Climate Change Impacts On Rice Farming Systems in
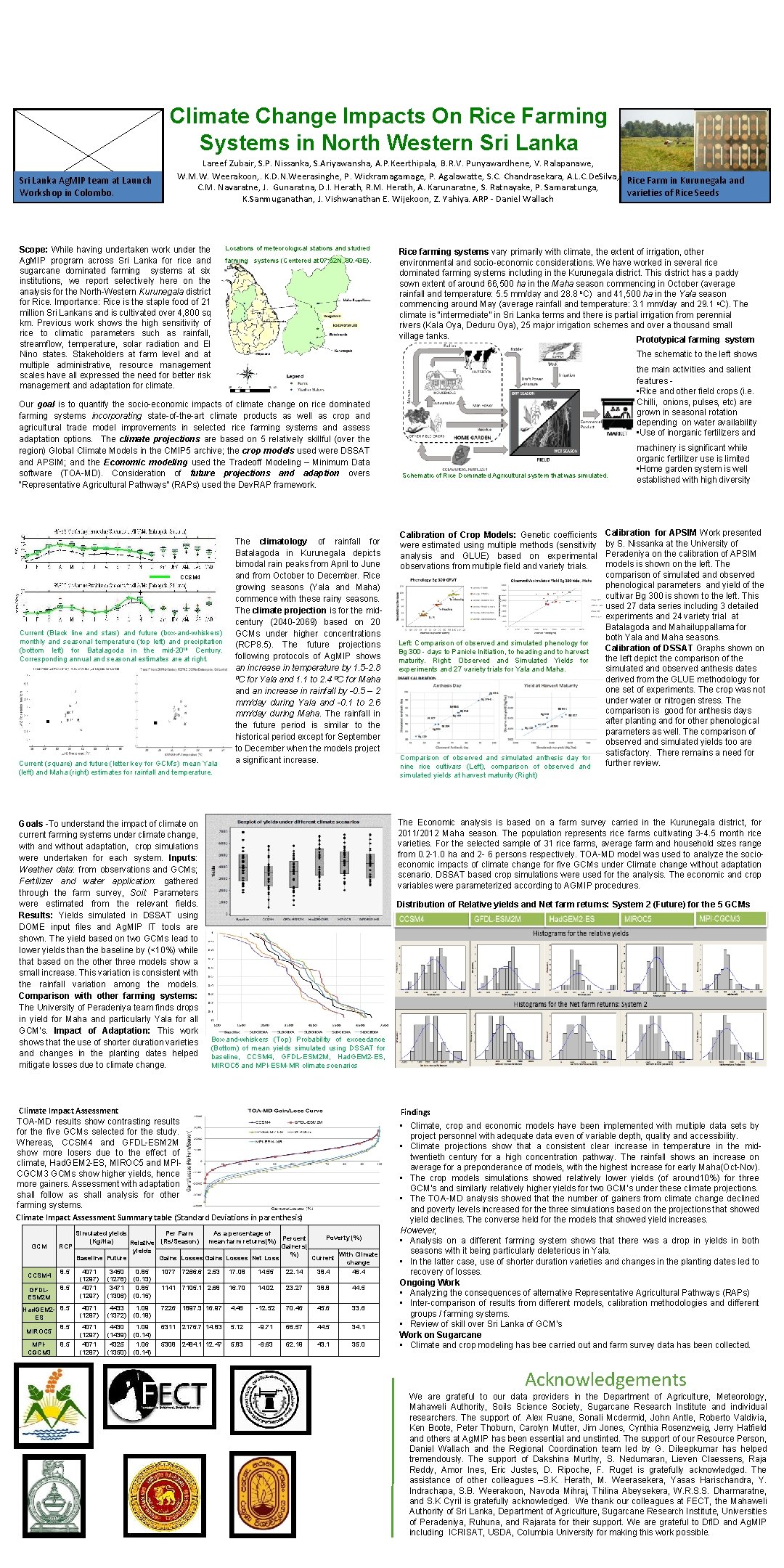
- Slides: 1
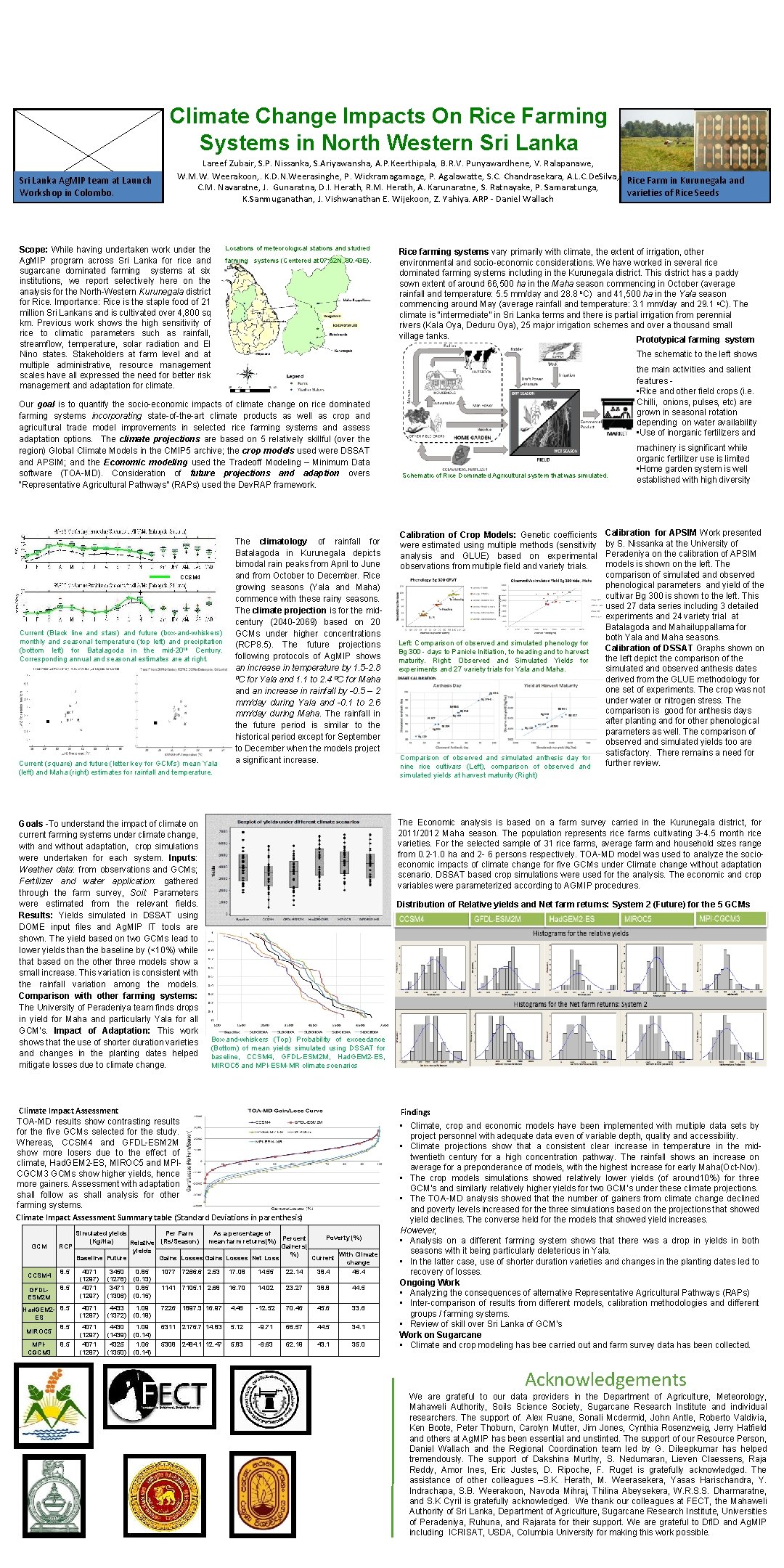
Climate Change Impacts On Rice Farming Systems in North Western Sri Lanka Ag. MIP team at Launch Workshop in Colombo. 1. Introduction 2. Farming Systems Scope: While having undertaken work under the Ag. MIP program across Sri Lanka for rice and sugarcane dominated farming systems at six institutions, we report selectively here on the analysis for the North-Western Kurunegala district for Rice. Importance: Rice is the staple food of 21 million Sri Lankans and is cultivated over 4, 800 sq km. Previous work shows the high sensitivity of rice to climatic parameters such as rainfall, streamflow, temperature, solar radiation and El Nino states. Stakeholders at farm level and at multiple administrative, resource management scales have all expressed the need for better risk management and adaptation for climate. Locations of meteorological stations and studied farming systems (Centered at 07. 52 N, 80. 43 E). 3. Climate Analysis Current (Black line and stars) and future (box-and-whiskers) monthly and seasonal temperature (top left) and precipitation (bottom left) for Batalagoda in the mid-20 th Century. Corresponding annual and seasonal estimates are at right. Current (square) and future (letter key for GCM’s) mean Yala (left) and Maha (right) estimates for rainfall and temperature. The climatology of rainfall for Batalagoda in Kurunegala depicts bimodal rain peaks from April to June and from October to December. Rice growing seasons (Yala and Maha) commence with these rainy seasons. The climate projection is for the midcentury (2040 -2069) based on 20 GCMs under higher concentrations (RCP 8. 5). The future projections following protocols of Ag. MIP shows an increase in temperature by 1. 5 -2. 8 ⁰C for Yala and 1. 1 to 2. 4 ⁰C for Maha and an increase in rainfall by -0. 5 – 2 mm/day during Yala and -0. 1 to 2. 6 mm/day during Maha. The rainfall in the future period is similar to the historical period except for September to December when the models project a significant increase. 5. Crop Analysis (Yield Simulations) Goals -To understand the impact of climate on current farming systems under climate change, with and without adaptation, crop simulations were undertaken for each system. Inputs: Weather data: from observations and GCMs; Fertilizer and water application: gathered through the farm survey, Soil: Parameters were estimated from the relevant fields. Results: Yields simulated in DSSAT using DOME input files and Ag. MIP IT tools are shown. The yield based on two GCMs lead to lower yields than the baseline by (<10%) while that based on the other three models show a small increase. This variation is consistent with the rainfall variation among the models. Comparison with other farming systems: The University of Peradeniya team finds drops in yield for Maha and particularly Yala for all GCM’s. Impact of Adaptation: This work shows that the use of shorter duration varieties and changes in the planting dates helped mitigate losses due to climate change. Schematic of Rice Dominated Agricultural system that was simulated. machinery is significant while organic fertilizer use is limited • Home garden system is well established with high diversity Calibration of Crop Models: Genetic coefficients were estimated using multiple methods (sensitivity analysis and GLUE) based on experimental observations from multiple field and variety trials. Left: Comparison of observed and simulated phenology for Bg 300 - days to Panicle Initiation, to heading and to harvest maturity. Right: Observed and Simulated Yields for experiments and 27 variety trials for Yala and Maha. Comparison of observed and simulated anthesis day for nine rice cultivars (Left), comparison of observed and simulated yields at harvest maturity (Right) Calibration for APSIM Work presented by S. Nissanka at the University of Peradeniya on the calibration of APSIM models is shown on the left. The comparison of simulated and observed phenological parameters and yield of the cultivar Bg 300 is shown to the left. This used 27 data series including 3 detailed experiments and 24 variety trial at Batalagoda and Mahailuppallama for both Yala and Maha seasons. Calibration of DSSAT Graphs shown on the left depict the comparison of the simulated and observed anthesis dates derived from the GLUE methodology for one set of experiments. The crop was not under water or nitrogen stress. The comparison is good for anthesis days after planting and for other phenological parameters as well. The comparison of observed and simulated yields too are satisfactory. There remains a need for further review. 6. Economic Analysis The Economic analysis is based on a farm survey carried in the Kurunegala district, for 2011/2012 Maha season. The population represents rice farms cultivating 3 -4. 5 month rice varieties. For the selected sample of 31 rice farms, average farm and household sizes range from 0. 2 -1. 0 ha and 2 - 6 persons respectively. TOA-MD model was used to analyze the socioeconomic impacts of climate change for five GCMs under Climate change without adaptation scenario. DSSAT based crop simulations were used for the analysis. The economic and crop variables were parameterized according to AGMIP procedures. Distribution of Relative yields and Net farm returns: System 2 (Future) for the 5 GCMs Box-and-whiskers (Top) Probability of exceedance (Bottom) of mean yields simulated using DSSAT for baseline, CCSM 4, GFDL-ESM 2 M, Had. GEM 2 -ES, MIROC 5 and MPI-ESM-MR climate scenarios 7. Synthesis Diagrams 8. Conclusions Climate Impact Assessment TOA-MD results show contrasting results for the five GCMs selected for the study. Whereas, CCSM 4 and GFDL-ESM 2 M show more losers due to the effect of climate, Had. GEM 2 -ES, MIROC 5 and MPICGCM 3 GCMs show higher yields, hence more gainers. Assessment with adaptation shall follow as shall analysis for other farming systems. Climate Impact Assessment Summary table (Standard Deviations in parenthesis) Findings Simulated yields Per Farm As a percentage of Poverty (%) Percent (Kg/Ha) Relative (Rs/Season) mean farm returns(%) GCM RCP Gainers( yields With Climate %) Baseline Future Gains Losses Net Loss Current change 8. 5 4071 3450 0. 85 1077 7266. 6 2. 53 17. 08 14. 55 22. 14 38. 4 46. 4 CCSM 4 (1297) (1278) (0. 13) 8. 5 4071 3471 0. 85 1141 7105. 1 2. 68 16. 70 14. 02 23. 27 38. 8 44. 5 GFDL(1297) (1306) (0. 15) ESM 2 M Had. GEM 2 - 8. 5 ES 4071 (1297) 4433 (1372) 1. 09 7226 (0. 19) 1897. 3 16. 97 4. 46 -12. 52 70. 46 45. 6 33. 6 8. 5 4071 (1297) 4430 (1439) 4325 (1350) 1. 09 6311 (0. 14) 1. 06 5308 (0. 14) 2176. 7 14. 83 5. 12 -9. 71 66. 57 44. 5 34. 1 2484. 1 12. 47 5. 83 -6. 63 62. 19 43. 1 35. 0 8. 5 the main activities and salient features • Rice and other field crops (i. e. Chilli, onions, pulses, etc) are grown in seasonal rotation depending on water availability • Use of inorganic fertilizers and 4. Crop Analysis CCSM 4 MPICGCM 3 Rice farming systems vary primarily with climate, the extent of irrigation, other environmental and socio-economic considerations. We have worked in several rice dominated farming systems including in the Kurunegala district. This district has a paddy sown extent of around 66, 500 ha in the Maha season commencing in October (average rainfall and temperature: 5. 5 mm/day and 28. 8 o. C) and 41, 500 ha in the Yala season commencing around May (average rainfall and temperature: 3. 1 mm/day and 29. 1 o. C). The climate is “intermediate” in Sri Lanka terms and there is partial irrigation from perennial rivers (Kala Oya, Deduru Oya), 25 major irrigation schemes and over a thousand small village tanks. Prototypical farming system The schematic to the left shows Our goal is to quantify the socio-economic impacts of climate change on rice dominated farming systems incorporating state-of-the-art climate products as well as crop and agricultural trade model improvements in selected rice farming systems and assess adaptation options. The climate projections are based on 5 relatively skillful (over the region) Global Climate Models in the CMIP 5 archive; the crop models used were DSSAT and APSIM; and the Economic modeling used the Tradeoff Modeling – Minimum Data software (TOA-MD). Consideration of future projections and adaption overs “Representative Agricultural Pathways” (RAPs) used the Dev. RAP framework. MIROC 5 Photo Lareef Zubair, S. P. Nissanka, S. Ariyawansha, A. P. Keerthipala, B. R. V. Punyawardhene, V. Ralapanawe, W. M. W. Weerakoon, . K. D. N. Weerasinghe, P. Wickramage, P. Agalawatte, S. C. Chandrasekara, A. L. C. De. Silva, Rice Farm in Kurunegala and C. M. Navaratne, J. Gunaratna, D. I. Herath, R. M. Herath, A. Karunaratne, S. Ratnayake, P. Samaratunga, varieties of Rice Seeds K. Sanmuganathan, J. Vishwanathan E. Wijekoon, Z. Yahiya. ARP - Daniel Wallach • Climate, crop and economic models have been implemented with multiple data sets by project personnel with adequate data even of variable depth, quality and accessibility. • Climate projections show that a consistent clear increase in temperature in the midtwentieth century for a high concentration pathway. The rainfall shows an increase on average for a preponderance of models, with the highest increase for early Maha(Oct-Nov). • The crop models simulations showed relatively lower yields (of around 10%) for three GCM’s and similarly relatively higher yields for two GCM’s under these climate projections. • The TOA-MD analysis showed that the number of gainers from climate change declined and poverty levels increased for the three simulations based on the projections that showed yield declines. The converse held for the models that showed yield increases. However, • Analysis on a different farming system shows that there was a drop in yields in both seasons with it being particularly deleterious in Yala. • In the latter case, use of shorter duration varieties and changes in the planting dates led to recovery of losses. Ongoing Work • Analyzing the consequences of alternative Representative Agricultural Pathways (RAPs) • Inter-comparison of results from different models, calibration methodologies and different groups / farming systems. • Review of skill over Sri Lanka of GCM's Work on Sugarcane • Climate and crop modeling has bee carried out and farm survey data has been collected. Acknowledgements We are grateful to our data providers in the Department of Agriculture, Meteorology, Mahaweli Authority, Soils Science Society, Sugarcane Research Institute and individual researchers. The support of. Alex Ruane, Sonali Mcdermid, John Antle, Roberto Valdivia, Ken Boote, Peter Thoburn, Carolyn Mutter, Jim Jones, Cynthia Rosenzweig, Jerry Hatfield and others at Ag. MIP has been essential and unstinted. The support of our Resource Person, Daniel Wallach and the Regional Coordination team led by G. Dileepkumar has helped tremendously. The support of Dakshina Murthy, S. Nedumaran, Lieven Claessens, Raja Reddy, Amor Ines, Eric Justes, D. Ripoche, F. Ruget is gratefully acknowledged. The assistance of other colleagues –S. K. Herath, M. Weerasekera, Yasas Harischandra, Y. Indrachapa, S. B. Weerakoon, Navoda Mihraj, Thilina Abeysekera, W. R. S. S. Dharmaratne, and S. K Cyril is gratefully acknowledged. We thank our colleagues at FECT, the Mahaweli Authority of Sri Lanka, Department of Agriculture, Sugarcane Research Institute, Universities of Peradeniya, Ruhuna, and Rajarata for their support. We are grateful to Df. ID and Ag. MIP including ICRISAT, USDA, Columbia University for making this work possible.