Content Driven Trust Propagation Framwork V G Vinod
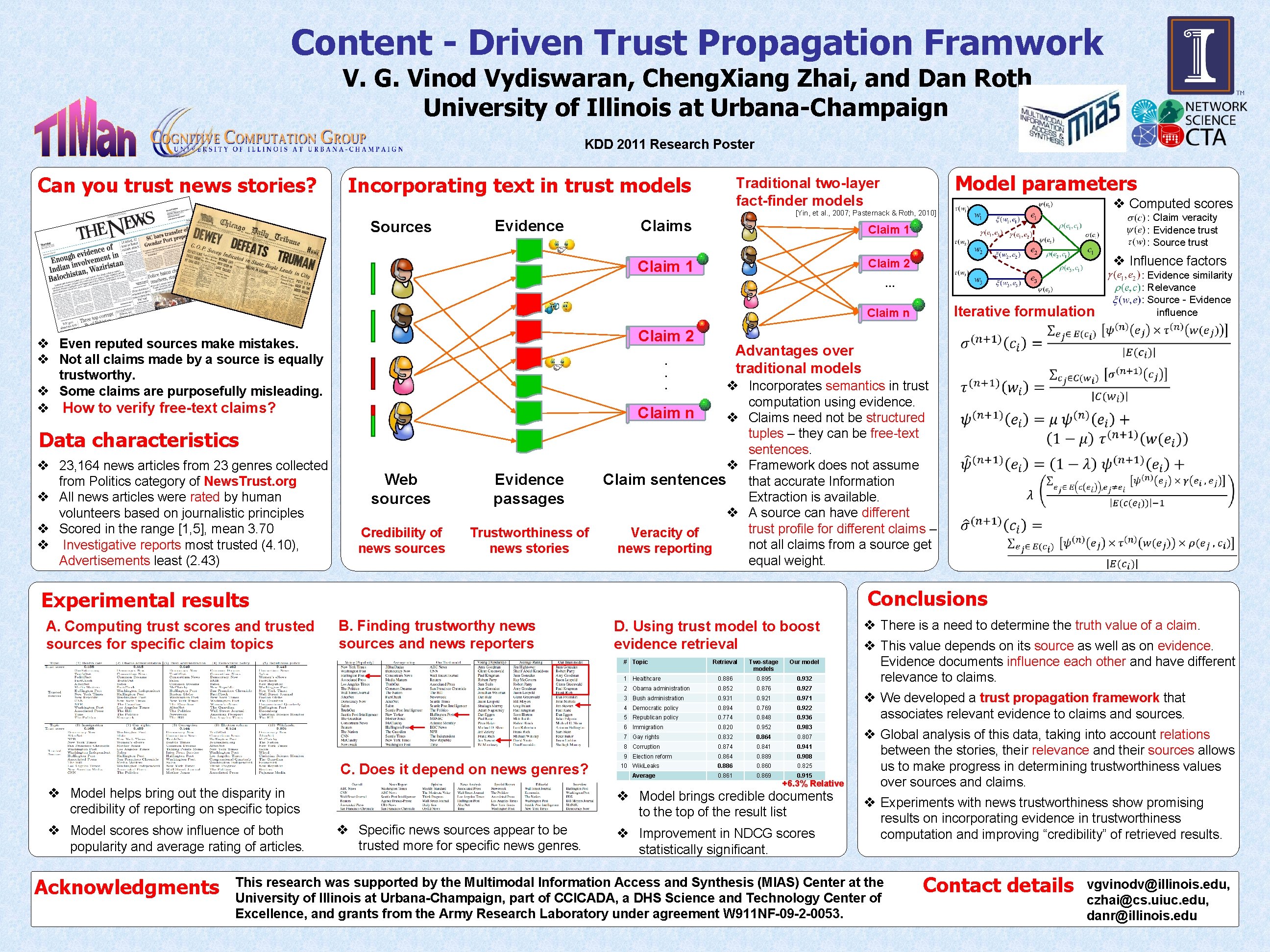
- Slides: 1
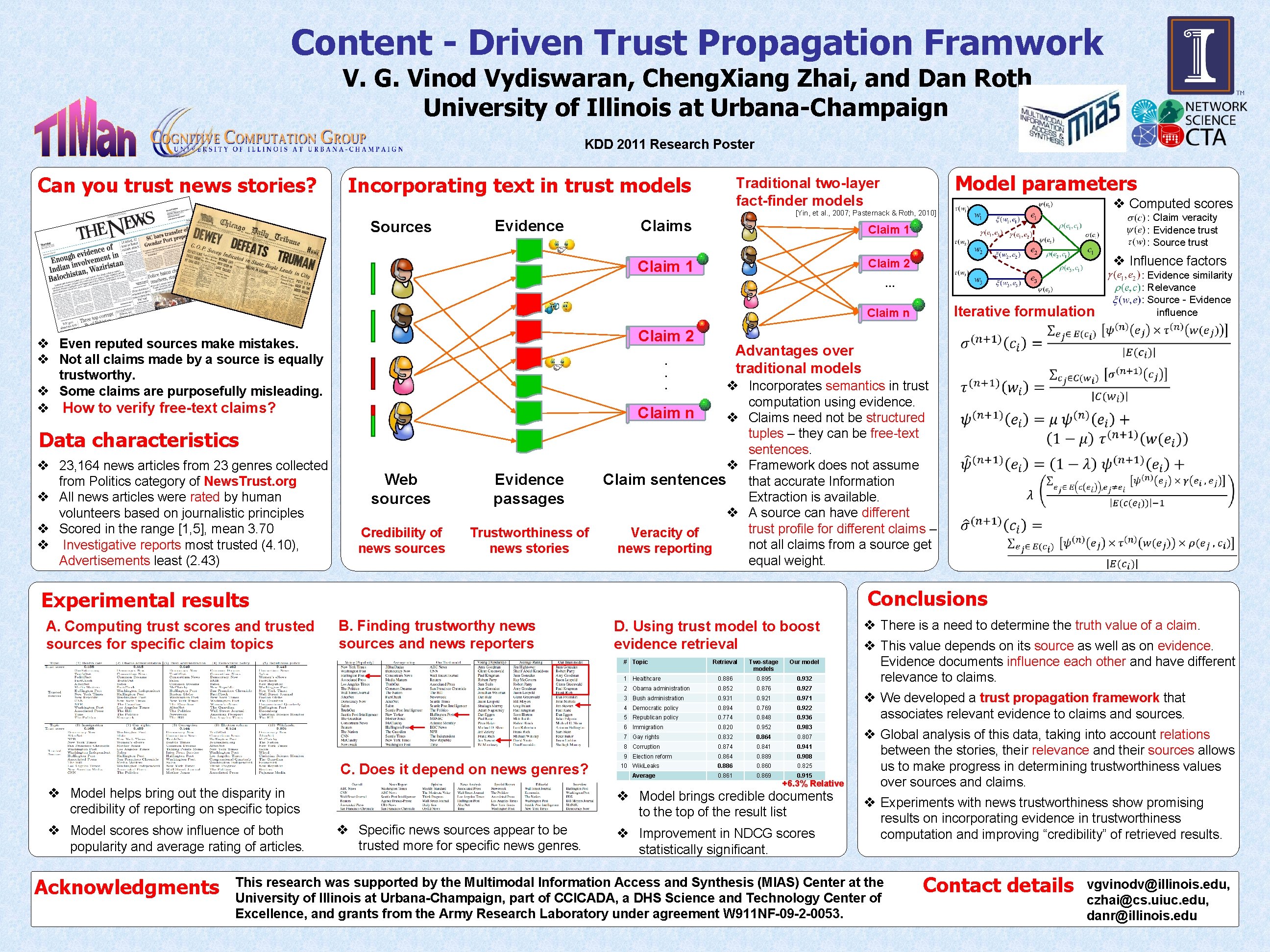
Content - Driven Trust Propagation Framwork V. G. Vinod Vydiswaran, Cheng. Xiang Zhai, and Dan Roth University of Illinois at Urbana-Champaign KDD 2011 Research Poster Can you trust news stories? Incorporating text in trust models Sources Evidence Model parameters Traditional two-layer fact-finder models v Computed scores [Yin, et al. , 2007; Pasternack & Roth, 2010] Claims : Claim veracity : Evidence trust : Source trust Claim 1 v Influence factors Claim 2 Claim 1 … Iterative formulation Claim 2 v Even reputed sources make mistakes. v Not all claims made by a source is equally trustworthy. v Some claims are purposefully misleading. v How to verify free-text claims? v 23, 164 news articles from 23 genres collected from Politics category of News. Trust. org v All news articles were rated by human volunteers based on journalistic principles v Scored in the range [1, 5], mean 3. 70 v Investigative reports most trusted (4. 10), Advertisements least (2. 43) Advantages over traditional models . . . Data characteristics Web sources Evidence passages Credibility of news sources Trustworthiness of news stories v Incorporates semantics in trust computation using evidence. Claim n v Claims need not be structured tuples – they can be free-text sentences. v Framework does not assume Claim sentences that accurate Information Extraction is available. v A source can have different trust profile for different claims – Veracity of not all claims from a source get news reporting equal weight. Conclusions Experimental results A. Computing trust scores and trusted sources for specific claim topics B. Finding trustworthy news sources and news reporters D. Using trust model to boost evidence retrieval # Topic C. Does it depend on news genres? v Model helps bring out the disparity in credibility of reporting on specific topics v Model scores show influence of both popularity and average rating of articles. Acknowledgments : Evidence similarity : Relevance : Source - Evidence influence Retrieval Two-stage models Our model 1 Healthcare 0. 886 0. 895 0. 932 2 Obama administration 0. 852 0. 876 0. 927 3 Bush administration 0. 931 0. 921 0. 971 4 Democratic policy 0. 894 0. 769 0. 922 5 Republican policy 0. 774 0. 848 0. 936 6 Immigration 0. 820 0. 952 0. 983 7 Gay rights 0. 832 0. 864 0. 807 8 Corruption 0. 874 0. 841 0. 941 9 Election reform 0. 864 0. 889 0. 908 0. 886 0. 860 0. 825 0. 861 0. 869 0. 915 10 Wiki. Leaks Average +6. 3% Relative v Model brings credible documents to the top of the result list v Specific news sources appear to be trusted more for specific news genres. v Improvement in NDCG scores statistically significant. v There is a need to determine the truth value of a claim. v This value depends on its source as well as on evidence. Evidence documents influence each other and have different relevance to claims. v We developed a trust propagation framework that associates relevant evidence to claims and sources. v Global analysis of this data, taking into account relations between the stories, their relevance and their sources allows us to make progress in determining trustworthiness values over sources and claims. v Experiments with news trustworthiness show promising results on incorporating evidence in trustworthiness computation and improving “credibility” of retrieved results. This research was supported by the Multimodal Information Access and Synthesis (MIAS) Center at the University of Illinois at Urbana-Champaign, part of CCICADA, a DHS Science and Technology Center of Excellence, and grants from the Army Research Laboratory under agreement W 911 NF-09 -2 -0053. Contact details vgvinodv@illinois. edu, czhai@cs. uiuc. edu, danr@illinois. edu