APC 2005 Plantwide MPC A Cooperative Decentralized Approach
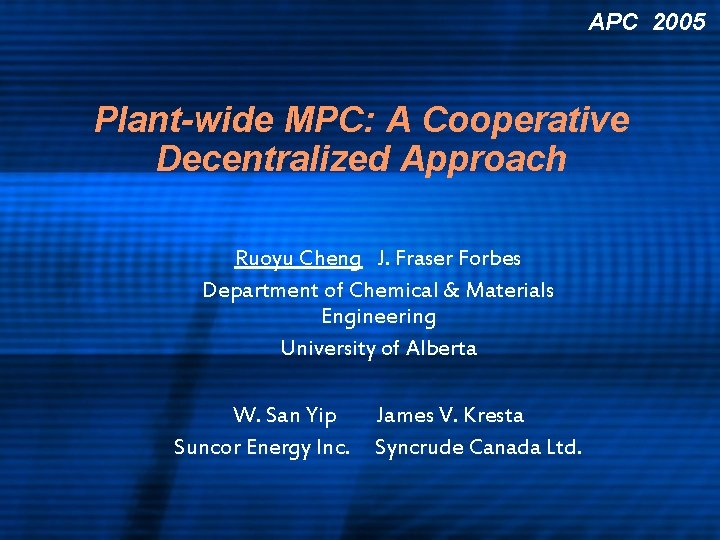
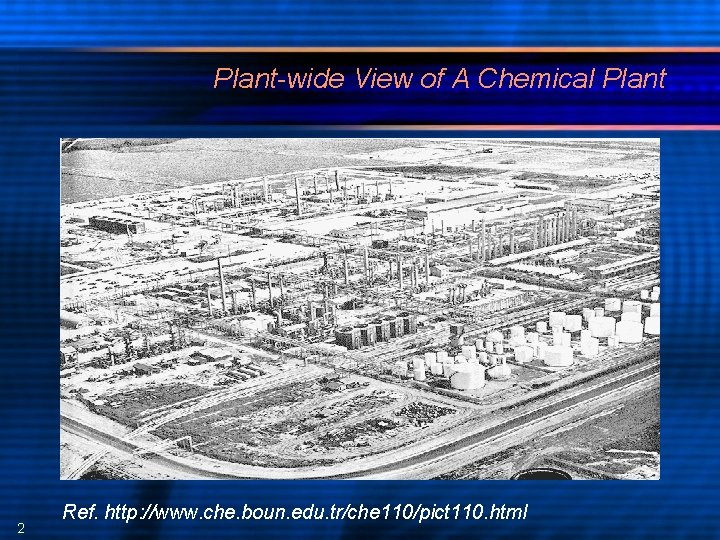
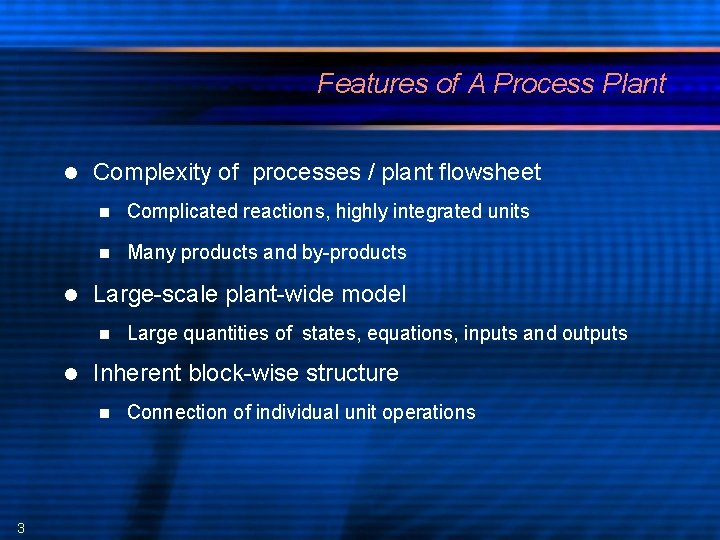
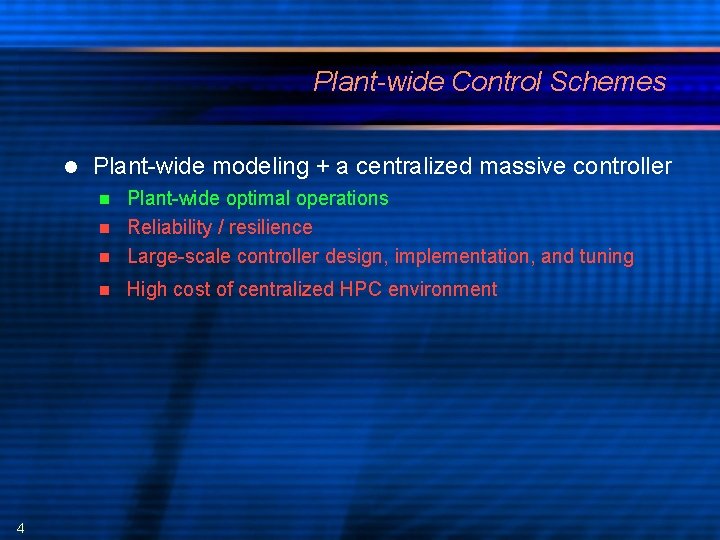
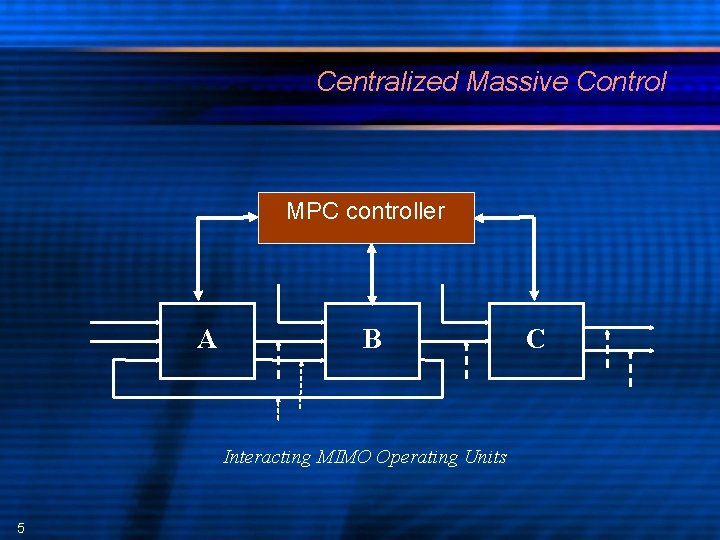
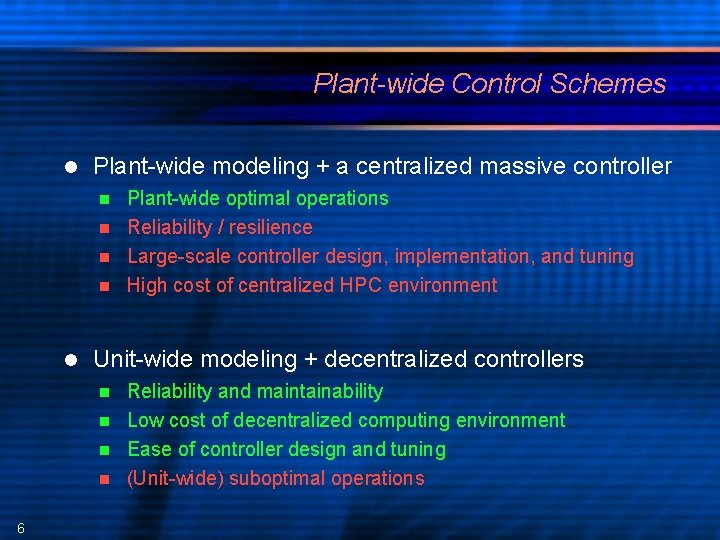
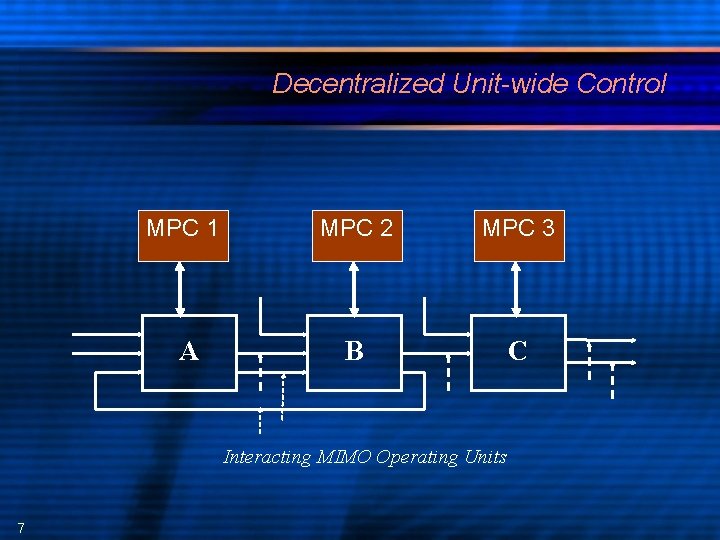
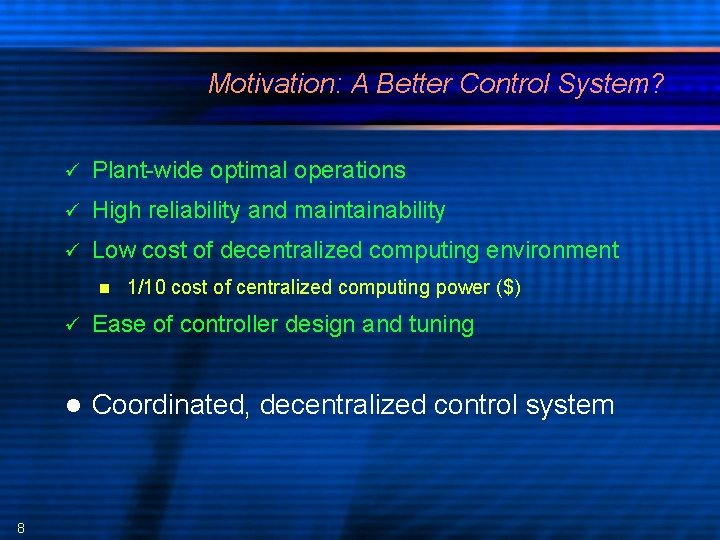
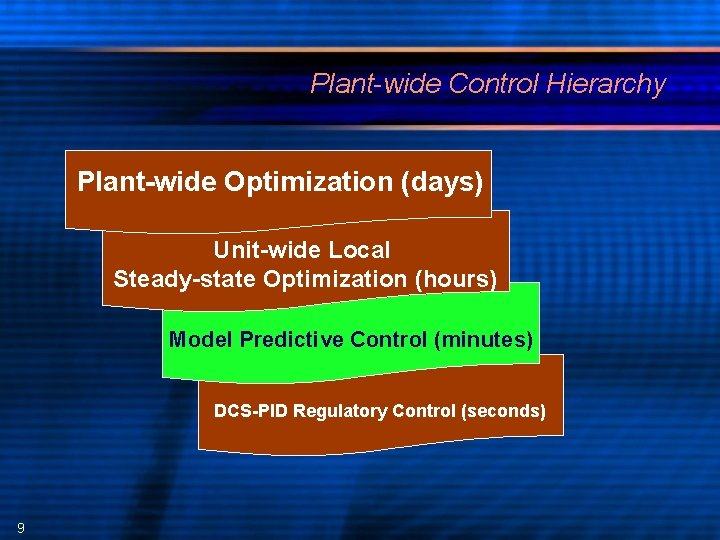
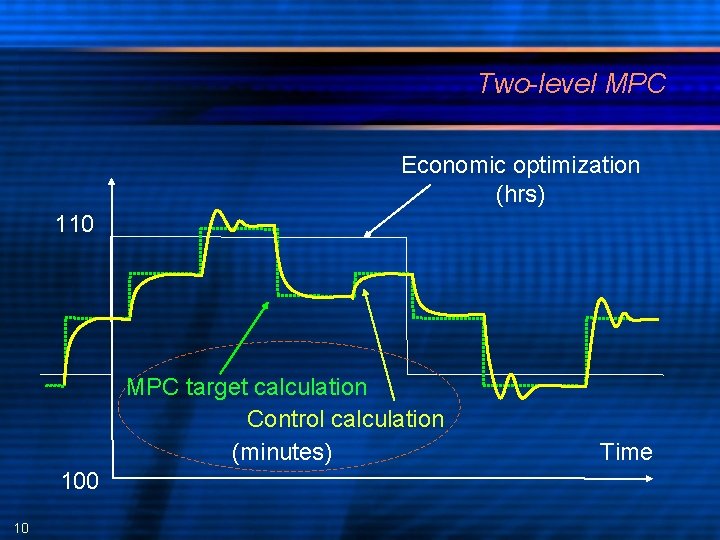
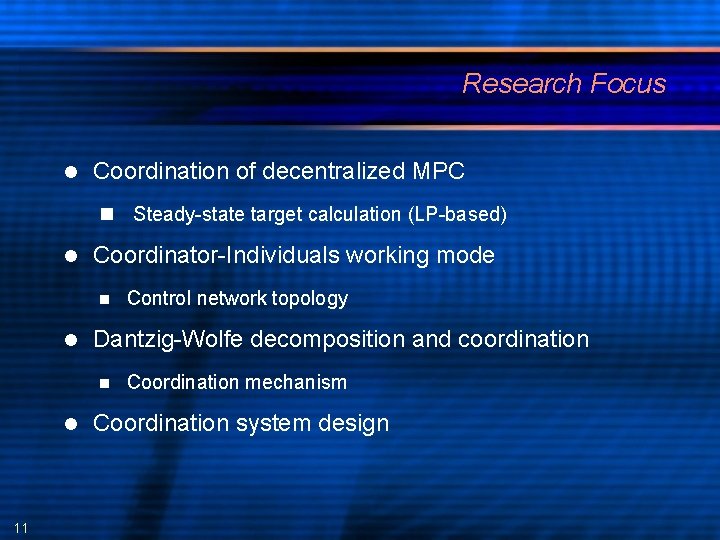
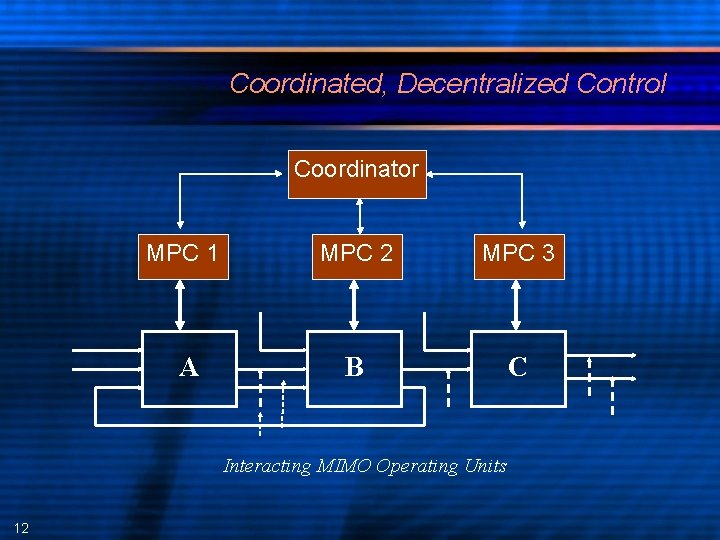
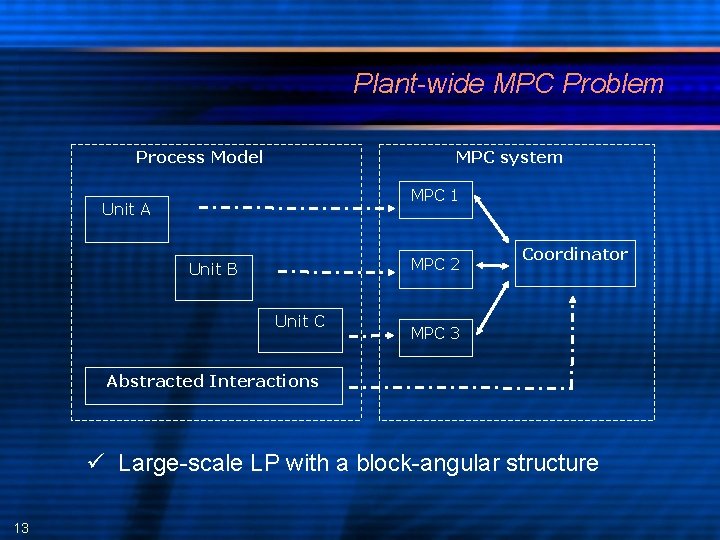
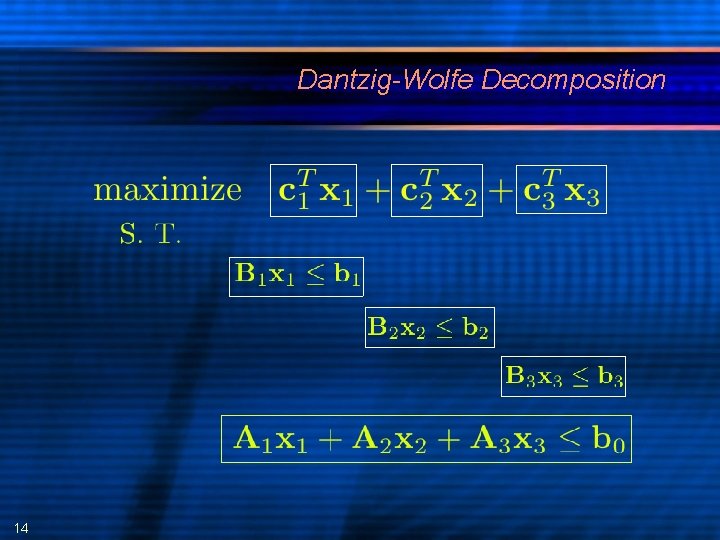
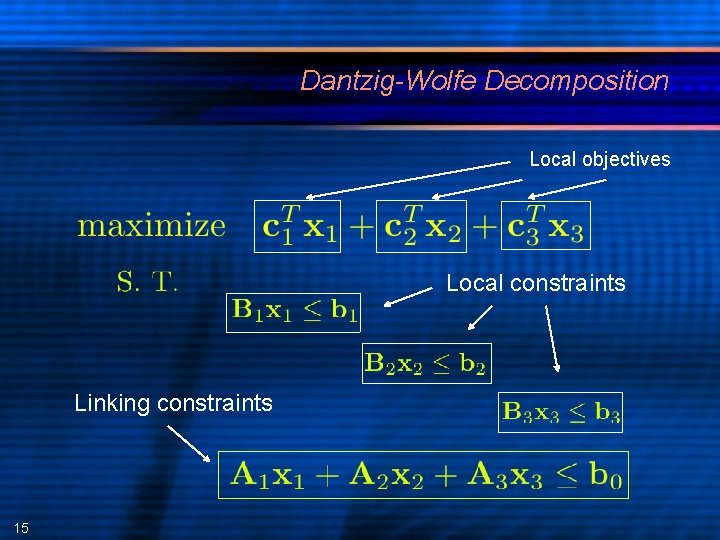
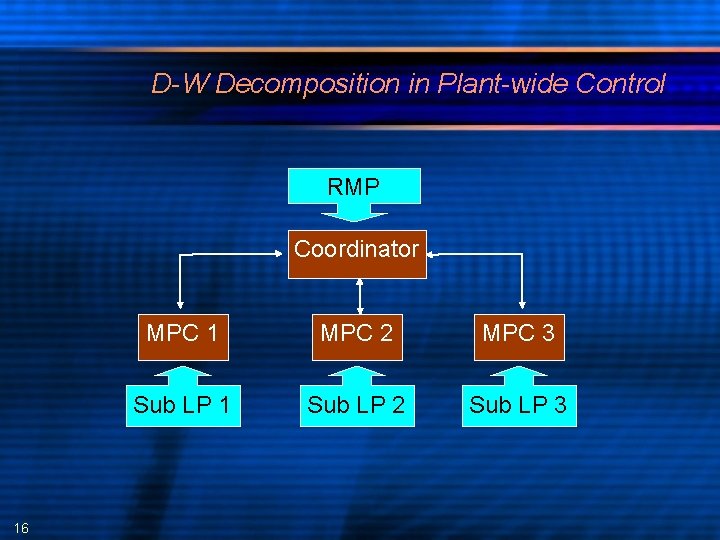
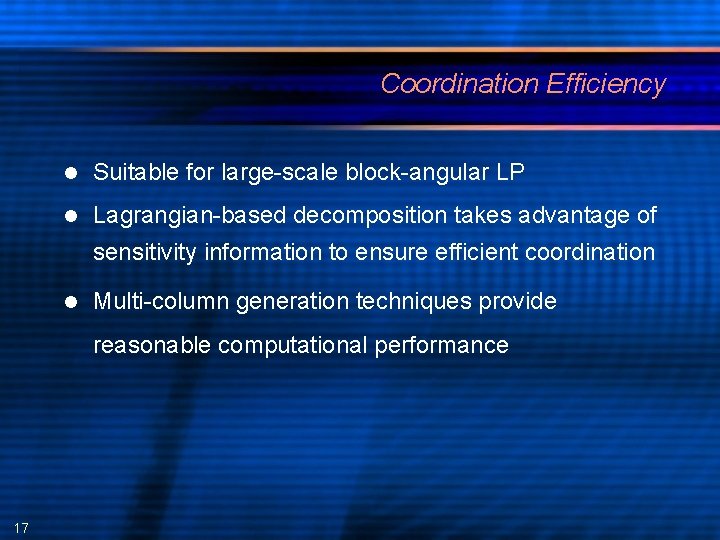
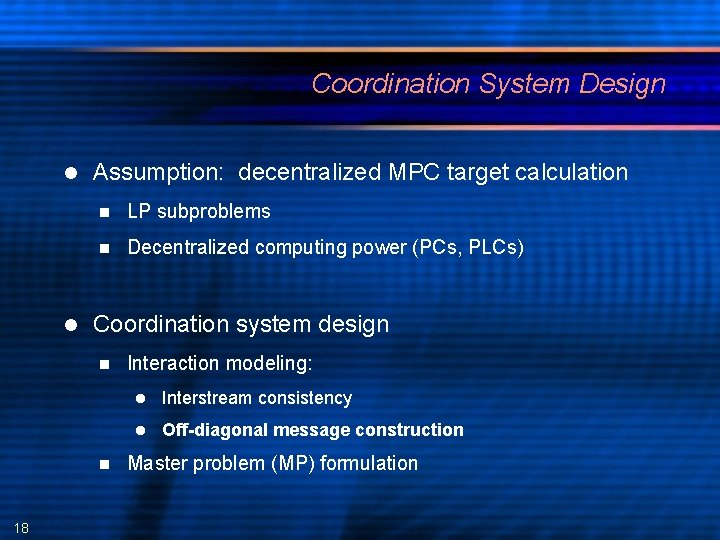
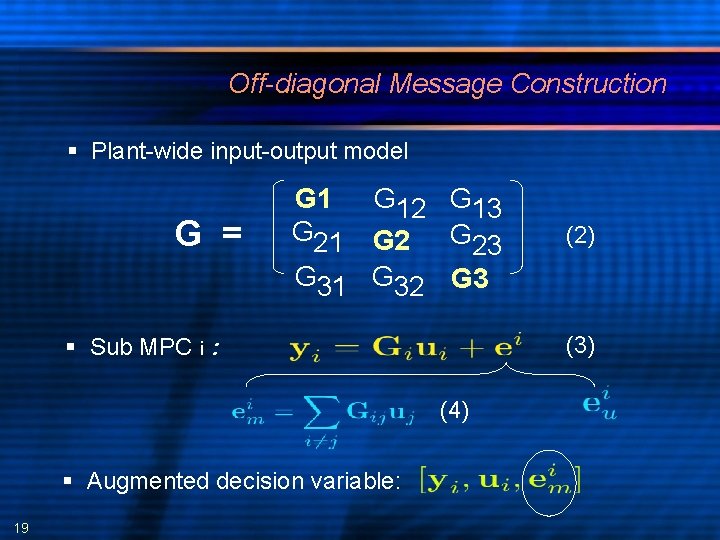
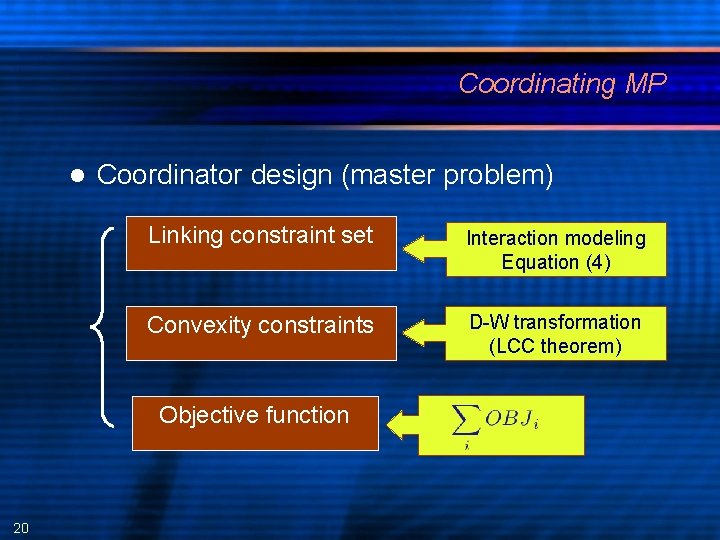
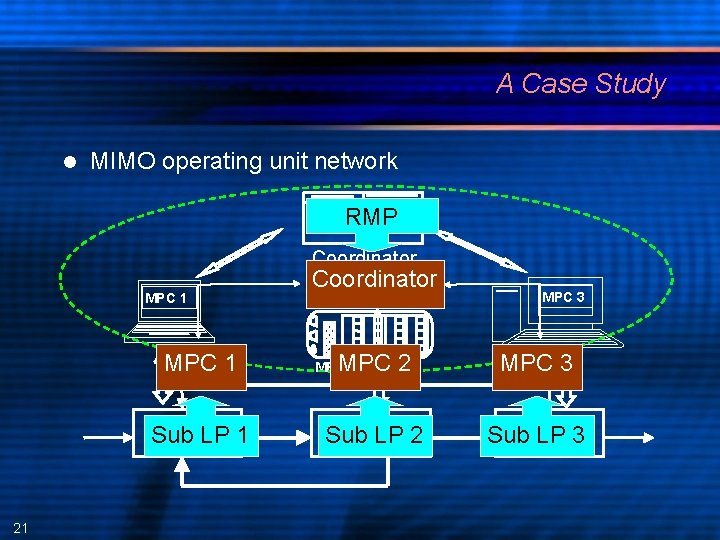
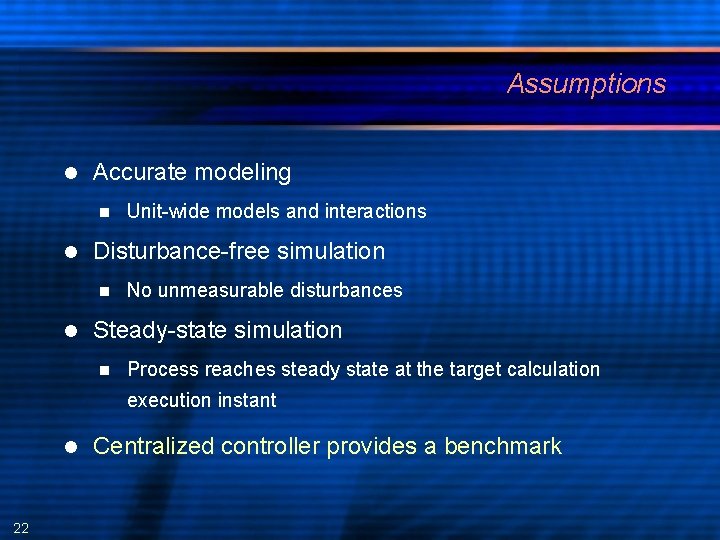
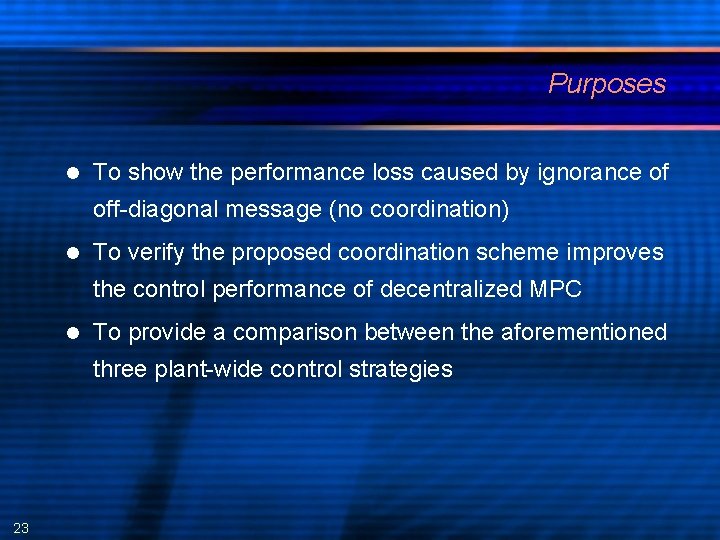
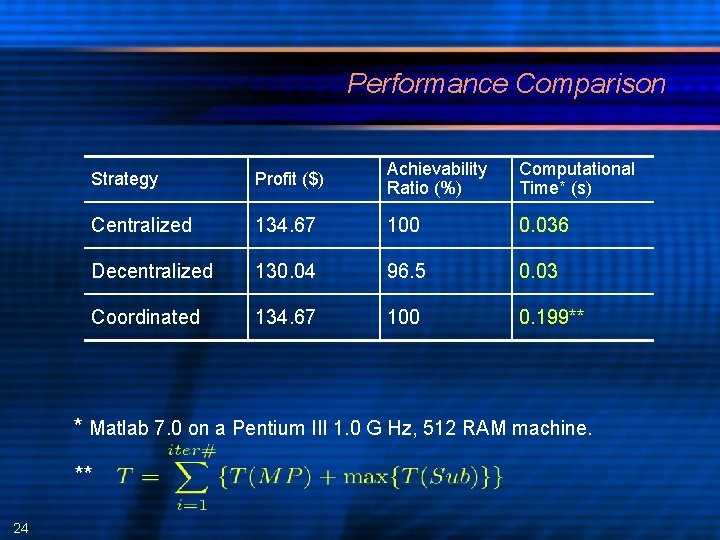
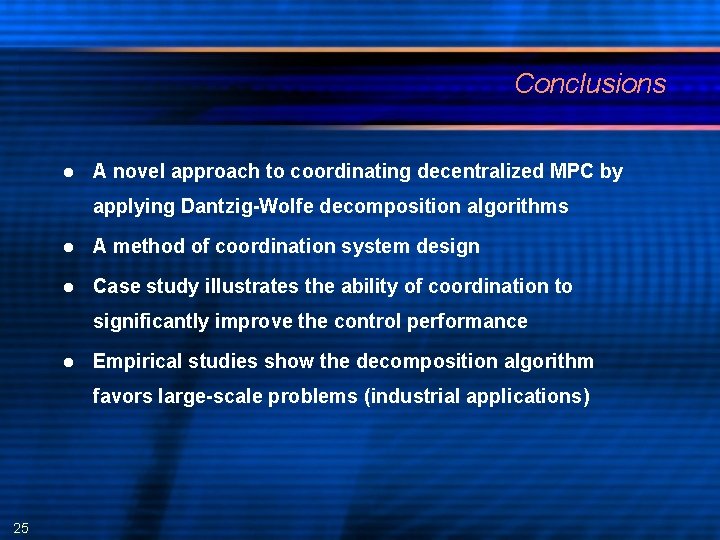
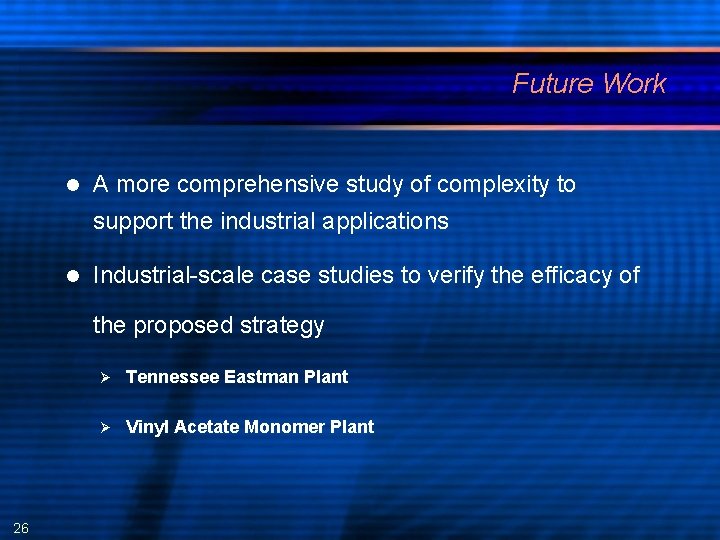
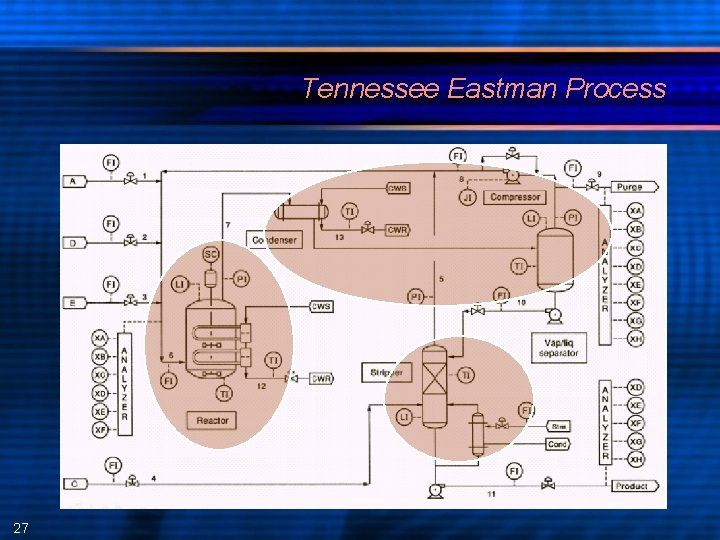
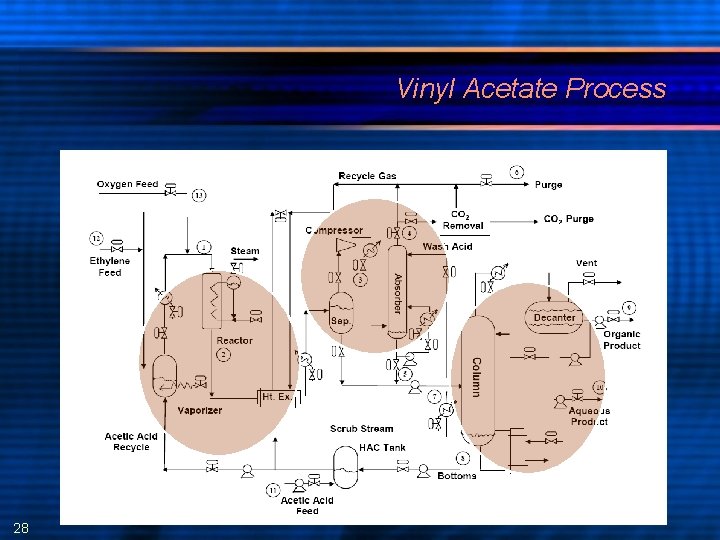
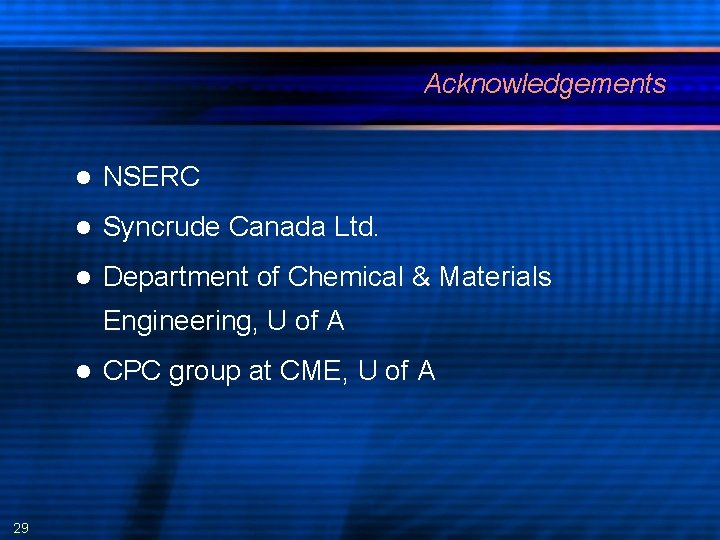
- Slides: 29
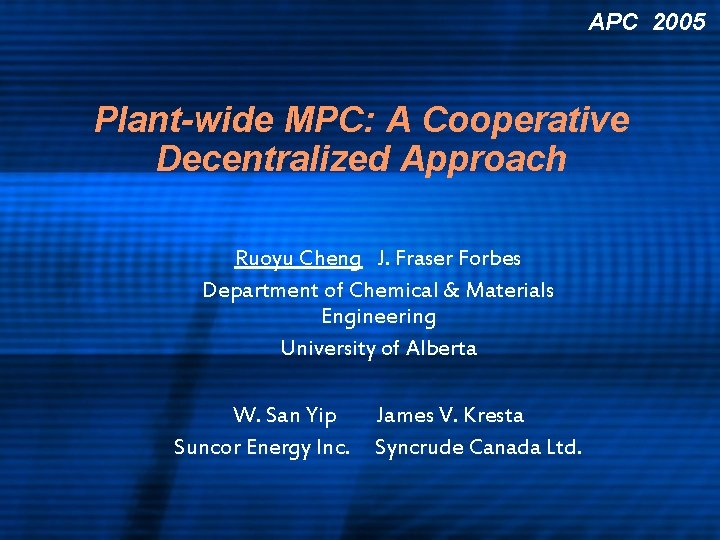
APC 2005 Plant-wide MPC: A Cooperative Decentralized Approach Ruoyu Cheng J. Fraser Forbes Department of Chemical & Materials Engineering University of Alberta W. San Yip Suncor Energy Inc. James V. Kresta Syncrude Canada Ltd.
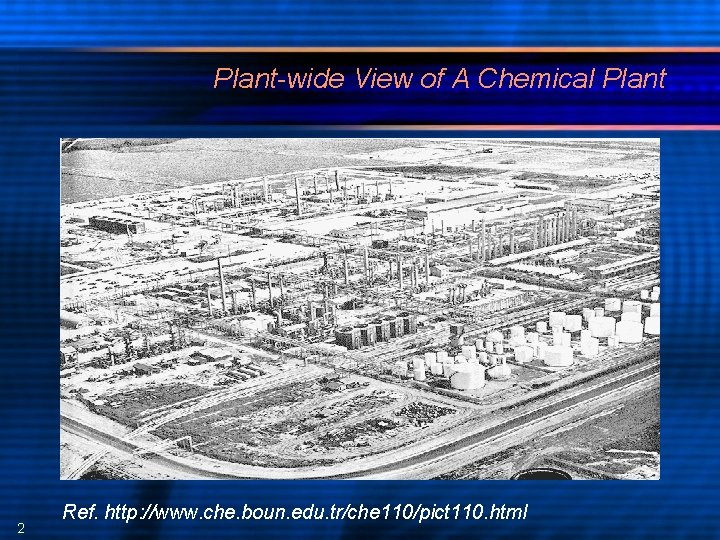
Plant-wide View of A Chemical Plant 2 Ref. http: //www. che. boun. edu. tr/che 110/pict 110. html
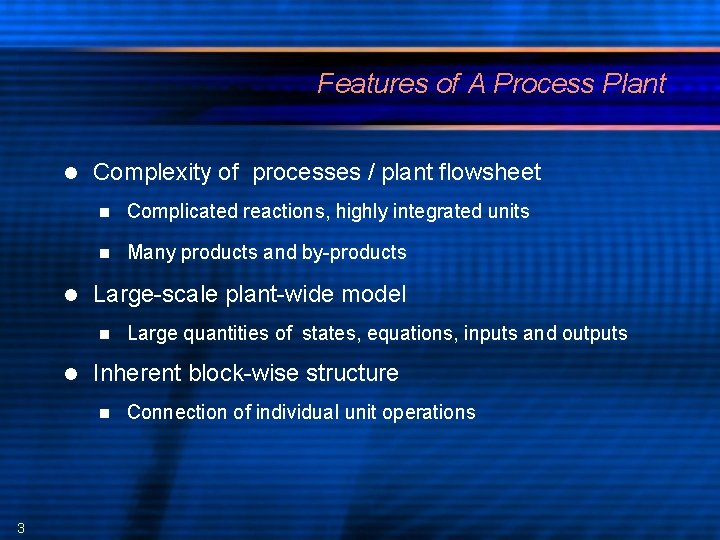
Features of A Process Plant Complexity of processes / plant flowsheet Complicated reactions, highly integrated units Many products and by-products Large-scale plant-wide model Inherent block-wise structure 3 Large quantities of states, equations, inputs and outputs Connection of individual unit operations
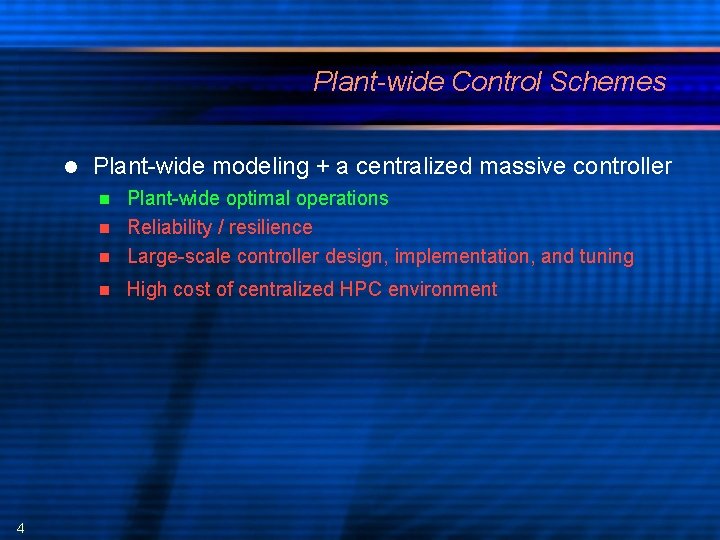
Plant-wide Control Schemes Plant-wide modeling + a centralized massive controller Plant-wide optimal operations Reliability / resilience Large-scale controller design, implementation, and tuning 4 High cost of centralized HPC environment
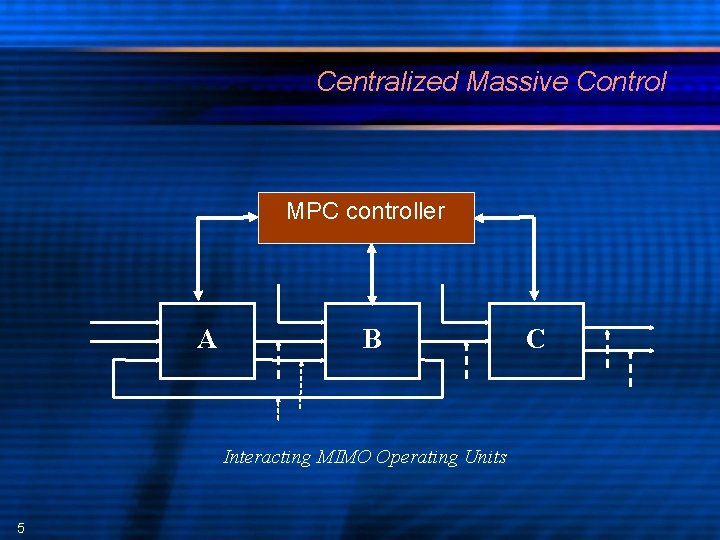
Centralized Massive Control MPC controller A B Interacting MIMO Operating Units 5 C
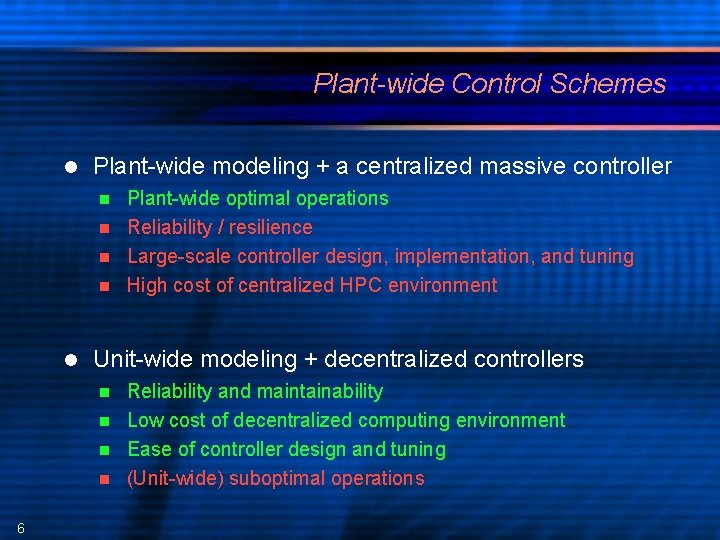
Plant-wide Control Schemes Plant-wide modeling + a centralized massive controller Plant-wide optimal operations Reliability / resilience Large-scale controller design, implementation, and tuning High cost of centralized HPC environment Unit-wide modeling + decentralized controllers Reliability and maintainability Low cost of decentralized computing environment Ease of controller design and tuning (Unit-wide) suboptimal operations 6
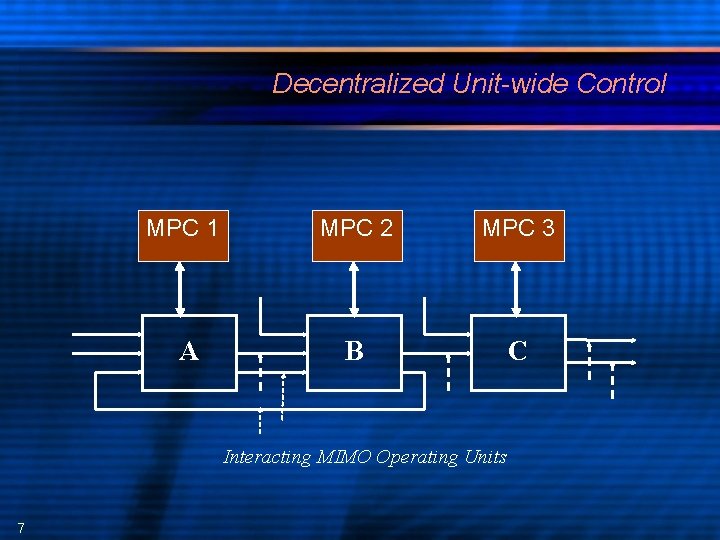
Decentralized Unit-wide Control MPC 1 MPC 2 MPC 3 A B C Interacting MIMO Operating Units 7
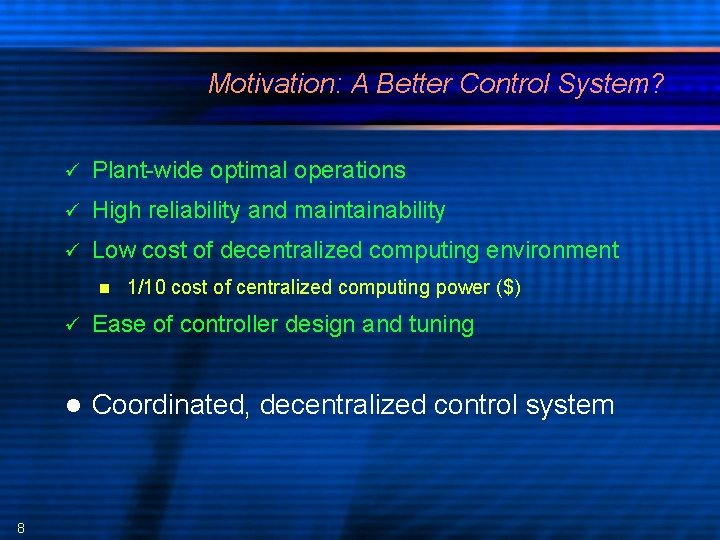
Motivation: A Better Control System? Plant-wide optimal operations High reliability and maintainability Low cost of decentralized computing environment 8 1/10 cost of centralized computing power ($) Ease of controller design and tuning Coordinated, decentralized control system
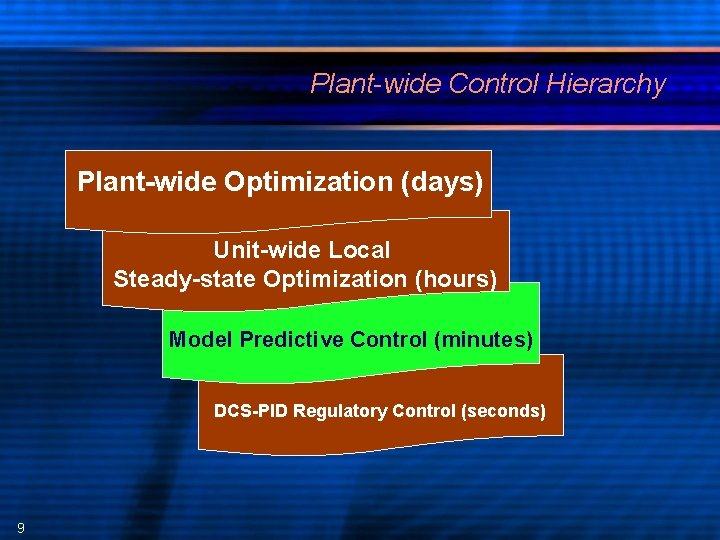
Plant-wide Control Hierarchy Plant-wide Optimization (days) Unit-wide Local Steady-state Optimization (hours) Model Predictive Control (minutes) DCS-PID Regulatory Control (seconds) 9
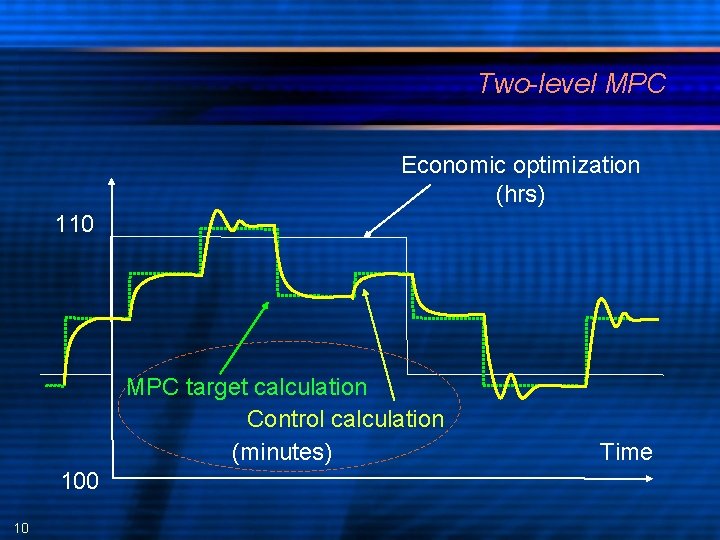
Two-level MPC Economic optimization (hrs) 110 MPC target calculation Control calculation (minutes) 100 10 Time
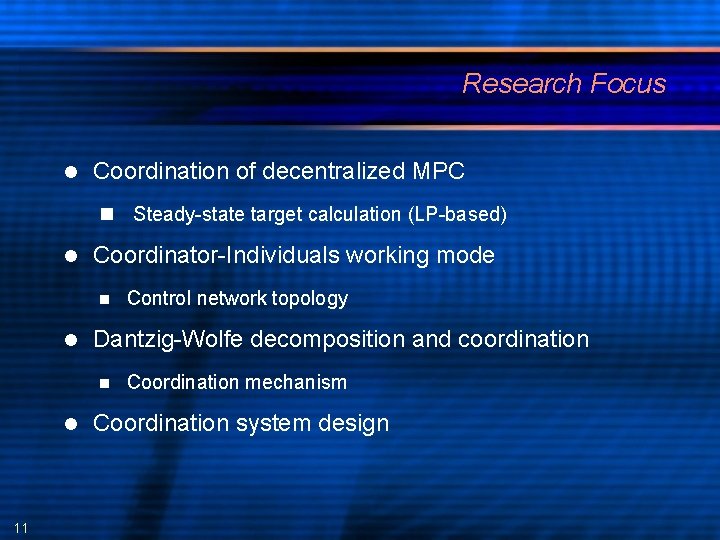
Research Focus Coordination of decentralized MPC Steady-state target calculation (LP-based) Coordinator-Individuals working mode Dantzig-Wolfe decomposition and coordination 11 Control network topology Coordination mechanism Coordination system design
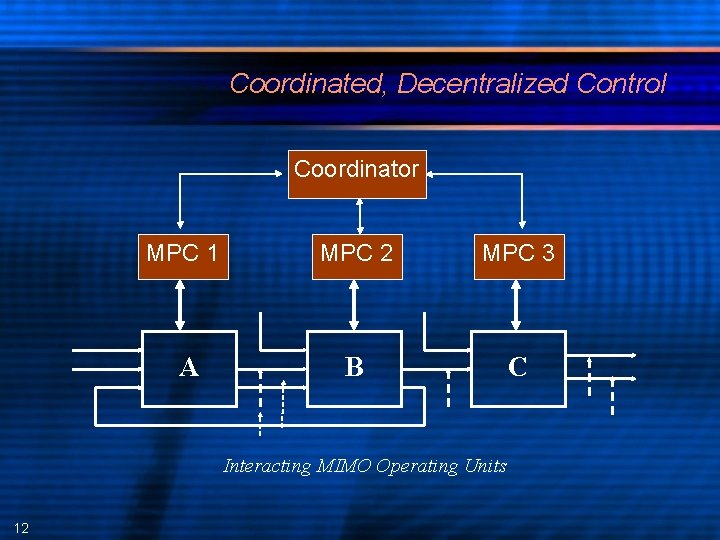
Coordinated, Decentralized Control Coordinator MPC 1 MPC 2 MPC 3 A B C Interacting MIMO Operating Units 12
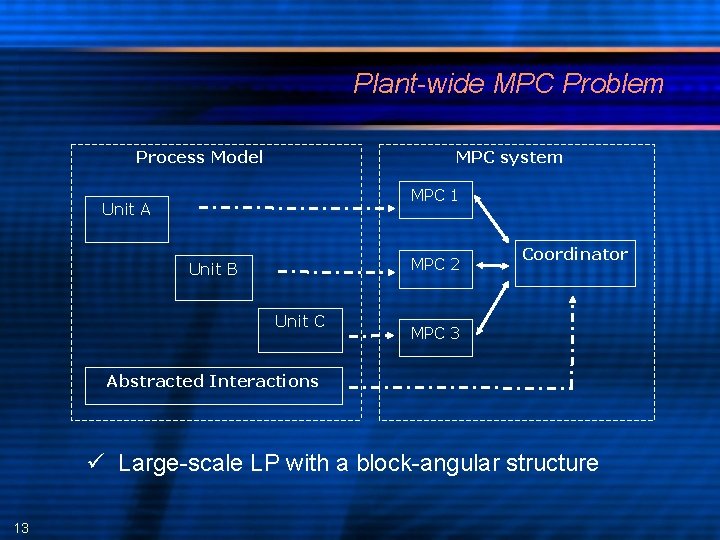
Plant-wide MPC Problem Process Model MPC system MPC 1 Unit A MPC 2 Unit B Unit C Coordinator MPC 3 Abstracted Interactions Large-scale LP with a block-angular structure 13
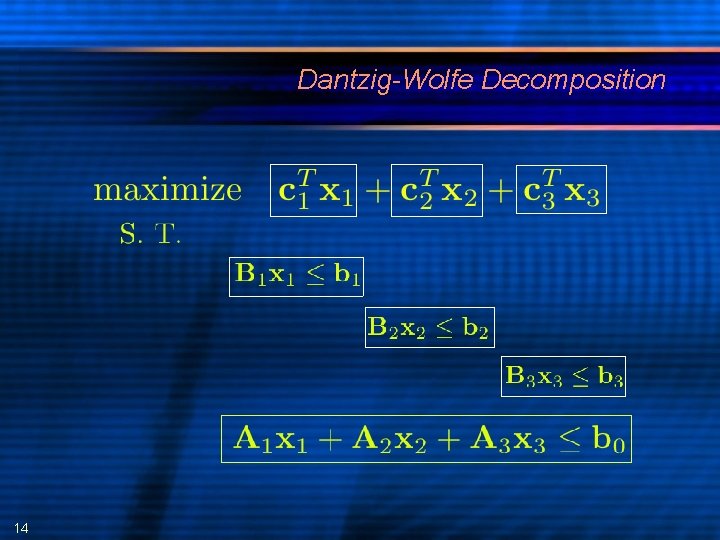
Dantzig-Wolfe Decomposition 14
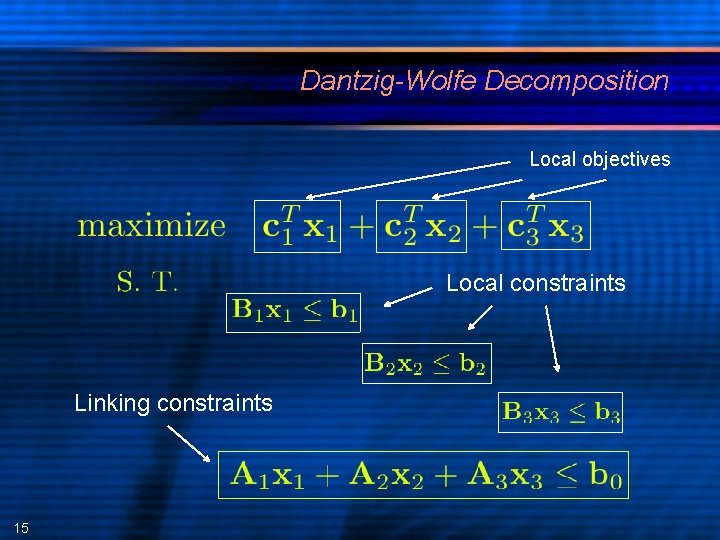
Dantzig-Wolfe Decomposition Local objectives Local constraints Linking constraints 15
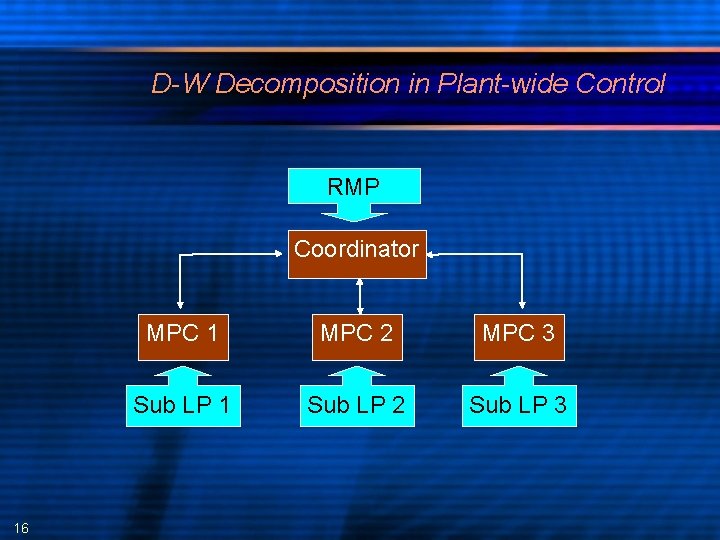
D-W Decomposition in Plant-wide Control RMP Coordinator 16 MPC 1 MPC 2 MPC 3 Sub LP 1 Sub LP 2 Sub LP 3
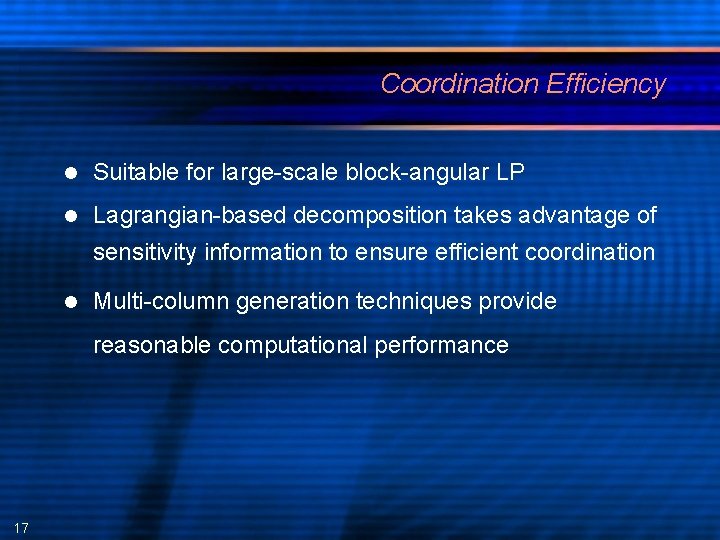
Coordination Efficiency Suitable for large-scale block-angular LP Lagrangian-based decomposition takes advantage of sensitivity information to ensure efficient coordination Multi-column generation techniques provide reasonable computational performance 17
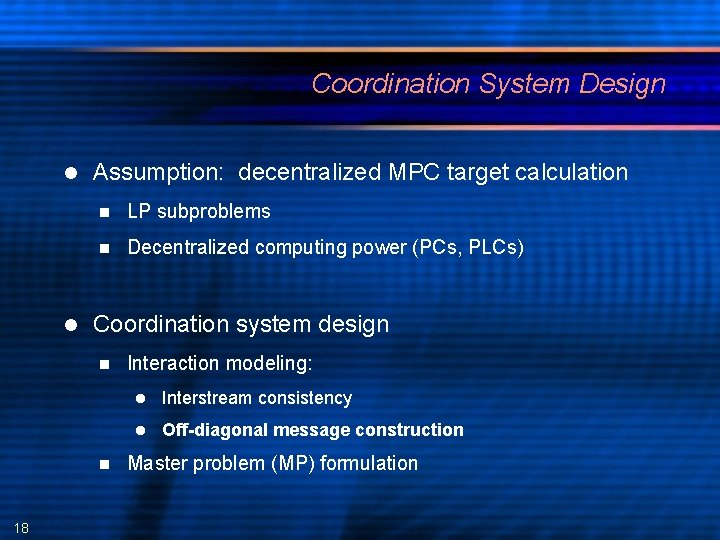
Coordination System Design Assumption: decentralized MPC target calculation LP subproblems Decentralized computing power (PCs, PLCs) Coordination system design 18 Interaction modeling: Interstream consistency Off-diagonal message construction Master problem (MP) formulation
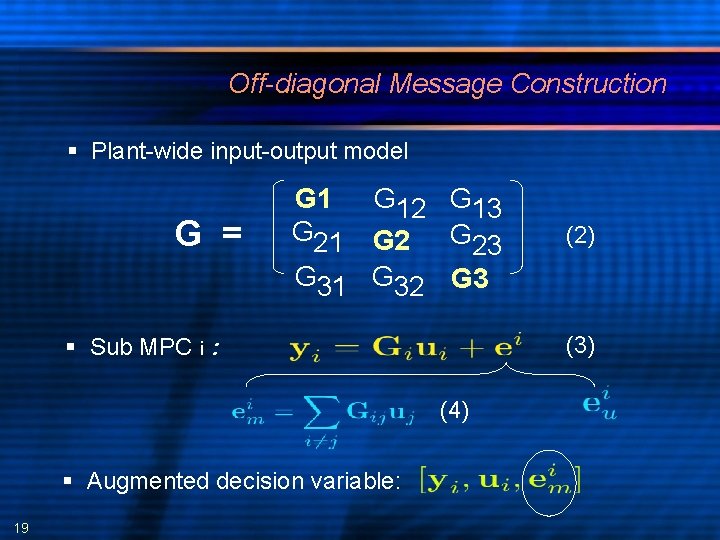
Off-diagonal Message Construction Plant-wide input-output model G = G 1 G 21 G 12 G 13 G 23 G 31 G 32 G 3 (3) Sub MPC i : (4) Augmented decision variable: 19 (2)
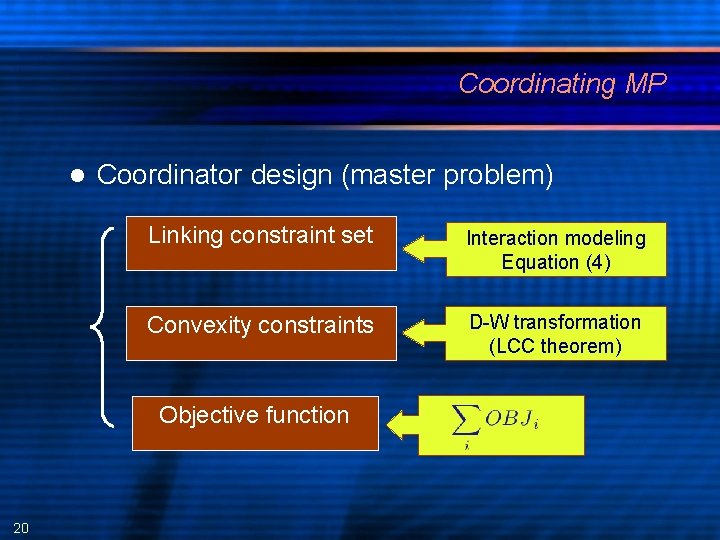
Coordinating MP Coordinator design (master problem) Linking constraint set Interaction modeling Equation (4) Convexity constraints D-W transformation (LCC theorem) Objective function 20
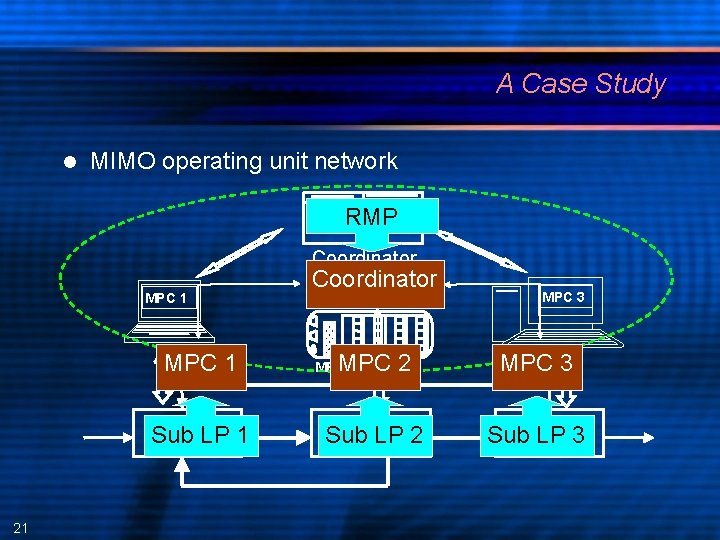
A Case Study MIMO operating unit network RMP Coordinator MPC 1 Sub A LP 1 21 Coordinator MPC 3 MPC 2 MPC 3 Sub. BLP 2 Sub LP C 3 MPC 2
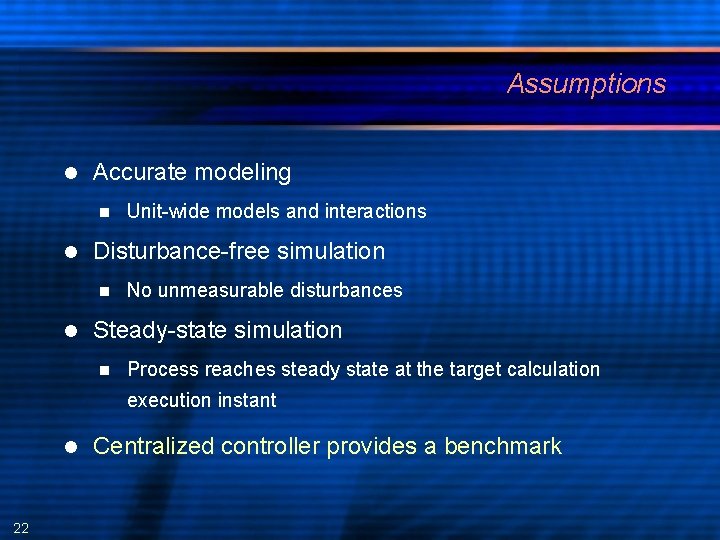
Assumptions Accurate modeling Disturbance-free simulation Unit-wide models and interactions No unmeasurable disturbances Steady-state simulation Process reaches steady state at the target calculation execution instant 22 Centralized controller provides a benchmark
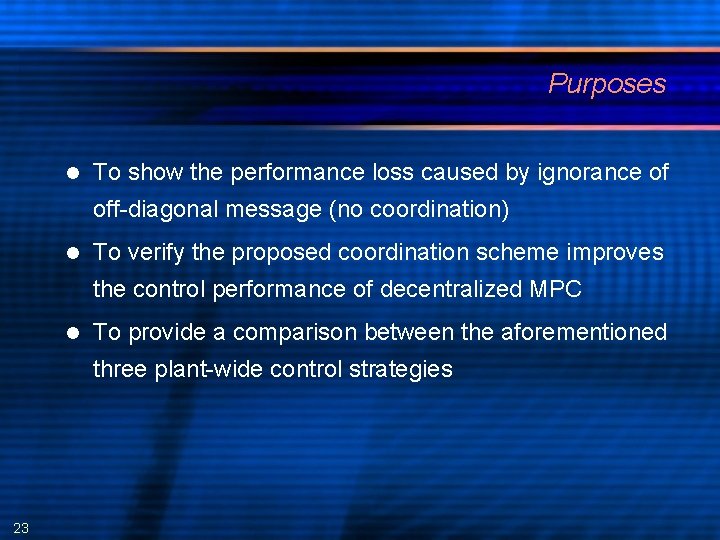
Purposes To show the performance loss caused by ignorance of off-diagonal message (no coordination) To verify the proposed coordination scheme improves the control performance of decentralized MPC To provide a comparison between the aforementioned three plant-wide control strategies 23
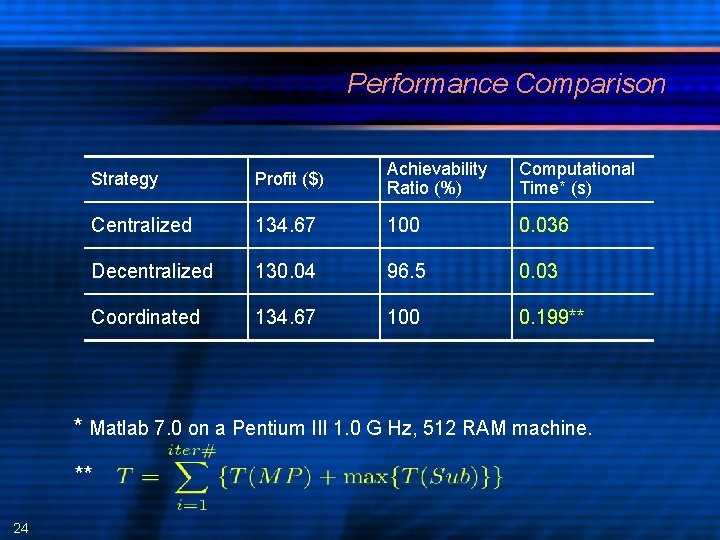
Performance Comparison Strategy Profit ($) Achievability Ratio (%) Computational Time* (s) Centralized 134. 67 100 0. 036 Decentralized 130. 04 96. 5 0. 03 Coordinated 134. 67 100 0. 199** * Matlab 7. 0 on a Pentium III 1. 0 G Hz, 512 RAM machine. ** 24
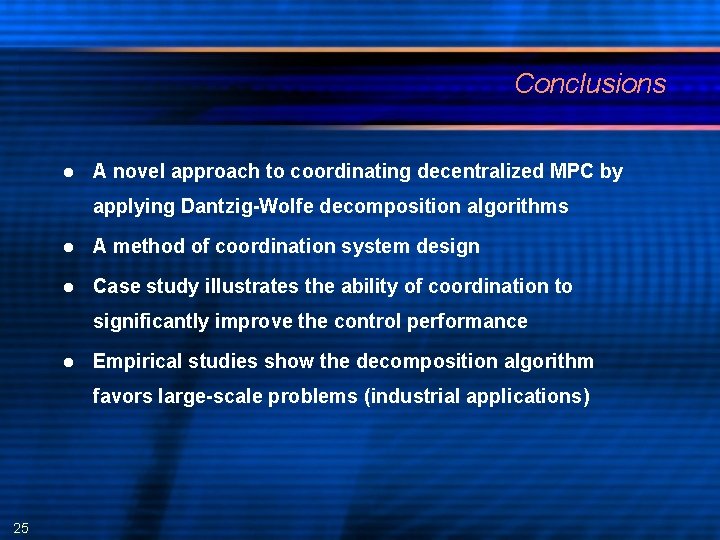
Conclusions A novel approach to coordinating decentralized MPC by applying Dantzig-Wolfe decomposition algorithms A method of coordination system design Case study illustrates the ability of coordination to significantly improve the control performance Empirical studies show the decomposition algorithm favors large-scale problems (industrial applications) 25
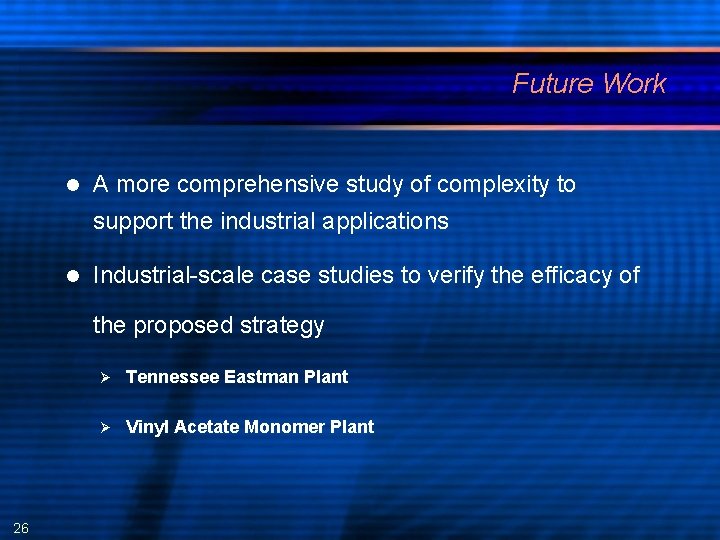
Future Work A more comprehensive study of complexity to support the industrial applications Industrial-scale case studies to verify the efficacy of the proposed strategy 26 Ø Tennessee Eastman Plant Ø Vinyl Acetate Monomer Plant
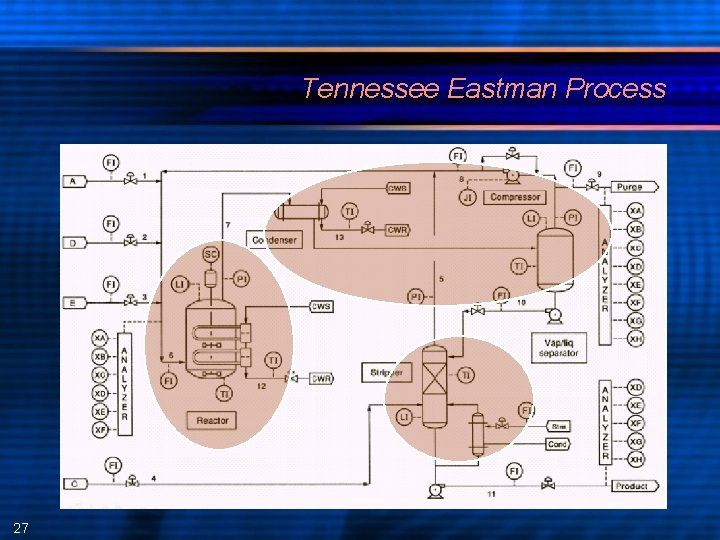
Tennessee Eastman Process 27
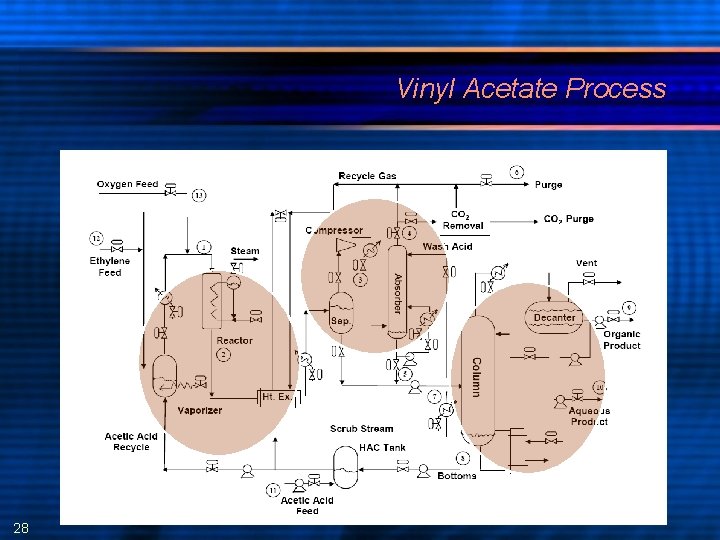
Vinyl Acetate Process 28
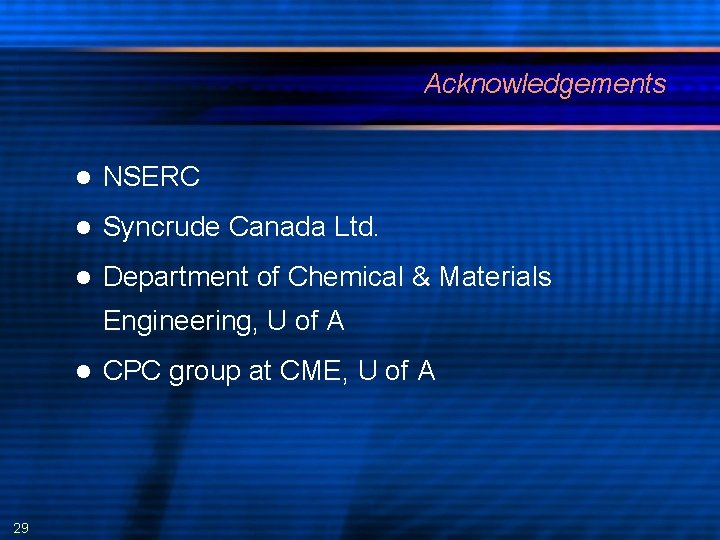
Acknowledgements NSERC Syncrude Canada Ltd. Department of Chemical & Materials Engineering, U of A 29 CPC group at CME, U of A