CrossView Action Synthesis Kara Schatz Dr Yogesh Rawat
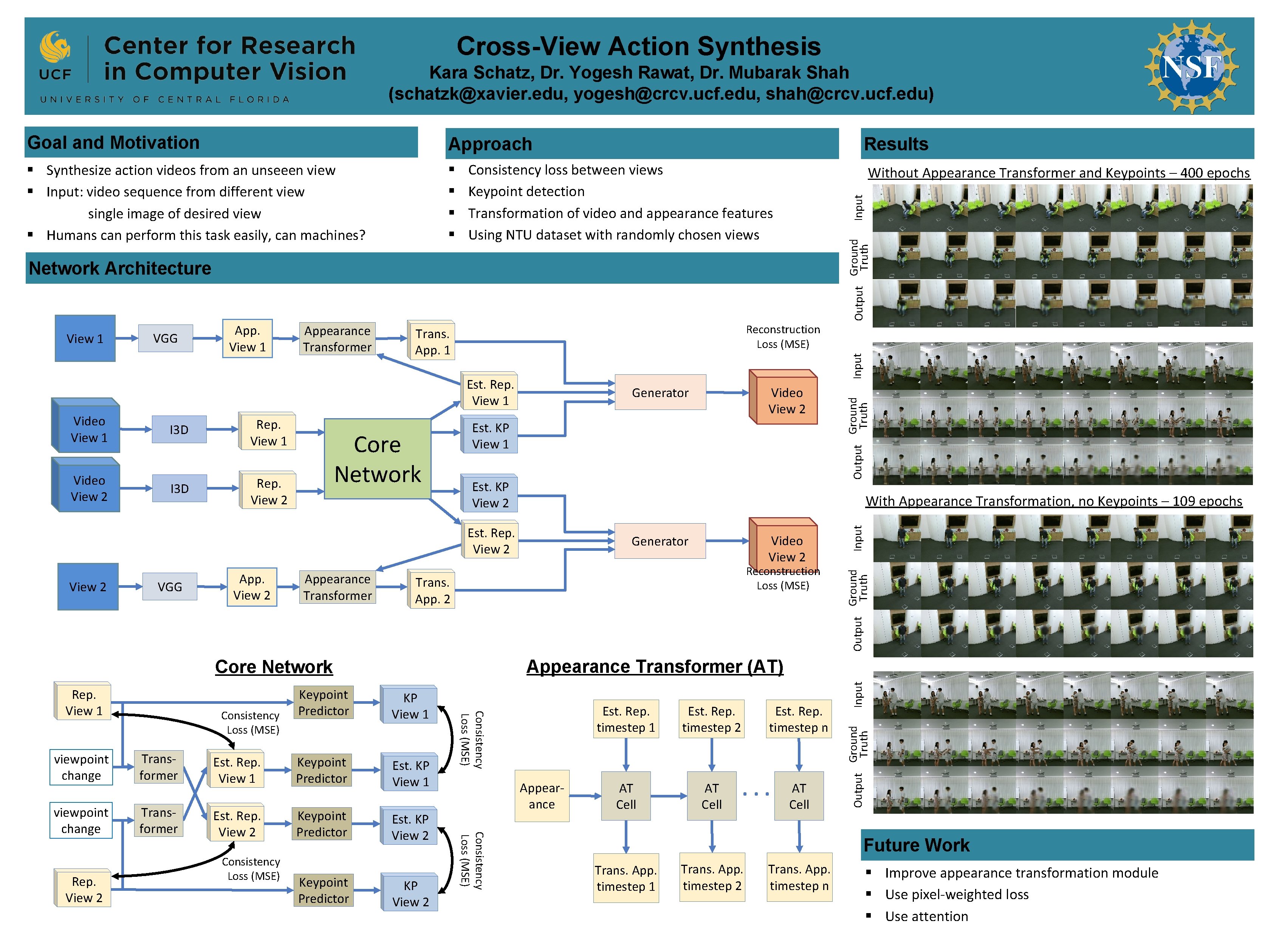
- Slides: 1
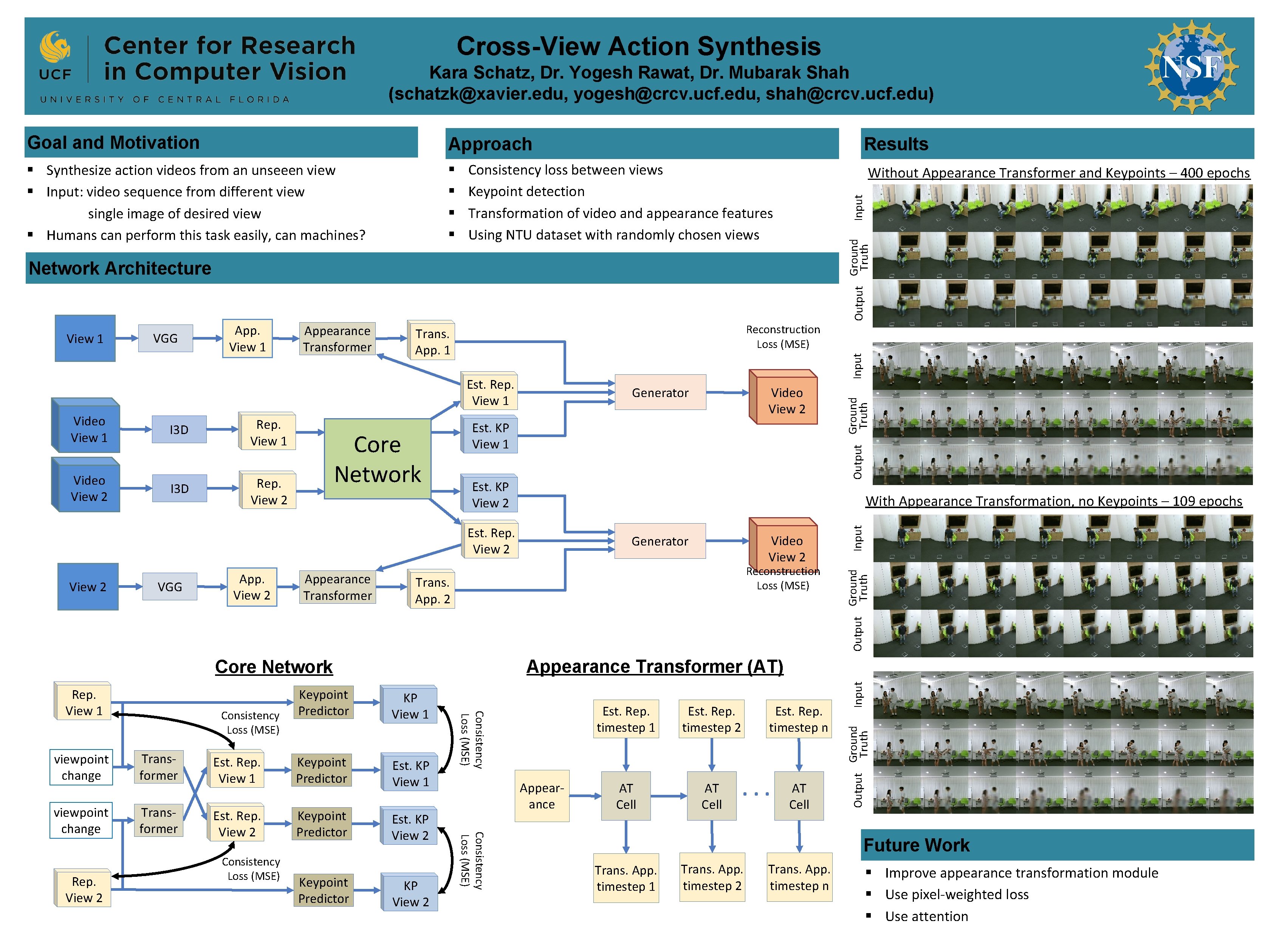
Cross-View Action Synthesis Kara Schatz, Dr. Yogesh Rawat, Dr. Mubarak Shah (schatzk@xavier. edu, yogesh@crcv. ucf. edu, shah@crcv. ucf. edu) Goal and Motivation Approach § Synthesize action videos from an unseeen view § Input: video sequence from different view single image of desired view § Humans can perform this task easily, can machines? § § Results Consistency loss between views Keypoint detection Transformation of video and appearance features Using NTU dataset with randomly chosen views Output Ground Truth Input Without Appearance Transformer and Keypoints – 400 epochs Network Architecture Reconstruction Loss (MSE) Est. Rep. View 1 I 3 D Rep. View 2 Core Network Est. KP View 1 Est. KP View 2 With Appearance Transformation, no Keypoints – 109 epochs Est. Rep. View 2 VGG App. View 2 Appearance Transformer Reconstruction Loss (MSE) Trans. App. 2 Appearance Transformer (AT) Core Network Consistency Loss (MSE) Keypoint Predictor KP View 1 Transformer Est. Rep. View 1 Keypoint Predictor Est. KP View 1 viewpoint change Transformer Est. Rep. View 2 Keypoint Predictor Est. KP View 2 Rep. View 2 Consistency Loss (MSE) Keypoint Predictor KP View 2 Appearance Consistency Loss (MSE) viewpoint change Consistency Loss (MSE) Rep. View 1 Video View 2 Generator Input Video View 2 I 3 D Rep. View 1 Video View 2 Output Ground Truth Video View 1 Generator Output Ground Truth Input Trans. App. 1 Est. Rep. timestep 2 AT Cell Est. Rep. timestep n . . . AT Cell Input VGG Appearance Transformer Ground Output Truth View 1 App. View 1 Future Work Trans. App. timestep 1 Trans. App. timestep 2 Trans. App. timestep n § Improve appearance transformation module § Use pixel-weighted loss § Use attention