Dr Liam J Mc Guffin RCUK Academic Fellow
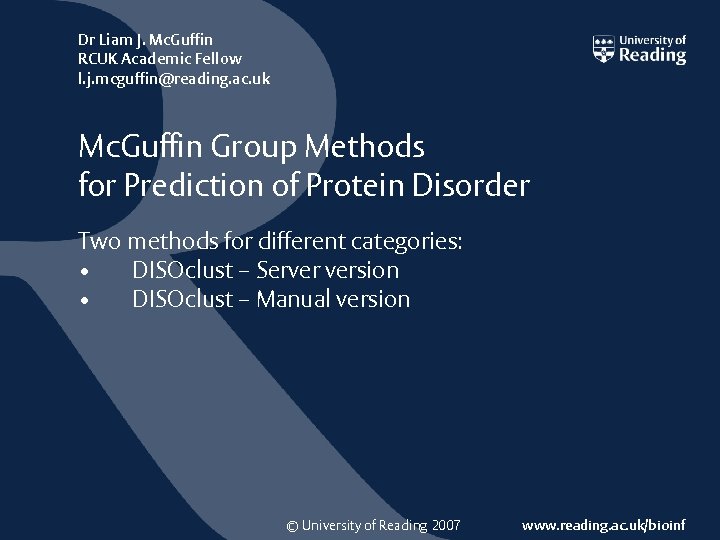
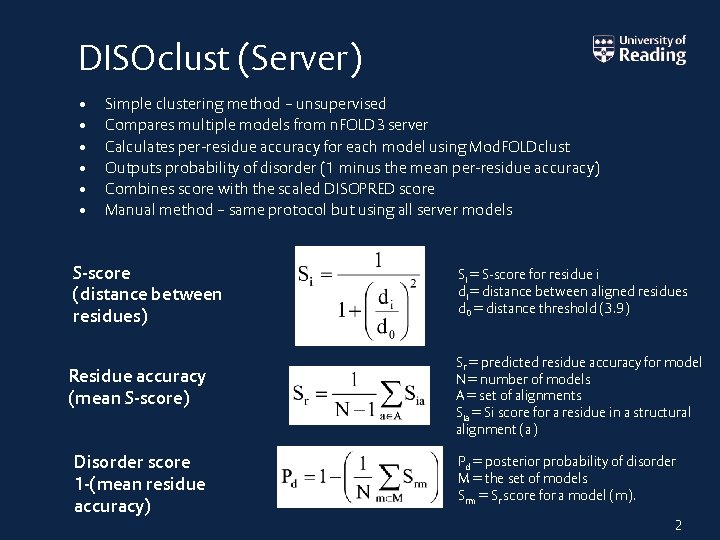
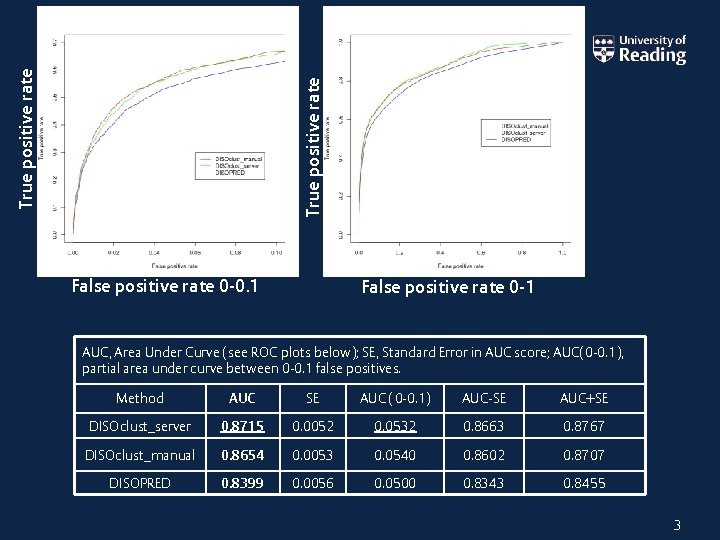
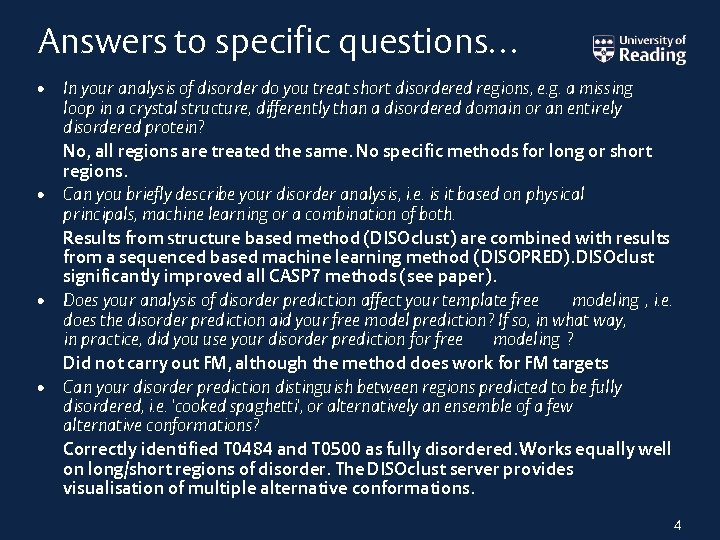
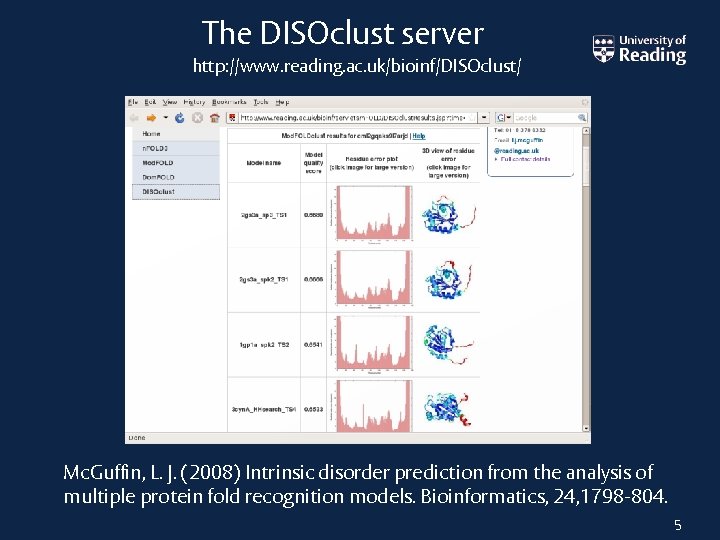
- Slides: 5
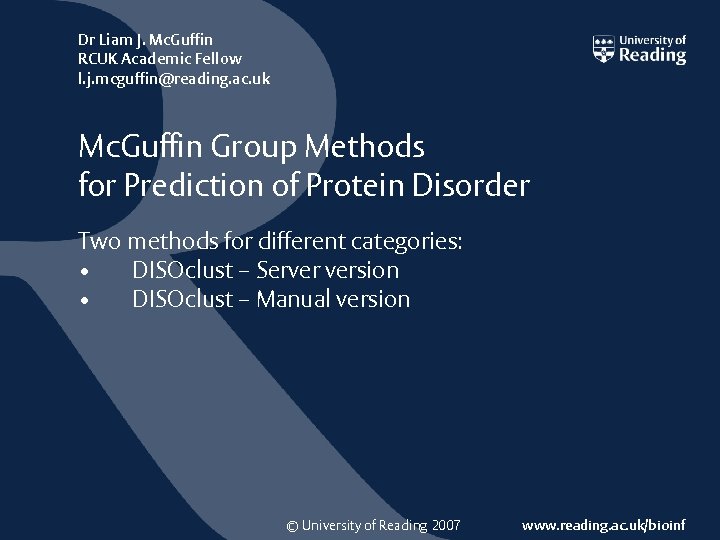
Dr Liam J. Mc. Guffin RCUK Academic Fellow l. j. mcguffin@reading. ac. uk Mc. Guffin Group Methods for Prediction of Protein Disorder Two methods for different categories: • DISOclust – Server version • DISOclust – Manual version © University of Reading 2007 www. reading. ac. uk/bioinf
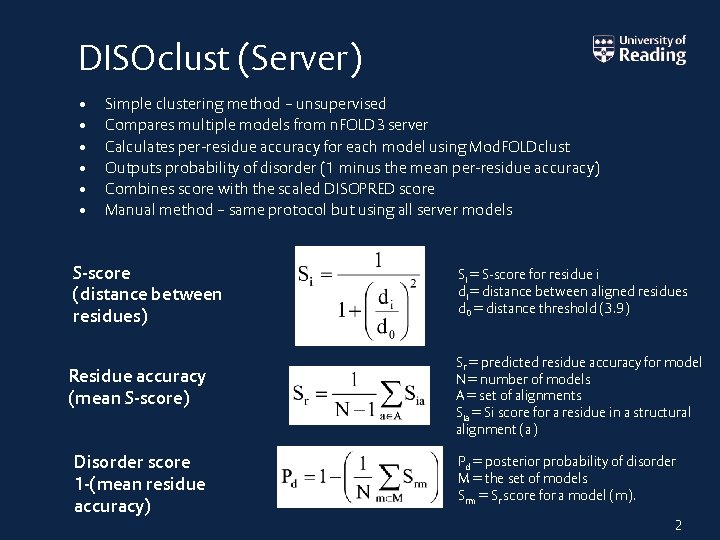
DISOclust (Server) • • • Simple clustering method – unsupervised Compares multiple models from n. FOLD 3 server Calculates per-residue accuracy for each model using Mod. FOLDclust Outputs probability of disorder (1 minus the mean per-residue accuracy) Combines score with the scaled DISOPRED score Manual method – same protocol but using all server models S-score (distance between residues) Residue accuracy (mean S-score) Disorder score 1 -(mean residue accuracy) Si = S-score for residue i di = distance between aligned residues d 0 = distance threshold (3. 9) Sr = predicted residue accuracy for model N = number of models A = set of alignments Sia = Si score for a residue in a structural alignment (a) Pd = posterior probability of disorder M = the set of models Srm = Sr score for a model (m). 2
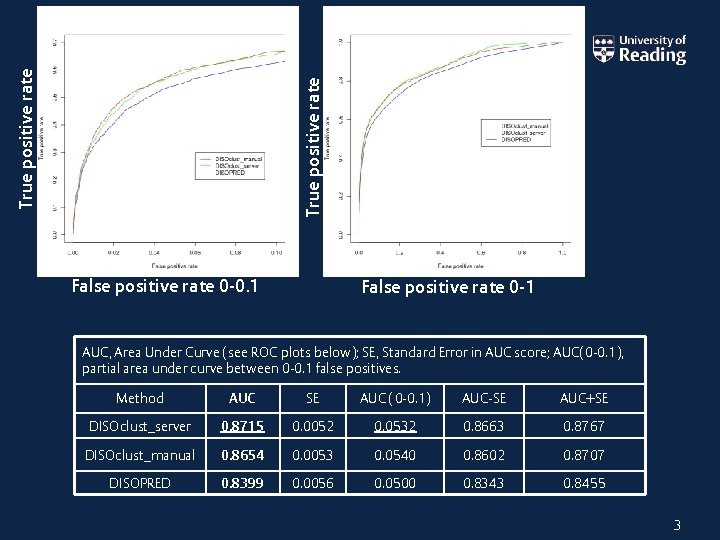
True positive rate False positive rate 0 -0. 1 False positive rate 0 -1 AUC, Area Under Curve (see ROC plots below); SE, Standard Error in AUC score; AUC(0 -0. 1), partial area under curve between 0 -0. 1 false positives. Method AUC SE AUC (0 -0. 1) AUC-SE AUC+SE DISOclust_server 0. 8715 0. 0052 0. 0532 0. 8663 0. 8767 DISOclust_manual 0. 8654 0. 0053 0. 0540 0. 8602 0. 8707 DISOPRED 0. 8399 0. 0056 0. 0500 0. 8343 0. 8455 3
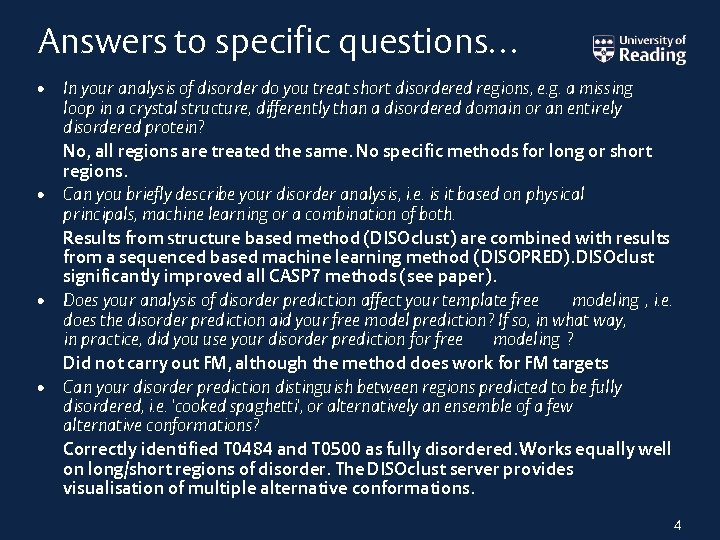
Answers to specific questions… • In your analysis of disorder do you treat short disordered regions, e. g. a missing loop in a crystal structure, differently than a disordered domain or an entirely disordered protein? No, all regions are treated the same. No specific methods for long or short regions. • Can you briefly describe your disorder analysis, i. e. is it based on physical principals, machine learning or a combination of both. Results from structure based method (DISOclust) are combined with results from a sequenced based machine learning method (DISOPRED). DISOclust significantly improved all CASP 7 methods (see paper). • Does your analysis of disorder prediction affect your template free modeling , i. e. does the disorder prediction aid your free model prediction? If so, in what way, in practice, did you use your disorder prediction for free modeling ? Did not carry out FM, although the method does work for FM targets • Can your disorder prediction distinguish between regions predicted to be fully disordered, i. e. 'cooked spaghetti', or alternatively an ensemble of a few alternative conformations? Correctly identified T 0484 and T 0500 as fully disordered. Works equally well on long/short regions of disorder. The DISOclust server provides visualisation of multiple alternative conformations. 4
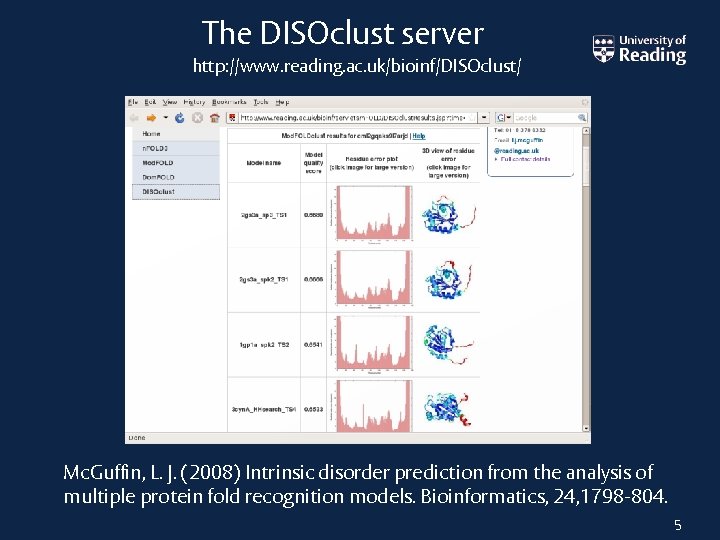
The DISOclust server http: //www. reading. ac. uk/bioinf/DISOclust/ Mc. Guffin, L. J. (2008) Intrinsic disorder prediction from the analysis of multiple protein fold recognition models. Bioinformatics, 24, 1798 -804. 5