Concepts And Generic Knowledge Concepts Concepts are categories
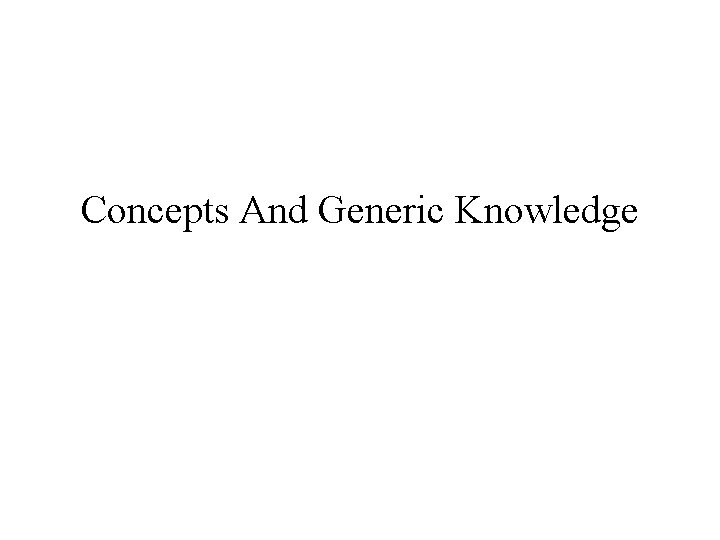
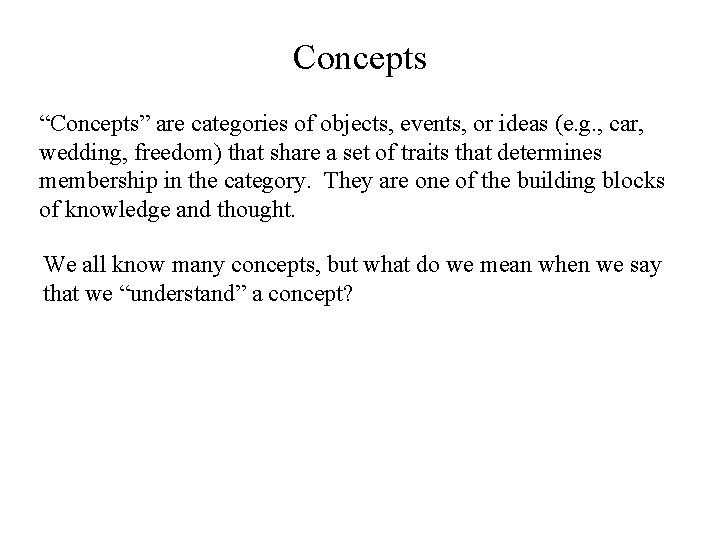
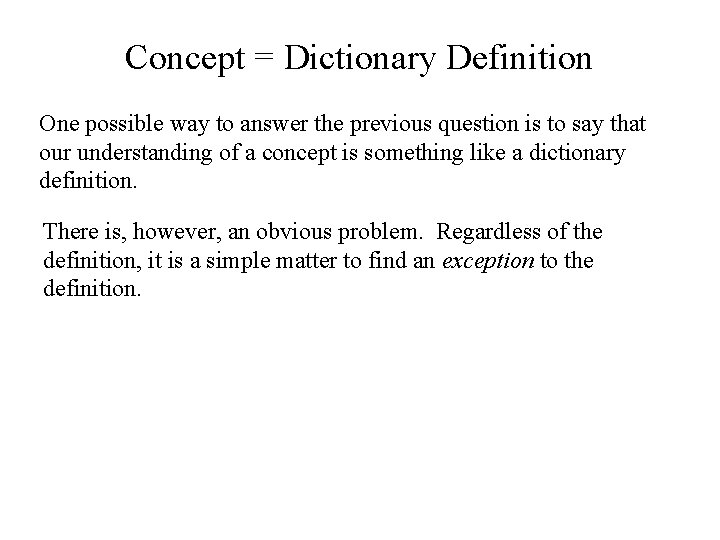
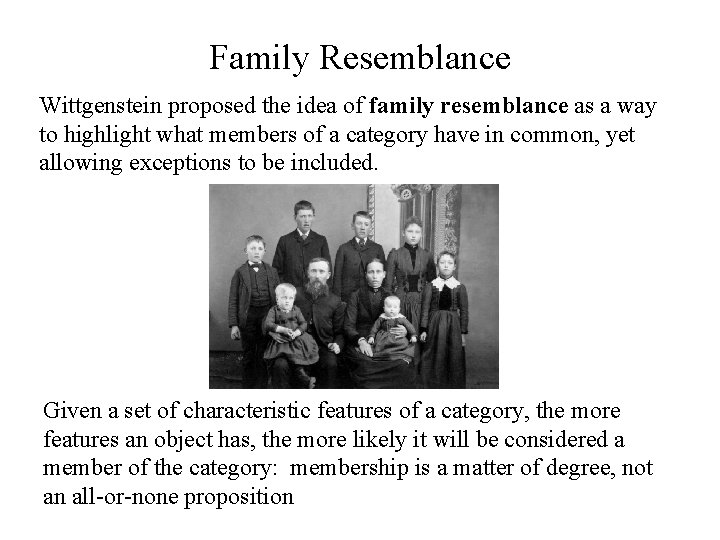
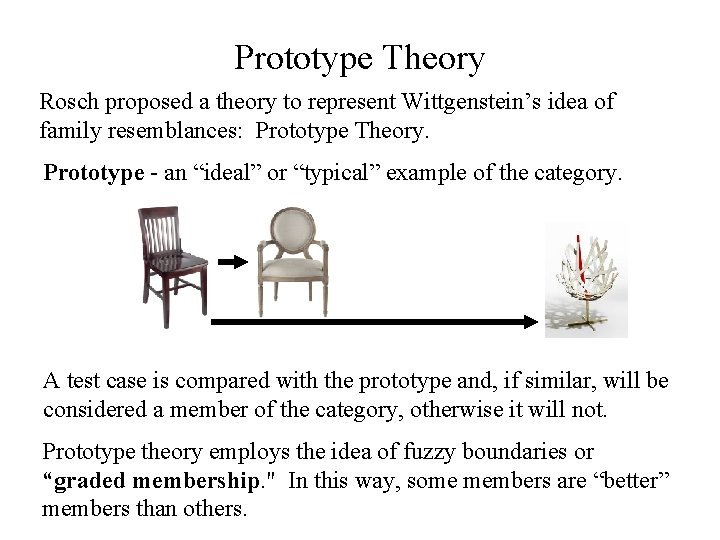
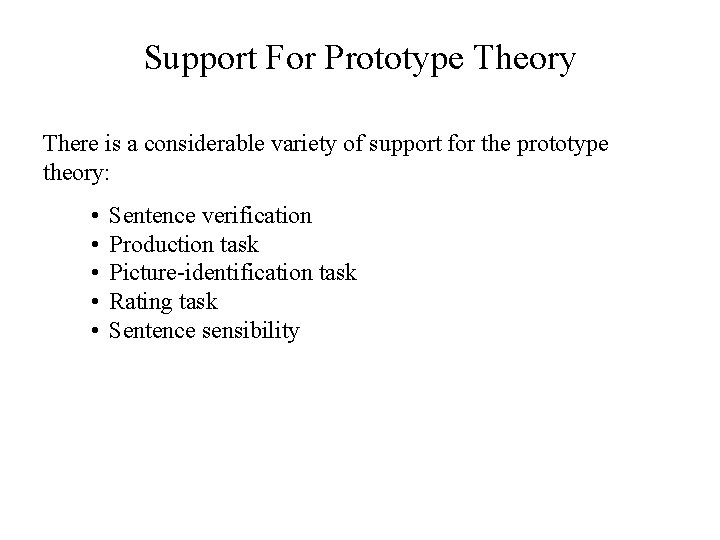
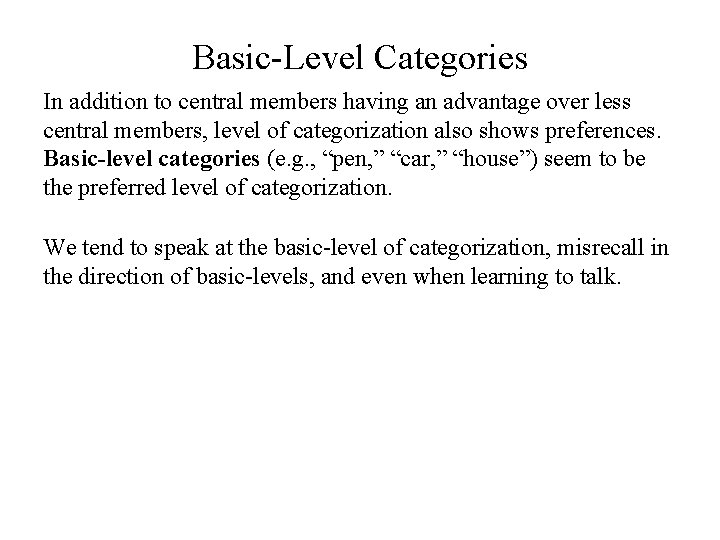
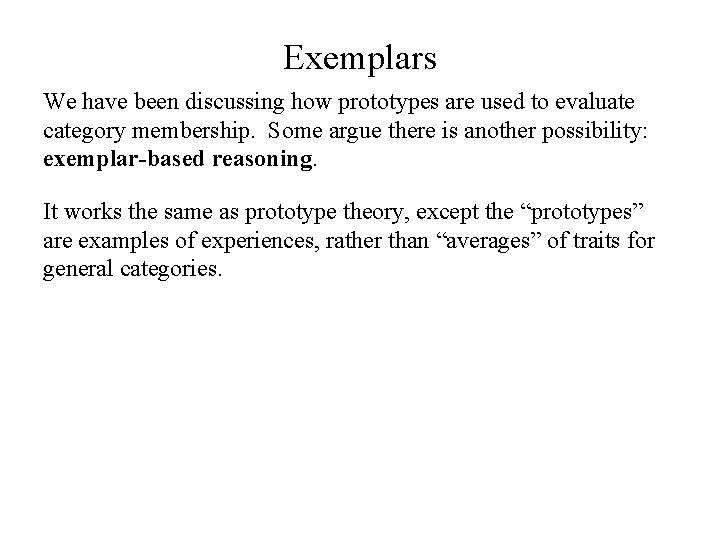
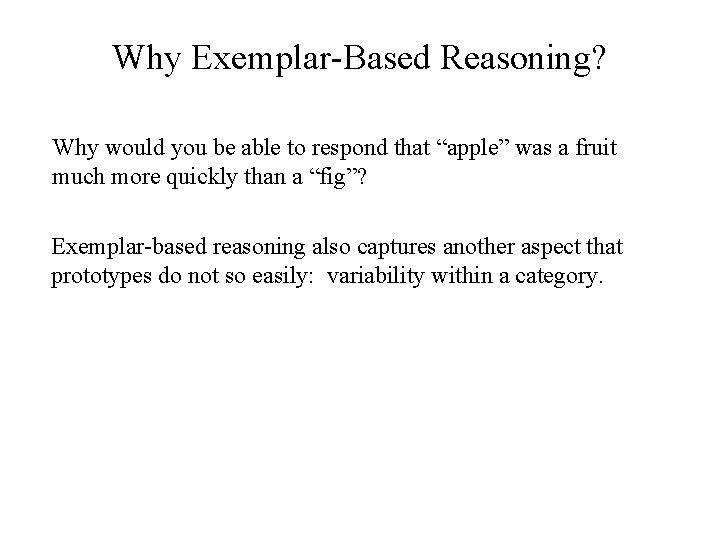
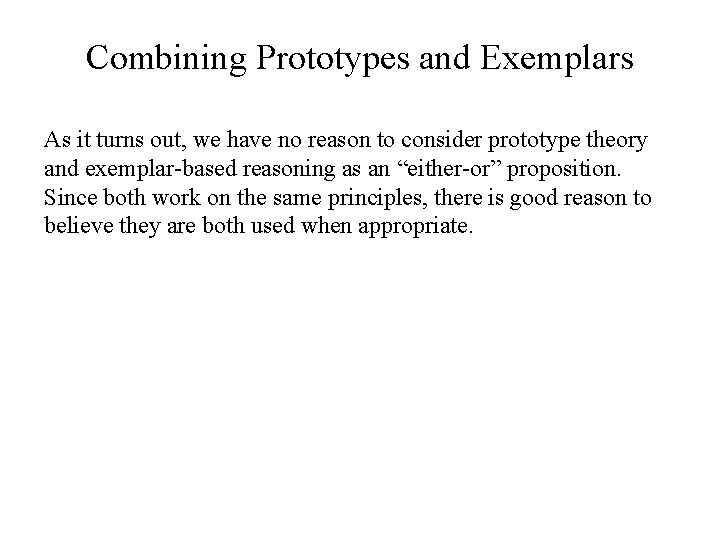
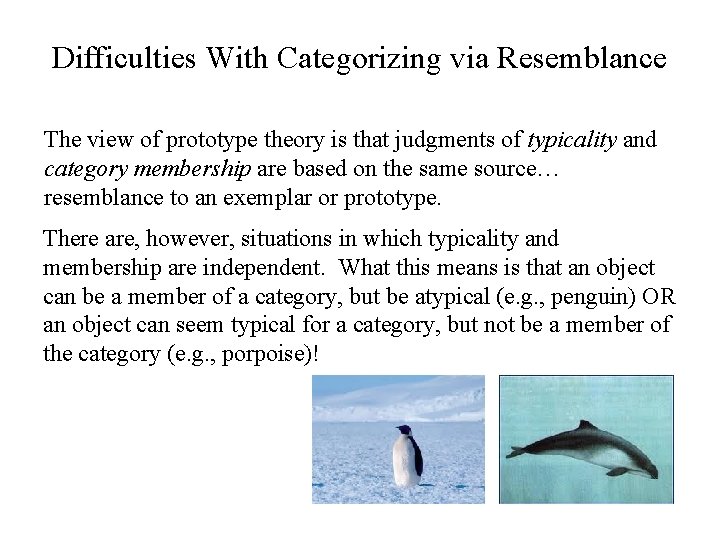
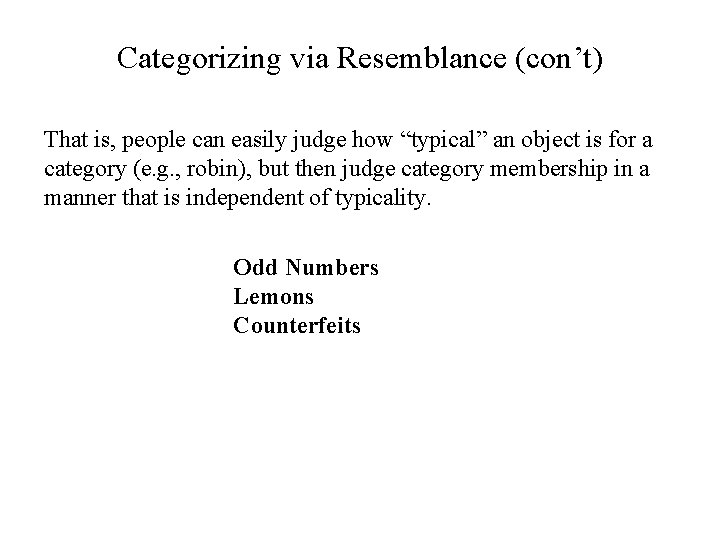
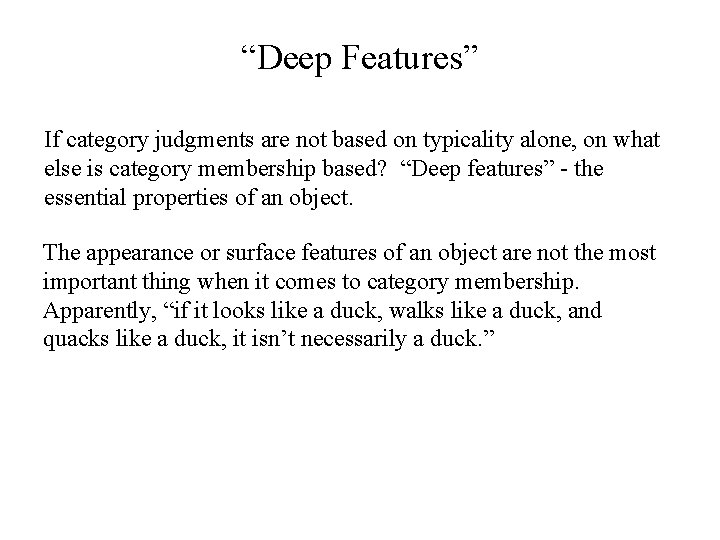
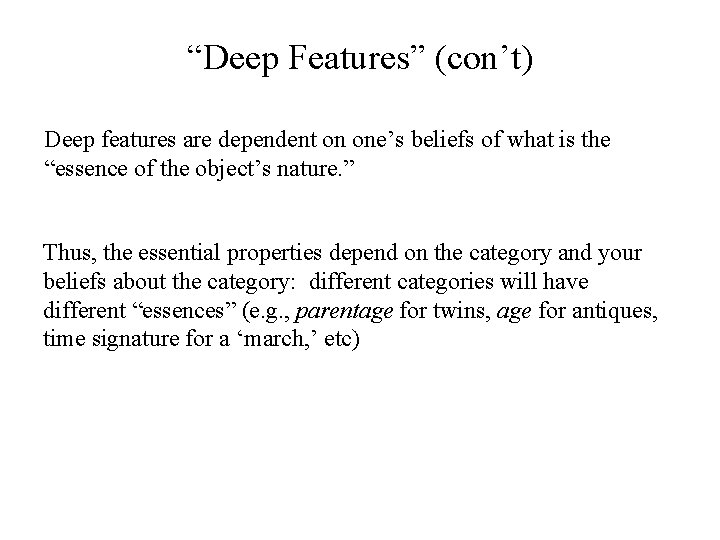
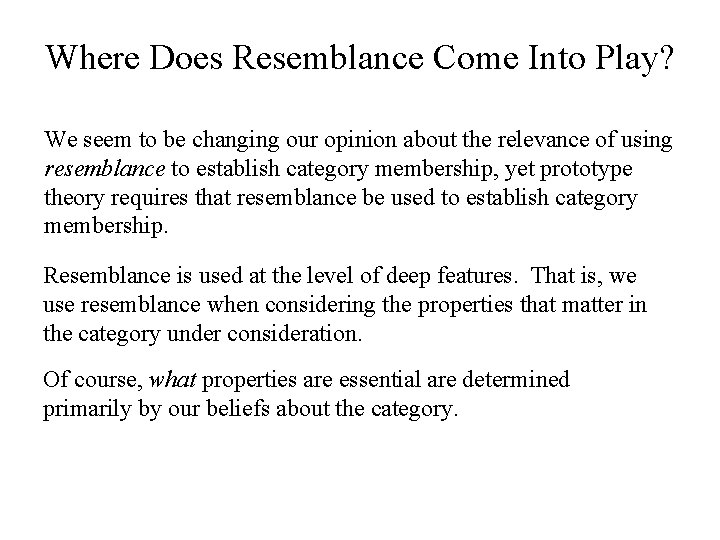
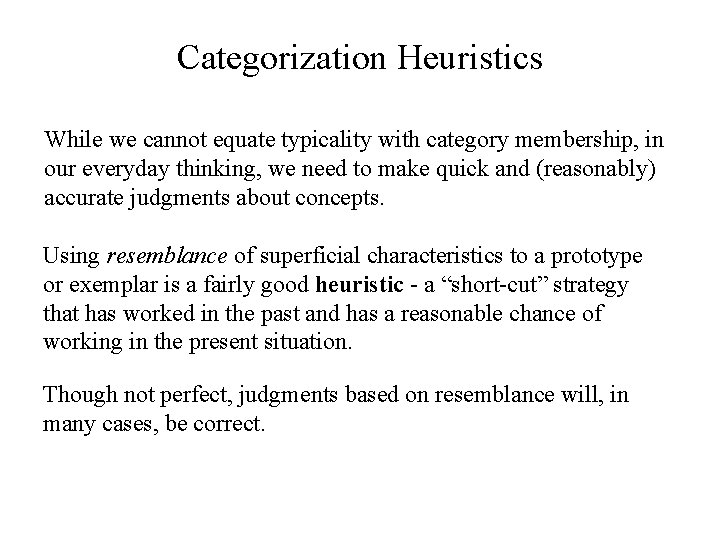
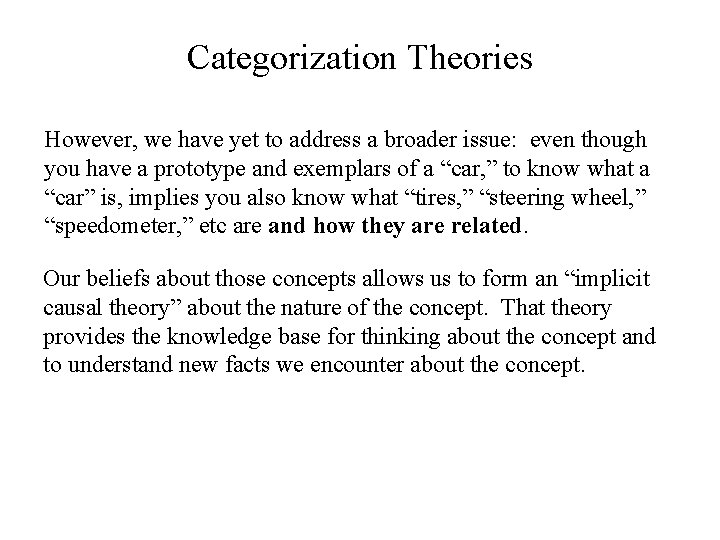
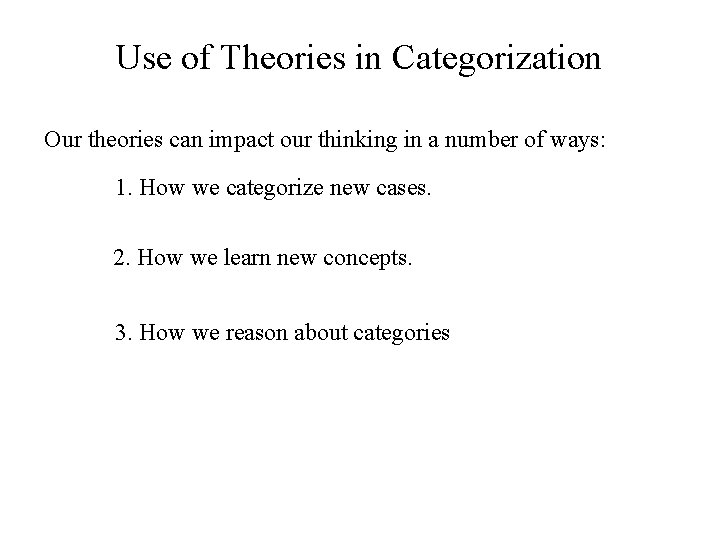
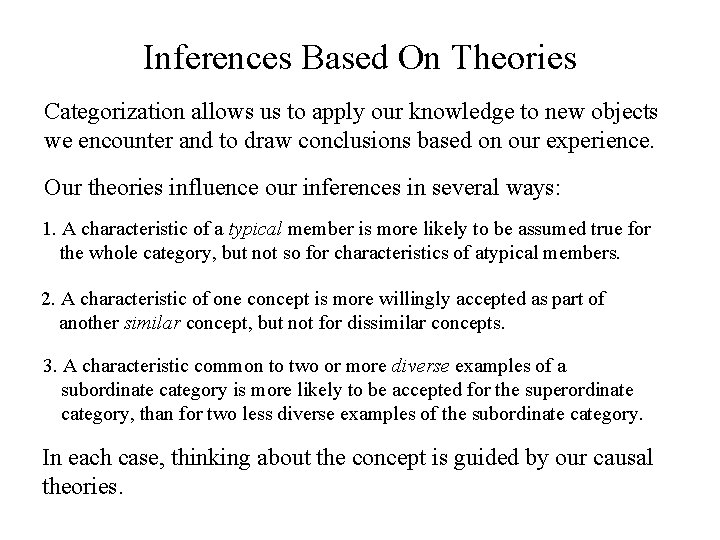
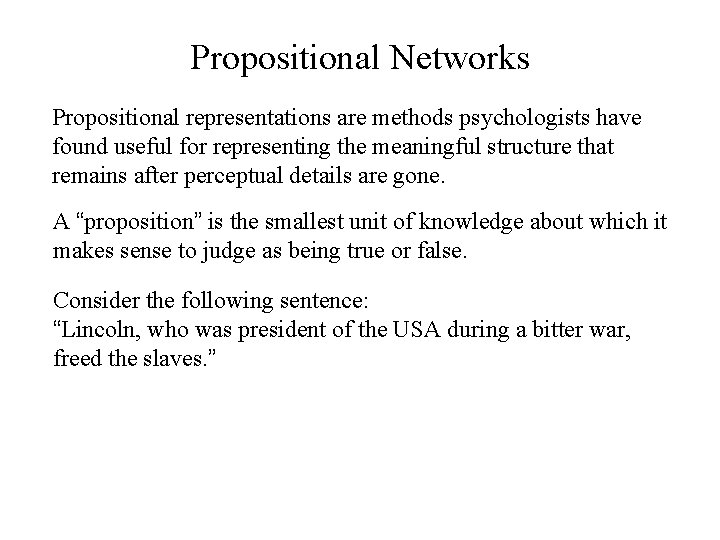
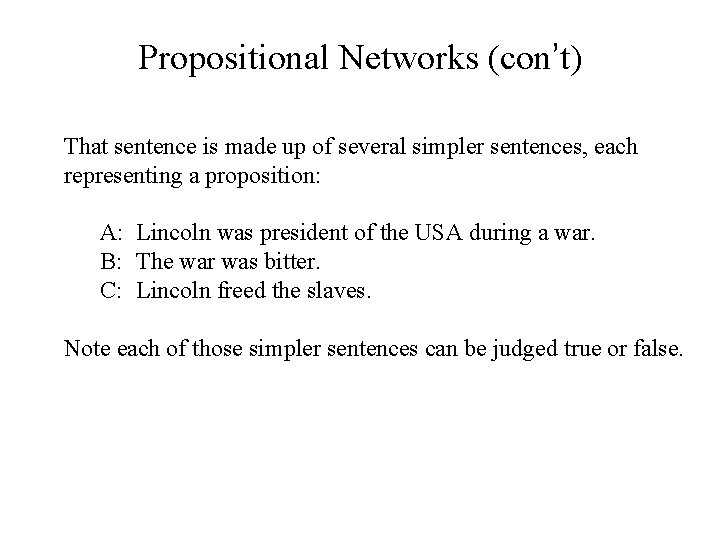
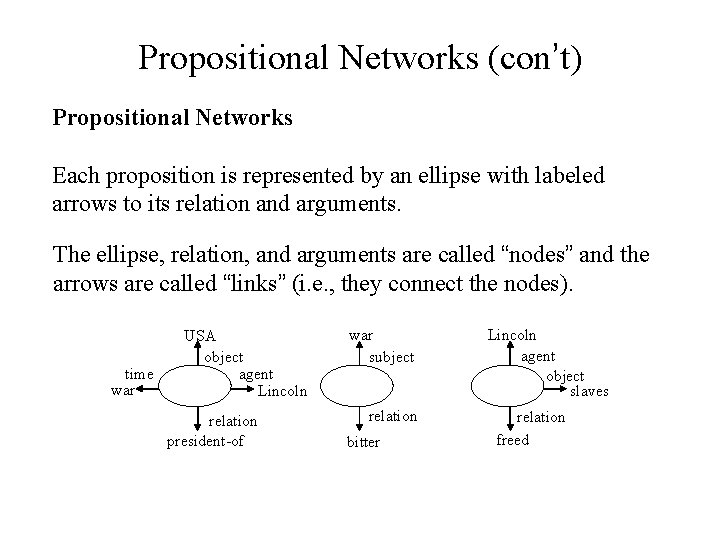
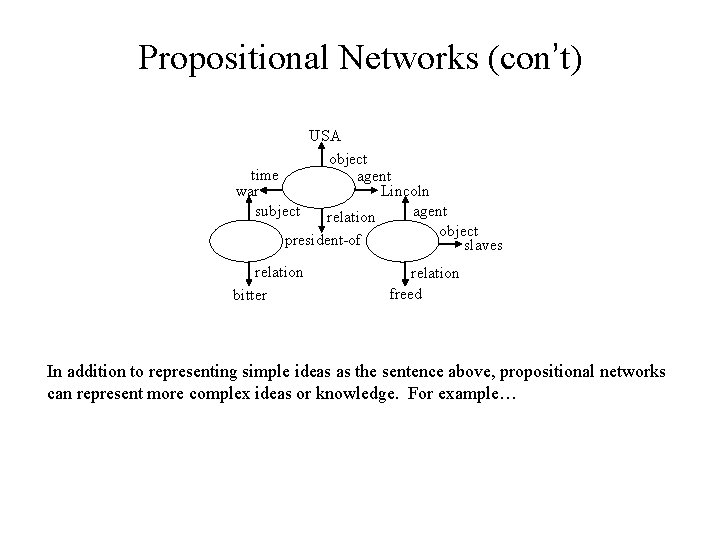
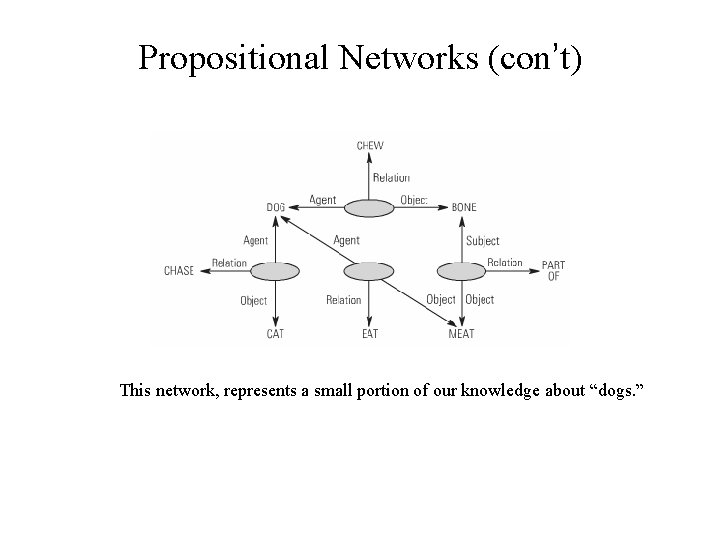
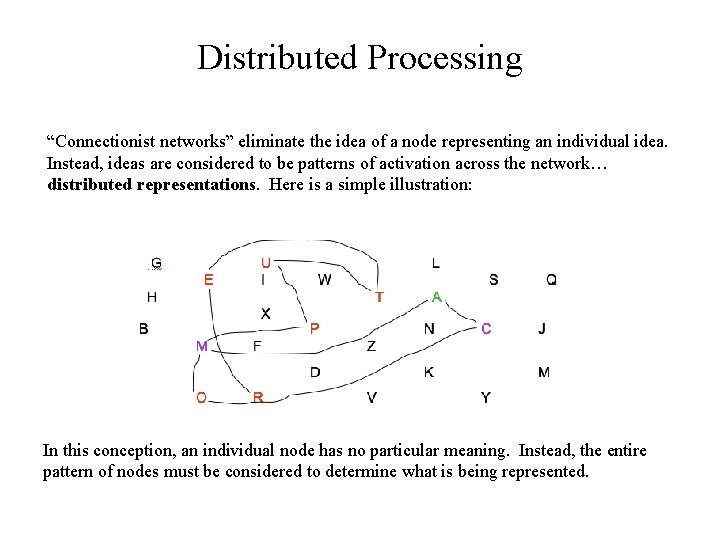
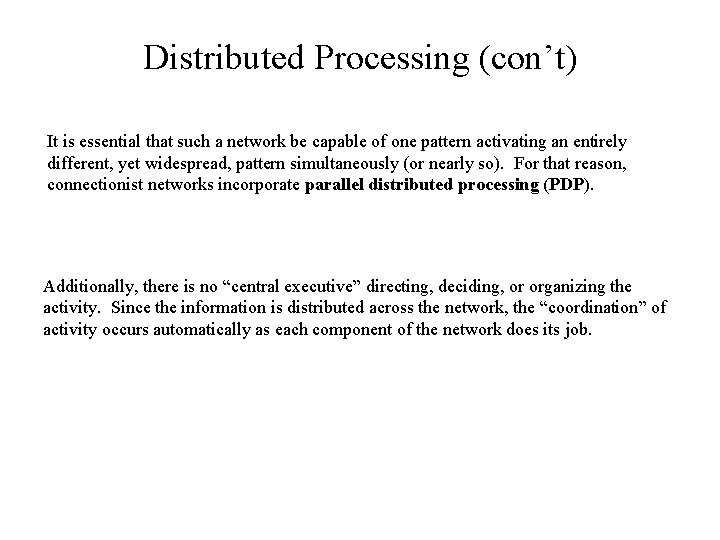
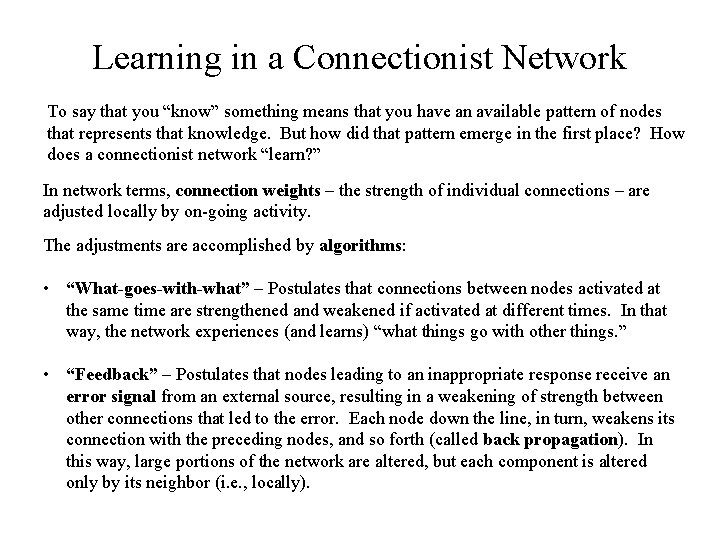
- Slides: 27
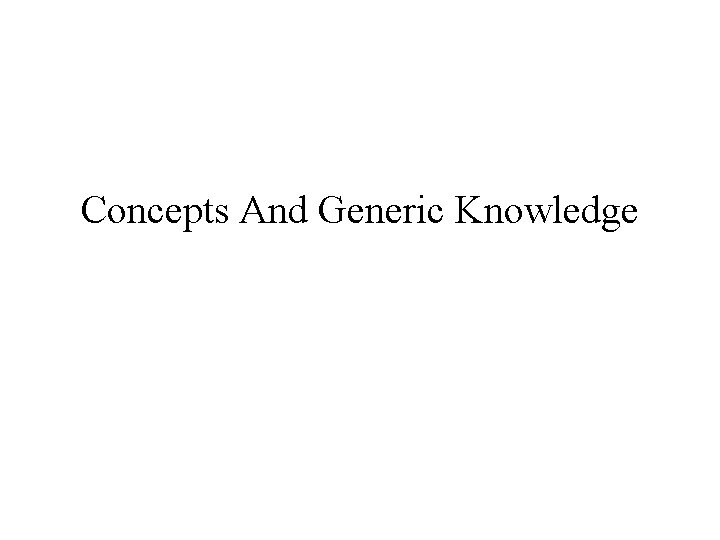
Concepts And Generic Knowledge
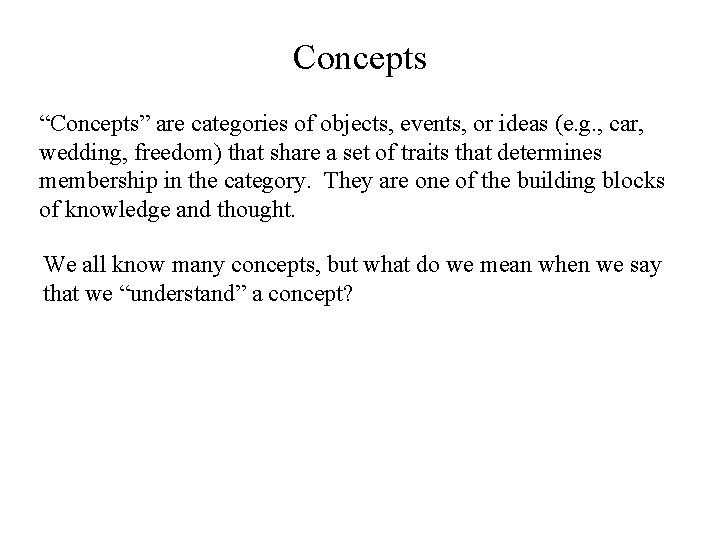
Concepts “Concepts” are categories of objects, events, or ideas (e. g. , car, wedding, freedom) that share a set of traits that determines membership in the category. They are one of the building blocks of knowledge and thought. We all know many concepts, but what do we mean when we say that we “understand” a concept?
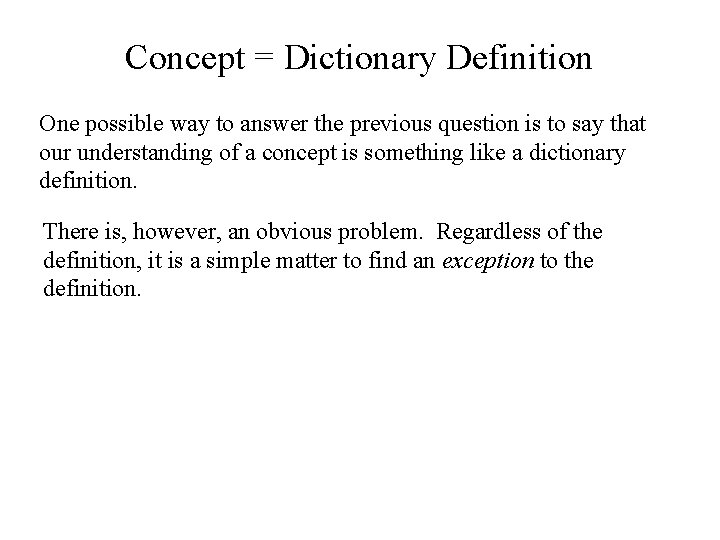
Concept = Dictionary Definition One possible way to answer the previous question is to say that our understanding of a concept is something like a dictionary definition. There is, however, an obvious problem. Regardless of the definition, it is a simple matter to find an exception to the definition.
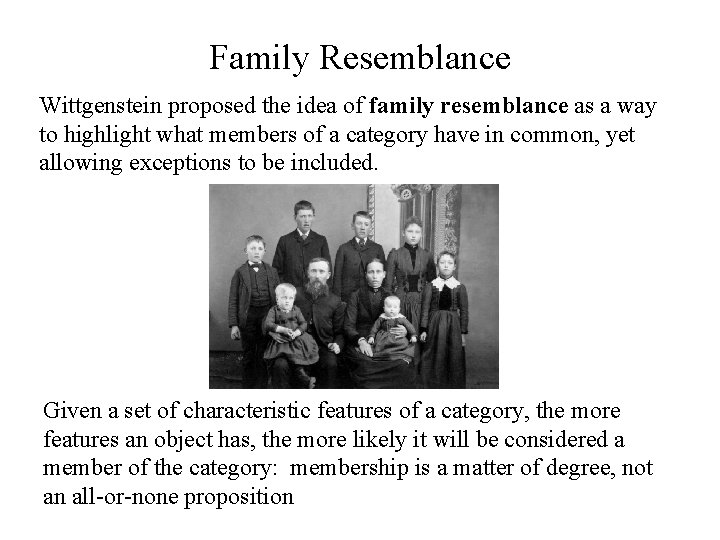
Family Resemblance Wittgenstein proposed the idea of family resemblance as a way to highlight what members of a category have in common, yet allowing exceptions to be included. Given a set of characteristic features of a category, the more features an object has, the more likely it will be considered a member of the category: membership is a matter of degree, not an all-or-none proposition
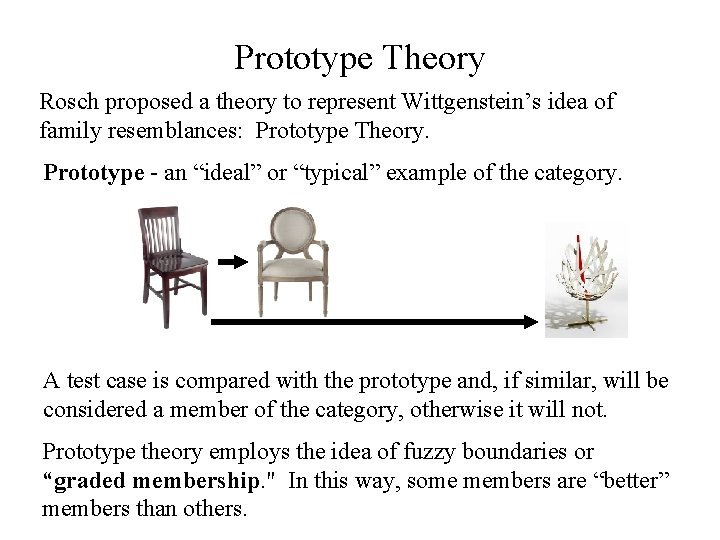
Prototype Theory Rosch proposed a theory to represent Wittgenstein’s idea of family resemblances: Prototype Theory. Prototype - an “ideal” or “typical” example of the category. A test case is compared with the prototype and, if similar, will be considered a member of the category, otherwise it will not. Prototype theory employs the idea of fuzzy boundaries or “graded membership. " In this way, some members are “better” members than others.
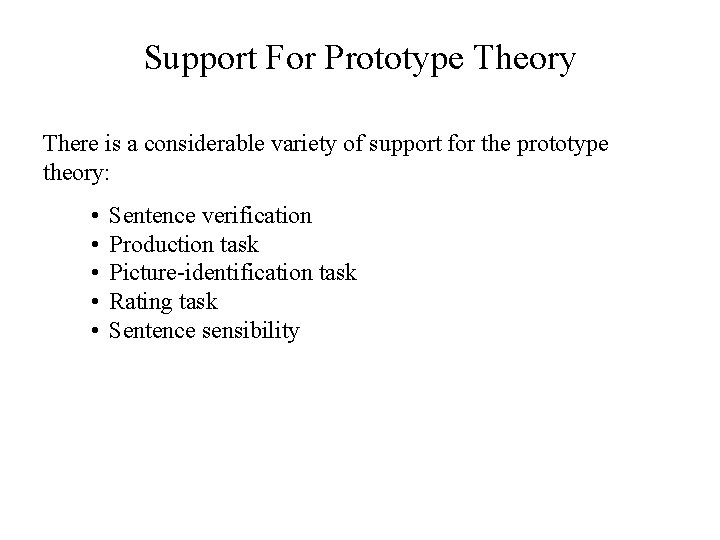
Support For Prototype Theory There is a considerable variety of support for the prototype theory: • • • Sentence verification Production task Picture-identification task Rating task Sentence sensibility
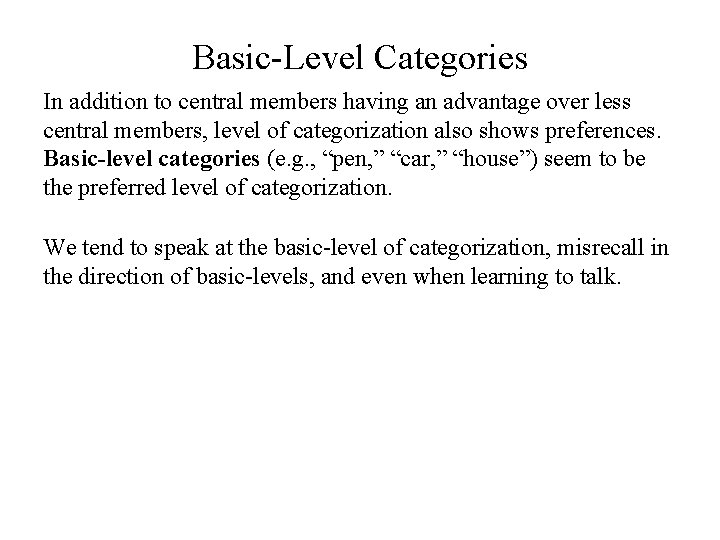
Basic-Level Categories In addition to central members having an advantage over less central members, level of categorization also shows preferences. Basic-level categories (e. g. , “pen, ” “car, ” “house”) seem to be the preferred level of categorization. We tend to speak at the basic-level of categorization, misrecall in the direction of basic-levels, and even when learning to talk.
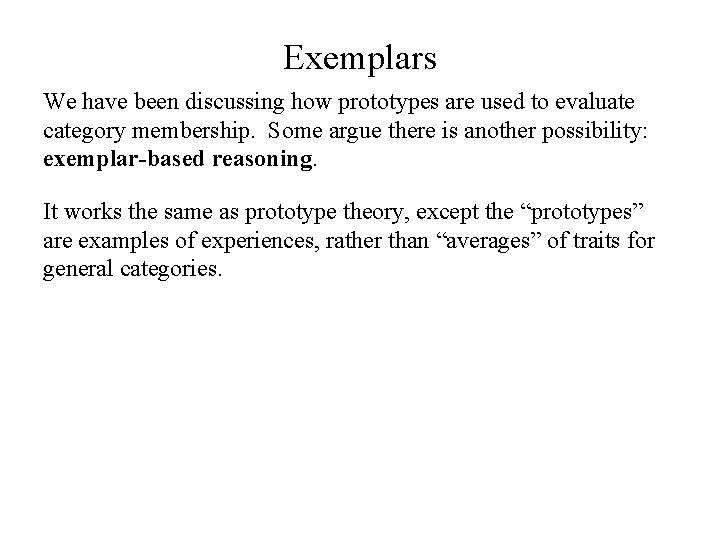
Exemplars We have been discussing how prototypes are used to evaluate category membership. Some argue there is another possibility: exemplar-based reasoning. It works the same as prototype theory, except the “prototypes” are examples of experiences, rather than “averages” of traits for general categories.
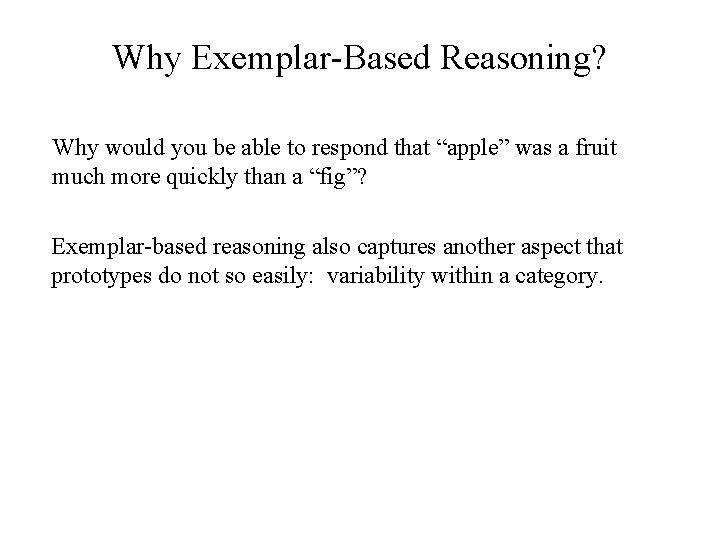
Why Exemplar-Based Reasoning? Why would you be able to respond that “apple” was a fruit much more quickly than a “fig”? Exemplar-based reasoning also captures another aspect that prototypes do not so easily: variability within a category.
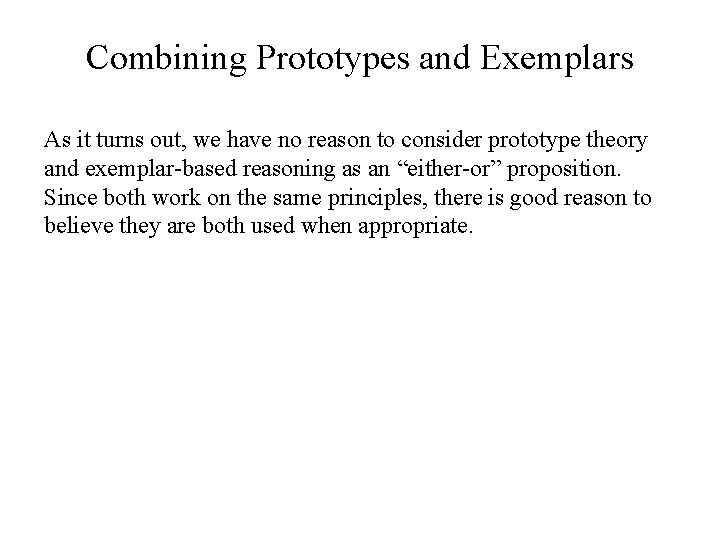
Combining Prototypes and Exemplars As it turns out, we have no reason to consider prototype theory and exemplar-based reasoning as an “either-or” proposition. Since both work on the same principles, there is good reason to believe they are both used when appropriate.
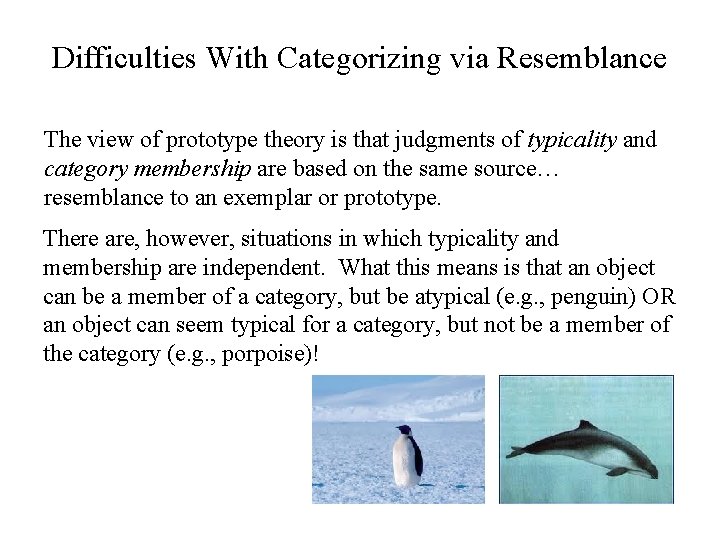
Difficulties With Categorizing via Resemblance The view of prototype theory is that judgments of typicality and category membership are based on the same source… resemblance to an exemplar or prototype. There are, however, situations in which typicality and membership are independent. What this means is that an object can be a member of a category, but be atypical (e. g. , penguin) OR an object can seem typical for a category, but not be a member of the category (e. g. , porpoise)!
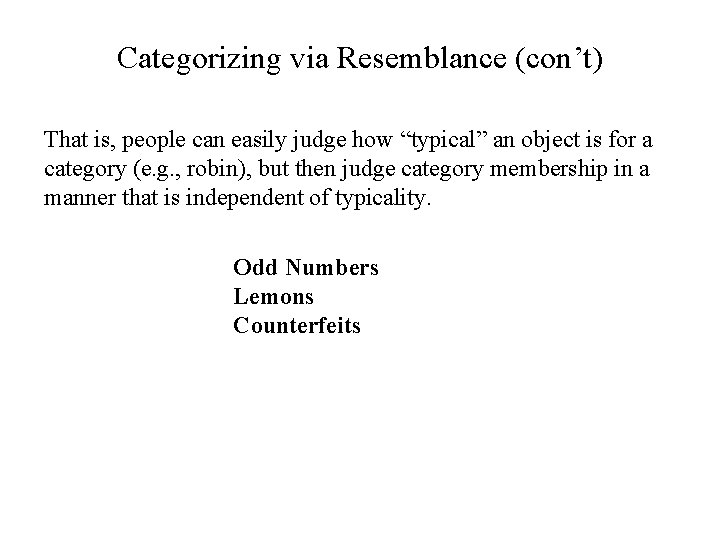
Categorizing via Resemblance (con’t) That is, people can easily judge how “typical” an object is for a category (e. g. , robin), but then judge category membership in a manner that is independent of typicality. Odd Numbers Lemons Counterfeits
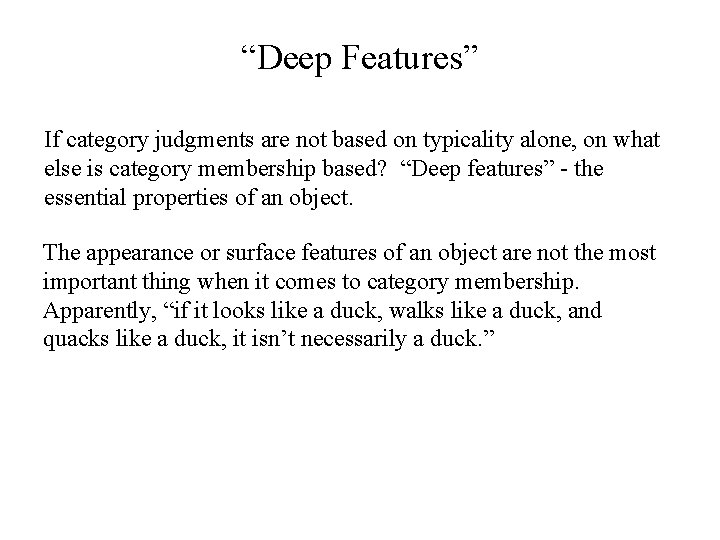
“Deep Features” If category judgments are not based on typicality alone, on what else is category membership based? “Deep features” - the essential properties of an object. The appearance or surface features of an object are not the most important thing when it comes to category membership. Apparently, “if it looks like a duck, walks like a duck, and quacks like a duck, it isn’t necessarily a duck. ”
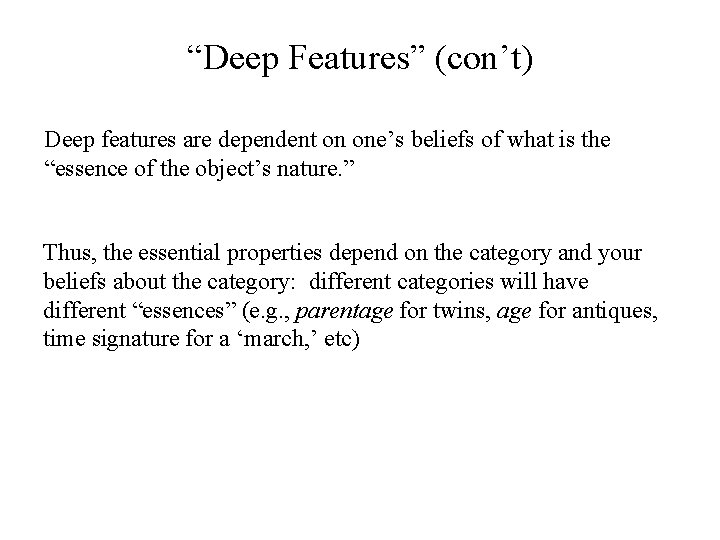
“Deep Features” (con’t) Deep features are dependent on one’s beliefs of what is the “essence of the object’s nature. ” Thus, the essential properties depend on the category and your beliefs about the category: different categories will have different “essences” (e. g. , parentage for twins, age for antiques, time signature for a ‘march, ’ etc)
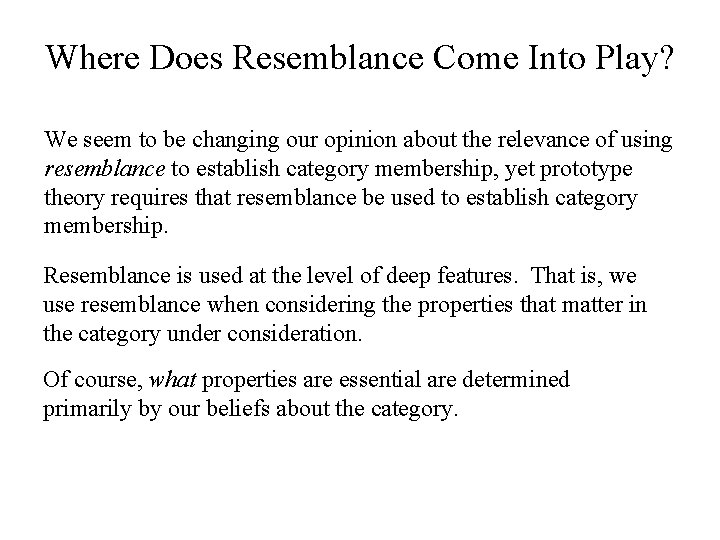
Where Does Resemblance Come Into Play? We seem to be changing our opinion about the relevance of using resemblance to establish category membership, yet prototype theory requires that resemblance be used to establish category membership. Resemblance is used at the level of deep features. That is, we use resemblance when considering the properties that matter in the category under consideration. Of course, what properties are essential are determined primarily by our beliefs about the category.
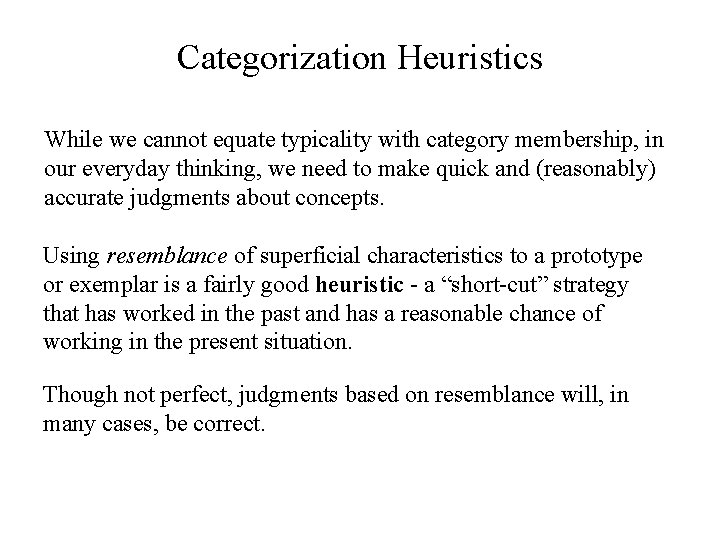
Categorization Heuristics While we cannot equate typicality with category membership, in our everyday thinking, we need to make quick and (reasonably) accurate judgments about concepts. Using resemblance of superficial characteristics to a prototype or exemplar is a fairly good heuristic - a “short-cut” strategy that has worked in the past and has a reasonable chance of working in the present situation. Though not perfect, judgments based on resemblance will, in many cases, be correct.
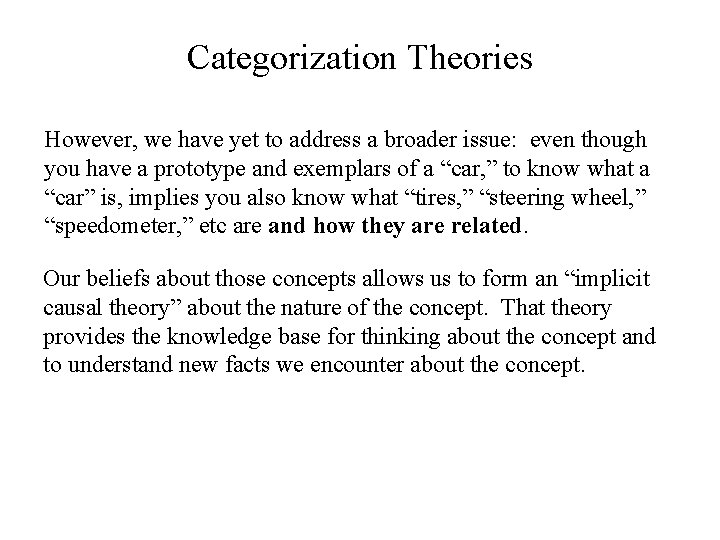
Categorization Theories However, we have yet to address a broader issue: even though you have a prototype and exemplars of a “car, ” to know what a “car” is, implies you also know what “tires, ” “steering wheel, ” “speedometer, ” etc are and how they are related. Our beliefs about those concepts allows us to form an “implicit causal theory” about the nature of the concept. That theory provides the knowledge base for thinking about the concept and to understand new facts we encounter about the concept.
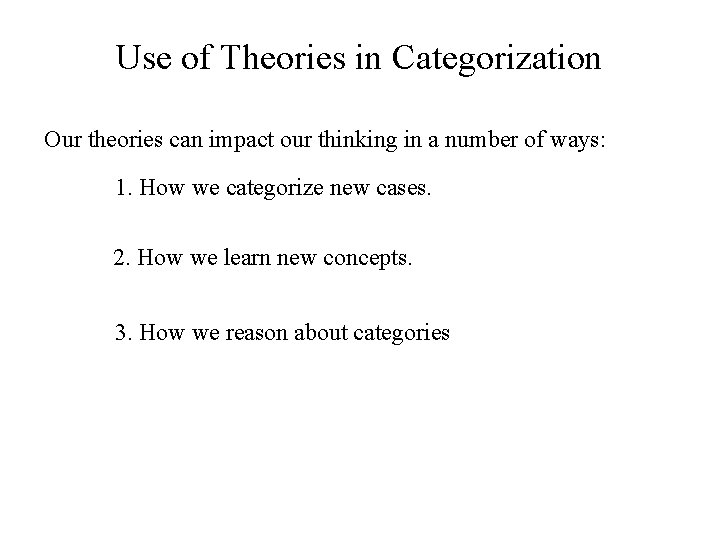
Use of Theories in Categorization Our theories can impact our thinking in a number of ways: 1. How we categorize new cases. 2. How we learn new concepts. 3. How we reason about categories
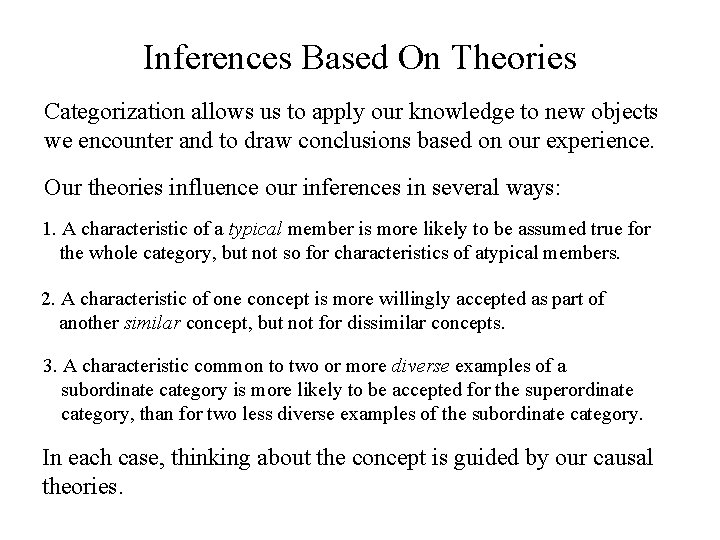
Inferences Based On Theories Categorization allows us to apply our knowledge to new objects we encounter and to draw conclusions based on our experience. Our theories influence our inferences in several ways: 1. A characteristic of a typical member is more likely to be assumed true for the whole category, but not so for characteristics of atypical members. 2. A characteristic of one concept is more willingly accepted as part of another similar concept, but not for dissimilar concepts. 3. A characteristic common to two or more diverse examples of a subordinate category is more likely to be accepted for the superordinate category, than for two less diverse examples of the subordinate category. In each case, thinking about the concept is guided by our causal theories.
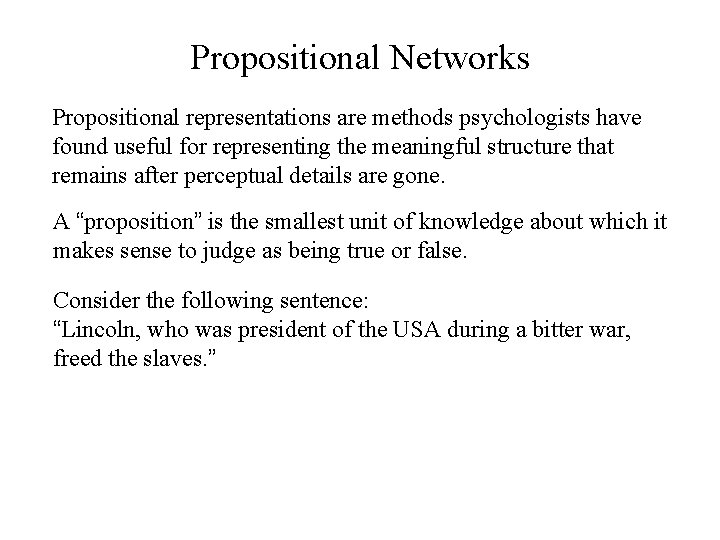
Propositional Networks Propositional representations are methods psychologists have found useful for representing the meaningful structure that remains after perceptual details are gone. A “proposition” is the smallest unit of knowledge about which it makes sense to judge as being true or false. Consider the following sentence: “Lincoln, who was president of the USA during a bitter war, freed the slaves. ”
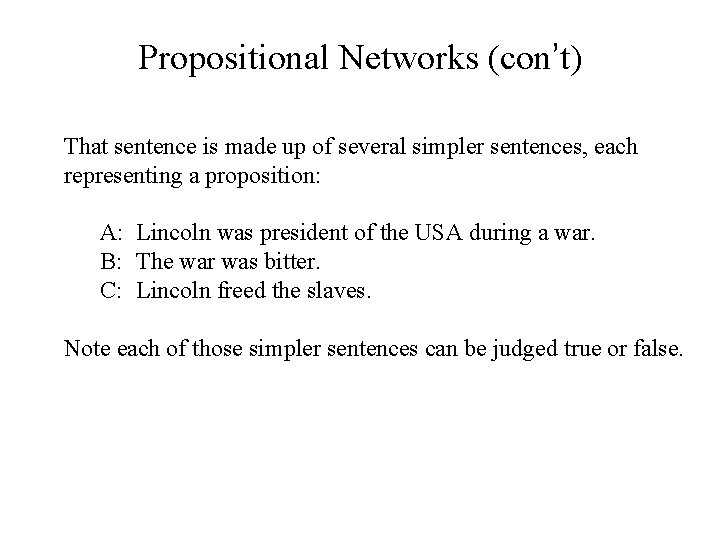
Propositional Networks (con’t) That sentence is made up of several simpler sentences, each representing a proposition: A: Lincoln was president of the USA during a war. B: The war was bitter. C: Lincoln freed the slaves. Note each of those simpler sentences can be judged true or false.
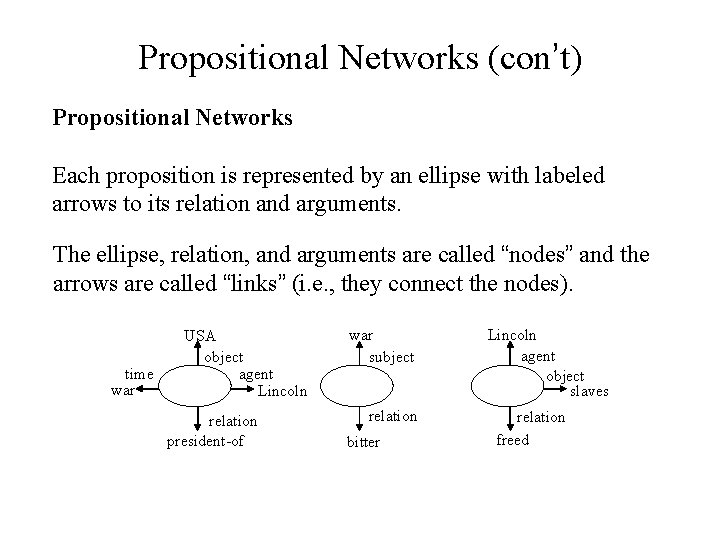
Propositional Networks (con’t) Propositional Networks Each proposition is represented by an ellipse with labeled arrows to its relation and arguments. The ellipse, relation, and arguments are called “nodes” and the arrows are called “links” (i. e. , they connect the nodes). time war USA object agent Lincoln relation president-of war subject relation bitter Lincoln agent object slaves relation freed
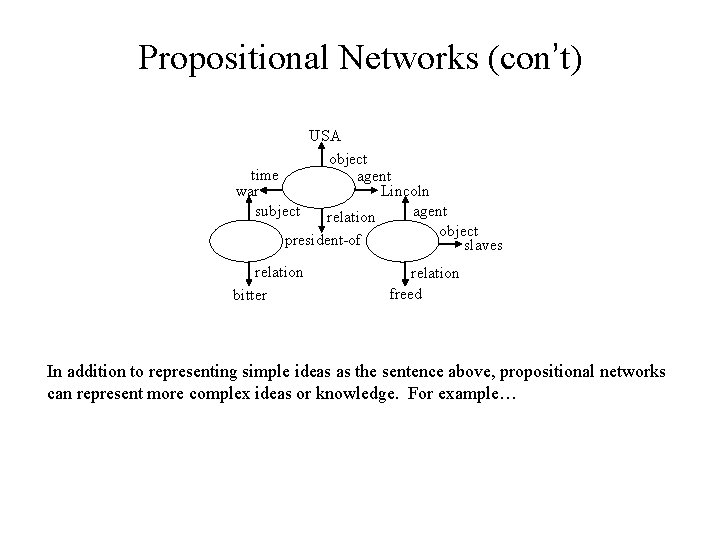
Propositional Networks (con’t) USA object time agent war Lincoln agent subject relation object president-of slaves relation bitter relation freed In addition to representing simple ideas as the sentence above, propositional networks can represent more complex ideas or knowledge. For example…
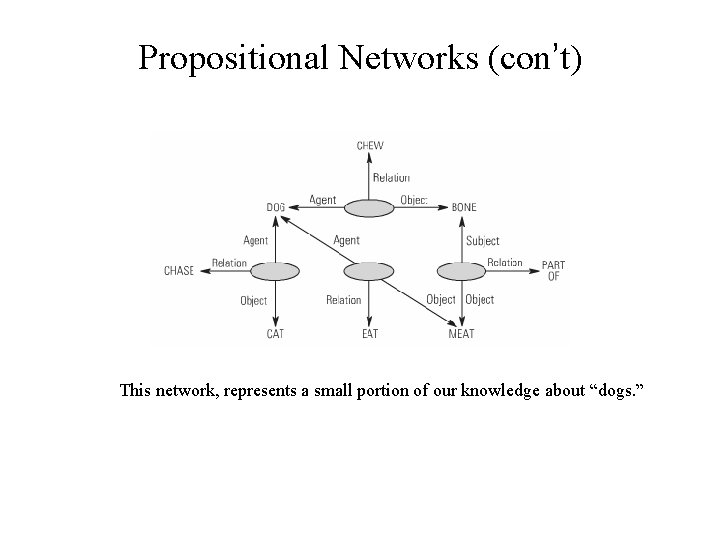
Propositional Networks (con’t) This network, represents a small portion of our knowledge about “dogs. ”
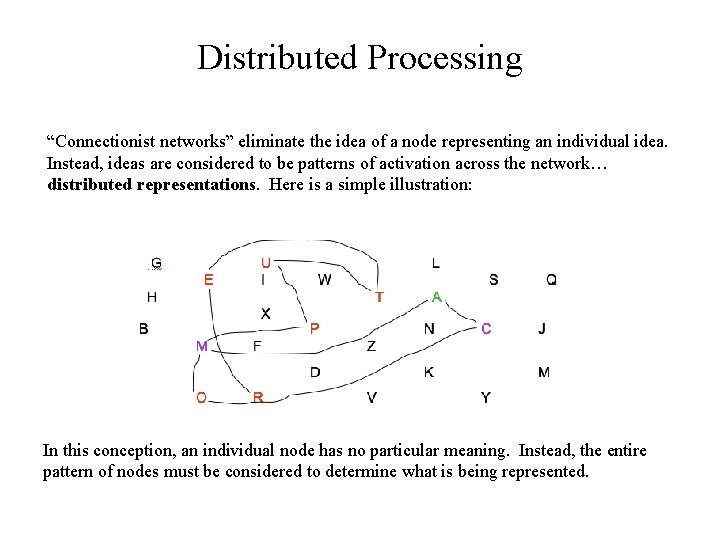
Distributed Processing “Connectionist networks” eliminate the idea of a node representing an individual idea. Instead, ideas are considered to be patterns of activation across the network… distributed representations. Here is a simple illustration: In this conception, an individual node has no particular meaning. Instead, the entire pattern of nodes must be considered to determine what is being represented.
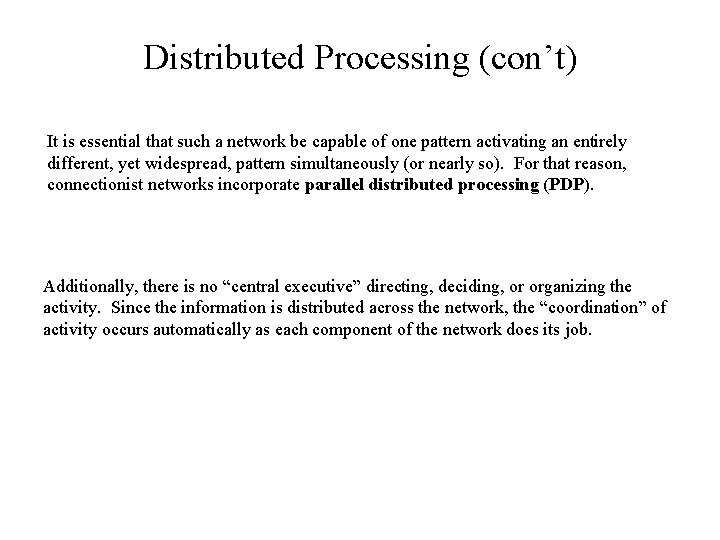
Distributed Processing (con’t) It is essential that such a network be capable of one pattern activating an entirely different, yet widespread, pattern simultaneously (or nearly so). For that reason, connectionist networks incorporate parallel distributed processing (PDP). Additionally, there is no “central executive” directing, deciding, or organizing the activity. Since the information is distributed across the network, the “coordination” of activity occurs automatically as each component of the network does its job.
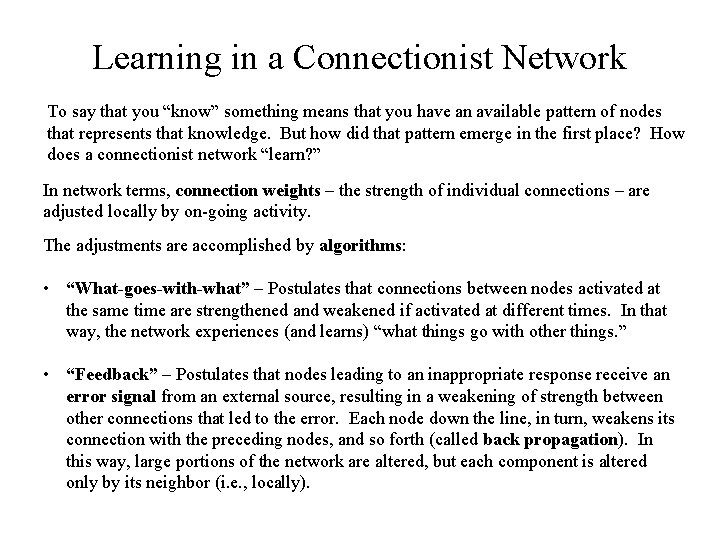
Learning in a Connectionist Network To say that you “know” something means that you have an available pattern of nodes that represents that knowledge. But how did that pattern emerge in the first place? How does a connectionist network “learn? ” In network terms, connection weights – the strength of individual connections – are adjusted locally by on-going activity. The adjustments are accomplished by algorithms: • “What-goes-with-what” – Postulates that connections between nodes activated at the same time are strengthened and weakened if activated at different times. In that way, the network experiences (and learns) “what things go with other things. ” • “Feedback” – Postulates that nodes leading to an inappropriate response receive an error signal from an external source, resulting in a weakening of strength between other connections that led to the error. Each node down the line, in turn, weakens its connection with the preceding nodes, and so forth (called back propagation). In this way, large portions of the network are altered, but each component is altered only by its neighbor (i. e. , locally).