Approaches
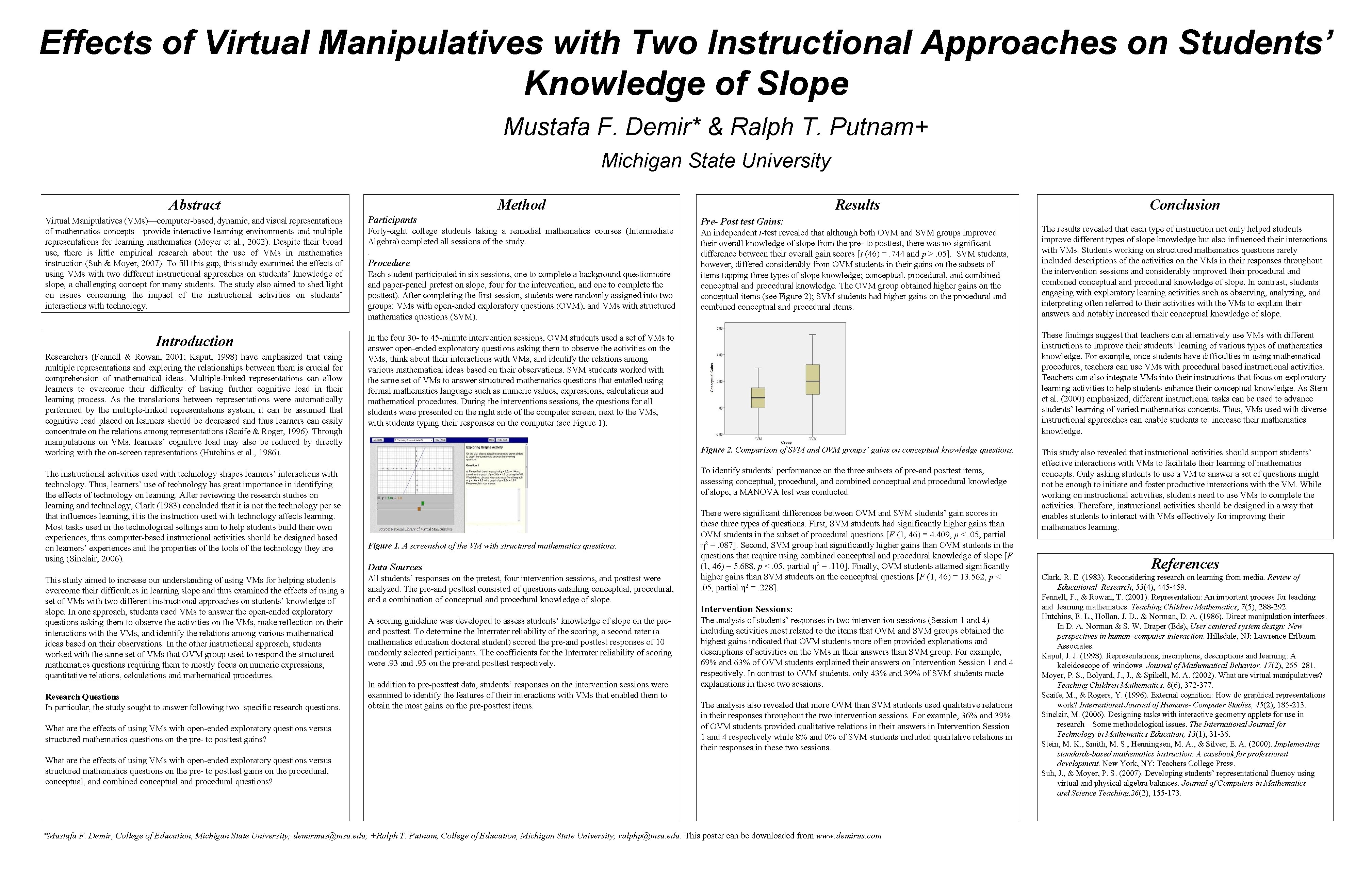
- Slides: 1
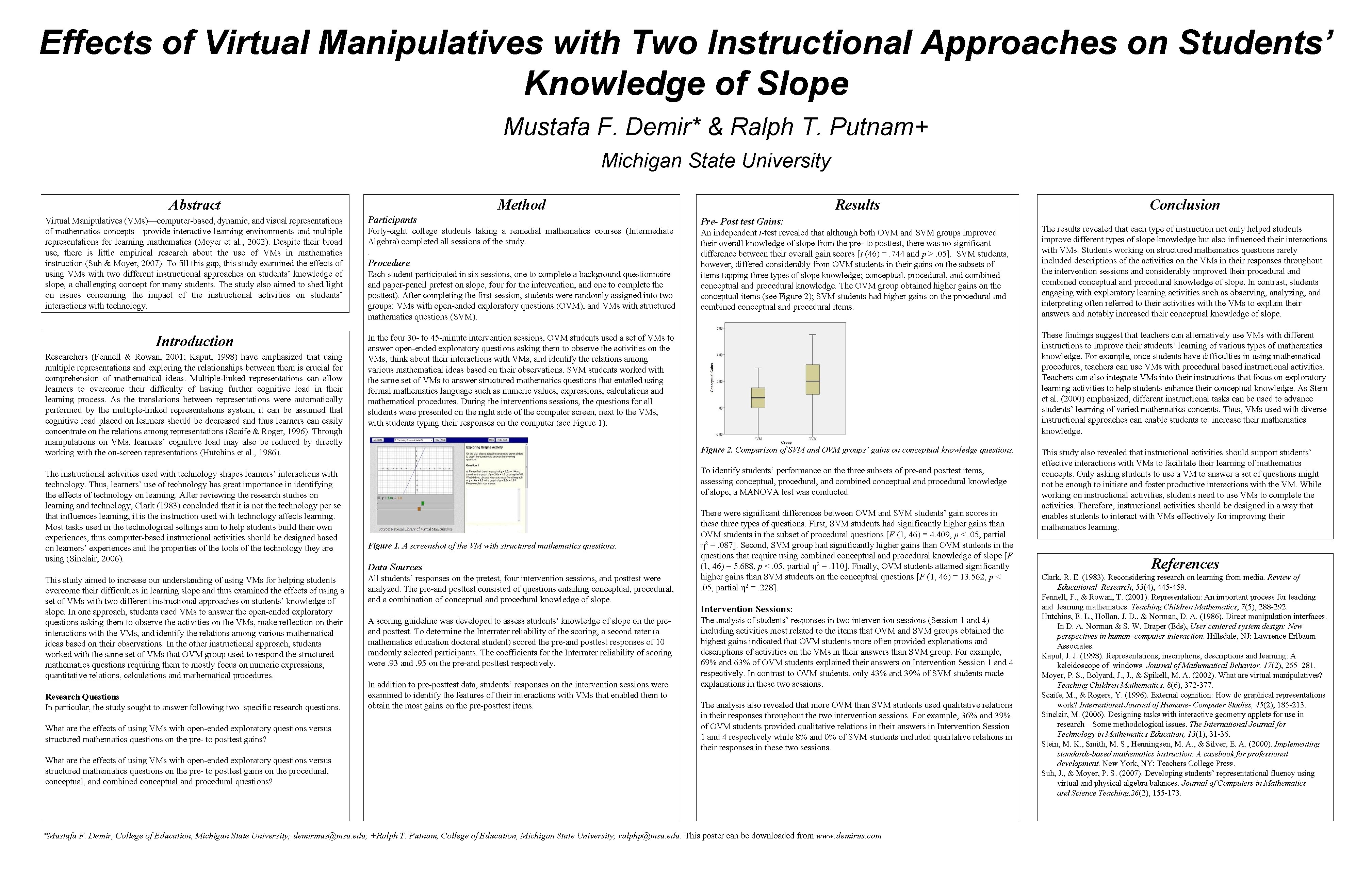
Effects of Virtual Manipulatives with Two Instructional Approaches on Students’ Knowledge of Slope Mustafa F. Demir* & Ralph T. Putnam+ Michigan State University Abstract Virtual Manipulatives (VMs)—computer-based, dynamic, and visual representations of mathematics concepts—provide interactive learning environments and multiple representations for learning mathematics (Moyer et al. , 2002). Despite their broad use, there is little empirical research about the use of VMs in mathematics instruction (Suh & Moyer, 2007). To fill this gap, this study examined the effects of using VMs with two different instructional approaches on students’ knowledge of slope, a challenging concept for many students. The study also aimed to shed light on issues concerning the impact of the instructional activities on students’ interactions with technology. Introduction Researchers (Fennell & Rowan, 2001; Kaput, 1998) have emphasized that using multiple representations and exploring the relationships between them is crucial for comprehension of mathematical ideas. Multiple-linked representations can allow learners to overcome their difficulty of having further cognitive load in their learning process. As the translations between representations were automatically performed by the multiple-linked representations system, it can be assumed that cognitive load placed on learners should be decreased and thus learners can easily concentrate on the relations among representations (Scaife & Roger, 1996). Through manipulations on VMs, learners’ cognitive load may also be reduced by directly working with the on-screen representations (Hutchins et al. , 1986). The instructional activities used with technology shapes learners’ interactions with technology. Thus, learners’ use of technology has great importance in identifying the effects of technology on learning. After reviewing the research studies on learning and technology, Clark (1983) concluded that it is not the technology per se that influences learning, it is the instruction used with technology affects learning. Most tasks used in the technological settings aim to help students build their own experiences, thus computer-based instructional activities should be designed based on learners’ experiences and the properties of the tools of the technology they are using (Sinclair, 2006). This study aimed to increase our understanding of using VMs for helping students overcome their difficulties in learning slope and thus examined the effects of using a set of VMs with two different instructional approaches on students’ knowledge of slope. In one approach, students used VMs to answer the open-ended exploratory questions asking them to observe the activities on the VMs, make reflection on their interactions with the VMs, and identify the relations among various mathematical ideas based on their observations. In the other instructional approach, students worked with the same set of VMs that OVM group used to respond the structured mathematics questions requiring them to mostly focus on numeric expressions, quantitative relations, calculations and mathematical procedures. Research Questions In particular, the study sought to answer following two specific research questions. What are the effects of using VMs with open-ended exploratory questions versus structured mathematics questions on the pre- to posttest gains? Method Participants Forty-eight college students taking a remedial mathematics courses (Intermediate Algebra) completed all sessions of the study. . Procedure Each student participated in six sessions, one to complete a background questionnaire and paper-pencil pretest on slope, four for the intervention, and one to complete the posttest). After completing the first session, students were randomly assigned into two groups: VMs with open-ended exploratory questions (OVM), and VMs with structured mathematics questions (SVM). Results Conclusion An independent t-test revealed that although both OVM and SVM groups improved their overall knowledge of slope from the pre- to posttest, there was no significant difference between their overall gain scores [t (46) =. 744 and p >. 05]. SVM students, however, differed considerably from OVM students in their gains on the subsets of items tapping three types of slope knowledge; conceptual, procedural, and combined conceptual and procedural knowledge. The OVM group obtained higher gains on the conceptual items (see Figure 2); SVM students had higher gains on the procedural and combined conceptual and procedural items. The results revealed that each type of instruction not only helped students improve different types of slope knowledge but also influenced their interactions with VMs. Students working on structured mathematics questions rarely included descriptions of the activities on the VMs in their responses throughout the intervention sessions and considerably improved their procedural and combined conceptual and procedural knowledge of slope. In contrast, students engaging with exploratory learning activities such as observing, analyzing, and interpreting often referred to their activities with the VMs to explain their answers and notably increased their conceptual knowledge of slope. Pre- Post test Gains: These findings suggest that teachers can alternatively use VMs with different instructions to improve their students’ learning of various types of mathematics knowledge. For example, once students have difficulties in using mathematical procedures, teachers can use VMs with procedural based instructional activities. Teachers can also integrate VMs into their instructions that focus on exploratory learning activities to help students enhance their conceptual knowledge. As Stein et al. (2000) emphasized, different instructional tasks can be used to advance students’ learning of varied mathematics concepts. Thus, VMs used with diverse instructional approaches can enable students to increase their mathematics knowledge. In the four 30 - to 45 -minute intervention sessions, OVM students used a set of VMs to answer open-ended exploratory questions asking them to observe the activities on the VMs, think about their interactions with VMs, and identify the relations among various mathematical ideas based on their observations. SVM students worked with the same set of VMs to answer structured mathematics questions that entailed using formal mathematics language such as numeric values, expressions, calculations and mathematical procedures. During the interventions sessions, the questions for all students were presented on the right side of the computer screen, next to the VMs, with students typing their responses on the computer (see Figure 1). Figure 2. Comparison of SVM and OVM groups’ gains on conceptual knowledge questions. To identify students’ performance on the three subsets of pre-and posttest items, assessing conceptual, procedural, and combined conceptual and procedural knowledge of slope, a MANOVA test was conducted. Figure 1. A screenshot of the VM with structured mathematics questions. Data Sources All students’ responses on the pretest, four intervention sessions, and posttest were analyzed. The pre-and posttest consisted of questions entailing conceptual, procedural, and a combination of conceptual and procedural knowledge of slope. A scoring guideline was developed to assess students’ knowledge of slope on the preand posttest. To determine the Interrater reliability of the scoring, a second rater (a mathematics education doctoral student) scored the pre-and posttest responses of 10 randomly selected participants. The coefficients for the Interrater reliability of scoring were. 93 and. 95 on the pre-and posttest respectively. In addition to pre-posttest data, students’ responses on the intervention sessions were examined to identify the features of their interactions with VMs that enabled them to obtain the most gains on the pre-posttest items. There were significant differences between OVM and SVM students’ gain scores in these three types of questions. First, SVM students had significantly higher gains than OVM students in the subset of procedural questions [F (1, 46) = 4. 409, p <. 05, partial η 2 =. 087]. Second, SVM group had significantly higher gains than OVM students in the questions that require using combined conceptual and procedural knowledge of slope [F (1, 46) = 5. 688, p <. 05, partial η 2 =. 110]. Finally, OVM students attained significantly higher gains than SVM students on the conceptual questions [F (1, 46) = 13. 562, p < . 05, partial η 2 =. 228]. Intervention Sessions: The analysis of students’ responses in two intervention sessions (Session 1 and 4) including activities most related to the items that OVM and SVM groups obtained the highest gains indicated that OVM students more often provided explanations and descriptions of activities on the VMs in their answers than SVM group. For example, 69% and 63% of OVM students explained their answers on Intervention Session 1 and 4 respectively. In contrast to OVM students, only 43% and 39% of SVM students made explanations in these two sessions. The analysis also revealed that more OVM than SVM students used qualitative relations in their responses throughout the two intervention sessions. For example, 36% and 39% of OVM students provided qualitative relations in their answers in Intervention Session 1 and 4 respectively while 8% and 0% of SVM students included qualitative relations in their responses in these two sessions. What are the effects of using VMs with open-ended exploratory questions versus structured mathematics questions on the pre- to posttest gains on the procedural, conceptual, and combined conceptual and procedural questions? *Mustafa F. Demir, College of Education, Michigan State University; demirmus@msu. edu; +Ralph T. Putnam, College of Education, Michigan State University; ralphp@msu. edu. This poster can be downloaded from www. demirus. com This study also revealed that instructional activities should support students’ effective interactions with VMs to facilitate their learning of mathematics concepts. Only asking students to use a VM to answer a set of questions might not be enough to initiate and foster productive interactions with the VM. While working on instructional activities, students need to use VMs to complete the activities. Therefore, instructional activities should be designed in a way that enables students to interact with VMs effectively for improving their mathematics learning. References Clark, R. E. (1983). Reconsidering research on learning from media. Review of Educational Research, 53(4), 445 -459. Fennell, F. , & Rowan, T. (2001). Representation: An important process for teaching and learning mathematics. Teaching Children Mathematics, 7(5), 288 -292. Hutchins, E. L. , Hollan, J. D. , & Norman, D. A. (1986). Direct manipulation interfaces. In D. A. Norman & S. W. Draper (Eds), User centered system design: New perspectives in human–computer interaction. Hillsdale, NJ: Lawrence Erlbaum Associates. Kaput, J. J. (1998). Representations, inscriptions, descriptions and learning: A kaleidoscope of windows. Journal of Mathematical Behavior, 17(2), 265– 281. Moyer, P. S. , Bolyard, J. , & Spikell, M. A. (2002). What are virtual manipulatives? Teaching Children Mathematics, 8(6), 372 -377. Scaife, M. , & Rogers, Y. (1996). External cognition: How do graphical representations work? International Journal of Humane- Computer Studies, 45(2), 185 -213. Sinclair, M. (2006). Designing tasks with interactive geometry applets for use in research – Some methodological issues. The International Journal for Technology in Mathematics Education, 13(1), 31 -36. Stein, M. K. , Smith, M. S. , Henningsen, M. A. , & Silver, E. A. (2000). Implementing standards-based mathematics instruction: A casebook for professional development. New York, NY: Teachers College Press. Suh, J. , & Moyer, P. S. (2007). Developing students’ representational fluency using virtual and physical algebra balances. Journal of Computers in Mathematics and Science Teaching, 26(2), 155 -173.