ECAI DEMO 27 TH 31 ST AUGUST 2012
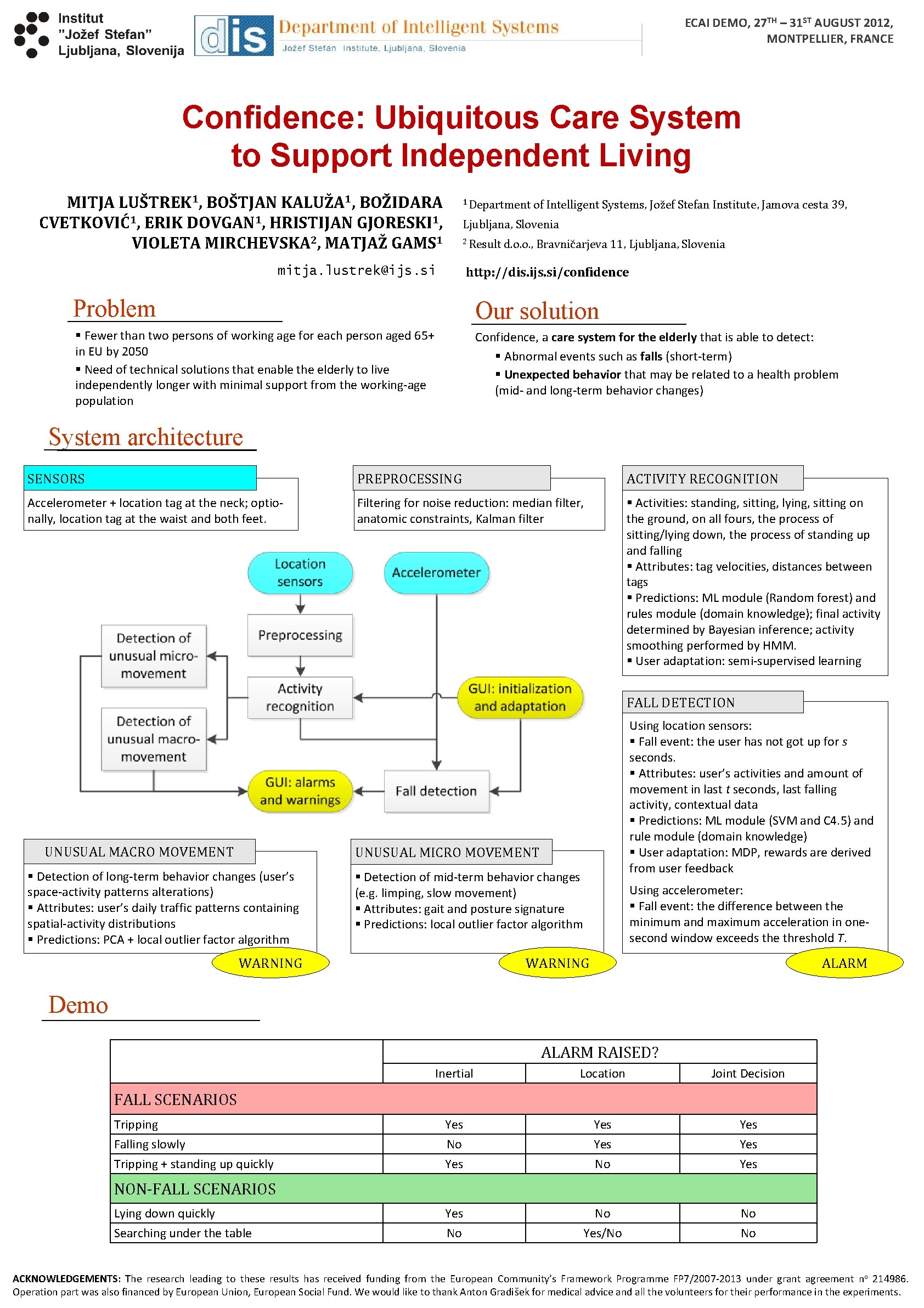
- Slides: 1
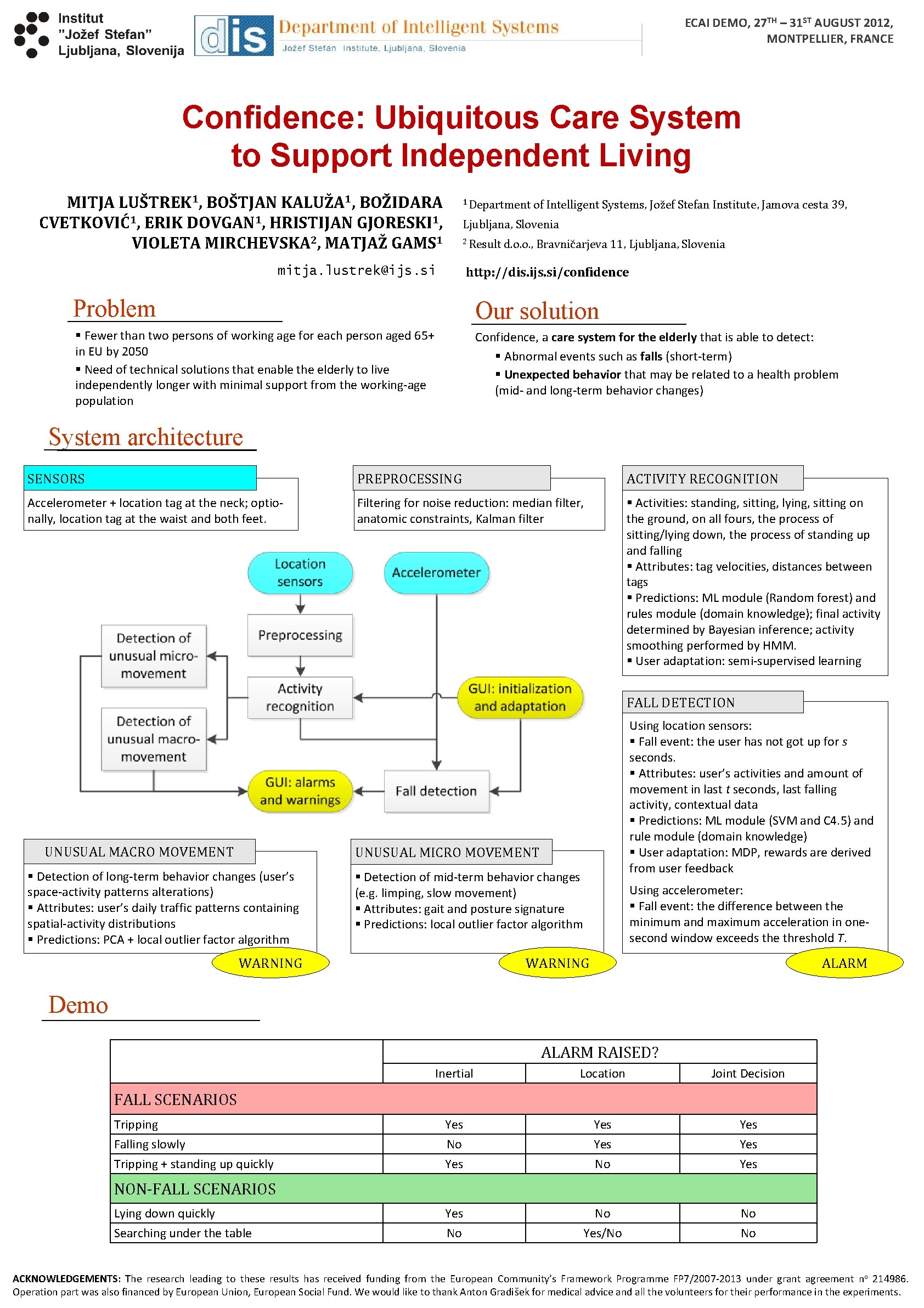
ECAI DEMO, 27 TH – 31 ST AUGUST 2012, MONTPELLIER, FRANCE Confidence: Ubiquitous Care System to Support Independent Living MITJA LUŠTREK 1, BOŠTJAN KALUŽA 1, BOŽIDARA CVETKOVIĆ1, ERIK DOVGAN 1, HRISTIJAN GJORESKI 1, VIOLETA MIRCHEVSKA 2, MATJAŽ GAMS 1 1 Department of Intelligent Systems, Jožef Stefan Institute, Jamova cesta 39, Ljubljana, Slovenia 2 Result d. o. o. , Bravničarjeva 11, Ljubljana, Slovenia http: //dis. ijs. si/confidence mitja. lustrek@ijs. si Problem Our solution § Fewer than two persons of working age for each person aged 65+ in EU by 2050 § Need of technical solutions that enable the elderly to live independently longer with minimal support from the working-age population Confidence, a care system for the elderly that is able to detect: § Abnormal events such as falls (short-term) § Unexpected behavior that may be related to a health problem (mid- and long-term behavior changes) System architecture SENSORS PREPROCESSING ACTIVITY RECOGNITION Accelerometer + location tag at the neck; optionally, location tag at the waist and both feet. Filtering for noise reduction: median filter, anatomic constraints, Kalman filter § Activities: standing, sitting, lying, sitting on the ground, on all fours, the process of sitting/lying down, the process of standing up and falling § Attributes: tag velocities, distances between tags § Predictions: ML module (Random forest) and rules module (domain knowledge); final activity determined by Bayesian inference; activity smoothing performed by HMM. § User adaptation: semi-supervised learning FALL DETECTION UNUSUAL MACRO MOVEMENT Using location sensors: § Fall event: the user has not got up for s seconds. § Attributes: user’s activities and amount of movement in last t seconds, last falling activity, contextual data § Predictions: ML module (SVM and C 4. 5) and rule module (domain knowledge) § User adaptation: MDP, rewards are derived from user feedback UNUSUAL MICRO MOVEMENT § Detection of long-term behavior changes (user’s space-activity patterns alterations) § Attributes: user’s daily traffic patterns containing spatial-activity distributions § Predictions: PCA + local outlier factor algorithm § Detection of mid-term behavior changes (e. g. limping, slow movement) § Attributes: gait and posture signature § Predictions: local outlier factor algorithm WARNING Using accelerometer: § Fall event: the difference between the minimum and maximum acceleration in onesecond window exceeds the threshold T. WARNING ALARM Demo ALARM RAISED? Inertial Location Joint Decision Tripping Yes Yes Falling slowly Tripping + standing up quickly No Yes Yes Yes No No Yes/No No No FALL SCENARIOS NON-FALL SCENARIOS Lying down quickly Searching under the table ACKNOWLEDGEMENTS: The research leading to these results has received funding from the European Community’s Framework Programme FP 7/2007 -2013 under grant agreement no 214986. Operation part was also financed by European Union, European Social Fund. We would like to thank Anton Gradišek for medical advice and all the volunteers for their performance in the experiments.